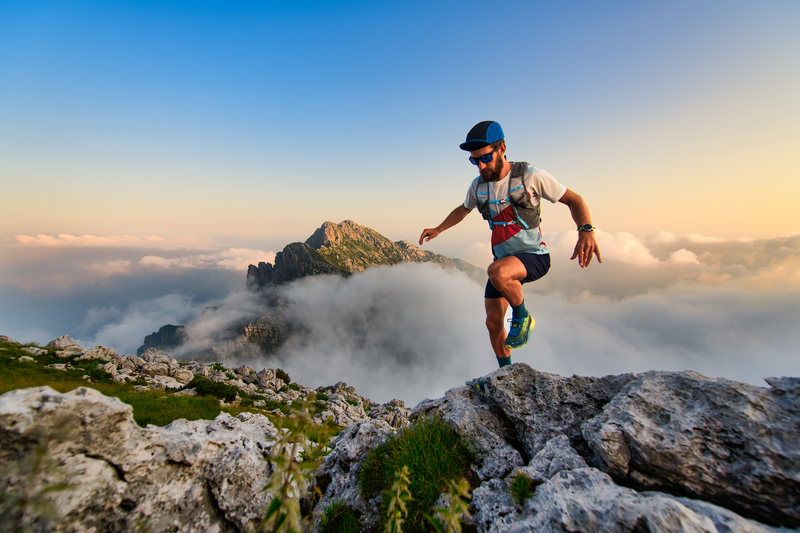
94% of researchers rate our articles as excellent or good
Learn more about the work of our research integrity team to safeguard the quality of each article we publish.
Find out more
ORIGINAL RESEARCH article
Front. Endocrinol. , 25 September 2018
Sec. Systems Endocrinology
Volume 9 - 2018 | https://doi.org/10.3389/fendo.2018.00564
Introduction: Published data regarding the association between solute carrier family 30, member 8 (SLC30A8) rs13266634 polymorphism and type 2 diabetes mellitus (T2DM) and impaired glucose regulation (IGR) risks in Chinese population are in-consistent. The purpose of this meta-analysis was to evaluate the association between SLC30A8 rs13266634 and T2DM/IGR in a Chinese population.
Material and Methods: Three English (PubMed, Embase, and Web of Science) and three Chinese databases (Wanfang, CNKI, and CBMD database) were used for searching articles from January 2005 to January 2018. Odds ratio (OR) and 95% confidence interval (95%CI) were calculated with the random-effect model. Trial sequential analysis was also utilized.
Results: Twenty-eight case-control studies with 25,912 cases and 26,975 controls were included for SLC30A8 and T2DM. Pooled risk allele C frequency for rs13266634 was 60.6% (95%CI: 59.2–62.0%) in the T2DM group and 54.8% (95%CI: 53.2–56.4%) in the control group which had estimated OR of 1.23 (95%CI: 1.17–1.28). Individuals who carried major homozygous CC and heterozygous CT genotype were at 1.51 and 1.23 times higher risk of T2DM, respectively, than those carrying minor homozygous TT. The most appropriate genetic analysis model was the co-dominant model based on comparison of OR1, OR2 and OR3. Five articles that involved 4,627 cases and 6,166 controls were included for SLC30A8 and IGR. However, no association was found between SLC30A8 rs13266634 and IGR (C vs. T, OR = 1.13, 95%CI: 0.98–1.30, p = 0.082). TSA revealed that the pooled sample sizes of T2DM exceeded the estimated required information size but not the IGR.
Conclusion: The present meta-analysis demonstrated that SLC30A8 rs13266634 was a potential risk factor for T2DM, and more studies should be performed to confirm the association between rs13266634 polymorphism and IGR.
Type 2 diabetes mellitus (T2DM) is an expanding global health problem (1). There are 347 million people worldwide with diabetes, and more than 80% of diabetes deaths occur in low- and middle-income countries. According to statistics, 9.7% of people in China have type 2 diabetes, which not only threatens health, but also reduces quality of life and life expectancy (2). The onset of T2DM is multifactorial due to the interplay of common variation in multiple genes and environmental factors, but the exact pathogenesis of T2DM remains unclear (3).
Before type 2 diabetes occurs, glucose control is altered, which is reflected by higher fasting glucose and/or higher post-prandial glucose. This phenomenon is called impaired glucose regulation (IGR) and IGR is regarded as pre-diabetic state that includes impaired fasting glucose (IFG) and/or impaired glucose tolerance (IGT) (4). High glucose may cause an adverse effect on insulin sensitivity and secretion, further developing into glucotoxicity (5). Previous studies have shown that 5–10% IGT individuals develop diabetes each year, although some revert to normal glucose tolerance (6).
Recent developments in the understanding of T2DM have been heightened by the potential relevance of dysfunctional zinc signaling in this disease. Zinc is an important element for insulin storage and secretion (7). Zinc transporter 8 (ZnT-8), a member of the zinc transporter family, has been shown to bind with insulin in beta cells to form a solid hexamer, which is stored in secretory vesicles (8). Zinc transporter solute carrier family 30 member 8 (SLC30A8) is located on chromosome 8q24.11. It encodes ZnT-8, which is highly expressed in pancreatic islets and beta cells. ZnT-8 transports zinc from the cytoplasm into insulin secretary vesicles. Some studies have shown that the polymorphisms of SLC30A8 are associated with β-cell function and insulin release in vivo (9, 10) and in vitro (11).
The non-synonymous single-nucleotide polymorphism, rs13266634, of SLC30A8 causes an amino acid change from arginine (R) to tryptophan (W) at position 325 (Arg325Trp). The association of rs13266634 polymorphism in IGR and T2DM has been demonstrated in different ethnic groups via GWAS (12–16). The major C allele of the rs13266634 polymorphism is strongly associated with Chinese IGR and T2DM patients. However, the results are contradictory, and not all variants associated with type 2 diabetes are related with impaired glucose. These different results might be due to racial and regional differences, and they may be due to the limitation of the number of patients per study. To reduce the influence of diverse genetic backgrounds, a meta-analysis based on the Chinese population was performed to assess the relationship between rs13266634 polymorphism and IGR/T2DM.
