- 1College of Water Resources and Civil Engineering, China Agricultural University, Beijing, China
- 2Center for Agricultural Water Research in China, China Agricultural University, Beijing, China
- 3College of Water Sciences, Beijing Normal University, Beijing, China
- 4Beijing Key Laboratory of Urban Hydrological Cycle and Sponge City Technology, Beijing, China
The Yarlung Zangbo River (YZR) basin occupies a crucial position in the formation and development of atmospheric circulation and climate change in the Tibetan Plateau, where is the potential trigger and amplifier in global climate fluctuations. Previous studies mainly focused on meteorological drought induced by variations of precipitation and temperature. In this study, a multi-index evaluation of drought characteristics from the perspective of meteorology and agriculture was implemented. GLDAS (Global Land Data Assimilation System) precipitation, surface air temperature and soil moisture data from 1982 to 2015 were used to calculate the meteorological drought index (Standardized Precipitation Evapotranspiration Index) and agricultural drought index (Soil Water Deficit Index), respectively. Meanwhile, the scPDSI (self-calibrating Palmer Drought Severity Index) dataset provided by CRU (Climate Research Unit) was also utilized to represent the meteorological drought, with the aim of comprehensively investigating the spatiotemporal evolution characteristics of drought in the YZR basin. Results indicated that although there was a slightly wetting tendency of the whole basin from 1982 to 2015, drought condition from the perspective of meteorology and agriculture at both annual and growing seasonal scales showed a transition from alleviation to aggravation during 1982–2015, with an abrupt change from wetting to drying occurring at the year of 2000 detected by multiple statistical tests including Mann-Kendall test, Moving t-test and Yamamoto test. Specifically, since the twenty-first century, the meteorological drought in the YZR basin has changed from moderate wet to moderate dry, while the agricultural drought relieved to moderate dry from severe dry with a much more complicated fluctuation. From the perspective of spatial pattern, the annual and growing season variation trends of all three drought indices were identically consistent during 1982–2015. Areas with extremely significant decreasing trend (2.24~21.09%) were mostly distributed in the west upstream and southwest downstream dominating the overall wetting trend of the YZR basin during the period of 1982–2015, while the transition from wet to dry after 2000 was attributed to the aggravating drought of the western upstream and southeastern downstream. Results of this study have important implications for drought monitoring and eco-environmental sustainability in alpine regions.
Introduction
Drought is a natural hazard developed slowly that would initiate considerable losses (Kelly et al., 2015; Schwalm et al., 2017; Jiang et al., 2019) which is also regarded as an elusive phenomenon with the indeterminate onset and demise (Greve et al., 2014; Bachmair et al., 2016a). Various drivers exert the occurrence of drought, among which low precipitation and high evapotranspiration are usually considered as the main causes (Mishra and Singh, 2010; Sheffield et al., 2012; Vicente-Serrano et al., 2015). A lot of studies reveal that continuous global warming has exacerbated global water cycle and triggered extreme droughts events (Dai, 2011; Huang et al., 2017; Chen and Sun, 2018). The increasing trend of drought has become a global concern during recent decades. Therefore, it is urgently necessary to detect and monitor drought under the process of global warming.
Drought is generally classified into four types in accordance with the performance characteristics and affected fields, i.e., meteorological drought, agricultural drought, hydrological drought, and socioeconomic drought (Yuan and Zhou, 2004; White and Walcott, 2009; Dai, 2011). Meteorological drought is the prerequisite for other types of drought (Tong et al., 2017). Consequently, numerous meteorological drought indices have been proposed to estimate the drought severity and improve the risk management, such as the Palmer Drought Severity Index (PDSI) (Palmer, 1965), Standard Precipitation Evapotranspiration Index (SPEI) (Vicente-Serrano et al., 2010), Standardized Wetness Index (SWI) (Liu et al., 2017), Standard Precipitation Index (SPI) (McKee et al., 1993), etc. PDSI was the first index to successfully quantify the severity of drought in various climatic conditions and played a milestone role in the development of the drought index (Zhai et al., 2010; Zambrano Mera et al., 2018), which has been widely used to evaluate meteorological drought (Shao et al., 2018; Li et al., 2019a). In order to overcome the poor applicability of PDSI in arid and semi-arid regions, scPDSI (self-calibrating Palmer Drought Severity Index) was put forward by Wells et al. (2004), which has been proven to be a particularly suitable index for detecting and monitoring the effects of global warming on drought conditions (Wang et al., 2016; Herrera and Ault, 2017; Zhu et al., 2018). It improves PDSI by adopting a self-calibration procedure that automatically adjusts the PDSI standardization coefficient to suit the local climate (Bai et al., 2020). Moreover, in the water balance model of scPDSI, dynamic changes of seasonal snowpack are considered (Van der Schrier et al., 2013), which is especially suitable for alpine region, such as the Yarlung Zangbo River (YZR) basin in the Qinghai-Tibet Plateau (QTP). And the high-resolution scPDSI dataset generated by the CRU (Climatic Research Unit) has been successfully applied around the world (Lewinska et al., 2016; Zhang et al., 2019a). With the combination of the sensitivity of the PDSI to changes in evaporation demand and robustness of the multi-temporal nature of the SPI, SPEI at various timescales has been developed and employed in an increasing number of climatological and hydrological studies (Vicente-Serrano et al., 2010; Yu et al., 2014; Spinoni et al., 2018; Li et al., 2019c). Li X. et al. (2015) evaluated the multi-scale patterns and the spatiotemporal extent of drought based on SPEI in Southwest China from 1982 to 2012. Wang et al. (2016) analyzed the performance of five climate-based drought indices, including SPEI and scPDSI, to estimate winter wheat drought threat during 2000–2013 in China. Unlike scPDSI, which obtains evapotranspiration based on the water balance model, SPEI considers the effects of evapotranspiration through the simple difference between precipitation and potential evapotranspiration (PET). In order to better analyze the drought condition in the YZR basin, two meteorological drought indices including scPDSI and SPEI were selected for cross-validation in this study.