We searched the PubMed, Embase, Web of Science, China National Knowledge Infrastructure (CNKI), Wan Fang and CBMD databases for articles published prior to January 2018. The searching languages contained both English and Chinese, and only published studies were considered. The search strategy was based on combination of “SLC30A8,” “rs13266634,” “polymorphism*,” “variant*,” “genotype*,” “diabetes,” “T2DM,” “impaired glucose regulation,” “IGR,” “Chinese,” and “China.” References of retrieved articles were also screened and selected.
Studies included in the meta-analysis met all of the following criteria: (1) the association between SLC30A8 rs13266634 polymorphism and T2DM/IGR; (2) Chinese population; (3) sufficient data about allele or genotype frequency in cases and controls; (4) providing the odds ratio (OR) and its 95% confidence interval (95%CI) of the polymorphism; and (5) and clear diagnosis of T2DM/IGR. Studies were excluded if genotype frequency data in the controls demonstrated departure from Hardy-Weinberg equilibrium (HWE). When the same data were included in more than one publication, only the most relevant articles with the largest data set were included in the final analysis.
The following data elements from each study were extracted: name of first author, year of publication, region of the study population, ethnicity of Chinese population, source of control, genotype method, diagnostic criteria, risk allele (C allele) frequency, number of cases and controls, mean (or median) body mass index (BMI), percentage of men in cases and controls, and mean (or median) age. Data were independently extracted by two investigators who reached a consensus on all of the items. If there was a lack of genotype information, we contacted the corresponding author to obtain required data.
The quality of studies was also independently assessed by two reviewers (DF and ZFF) based on a risk of bias assessment for genetic association study which was modified on the basis of both traditional epidemiologic considerations and genetic issues that were developed by Thakkinstian et al. (17, 18). The score was divided into five domains, including information bias, confounding bias, selective reporting of outcomes, population stratification, and Hardy-Weinberg equilibrium (HWE) in the control group. Quality scores of each study ranged from 0 (lowest) to 15 (highest). Studies with scores ≤10 were categorized into low quality, while those with scores >10 were considered as high quality. Disagreement between the two reviewers was solved by a senior reviewer (JCX).
We used Stata 11.0 (College Station, TX, USA) for all statistical analyses. The HWE was examined in control groups by Fisher's Exact Test. If the study was found not to be in HWE with P-value <0.05, it was considered to be disequilibrium. Both per-allele and per-genotype approaches were performed to estimate the effect of the rs13266634 polymorphism on the risk of T2DM or IGR.
Per-allele analysis: The risk allele C frequency for rs13266634 was estimated for each study by reported data, and the overall prevalence and 95% confidence interval (95%CI) were estimated. Odds ratios (ORs), as well as 95%CI, were also estimated.
Per-genotype analysis: The genotype effect was also calculated if the genotype data could be extracted from the study. Three odds ratios were estimated: CC vs. TT (OR1), CT vs. TT (OR2), and CC vs. CT (OR3). If the main effect of the genotype was statistically significant, further comparisons of OR1, OR2 and OR3 were explored. These pairwise differences were used to indicate the most appropriate genetic model as follows: (1). If OR1 = OR3≠1 and OR2 = 1, a recessive model was suggested. (2). If OR1 = OR2 ≠ 1 and OR3 = 1, then a dominant model was suggested. (3). If OR2 = 1/OR3 ≠ 1 and OR1 = 1, then a complete overdominant model is suggested. (4). If OR1 > OR2 > 1 and OR1 > OR3 > 1 (or OR1 < OR2 < 1 and OR1 < OR3 < 1), a co-dominant model was suggested.
The estimation of the allele or genotype effect on T2DM was calculated by an OR and 95%CI. The Z-test was used to determine the statistical significance of the pooled OR, and its P-value was used to determine if the overall SNP effect was significant (α = 0.05). The Q test based on a Chi-square analysis was used to assess the existence of heterogeneity. p = 0.01 was selected as the boundary value of the judgment to minimize type 2 errors (19). The pooled OR with 95% CI was calculated using the random-effects model based on the DerSimonian and Laird method (20). The random-effects model was chosen a priori because it is considered as more conservative than the fixed-effects model, as it accounts for both within- and between-study heterogeneity (21). In addition, the degree of heterogeneity was quantified using I2 (I2 < 25%, no heterogeneity; 25% < I2 < 50%, moderate heterogeneity; 50% < I2 < 75%, large heterogeneity; and I2 > 75% extreme heterogeneity) (22). A random-effects model was selected if I2 was >50%. The cause of heterogeneity was explored by fitting covariates (i.e., age, body mass index, percentage of men, source of control, genotype method, or sample size) in a meta-regression if these data were available. A subgroup analysis was performed according to publication year, source of control, sample size, and quality scores.
A sensitive analysis with a single study being removed each time was performed to reflect the influence of the individual data set on the pooled OR. Publication bias was evaluated using Egger's linear regression asymmetry test and visual inspection of funnel plots, and the influence of potential publication bias on results was explored by using the Duval and Tweedie trim-and-fill procedure (23, 24). P < 0.05 was considered statistically significant in all analyses, except for the Egger test (p < 0.10) because of the low power of the test.
Meta-analyses might result in type-I errors owing to an increased risk of random error when fewer patients are involved, and due to continuous significance testing when a cumulative meta-analysis is updated with new studies (25, 26). Therefore, to assess the risks of random errors, trial sequential analysis (TSA) was performed using Stata 11.0 software package (metacumbounds command), which combines information size estimation for meta-analysis (cumulated sample size of included trials) with an adjusted threshold for statistical significance in the cumulative meta-analysis. TSA was conducted with the intention to maintain an overall 5% risk of a type I error and a power of 80%. For the calculation of the required information size, an intervention effect of a 20% relative risk reduction (RRR) was anticipated using the control event proportion calculated from the actual meta-analyses.