Most meteorological drought indices are predominantly applicable to assess drought conditions in global or basin scales, while agricultural drought indices provide a fine-grained assessment of a small area during the growing season (Mishra and Singh, 2011; Zargar et al., 2011). Agricultural drought is considered to start when soil moisture availability reaches a certain low level that will lead to a negative impact on crop yield (Dai, 2011; Zhu et al., 2019). That is, meteorological drought will induce soil water deficit and further agricultural drought. In addition, the water supply of crops is mainly absorbed directly from the soil by the root system (Schoppach and Sadok, 2012). When crop canopy greenness is stressed by soil moisture, it will be lower than normal growth vegetation. In short, agricultural drought events threaten local agri-food markets and food security (Lesk et al., 2016; Yang et al., 2020). The adaptability of agricultural drought can effectively reduce the loss of agricultural drought (Dong et al., 2012). If severe agricultural drought occurs frequently in a region, local people will take long-term measures (such as crop irrigation technology) to reduce the sensitivity of agriculture to drought as well as the impact of agricultural drought on their lives. Therefore, soil water condition is a key indicator in agricultural drought prediction and assessment and irrigation management. The monitoring and early warning systems based on soil moisture are essential for agricultural activities and risk assessment (Bachmair et al., 2016b). Effective quantification of agricultural drought impacts can mitigate crop losses and ameliorate adverse effects (Liu et al., 2016; Baik et al., 2019). However, SPEI ignoring effects of soil moisture could not capture the characteristics of agricultural drought (Bezdan et al., 2019; Teweldebirhan Tsige et al., 2019). Among numerous drought indices calculated based on soil water content, the Soil Water Deficit Index (SWDI) is a more mature indicator to judge crop drought on the basis of wilting point content and field water capacity, which has been widely used in agricultural drought detection (Wagner et al., 2013; Martínez-Fernández et al., 2015; Bai et al., 2018). Martínez-Fernández et al. (2016) further demonstrated that SWDI can reproduce soil water balance dynamics and track agricultural drought preferably. Bai et al. (2018) employed SWDI to evaluate the soil moisture products of Soil Moisture Active Passive (SMAP) in China.
The Yarlung Zangbo River basin is located in the southeastern part of the QTP, China, which is characterized by complex and changeable climatic conditions, rich biological diversities, and distinct vertical vegetation belts (Liu et al., 2007; Chen B. et al., 2015). Climate change on the QTP occupies a vital position of global climate change because it is the potential trigger and amplifier in global climate fluctuations (Shen et al., 2015; Li et al., 2019a). The YZR basin is the source of precipitation on the QTP, where has vast channels carrying moisture from the Indian Ocean to the interior of the Plateau. Hence, the climate of the YZR basin plays a crucial role in the formation and development of atmospheric circulation and climate change in the QTP (Liu et al., 2002; Zhou et al., 2010; Lv et al., 2013). Scholars have done a lot of researches on climate change characteristics of the YZR basin, pointing out that a much more severe drought has been observed in recent decades (Li et al., 2014, 2019a; Li B. et al., 2015; Shen et al., 2015). However, there is a substantial resistance to conduct a study in the YZR basin due to its great altitude gradient, noticeable vertical vegetation belts and sparse observation stations, leading to research focus are limited to analyses on precipitation and temperature changes or meteorological drought only. Therefore, the objectives of this study are: (1) to implement a multi-index evaluation of drought characteristics from the comprehensively perspective of meteorology and agriculture based on multiple remotely sensed data; (2) to identify the spatiotemporal evolution mechanism of dry-wet regime through multiple statistical methods; (3) to explore possible causes of the detected variation characteristics of drought in the YZR basin. Given that few studies focus on investigating different types of drought for monitoring annual and growing season drought over the YZR basin, this study can facilitate water resources management in the YZR basin and provide a solid basis for maintaining the ecosystem sustainability of the QTP.
Materials and Methods
Study Area
The Yarlung Zangbo River (28°00′ ~ 31°16′N, 82°00′ ~ 97°07′E) originates from Gemma Yangzong Glacier in the southwest of Tibet, and its elevation gradually decreases from northwest to southeast, with an average elevation of more than 4,000 m and an altitude drop of 7,120 m, making it one of the highest rivers in the world. It covers an area of about 240,000 km2 and runs east-west through the southeast of the Tibetan Plateau, traversing four prefecture-level cities and 23 counties.
The climate conditions of the YZR basin are greatly influenced by the southwest warm moist air flowing over the Bay of Bengal as well as the westerlies. Thus, the temperature exhibits an obvious vertical belt with the change of elevation, which gradually increases from northwest to southeast (You et al., 2007). Li B. et al. (2015) has proven that the warming rate of annual and seasonal air temperature from 1961 to 2014 in the YZR basin were larger than that in many other regions of the world. Due to its peculiar location and topographic characteristics, the climate of the basin from upstream to downstream shows significant heterogeneities. The period from November to April of next year that has less precipitation is considered as the winter half year and the rest of the year is considered as the summer half. Figure 1 shows the distribution of national meteorological and hydrological stations in the YZR basin. In this study, Nugesha and Nuxia hydrological stations were taken as the outlets of the upstream and midstream, respectively, which divided the YZR basin into three sub-basins. The region between the Gemma Yangzong Glacier and Nugesha hydrological station is defined as the upstream, which is an arid and semi-arid region with annual mean precipitation of <300 mm. The region between the Nugesha and Nuxia hydrological stations is defined as the midstream, which has a temperate climate. The region below the Nuxia hydrological station, with an annual mean precipitation of more than 2,000 mm, is defined as the downstream characterized by a warm and wet climate. The glaciers in the YZR basin cause the upstream to be mostly unused land, while human activities of the downstream are much more frequent than that of the upstream.
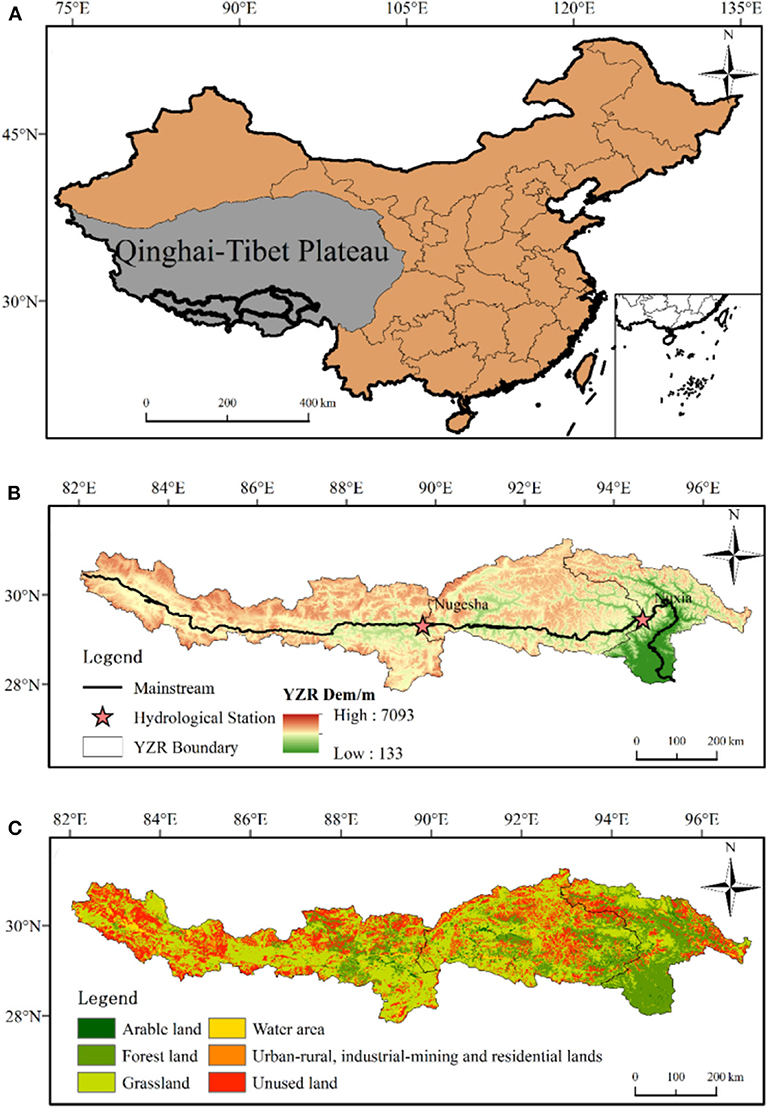
Figure 1. The geographical location (A), hydrometeorological stations (B), and land use types of the Yarlung Zangbo River (YZR) basin (C).