A total of 381 articles were retrieved by literature search (Figure 1). After removal of 124 duplicates, 257 studies were screened for title and abstract as well as full text with 38 articles determined to be eligible. The following 10 studies were further excluded: five duplicate publications (27–31); four articles (32–35) with controls not in HWE; and one study (36) that reported data in combination with T2DM and IGR cases. Finally, 28 case-control studies with 25,912 cases and 26,975 controls were associated with T2DM, and five studies with 4,627 cases and 6,166 controls were associated with IGR. The characteristics and genotype distribution of included studies are listed in Table 1; Supplementary Table 1.
Per-allele analysis: The pooled allele frequency was calculated in both case and control groups. The risk allele C frequency was 60.6% (95%CI: 59.2–62.0%) in the T2DM group with high heterogeneity (I2 = 88.8%, p < 0.001) and 54.8% (95%CI: 53.2–56.4%) in the control group (I2 = 91.5%, p < 0.001). The odds ratio (C vs. T) was largely heterogenous (χ2 = 34.24, p = 0.008, I2 = 50.35) with a pooled odds ratio of 1.23 (95%CI: 1.17–1.28), and the overall SNP effect estimated by the random effect model was significant (p < 0.001; Table 2; Figure 2). These results suggested that individuals carrying the major C allele had 23% increased risk of developing T2DM than those carrying the minor T allele.
Table 2. Total and stratified analyses of SLC30A8 rs13266634 polymorphism and T2DM risk among Chinese.
Figure 2. Forest plot of association between SLC30A8 rs13266634 polymorphism and T2DM risk in C vs. T model.
The pooled OR and 95% CI for the association between the rs13266634 polymorphism and T2DM risk was 1.51 (1.38, 1.65; p < 0.001) with large between-study heterogeneity (I2 = 53.9%) for CC vs. TT, 1.23 (1.15, 1.30; p < 0.001) with small heterogeneity (I2 = 19.4%) for CT vs. TT, and 1.19 (1.14, 1.25; p < 0.001) with small heterogeneity (I2 = 12.2%) for CC vs. CT, which suggests a co-dominant model (CC vs. CT vs. TT) for the putative susceptibility allele C with T2DM (Table 2).
Stratified analysis indicated significantly stronger associations among controls coming from hospitals, among studies with lower number of participants, and among studies with lower quality for C vs. T (OR for controls from hospitals vs. populations: 1.35 vs. 1.17, p interaction = 0.008; OR for studies with sample size < 1,000 vs. ≥1,000: 1.40 vs. 1.19, p interaction = 0.023; OR for studies with quality score < 10 vs. ≥10: 1.35 vs. 1.18, p interaction = 0.018), for CC vs. TT (OR for controls from hospitals vs. populations: 1.88 vs. 1.36, p interaction = 0.002; OR for studies with sample size < 1,000 vs. ≥1,000: 1.51 vs. 1.32, p interaction = 0.018; OR for studies with quality score < 10 vs. ≥10: 1.51 vs. 1.30, p interaction = 0.009), and for CC vs. CT (OR for controls from hospitals vs. populations: 1.45 vs. 1.16, p interaction = 0.006; OR for studies with sample size < 1,000 vs. ≥1,000: 1.48 vs. 1.19, p interaction = 0.049; OR for studies with quality score < 10 vs. ≥10: 1.42 vs. 1.18, p interaction = 0.029; Table 2). Meta-regression further confirmed the effect of the source of control and quality score, but not total sample size, for C vs. T, CC vs. TT, and CC vs. CT comparisons (Supplementary Table 2). Influence analyses by removing one study each time revealed that the pooled ORs remained significant for all comparisons (Table 2).
Egger's test showed significant evidence of publication bias (C vs. T: p = 0.014; CC vs. TT: p = 0.008; CC vs. TT: p = 0.015; CC vs. TT: p = 0.090), and the funnel plots for all comparisons were asymmetric. However, after imputing 6, 6, 4, and 1 missing studies for C vs. T, CC vs. TT, CC vs. TT, and CC vs. TT, respectively, by using the trim-and-fill method, the recalculated pooled ORs were not substantially different from the initial (Figure 3; Supplementary Table 3).
Figure 3. Trill and fill plot of associations between SLC30A8 rs13266634 polymorphism and T2DM risk in C vs. T model.
Trial sequential analyses: Because both the monitoring boundaries and information size had a cumulative Z-statistic >1.96, the evidence confirmed a risk effect of C allele on prevalence of T2DM (Figure 4).
Figure 4. Trial sequential analysis for association between SLC30A8 rs13266634 polymorphism and T2DM risk in C vs. T model.
The pooled frequency of the major C allele was estimated to be 57.2% (95%CI: 55.8–58.7%) with moderate heterogeneity (I2 = 43.0%) in IGR group, and it was 54.0% (95%CI: 50.5–57.5%; I2 = 90.0%) in the control group. The pooled OR of C vs. T was 1.13 (95% CI: 0.98–1.30; p = 0.082) with significant between-study heterogeneity (I2 = 81.0%; Figure 5). Egger's test (p = 0.257) suggested that there was no publication bias.
Figure 5. Forest plot of association between SLC30A8 rs13266634 polymorphism and IGR risk in C vs. T model.
Per-genotype analysis also indicated that there were no significant associations between rs13266634 polymorphism and IGR risk (OR1: 1.27, 0.96–1.68, p = 0.089; OR2: 1.13, 0.95–1.34, p = 0.169; OR3: 1.08, 0.95–1.21, p = 0.243; Table 2).
Trial sequential analyses: because the cumulative z-curve fluctuated around both the traditional boundary and the trial sequential monitoring boundary, the evidence was not conclusive for the outcome (Figure 6).
Figure 6. Trial sequential analysis for association between SLC30A8 rs13266634 polymorphism and IGR risk in C vs. T model.
A systematic review and meta-analysis was performed to investigate the association between SLC30A8 rs13266634 and T2DM and IGR in a Chinese population, including 25,912 cases and 26,975 controls from 28 studies associated with T2DM, as well as 4,627 cases and 6,166 controls from five studies associated with IGR. The results suggested a significant association between the rs13266634 polymorphism and T2DM, which is consistent with previous results (64) but different from a study in Arab ethnicity (65). This association was also reported as significant in an African population under the allelic model, but neither under the codominant or recessive model (66). The expression differences of the same polymorphism between different ethnic groups might be caused by different genetic backgrounds and various environmental factors (67). In the present meta-analysis, individuals who carried the C allele had 23% increased risk of developing T2DM relative to those carrying the T allele in the Chinese ethnicity. These results were consistent but slightly higher than the GWAS database, which reports that people carrying the C allele may have an increased 18% risk of developing T2DM in a Finnish population (13). The genotype effect calculation showed that people with homozygous and heterozygous genotypes had 51 and 23%, respectively, higher risk of developing T2DM. The result agreed with GWAS research showing that homozygous and heterozygous individuals had 53 and 18%, respectively, higher risk of having T2DM (15). The frequency of risk allele C was 54.8% in the healthy Chinese group, which was lower than that in French (69.9%), Austrian (74.03%), and African American populations (91.59%) (15, 68). There were five case-control studies that described the association between the rs13266634 polymorphism and IGR, which included 4,627 cases and 6,166 controls. The evaluation indicated that the rs13266634 polymorphism was not associated with IGR in the Chinese population (OR = 1.13, 95%CI = 0.98–1.30, p = 0.082). It should be noted that this result was not consistent with the study in Europeans (13). These discrepancies may be attributed to the difference between genetic backgrounds of population substructure and sample size.
Zinc is necessary in β-cells for insulin crystallization in hexamers. Zinc is co-secreted with insulin, and participates in the regulation of β-cell mass by antioxidant actions (69, 70). Zinc plays an important role in β-cell function and insulin homeostasis. The ZnT8 transporter is primarily expressed in β-cells and co-localizes with insulin-containing secretory granules (71), and the alteration of ZnT8 expression may modulate insulin secretion. A previously study suggested that the SLC30A8 rs13266634 polymorphism impairs ZnT8 expression in islets by disrupting the protein kinase A and protein kinase C recognition motif (R-X-S/T) in the ZnT8 molecule (7). Recent research has shown that the SLC30A8 variant may affect glucose via modulating total zinc intake (72). These studies provided information for the underlying mechanisms of impaired glucose regulation and T2DM, potentially aiding the development of novel and individualized medical therapies. However, neither environmental triggers nor genetics alone can explain type 2 diabetes as a multifactorial disease. Thus, a close interaction between genetics and environment is presumed. Hence, it is still too early to draw such a conclusion until more functional research and larger population-based validation tests are performed.
Stratified analysis of control source, sample size, and quality score for T2DM showed that a significantly higher risk of T2DM was found in studies conducted using control source from hospitals with sample size <1,000 and with quality score <10. These results suggested that studies using controls from hospitals, small sample size, and low quality tend to overestimate the overall effect. However, subgroup analyses revealed that the risk effect of C allele from studies with controls from population, large sample size, and higher quality persisted. In addition, after excluding studies with controls from hospitals, small sample size, and especially low quality studies, the significant heterogeneity for allele comparison was markedly reduced from 55.5 to 27.1–42.8% for the CC vs. TT comparison as well as from 53.9 to 24.5–36.9% for the C vs. T comparison. The effect of quality score was the most evident because calculation of quality scores considered both the source of controls and sample size.
To the best of our knowledge, this is one of the most comprehensive meta-analysis for the association of SLC30A8 rs13266634 polymorphism in IGR and T2DM risk in a Chinese population. A detailed search strategy was used in multiple databases, which was applied to include as many eligible studies as possible. Data extraction was performed in duplicate, and the qualities of included studies were evaluated by similar scale. Previous studies have confirmed the association between SLC30A8 and T2DM (64, 73), but subgroup analysis was based only on different continents. Thus, it was necessary to perform a meta-analysis in the Chinese population due to its large population base and complicated genetic backgrounds. In addition, TSA was conducted to test if sufficient information size had been reached, minimizing potentially false positive results and providing the basis for further studies. TSA indicated that the cumulative Z-curve of the IGR fluctuated around both the traditional boundary and the trial sequential monitoring boundary, suggesting that additional studies are needed for this endpoint.
There were limitations in our study. First, case-control studies may overestimate the effect size of the association, which make the relationship between exposure and outcome less clear. To avoid such bias, the population should be based on a nested case-control study. Second, there were 19 articles with genotype data (19/28), and the estimation of genotype effects on T2DM may not be strong enough. Thus, a more precise association should be explored with sufficient data. These results should be interpreted with caution until further verification of sequencing approaches and larger meta-analysis are performed. Finally, significant publication bias was observed for all comparisons for T2DM. However, after imputing missing studies by using the trim-and-fill method, the recalculated pooled ORs were not substantially changed.
The present meta-analysis indicated that the rs13266634 C allele in the SLC30A8 gene was associated with T2DM risk in the Chinese population. More studies are needed to confirm the association between rs13266634 polymorphism and IGR risk. The current evidence is insufficient to inform clinical decision making or policies until better understanding of the full functional implications is obtained. In addition, comprehensive analyses of gene-gene and gene-environment interactions should also be evaluated in the future.
CJ, GY, and FZ designed the study. FD and BZ extracted the data. SZ, XH, XD, and KZ performed the analyses. FD and FZ wrote the draft. CJ, GY, FZ, XC, JW, DL, ZW, XZ, YL, and SD revised it.
The authors declare that the research was conducted in the absence of any commercial or financial relationships that could be construed as a potential conflict of interest.