GLDAS_NOAH Data
GLDAS (Global Land Data Assimilation System) data are jointly developed by Goddard Space Flight Center of NASA (National Aeronautics and Space Administration) and NCEP (National Centers for Environmental Prediction), a high-resolution land surface data assimilation system, of NOAA (National Oceanic and Atmospheric Administration), which is available from http://ldas.gsfc.nasa.gov/gldas/GLDASvegetation.php. Utilizing satellite remote sensing and ground observation data, this dataset drives four land surface process models, namely Mosaic, NOAH, CLM, and VIC. Through data simulation and assimilation of four different models, 28 surface variables, such as precipitation (mm), air temperature (°C), and soil moisture content (kg/m2), are generated. At present, the land data assimilation system can provide two versions of data, GLDAS-1 and GLDAS-2, whose time series are from 1970 to 2010 and 2000 to present, respectively, with a time span of 50 years. The spatial and temporal resolutions of the data are 0.25° and 1 month, respectively. In this study, GLDAS-1 and GLDAS-2 data generated by NOAH model from 1982 to 2010 and 2010 to 2015 were spliced to obtain a new time series covering the period of 1982–2015. Performance evaluation of GLDAS data in the YZR basin has already been conducted by Liu et al. (2019) and Li et al. (2019b), indicating a good representation of spatiotemporal characteristics of precipitation, temperature and soil moisture.
Drought Indices
The Self-Calibrated Palmer Drought Severity Index (scPDSI)
The scPDSI was proposed by Wells et al. (2004) to overcome the weakness of the PDSI. Similar to the PDSI, the scPDSI is calculated based on the time series of the precipitation, temperature and the soil moisture with each position related to the surface of fixed parameters, but it can substitute actual vegetation as reference crop drought index owing to considering the seasonal changes, such as snow, thus the scPDSI owns greater spatial applicability.
The CRU-TS3.24 dataset that time series length is 1901–2016 can be acquired from www.cru.uea.ac.uk/data/, which is released by the Climatic Research Unit (CRU), University of East Anglia. The temporal and spatial resolutions of the scPDSI are one 1 month and 0.5° × 0.5°. It classifies the dry and wet characteristics into 9 specific grades, shown in Table 1. The classified scPDSI has been successfully applied to represent the multi-scale drought characteristics in the YZR basin according to the verification results by Li et al. (2019a).
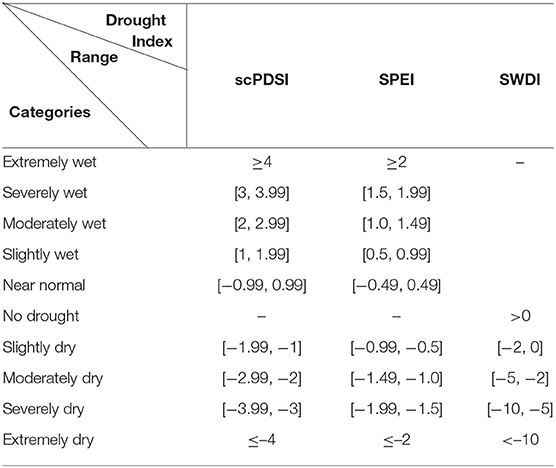
Table 1. Drought index classification for the scPDSI (Wells et al., 2004), SPEI (Vicente-Serrano et al., 2010), and SWDI (Martínez-Fernández et al., 2016).
The Standardized Precipitation Evapotranspiration Index (SPEI)
The SPEI based on the probability model is established by combining the sensitivity to sensibilities in evaporation demand of the PDSI and the robustness of the multi-temporal nature of the SPI (McKee et al., 1993), and has been widely used in many studies so far (Yu et al., 2014; Spinoni et al., 2018; Li et al., 2019c). The calculation formulas of the SPEI are as follows:
(1) PET based on the Thornthwaite method is calculated as:
where T is the monthly mean temperature (°C); K is a correction coefficient that depends on the latitude and month; I is a heat index, which is calculated as the sum of 12 monthly index values,
a is a coefficient depending on I, and it is computed as:
(2) Deficit or surplus of water balance between the PET and precipitation is defined as:
where Di is the difference between monthly precipitation Pi and potential evapotranspiration PETi.
(3) Normalize the water balance into a log-logistic probability distribution to obtain the SPEI index series.
The log-logistic of three parameters is adopted to normalize the aggregated values of the sequence of Di data:
where F(x) is the probability distribution function; α, β, and γ are scale, shape, and origin parameters, respectively, which could all be estimated by the linear distance method.
The SPEI can be obtained as follows:
where p is the standardizing probability density function; the constants are C0 = 2.515517, C1 = 0.802853, C2 = 0.010328, d1 = 1.432788, d2 = 0.189269, and d3 = 0.001308. The 12-month SPEI was adopted to analyze the spatiotemporal characteristics of drought in this study due to its best performance compared with 1-, 3-, and 6-month SPEI (Liu et al., 2019).
The Soil Water Deficit Index (SWDI)
Based on the time series and basic parameters of soil moisture, SWDI can be used to capture agricultural drought conditions through biophysical principles (Vicente-Serrano et al., 2010; Martínez-Fernández et al., 2016). Soil moisture of GLDAS has been proven to have a high consistency and low bias with in situ measurements (Li et al., 2019b). The SWDI is formulated in this study as follows:
where θ is the time series of 0–10 cm soil moisture data from GLDAS (kg/m3); θFC, θWP, and θAWC represent the field capacity, wilting point, and available water capacity, respectively. The 5th percentile and 95th percentile based on GLDAS soil moisture time series are estimators of θWP and θFC.
A positive value of SWDI indicates the excess of soil water content, while negative values imply the occurrence of agricultural drought. The classification criteria of the scPDSI, SPEI and SWDI are shown in Table 1, respectively.