This work was supported in part by Training Program of the Major Research Plan of the National Natural Science Foundation of China (Grant numbers: 91543132), National Natural Science Foundation of China (Grant numbers: 81541070, 81101267, 30901249, and 81602853), Guangdong Natural Science Foundation (Grant numbers: 2018A030313601, 2016A030313089, 10151063201000036, and S2011010002526), Guangdong Province Medical Research Foundation (Grant number: A2014374 and A2015310) and Project from Jinan University (Grant number: 21612426, 21615426, JNUPHPM2016001, and JNUPHPM2016002).
The Supplementary Material for this article can be found online at: https://www.frontiersin.org/articles/10.3389/fendo.2018.00564/full#supplementary-material
95%CI, 95% confidence interval; BMI, body mass index; CNKI, China National Knowledge Infrastructure; GWAS, genome-wide association study; HWE, Hardy-Weinberg equilibrium; IFG, impaired fasting glucose; IGR, impaired glucose regulation; IGT, impaired glucose tolerance; LDR, ligase detection reaction; OR, odds ratio; PCR-HRM, polymerase chain reaction–high resolution melt; PCR-RFLP, cleaved amplification polymorphism sequence-tagged sites; RRR, relative risk reduction; SLC30A8, zinc transporter solute carrier family 30 member 8 gene; SNP, single nucleotide polymorphism; T2DM, type 2 diabetes mellitus; TSA, trial sequential analysis; WHO, World Health Organization; ZnT-8, zinc transporter 8
1. DeFronzo RA, Ferrannini E, Groop L, Henry RR, Herman WH, Holst JJ, et al. Type 2 diabetes mellitus. Nat Rev Dis Primers (2015) 1:15019. doi: 10.1038/nrdp.2015.192
2. Yang W, Lu J, Weng J, Jia W, Ji L, Xiao J, et al. Prevalence of diabetes among men and women in China. N Engl J Med. (2010) 362:1090–101. doi: 10.1056/NEJMoa0908292
3. Malecki MT. Genetics of type 2 diabetes mellitus. Diabetes Res Clin Pract. (2005) 68:S10–21. doi: 10.1016/j.diabres.2005.03.003
4. Tabak AG, Jokela M, Akbaraly TN, Brunner EJ, Kivimaki M, Witte DR. Trajectories of glycaemia, insulin sensitivity, and insulin secretion before diagnosis of type 2 diabetes: an analysis from the Whitehall II study. Lancet (2009) 373:2215–21. doi: 10.1016/S0140-6736(09)60619-X
5. Poitout V, Robertson RP. Glucolipotoxicity: fuel excess and beta-cell dysfunction. Endocr Rev. (2008) 29:351–66. doi: 10.1210/er.2007-0023
6. Saad MF, Knowler WC, Pettitt DJ, Nelson RG, Mott DM, Bennett PH. The natural history of impaired glucose tolerance in the pima Indians. N Engl J Med. (1988) 319:1500–6. doi: 10.1056/NEJM198812083192302
7. Kang ES, Kim MS, Kim YS, Kim CH, Han SJ, Chun SW, et al. A polymorphism in the zinc transporter gene SLC30A8 confers resistance against posttransplantation diabetes mellitus in renal allograft recipients. Diabetes (2008) 57:1043–7. doi: 10.2337/db07-0761
8. Chimienti F, Devergnas S, Favier A, Seve M. Identification and cloning of a beta-cell-specific zinc transporter, ZnT-8, localized into insulin secretory granules. Diabetes (2004) 53:2330–7. doi: 10.2337/diabetes.53.9.2330
9. Nicolson TJ, Bellomo EA, Wijesekara N, Loder MK, Baldwin JM, Gyulkhandanyan AV, et al. Insulin storage and glucose homeostasis in mice null for the granule zinc transporter ZnT8 and studies of the type 2 diabetes-associated variants. Diabetes (2009) 58:2070–83. doi: 10.2337/db09-0551
10. Fu Y, Tian W, Pratt EB, Dirling LB, Shyng SL, Meshul CK, et al. Down-regulation of ZnT8 expression in INS-1 rat pancreatic beta cells reduces insulin content and glucose-inducible insulin secretion. PLoS ONE (2009) 4:e5679. doi: 10.1371/journal.pone.0005679
11. Kirchhoff K, Machicao F, Haupt A, Schafer SA, Tschritter O, Staiger H, et al. Polymorphisms in the TCF7L2, CDKAL1 and SLC30A8 genes are associated with impaired proinsulin conversion. Diabetologia (2008) 51:597–601. doi: 10.1007/s00125-008-0926-y
12. Saxena R, Voight BF, Lyssenko V, Burtt NP, de Bakker PI, Chen H, et al. Genome-wide association analysis identifies loci for type 2 diabetes and triglyceride levels. Science (2007) 316:1331–6. doi: 10.1126/science.1142358
13. Scott LJ, Mohlke KL, Bonnycastle LL, Willer CJ, Li Y, Duren WL, et al. A genome-wide association study of type 2 diabetes in Finns detects multiple susceptibility variants. Science (2007) 316:1341–5. doi: 10.1126/science.1142382
14. Scuteri A, Sanna S, Chen WM, Uda M, Albai G, Strait J, et al. Genome-wide association scan shows genetic variants in the FTO gene are associated with obesity-related traits. PLoS Genet. (2007) 3:e115. doi: 10.1371/journal.pgen.0030115
15. Sladek R, Rocheleau G, Rung J, Dina C, Shen L, Serre D, et al. A genome-wide association study identifies novel risk loci for type 2 diabetes. Nature (2007) 445:881–5. doi: 10.1038/nature05616