Detection of Abrupt Change
In this study, scPDSI and SPEI were used to represent the meteorological drought, while the agricultural drought was represented by SWDI. In order to accurately evaluate the spatiotemporal variation characteristics of drought in the YZR basin, non-parametric methods including Mann-Kendall test, Moving t-test and Yamamoto test were used to conduct abrupt change detection based on long-term time series of annual and growing season drought indices.
Mann-Kendall Test
The non-parametric Mann-Kendall test, hereinafter referred to as M-K test, was employed for detecting abrupt changes of the time series (Mann, 1945; Kendall, 1975). The advantage is that the sample to be tested does not have to obey a certain distribution, and a few outliers have little effects on the overall data sequence. Two standardized statistic series (UB and UF) are constructed for two unified data time series.
Given a time series of X = (x1, x2, x3, ……, xn), the statistical parameter fk is calculated as follows:
where n is the length of the time series; when xi > xj, logistic (xi > xj) is 1, and 0 otherwise. A positive value of UF represents an ascending trend whereas the inverse represents a descending trend. Under the assumption that the time series is randomly independent, the standardized fk is computed as follows:
where E (fk) and var (fk) are the mean and variance of fk, which are defined as:
The equation of calculating UB that used reversed data series is as follows:
where fk, E (fk), and var (fk) are calculated using the same equations of UF(k). Note that if an intersection of UB and UF curves is within the confidence zone where the confidence level of 95% is treated as the boundary lines, the null hypothesis is considered to be rejected and a significant abrupt change occurred, otherwise, the detected trend is proven to be not significant.
Moving t-Test
The Moving t-test for identification of the abrupt change point is to test whether the mean values of two subsequences change significantly or not at the significance level of 0.05 (Zhao et al., 2008; Liu L. et al., 2012). Comparing mean values of the two subsequences in the climate series for significant test, analyzing variances of the two subsequences and constructing the T statistics, if the statistics pass the confidence level test, then the point where has reached the maximum absolute value of statistic is the abrupt change point most likely (Fu and Wang, 1992). Two subsequences of almost equivalent length are obtained by defining a datum point manually. The method is calculated as follows:
where n1 and n2 are the lengths of two subsequences; x1 and x2 are the two sets of subsequences; , , s1, and s2 are mean values and standard deviations of x1 and x2, respectively. Equation (17) follows the t distribution of n1+n2−2 DOF (Degree of Freedom). When t > t1−α/2 (i.e., α is the significance level of the student test and equals 0.05 in this study), it can be considered that the point of abrupt change has befallen at the significance level of 0.05.
Yamamoto Test
The principle of the Yamamoto test, which is defined mainly from the ratio of climate information and noise, is similar with Moving t-test in that an artificially defined reference point (Yamamoto et al., 1985; Fu and Wang, 1992). Two subsequences before and after the artificial reference point are defined as n1 and n2; the signal-to-noise ratio (SNR) is defined as follows:
where the absolute deviations between and are signals of climate change; the sum of s1 and s2 is the variability of climate change; RSN is the value of the SNR, namely the mutagenic index, and the mutation occurs at the certain time when RSN ≥ 1.
Trend Analysis
One-dimensional linear regression (Hao et al., 2016) was employed to conduct the long-term trend analysis on the time series of scPDSI, SPEI, and SWDI in the study area to describe the spatiotemporal variations from 1982 to 2015. The calculation formula is as follows:
where slope represents the changing trends of scPDSI, SPEI, and SWDI; n is the study temporal interval, n = 34 in this study; and Ci represents scPDSI, SPEI, or SWDI for the year i. A significance test was performed on the variation trends of three drought indices (P < 0.01 indicates an extremely significant trend, P < 0.05 indicates a significant trend, and P > 0.05 implies that the variation trend is not significant).
Results
A Change From Wetting to Drying Inferred by Temporal Variations
Drought is more destructive when it occurs during the growing season (Ahmed et al., 2016; Shiru et al., 2019). Scholars have revealed that vegetation conditions under global warming are significantly correlated with growing season drought (Ji and Peters, 2003; Liu X. et al., 2012; Luo et al., 2015). Thus, growing season drought was investigated along with annual drought in this study, and the growing season in the YZR basin was defined as the period from May to September within a year according to the variation characteristic of vegetation growth, precipitation, and temperature. As shown in Figure 2, the annual and growing season variation trends of all three drought indices were fitted by quadratic polynomial curves, showing the highest correlation with changing pattern of drought indices. It can be seen that values of scPDSI, SPEI, and SWDI at both annual and growing season scales all showed a change from increasing to declining in the later 1990s, inferring that the drought condition in the YZR basin during the period of 1982–2015 was firstly alleviated and then gradually aggravated from both the meteorological and agricultural perspectives. This phenomenon was consistent with the findings about extreme precipitation events in the YZR basin by Liu et al. (2018a), which demonstrated that almost of six extreme precipitation indices mutated in 1995 during the period of 1973–2016.
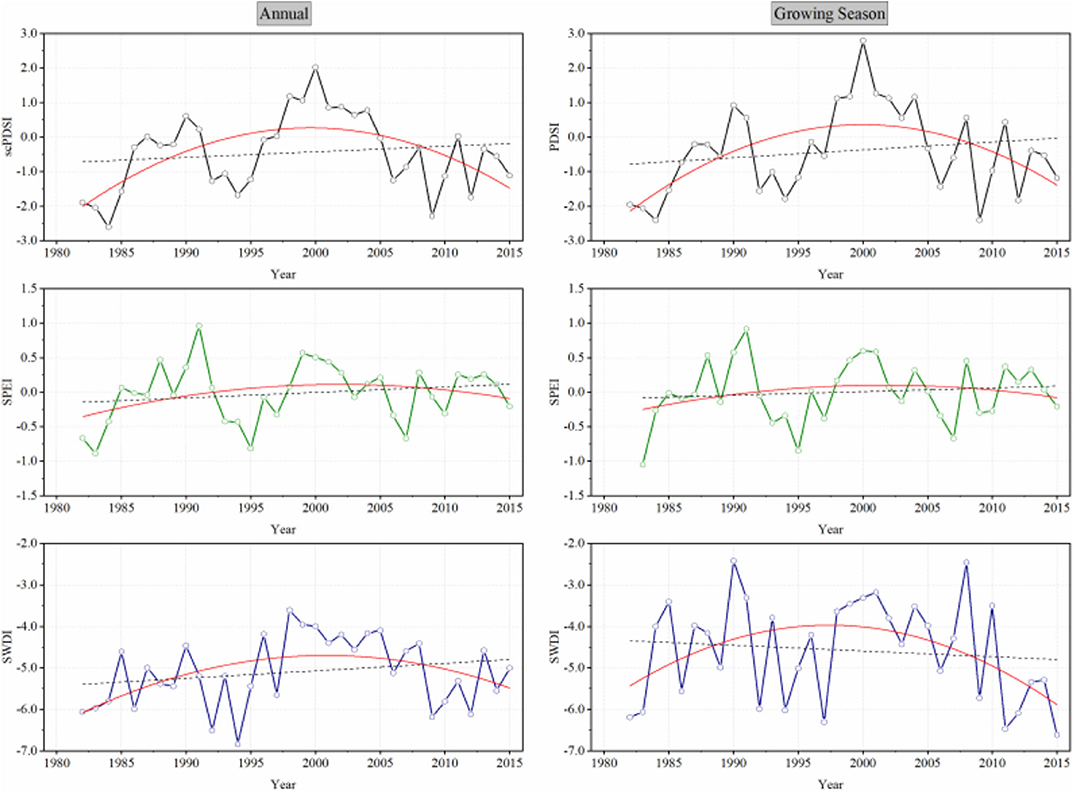
Figure 2. Variation trend of three drought indices (the black, green, and blue curves are scPDSI, SPEI, and SWDI, respectively. The red solid lines are curves fitted by quadratic polynomial. The black dashed lines are linear trend lines from 1982 to 2015. And the left column is annual variations of three indices, while the left column is variations of growing season).