16. Zeggini E, Weedon MN, Lindgren CM, Frayling TM, Elliott KS, Lango H, et al. Replication of genome-wide association signals in UK samples reveals risk loci for type 2 diabetes. Science (2007) 316:1336–41. doi: 10.1126/science.1142364
17. Thakkinstian A, McKay GJ, McEvoy M, Chakravarthy U, Chakrabarti S, Silvestri G, et al. Systematic review and meta-analysis of the association between complement component 3 and age-related macular degeneration: a HuGE review and meta-analysis. Am J Epidemiol. (2011) 173:1365–79. doi: 10.1093/aje/kwr025
18. He J, Liao XY, Zhu JH, Xue WQ, Shen GP, Huang SY, et al. Association of MTHFR C677T and A1298C polymorphisms with non-Hodgkin lymphoma susceptibility: evidence from a meta-analysis. Sci Rep. (2014) 4:6159. doi: 10.1038/srep06159
19. Petitti DB. Approaches to heterogeneity in meta-analysis. Stat Med. (2001) 20:3625–33. doi: 10.1002/sim.1091
20. DerSimonian R, Laird N. Meta-analysis in clinical trials. Control Clin Trials (1986) 7:177–88. doi: 10.1016/0197-2456(86)90046-2
21. Riley RD, Higgins JP, Deeks JJ. Interpretation of random effects meta-analyses. BMJ (2011) 342:d549. doi: 10.1136/bmj.d549
22. Higgins JP, Thompson SG, Deeks JJ, Altman DG. Measuring inconsistency in meta-analyses. BMJ (2003) 327:557–60. doi: 10.1136/bmj.327.7414.557
23. Egger M, Davey Smith G, Schneider M, Minder C. Bias in meta-analysis detected by a simple, graphical test. BMJ (1997) 315:629–34. doi: 10.1136/bmj.315.7109.629
24. Duval S, Tweedie R. Trim and fill: A simple funnel-plot-based method of testing and adjusting for publication bias in meta-analysis. Biometrics (2000) 56:455–63. doi: 10.1111/j.0006-341X.2000.00455.x
25. Wetterslev J, Thorlund K, Brok J, Gluud C. Trial sequential analysis may establish when firm evidence is reached in cumulative meta-analysis. J Clin Epidemiol. (2008) 61:64–75. doi: 10.1016/j.jclinepi.2007.03.013
26. Brok J, Thorlund K, Wetterslev J, Gluud C. Apparently conclusive meta-analyses may be inconclusive–trial sequential analysis adjustment of random error risk due to repetitive testing of accumulating data in apparently conclusive neonatal meta-analyses. Int J Epidemiol. (2009) 38:287–98. doi: 10.1093/ije/dyn188
27. Steinthorsdottir V, Thorleifsson G, Reynisdottir I, Benediktsson R, Jonsdottir T, Walters GB, et al. A variant in CDKAL1 influences insulin response and risk of type 2 diabetes. Nat Genet. (2007) 39:770–5. doi: 10.1038/ng2043
28. Ng MC, Park KS, Oh B, Tam CH, Cho YM, Shin HD, et al. Implication of genetic variants near TCF7L2, SLC30A8, HHEX, CDKAL1, CDKN2A/B, IGF2BP2, and FTO in type 2 diabetes and obesity in 6,719 Asians. Diabetes (2008) 57:2226–33. doi: 10.2337/db07-1583
29. Zhang SL, Liu J, Liu JX, Liu J, Xu YJ, Wei SH, et al. The research of relationship between SLC30A8 gene rsl3266634 C/T polymorphism and type 2 diabetes mellitus risk in the Dongxiang nationality versus the Moselm nationality in Gansu. Chin J Diabetes (2014) 22:5903.
30. Zhang SL, Liu J, Liu JX, Liu J, Xu YJ, Wei SH. The research of relationship between SLC30A8 gene rs 13266634 C/T polymorphism and type 2 diabetes mellitus risk in the Dongxiang and Moselm nationality in Gansu. Chin J Gerontol. (2015) 35:898–900.
31. Su YX, Ma Y, Yao H, Zhu J, Wang TT, Wang ZQ, et al. Association between polymorphisms of SLC30A8 and their interaction and type 2 diabetes in Uyhgur nationality. Chin J Diabetes (2016) 24:12–6.
32. Huang Q, Yin JY, Dai XP, Wu J, Chen X, Deng CS, et al. Association analysis of SLC30A8 rs13266634 and rs16889462 polymorphisms with type 2 diabetes mellitus and repaglinide response in Chinese patients. Eur J Clin Pharmacol. (2010) 66:1207–15. doi: 10.1007/s00228-010-0882-6
33. Huang Y, Sheng HG. Relationship between SLC30A8 gene polymorphism and type 2 diabetes in Southern Chinese population. J Clin Res. (2011) 28:20–4.
34. Shan Z, Bao W, Zhang Y, Rong Y, Wang X, Jin Y, et al. Interactions between zinc transporter-8 gene (SLC30A8) and plasma zinc concentrations for impaired glucose regulation and type 2 diabetes. Diabetes (2014) 63:1796–803. doi: 10.2337/db13-0606
35. Luo XJ, Cheng CM, Xie Y. Study on the correlation between the seven single nucleotide polymorphisms and genesis of type 2 diabetes mellitus. Chin J Diabetes (2015):115–8.
36. Zhang Y, Xiao X, Zhang Z, Ma X, Xu T, Li W, et al. Role of high-risk variants in the development of impaired glucose metabolism was modified by birth weight in Han Chinese. Diabetes Metab Res Rev. (2015) 31:790–5. doi: 10.1002/dmrr.2670
37. Wang ZH, Zhang SH, Wang ZC, Gong LL, Li R, Ren W, et al. Relationship of rs12366634 polymorphism in SLA30A8 (solute carrier family, member 8) gene with type 2 diabetes in Chinese Han population. Shanghai Med J. (2008) 31:323–7.