In terms of the annual scPDSI, it fluctuated between −3 and +3 corresponding to moderately dry and moderately wet in the past 34 years, with the maximum value (2.02) occurring in 2000. Similarly, the growing season scPDSI also experienced a fluctuating period from 1982 to 2015, with the maximum value (2.79) occurring in 2000. Similar to scPDSI, SPEI waved between −1.5 and +1.5, indicating that the dry-wet condition in the YZR basin during 1982–2015 varied between moderate humidity and moderate aridity consistent with the drought grades deduced by scPDSI. It can be seen from Figure 2 that the highest points of the fitted curves all located around 2000, revealing that abrupt changes of meteorological drought occurred in the late 1990s and early 2000s. However, the change from wetting to drying implicated by the fitted curve of SPEI was not as significant as that of scPDSI, due to the different occurring time of the maximum value, which was located in the year of 1992. From the perspective of agricultural drought indicated by the SWDI, the YZR basin suffered a harsh drought condition where it varied between moderate drought and severe drought with a much more dramatic fluctuation. But growing season SWDI showed a downward tendency in the 34-year linear trend line, and the annual SWDI was opposite. This situation illustrates that the aggravation drought of the non-growing season made less contribution to the annual agricultural drought than that of the growing season. Contradistinguishing to the annual and growing season variation trends, although the changes of SPEI and SWDI from wet to dry implicated by fitted curves were not as significant as that of scPDSI, such as the different occurring time of maximum values and more dramatic fluctuations, the upward and downward tendencies of SPEI and SWDI were coincide with that of scPDSI on the whole. That is, they all showed a trend of wetting first and then drying around 2000. Differences between meteorological and agricultural drought indices are mainly attributed to the fact that in addition to rainfall and irrigation, other water supply ways such as meltwater of snow and glacier are also the significant water sources of the YZR basin, further illustrating that the influence of global warming on the YZR basin has progressively increased. The main reason for the differences is that the soil moisture in the YZR basin showed a persistently decreasing trend (Zhang et al., 2019b) and SWDI was calculated by soil moisture parameters, implying that it would change immediately as the soil moisture changes. Other factors, such as soil properties, cropping systems, and the amount of irrigation, can also affect agricultural drought (Liu et al., 2016). However, two meteorological drought indices are more related to precipitation and evapotranspiration. If irrigation or other agricultural measures can be provided to crops in time to maintain soil moisture after meteorological drought, then there would no agricultural drought occurring.
Multi-Index Detection of the Changing Point From Wetting to Drying
In order to identify whether there was an abrupt change from wetting process to drying process in the YZR basin, the M-K test, Moving t-test and Yamamoto test were adopted to implement the detection at both annual and growing season time scales based on the long-term time series of scPDSI, SPEI and SWDI. As demonstrated in section A Change From Wetting to Drying Inferred by Temporal Variations, there was a tendency from wetting to drying occurred during the period of the late 1990s to the early 2000s. Li et al. (2019a) pointed out that from 1956 to 2015, scPDSI also had a transition during the mid-twentieth century through locally-weighted scatter point smoothing method, and previous studies revealed that precipitation, temperature, and PET all changed significantly around 2000 in the YZR basin (Liu, 2015; Wang, 2016). As shown in Table 2, the highest occurrence frequency of changing point for annual and growing season droughts indicated by three indices based on M-K test, Moving t-test and Yamamoto test was 1997, followed by the year of 1983 and 2005. However, the changing point of 1983 was only identified by the M-K test, while much more abrupt change points detected by Moving t-test and Yamamoto test were concentrated in the period from the late of 1990s to the early of 2000s, further verifying there was an abrupt change around 2000 from wetting to drying in the YZR basin.
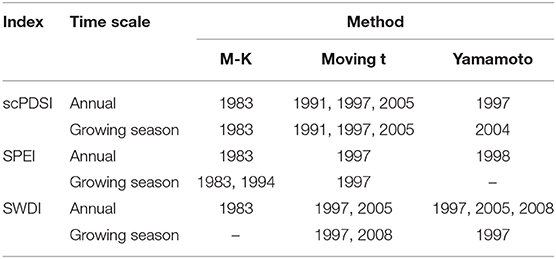
Table 2. Results of abrupt change detection for three drought indices at annual and growing season scales.
Determination of the abrupt change point of the dry-wet condition in the YZR basin was of great importance to conduct further investigation in this study. Given that runoff has been regarded as the most direct indicator to represent the dry-wet characteristics at the river basin scale (Liu et al., 2014; Yang et al., 2017). Located in the lower reaches of the YZR basin, the Nuxia hydrological station controls nearly 80% area of the basin, and its long-term variation of runoff can effectively reflect the dry-wet condition of the whole basin. Thus, the runoff time series from 1982 to 2015 at the Nuxia hydrological station were used in this study to further identify the abrupt change point from wetting to drying. Results of the M-K test for monotonic trend showed that, taking 2000 as the changing point, the runoff from 1982 to 1999 exhibited a significant increasing trend (P < 0.1) and a significant decreasing trend from 2000 to 2015 (P < 0.1). However, if taking 1998, 1999, or any other year during the period from the late of 1990s to the early of 2000s as the changing point, runoff during the divided two periods according to the changing point could not show simultaneously significant increasing trend and significant decreasing trend (Figure 3). Combining with the long-term variations of drought indicated by three indices, it could be determined that there was an abrupt change from wetting to drying in the YZR basin, which occurred at the year of 2000. The transition characteristics of drought from 1982 to 2015 in the YZR basin had significant impacts on water projects and water safety in terms of water allocation, regulation, and water resource utilization (Alfieri et al., 2007; Chen X. et al., 2015). In consideration of utilizing the water resource in the downstream and relieving water shortage in some northern areas, it is an effective way to build pumped storage hydropower stations in the midstream.
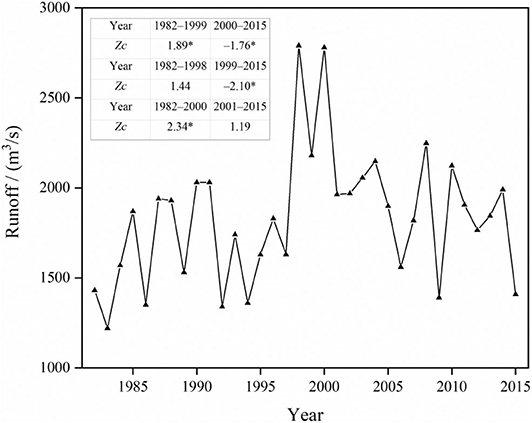
Figure 3. Variation trend of runoff at the Nuxia hydrological station from 1982 to 2015 (*a statistically significant variation trend at the confidence level of 90%).
Spatial Variation of Drought Associated With the Change From Wetting to Drying
In order to characterize the regional differences in meteorological and agricultural drought change trends in the watershed for 34 years, it is necessary to analyse the spatial evolution process and occurrence characteristics of drought in the YZR basin. Due to the significant spatial heterogeneities of meteorological and underlying conditions, the spatial distributions of the annual and growing season values of three drought indices in the YZR basin before and after 2000 were obtained by using Kriging interpolation method, aiming to further investigate the drought evolution mechanism from wetting to drying. As shown in Figure 4, it could be obviously found that the annual spatial distribution of scPDSI, SPEI, and SWDI showed a high consistency with that of the growing season during both periods (1982–1999 and 2000–2015). Before 2000, the dry areas were mainly located in the east upstream and midstream of the study area, while the west upstream and southeast downstream were relatively wet. Similar to the temporal abrupt change phenomenon from wetting to drying at the year of 2000, the spatial pattern of scPDSI, SPEI and SWDI all displayed a reversal phenomenon from the period of 1982–1999 to the period of 2000–2015, i.e., areas where used to be wet during 1982–1999 were getting dry during 2000–2015 and formerly dry areas were getting wet, mainly attributed to the aggravation of drought in the west and southeast of the upstream and the alleviation of drought in the midstream from 1982 to 2015. These results were consistent with the finding obtained by Li et al. (2019a). However, meteorological drought represented by scPDSI and SPEI showed a different spatial variation of drought level with agricultural drought indicated by SWDI. The driest area indicated by meteorological drought in the basin moved from the midstream to the southeast downstream, while the driest area deduced by agricultural drought shifted from the east midstream and north downstream to the south downstream. Furthermore, during 1982–2015, values of SWDI decreased in the northeast midstream and northwest downstream, causing the degree of agricultural drought turned from severe drought to moderate drought. Two meteorological drought indices showed that the upstream changed from slightly wet to slightly dry, while both meteorological and agricultural drought showed a moderate drought in the downstream after 2000. According to Figures 2, 4, both the annual meteorological and agricultural drought alleviated, and spatially the overall wetting tendency of the YZR basin was mainly attributed to the significant wetting trend of the midstream from 1982 to 2000, while the abrupt change from wetting to drying from 2000 to 2015 in the basin was ascribed to drought aggravation that was situated in the west upstream and south downstream.
To further probe into the mechanism of spatial variations of drought in the YZR basin, the annual and growing season variation trends with the significance test of scPDSI, SPEI, and SWDI were analyzed at the pixel scale (Figure 5). The area proportions occupied by extremely significant, significant and non-significant increases and decreases of the three drought indices were shown in Figure 6.
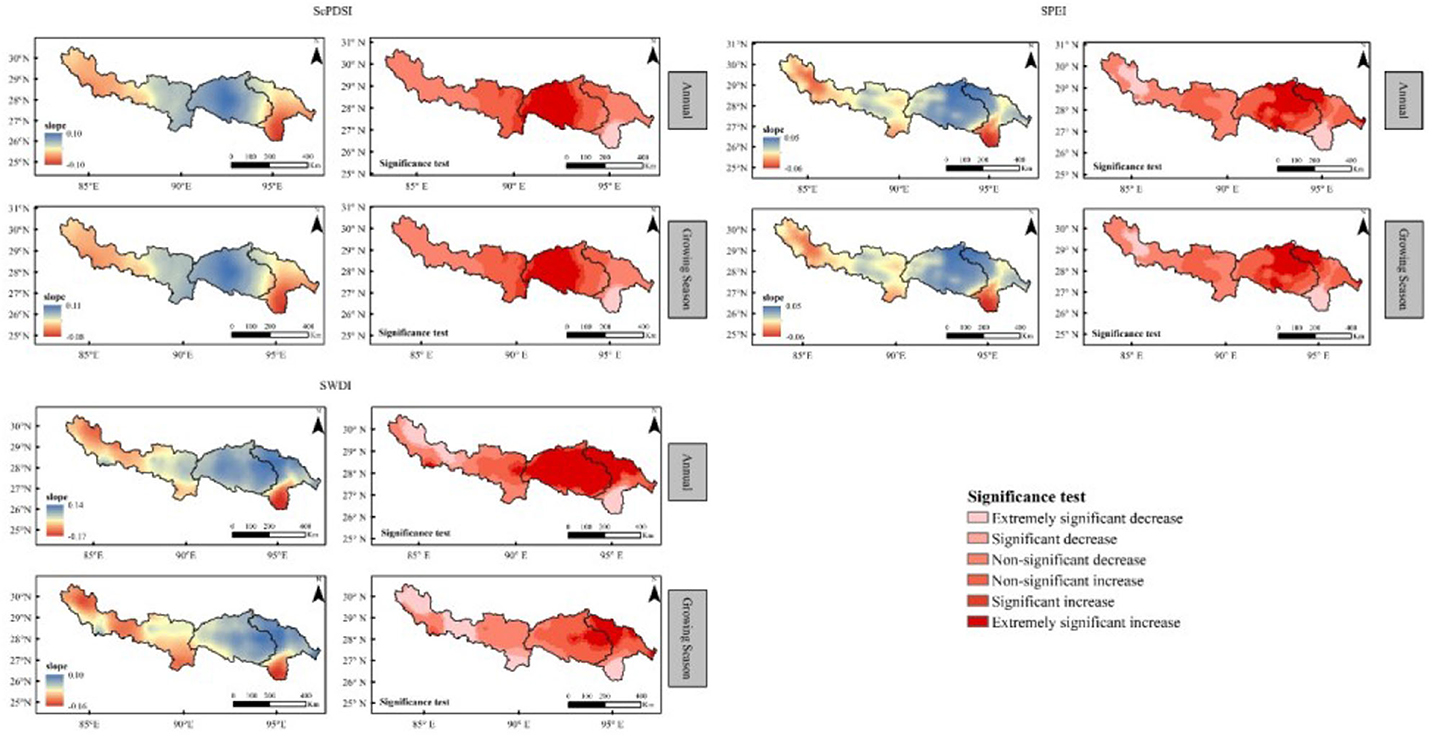
Figure 5. Annual and growing season variation trends of three drought indices with the significance test.