38. Wu Y, Li H, Loos RJ, Yu Z, Ye X, Chen L, et al. Common variants in CDKAL1, CDKN2A/B, IGF2BP2, SLC30A8, and HHEX/IDE genes are associated with type 2 diabetes and impaired fasting glucose in a Chinese Han population. Diabetes (2008) 57:2834–42. doi: 10.2337/db08-0047
39. Xiang J, Li XY, Xu M, Hong J, Huang Y, Tan JR, et al. Zinc transporter-8 gene (SLC30A8) is associated with type 2 diabetes in Chinese. J Clin Endocrinol Metab. (2008) 93:4107–12. doi: 10.1210/jc.2008-0161
40. Hu C, Zhang R, Wang C, Wang J, Ma X, Lu J, et al. PPARG, KCNJ11, CDKAL1, CDKN2A-CDKN2B, IDE-KIF11-HHEX, IGF2BP2 and SLC30A8 are associated with type 2 diabetes in a Chinese population. PLoS ONE (2009) 4:e7643. doi: 10.1371/journal.pone.0007643
41. Han X, Luo Y, Ren Q, Zhang X, Wang F, Sun X, et al. Implication of genetic variants near SLC30A8, HHEX, CDKAL1, CDKN2A/B, IGF2BP2, FTO, TCF2, KCNQ1, and WFS1 in type 2 diabetes in a Chinese population. BMC Med Genet. (2010) 11:81. doi: 10.1186/1471-2350-11-81
42. Lin Y, Li P, Cai L, Zhang B, Tang X, Zhang X, et al. Association study of genetic variants in eight genes/loci with type 2 diabetes in a Han Chinese population. BMC Med Genet. (2010) 11:97. doi: 10.1186/1471-2350-11-97
43. Shu XO, Long J, Cai Q, Qi L, Xiang YB, Cho YS, et al. Identification of new genetic risk variants for type 2 diabetes. PLoS Genet. (2010) 6:e1001127. doi: 10.1371/journal.pgen.1001127
44. Tan JT, Ng DP, Nurbaya S, Ye S, Lim XL, Leong H, et al. Polymorphisms identified through genome-wide association studies and their associations with type 2 diabetes in Chinese, Malays, and Asian-Indians in Singapore. J Clin Endocrinol Metab. (2010) 95:390–7. doi: 10.1210/jc.2009-0688
45. Xu M, Bi Y, Xu Y, Yu B, Huang Y, Gu L, et al. Combined effects of 19 common variations on type 2 diabetes in Chinese: results from two community-based studies. PLoS ONE (2010) 5:e14022. doi: 10.1371/journal.pone.0014022
46. Li XJ, Su Y, Yan CL, Zhang JJ, Gu L, Qin WB, et al. Association of rs13266634 Polymorphism in SLC30A8 Gene with Type 2 diabetes in Han population of inner Mongolia. Prog Modern Biomed. (2011):2221–3.
47. Wang JP, Li J, Deng SS. The relationship between polymorphisms of solute carrier family 30, member 8 gene and type 2 diabetes. Chin General Pract. (2011) 14:1296–9.
48. Fu LL, Lin Y, Yang ZL, Yin YB. Association analysis of genetic polymorphisms of TCF7L2, CDKAL1, SLC30A8, HHEX genes and microvascular complications of type 2 diabetes mellitus. Zhong Hua Yi Xue Yi Chuan Xue Za Zhi (2012) 29:194–9. doi: 10.3760/cma.j.issn.1003-9406.2012.02.017
49. Zheng X, Ren W, Zhang S, Liu J, Li S, Li J, et al. Association of type 2 diabetes susceptibility genes (TCF7L2, SLC30A8, PCSK1 and PCSK2) and proinsulin conversion in a Chinese population. Mol Biol Rep. (2012) 39:17–23. doi: 10.1007/s11033-011-0705-6
50. Chen G, Xu Y, Lin Y, Lai X, Yao J, Huang B, et al. Association study of genetic variants of 17 diabetes-related genes/loci and cardiovascular risk and diabetic nephropathy in the Chinese She population. J Diabetes (2013) 5:136–45. doi: 10.1111/1753-0407.12025
51. Tam CH, Ho JS, Wang Y, Lam VK, Lee HM, Jiang G, et al. Use of net reclassification improvement (NRI) method confirms the utility of combined genetic risk score to predict type 2 diabetes. PLoS ONE (2013) 8:e83093. doi: 10.1371/journal.pone.0083093
52. Chang YC, Liu PH, Yu YH, Kuo SS, Chang TJ, Jiang YD, et al. Validation of type 2 diabetes risk variants identified by genome-wide association studies in Han Chinese population: a replication study and meta-analysis. PLoS ONE (2014) 9:e95045. doi: 10.1371/journal.pone.0095045
53. Chen HQ, Liu J, Zhang Q, Liu JX, Zhao J, Wang YF, et al. Correlation between solute carrier family 30, member 8 gene polymorphism and type 2 diabetes mellitus in Gansu Han population. J Lanzhou Univ. (2014):21–5.
54. Jin JJ, Gong KY, Zhou WJ. Correlation and interaction between minor genes and type 2 diabetes mellitus in Korean-Chinese. J Med Sci Yanbian Univ. (2014):84–8.
55. Zhang SL, Liu J, Liu JX, Wei SH. Association of rs13266634 polymorphism in SLC30A8 gene with type 2 diabetes in Chinese Dongxiang Nationality. Gansu Sci Technol. (2014) 30:138–9.
56. Chen Y, Zhao Y, Liu GF. Association of SLC30A8, CDKN2A/2B, HHEX and TCF7L2 gene polymorphisms with type 2 diabetes in northeastern China. Chin J Gerontol. (2015):4201–4.
57. Bali K. Study on Realtionship Between the rs12366634 Polymorphism of TCF7L2 Gene and Type 2 Diabetes in Xinjiang Han Populations. Master Thesis, Xinjiang Medcial School, Urumqi (2015).
58. Liu Y, Wang ZY, Chi ZH, Wang T. Research on Correlation between rs13266634 C/T SNP of SLC30A8 gene and susceptibility to type 2 diabetes mellitus. J China Med Univ. (2015):494–7.