Although the spatial distribution of SWDI representing agricultural drought was slightly different from that of the other two meteorological drought indices (illustrated in Figure 4), the spatial variation trends with the significance test of all three indices at the annual scale exhibited highly consistent characteristics (shown in Figure 5). During 1982–2015, the northwest upstream and southeast downstream showed an increasing trend of drought, while the southeast upstream and middle reaches experienced a contrary characteristic. And areas with extremely significant decreasing trend were mostly distributed in the west upstream and southwest downstream, whereas the midstream and northwest downstream mainly showed extremely significant increasing trends, accounting for the spatial reversal phenomenon from wetting to drying after 2000. The spatial variation trends with the significance test of growing season drought were consistent with those of annual drought. Areas occupied by non-significant increasing and non-significant decreasing trends of the annual and growing season meteorological drought consistently accounted for the greatest and almost equivalent proportions, followed by area proportions occupied by the extremely significant increase, leading to the overall wetting tendency in the YZR basin from 1982 to 2015. There have some differences with the results obtained by Zhang et al. (2019c), which revealed that the trend of agricultural drought was significantly increasing at 95% confidence level during 2000–2014 in the YZR basin. This may be due to discrepancies in length of observation time series and size of the focusing study area. Nevertheless, areas with the greatest proportions for the annual and growing season SWDI were, respectively, located in extremely significant increase and non-significant decrease, and areas with an increasing trend of the annual and growing season SWDI accounted for 62.95 and 43.7% of the basin area, respectively, further giving an explanation for overall alleviated agricultural drought from severe dry to moderate dry and manifesting that the degree of alleviated agricultural drought in the YZR basin was dominated by the alleviated drought in the non-growing season period from January to April and from October to December. With the combination of the results obtained in the study and Zhang et al. (2019c), it is recommended to plant more crops with drought resistance, cold resistance and high yield, such as wheat, in some well-irrigated valleys of the middle and lower reaches for the purpose of coping with growing season drought and guaranteeing the food security in the YZR basin.
Discussion
Droughts cause agricultural loss, forest mortality, and drinking water scarcity. Their current increase in recurrence and intensity poses serious threats to future food security. Still today, our knowledge of how droughts start and evolve remains limited (Zhang et al., 2017; Zhai et al., 2018; Chen et al., 2019; Huang et al., 2019). Zhang et al. (2010) pointed out that temperature rise and precipitation decrease were two important drought-causing factors in the north, northeast, and eastern part of northwest China with the most significant trend of drought since the 1980s. Feng and Fu (2013) considered the rising temperature as the main reason of global drought. Zeng et al. (2019) attributed the spring and winter drought to a decrease in relative humidity which led to an increase in reference evapotranspiration, while the duration of sunshine was the dominant factor of summer and autumn drought in southwest China from 1960 to 2000. In addition, soil drought (low soil moisture) and atmospheric aridity (high vapor pressure deficit, VPD) have been regarded as the two main factors responsible for drought, which influenced terrestrial water use and carbon uptake further (Novick et al., 2016; Zhou et al., 2019b). Ding et al. (2018) demonstrated that alpine grasslands in Tibetan Plateau would face increasingly severe meteorological drought pressure in the future due to the negative influence of VPD. What's more, there is a significant correlation between vegetation degradation and climate change in the YZR basin, emphasizing the importance of VPD as the driving factor of drought (Han et al., 2018). Meanwhile, low correlations between scPDSI and air temperature were found in the YZR basin, indicating that meteorological drought variations were largely unaffected by temperature (Li et al., 2019a). Regarded as the difference between the water vapor pressure at saturation and the actual water vapor pressure for a given temperature, VPD has been used to estimate atmospheric aridity for the implication of drought. Zhou et al. (2019a) demonstrated that VPD had a strong coupling with soil moisture and then would affect the initiation and perpetuation of drought. Increased VPD will increase the atmospheric demand of evapotranspiration water (Penman, 1948; Monteith, 1965). It also will reduce stomatal conductance by closing stomata (Massmann et al., 2019), and changes of VPD substantially influences the terrestrial ecosystem structure and function through vegetation productivity (Konings et al., 2017), further resulting in a significant change in evapotranspiration that leads to meteorological drought (Rawson et al., 1977; Zhou et al., 2019a). Therefore, facing the significant increase of VPD under global warming, VPD calculated by the improved Magus Empirical Formula (Zeng, 1974) was taken into consideration in this study to implement a further investigation on the evolution mechanism of drought in the YZR basin.
According to the temporal variation trends of VPD (Figure 7), the YZR basin got drier during the period of 1982–2015. Obviously, it can be found that there was a huge jump of annual and growing season VPD in 2011 and VPD remained high in the following years. This is consistent with the historical record that severe drought had happened in 2011 (Shao et al., 2018). However, only SWDI indicated such severe drought event, while two meteorological drought indices used in this study indicated that the dry-wet condition of the YZR basin were nearly normal in 2011. The main reason for this consistent implication from VPD and SWDI could be attributed to the fact that rising temperature accelerates the deficit of soil water by increasing soil evaporation and simultaneously aggravates the difference between saturated and actual water vapor pressures by increasing VPD. Nevertheless, meteorological drought was dominated by precipitation rather than temperature demonstrated by Li et al. (2019a), giving an explanation for the inconsistent implication from VPD and two meteorological drought indices, and further emphasizing that VPD could not be independently used to represent the meteorological drought in the YZR basin. As shown in Figure 8, values of annual and growing season VPD from 2000 to 2015 (2.6 and 3.1) were both approximately 1.5 times those from 1982 to 1999 (1.8 and 1.9). The maximum values of annual and growing season VPD (5.5 and 6.0) were all located in the southern downstream and the junction of upstream and midstream, which were consistent with the agricultural drying tendency of upstream and southeast downstream of the basin represented by SWDI. However, the agricultural drought in the northeast midstream and the northwest downstream were alleviating from 1982 to 2015, which is not in line with the spatial distribution of VPD. That may be due to a decrease trend of snow cover in these places, which in turn leads to an increase in soil water content (Liu et al., 2018b). The meteorological drought in the east upstream, nearly the entire midstream and the northwest downstream changed from slightly dry to near normal while VPD of almost the whole basin has increased, which further confirmed that other factors besides VPD comprehensively affect the meteorological drought in the YZR basin. Similar results were also obtained by Liu et al. (2019), demonstrating a trend of humidification occurring in the eastern upstream and midstream regions. Combined Figures 1C, 8, the drought characteristics inferred by VPD might be related to frequent human activities concentrated in the midstream. Although VPD has been well-known to play an important role in the evolution mechanism of drought, it is not suitable to be independently applied as an indicator of drought in alpine regions like the YZR basin. Even more noteworthy is the fact that VPD does not have a clear drought classification yet. Given that VPD has a consistency with agricultural drought and a heterogeneity with meteorological drought, it is better to comprehensively analyze rather than pick out a single factor separately when identifying the influencing factors of drought in the YZR basin, and more attention should be paid to VPD variation characteristics to improve the understanding of drought variation and its impacts on ecosystem.