59. Qian Y, Lu F, Dong M, Lin Y, Li H, Dai J, et al. Cumulative effect and predictive value of genetic variants associated with type 2 diabetes in Han Chinese: a case-control study. PLoS ONE (2015) 10:e0116537. doi: 10.1371/journal.pone.0116537
60. Su YX, Wang ZQ, Yao H, Wang TT, Ma Q, Zhu J, et al. Association between type 2 diabetes in Uygur and polymorphisms of SLC30A8 and its interaction with smoking. Chin J Epidemiol. (2015) 36:1167–71.
61. Zhang SL, Liu J, Liu JX, Liu J, Xu YJ, Wei SH. The research of relationship between SLC30A8 gene rs 13266634 C/T polymorphism and type 2 diabetes mellitus risk in the Dongxiang and Moselm nationality in Gansu. Chin J Gerontol. (2015) 35:898–900.
62. Zhao LJ, Xu KF, Yang T, Dai H, Ma BQ, Ji L, et al. Relationship between SLC30A8 and PTPRD gene polymorphisms and type 2 diabetes in middle aged and elderly people in Nanjing area. Int J Endocrinol Metab. (2015) 35:145–8.
63. Zou J. Serum Levels of Specific Autoantibodies in Patients With Autoimmune Diabetes and Thyroid Disease and Gene Polymorphism Correlation Analysis. Master Thesis, Jilin University, Changchun (2016).
64. Xu K, Zha M, Wu X, Yu Z, Yu R, Xu X, et al. Association between rs13266634 C/T polymorphisms of solute carrier family 30 member 8 (SLC30A8) and type 2 diabetes, impaired glucose tolerance, type 1 diabetes–a meta-analysis. Diabetes Res Clin Pract. (2011) 91:195–202. doi: 10.1016/j.diabres.2010.11.012
65. Al-Rubeaan K, Siddiqui K, Saeb AT, Nazir N, Al-Naqeb D, Al-Qasim S. ACE I/D and MTHFR C677T polymorphisms are significantly associated with type 2 diabetes in Arab ethnicity: a meta-analysis. Gene (2013) 520:166–77. doi: 10.1016/j.gene.2013.02.017
66. Fan M, Li W, Wang L, Gu S, Dong S, Chen M, et al. Association of SLC30A8 gene polymorphism with type 2 diabetes, evidence from 46 studies: a meta-analysis. Endocrine (2016) 53:381–94 doi: 10.1007/s12020-016-0870-4
67. Bougneres P. Genetics of obesity and type 2 diabetes: tracking pathogenic traits during the predisease period. Diabetes (2002) 51:S295–303. doi: 10.2337/diabetes.51.2007.S295
68. Tabara Y, Osawa H, Kawamoto R, Onuma H, Shimizu I, Miki T, et al. Replication study of candidate genes associated with type 2 diabetes based on genome-wide screening. Diabetes (2009) 58:493–8. doi: 10.2337/db07-1785
69. Rungby J. Zinc, zinc transporters and diabetes. Diabetologia (2010) 53:1549–51. doi: 10.1007/s00125-010-1793-x
70. Wijesekara N, Chimienti F, Wheeler MB. Zinc, a regulator of islet function and glucose homeostasis. Diabetes Obes Metab. (2009) 11:202–14. doi: 10.1111/j.1463-1326.2009.01110.x
71. Chimienti F, Devergnas S, Pattou F, Schuit F, Garcia-Cuenca R, Vandewalle B, et al. In vivo expression and functional characterization of the zinc transporter ZnT8 in glucose-induced insulin secretion. J Cell Sci. (2006) 119:4199–206. doi: 10.1242/jcs.03164
72. Kanoni S, Nettleton JA, Hivert MF, Ye Z, van Rooij FJ, Shungin D, et al. Total zinc intake may modify the glucose-raising effect of a zinc transporter (SLC30A8) variant: a 14-cohort meta-analysis. Diabetes (2011) 60:2407–16. doi: 10.2337/db11-0176
Keywords: type 2 diabetes mellitus, impaired glucose regulation, SLC30A8, rs13266634, polymorphism, meta-analysis
Citation: Dong F, Zhang B, Zheng S, Huang X, Du X, Zhu K, Chen X, Wu J, Liu D, Wen Z, Zou X, Liu Y, Dong S, Zeng F, Yang G and Jing C (2018) Association Between SLC30A8 rs13266634 Polymorphism and Risk of T2DM and IGR in Chinese Population: A Systematic Review and Meta-Analysis. Front. Endocrinol. 9:564. doi: 10.3389/fendo.2018.00564
Received: 26 February 2018; Accepted: 04 September 2018;
Published: 25 September 2018.
Edited by:
David Meyre, McMaster University, CanadaReviewed by:
Eusebio Chiefari, Università degli Studi Magna Graecia di Catanzaro, ItalyCopyright © 2018 Dong, Zhang, Zheng, Huang, Du, Zhu, Chen, Wu, Liu, Wen, Zou, Liu, Dong, Zeng, Yang and Jing. This is an open-access article distributed under the terms of the Creative Commons Attribution License (CC BY). The use, distribution or reproduction in other forums is permitted, provided the original author(s) and the copyright owner(s) are credited and that the original publication in this journal is cited, in accordance with accepted academic practice. No use, distribution or reproduction is permitted which does not comply with these terms.
*Correspondence: Fang-fang Zeng, emVuZ2Zmam51QDEyNi5jb20=
Guang Yang, dHlhbmdndWFuZ0BlbWFpbC5qbnUuZWR1LmNu
Chun-xia Jing, amN4cGhkQGdtYWlsLmNvbQ==
†Co-first authors
Disclaimer: All claims expressed in this article are solely those of the authors and do not necessarily represent those of their affiliated organizations, or those of the publisher, the editors and the reviewers. Any product that may be evaluated in this article or claim that may be made by its manufacturer is not guaranteed or endorsed by the publisher.
Research integrity at Frontiers
Learn more about the work of our research integrity team to safeguard the quality of each article we publish.