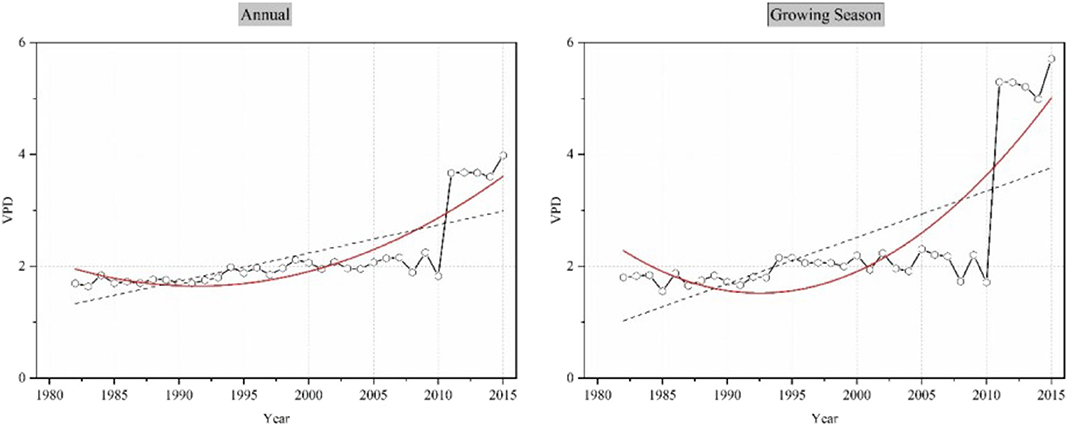
Figure 7. Variation trend of annual and growing season VPD (The red solid lines are curves fitted by quadratic polynomial. The black dashed lines are linear trend lines from 1982 to 2015).
Conclusion
Based on the scPDSI, SPEI, and SWDI derived from GLDAS and CRU datasets, a multi-index evaluation of drought characteristics during 1982–2015 in the Yarlung Zangbo River basin from the perspective of meteorology and agriculture was conducted in this study. Non-parametric statistical tests including M-K test, Moving t-test and Yamamoto test were adopted to implement long-term trend analysis and abrupt change detection, further exploring the possible causes of drought evolution. The conclusions are as follows:
(1) The whole basin presented a wetting trend during 1982 to 2015. Meteorological and agricultural drought of annual and growing season scales in the YZR basin showed an abrupt change from wetting to drying in the year of 2000, indicating a trend of alleviating first and then aggravating during 1982 to 2015. Meteorological drought represented by scPDSI and SPEI changed from moderate drought to moderate wet before 2000 while it was opposite after 2000, and agricultural drought alleviated from severe drought to moderate drought during 34 years.
(2) Both meteorological and agricultural drought in the basin showed a moderate drought in the downstream after 2000 and the upstream changed to slightly dry. The driest area indicated by meteorological drought in the basin moved from the midstream to the southeast downstream, while it indicated by agricultural drought shifted from the east midstream and north downstream to the south downstream.
(3) Non-significant increasing and non-significant decreasing trends of the annual and growing season meteorological drought consistently hold the greatest and almost equivalent proportions. Areas with extremely significant decreasing trend were mostly distributed in the west upstream and southwest downstream, whereas the midstream and northwest downstream mainly showed extremely significant increasing trends.
Data Availability Statement
Publicly available datasets were analyzed in this study. This data can be found here: Land Data Assimilation System (http://ldas.gsfc.nasa.gov/gldas/GLDASvegetation.php) and Climatic Research Unit (www.cru.uea.ac.uk/data/).
Author Contributions
LL: conceptualization, resources, and supervision. LL and JH: methodology. JH: software. QN, JH, and LL: validation. QN and JH: formal analysis and investigation. JH, HL, and QN: data curation and visualization. QN and LL: writing—original draft preparation and writing—review and editing. LL and ZX: project administration and funding acquisition. All authors contributed to the article and approved the submitted version.
Funding
This research was financially supported by the National Key R&D Program of China (No. 2018YFC1508702) and the National Natural Science Foundation of China (91647202, 41890822, and 51509247).
Conflict of Interest
The authors declare that the research was conducted in the absence of any commercial or financial relationships that could be construed as a potential conflict of interest.
Acknowledgments
The authors would like to thank the Climatic Research Unit at the University of East Anglia and the Global Land Data Assimilation System team for making the data freely available.
References
Ahmed, K., Shahid, S., Harun, S. B., and Wang, X. (2016). Characterization of seasonal droughts in balochistan province, Pakistan. Stoch. Environ. Res. Risk Assess. 30, 747–762. doi: 10.1007/s00477-015-1117-2
Alfieri, J. G., Blanken, P. D., Yates, D. N., and Steffen, K. (2007). Variability in the environmental factors driving evapotranspiration from a grazed rangeland during severe drought conditions. J. Hydrometeorol. 8, 207–220. doi: 10.1175/JHM569.1
Bachmair, S., Stahl, K., Collins, K., Hannaford, J., Acreman, M., Svoboda, M., et al. (2016a). Drought indicators revisited: the need for a wider consideration of environment and society. Wiley Interdiscip. Rev. Water 3, 516–536. doi: 10.1002/wat2.1154
Bachmair, S., Svensson, C., Hannaford, J., Barker, L. J., and Stahl, K. (2016b). A quantitative analysis to objectively appraise drought indicators and model drought impacts. Hydrol. Earth Syst. Sci. 20, 2589–2609. doi: 10.5194/hess-20-2589-2016
Bai, J., Cui, Q., Chen, D., Yu, H., Mao, X., Meng, L., et al. (2018). Assessment of the SMAP-Derived Soil Water Deficit Index (SWDI-SMAP) as an agricultural drought index in China. Remote Sens.10:1302. doi: 10.3390/rs10081302
Bai, X., Shen, W., Wu, X., and Wang, P. (2020). Applicability of long-term satellite-based precipitation products for drought indices considering global warming. J. Environ. Manage. 255:109846. doi: 10.1016/j.jenvman.2019.109846
Baik, J., Zohaib, M., Kim, U., Aadil, M., and Choi, M. (2019). Agricultural drought assessment based on multiple soil moisture products. J. Arid Environ. 167, 43–55. doi: 10.1016/j.jaridenv.2019.04.007
Bezdan, J., Bezdan, A., Blagojević, B., Mesaroš, M., Pejić, B., Vranešević, M., et al. (2019). SPEI-based approach to agricultural drought monitoring in vojvodina region. Water 11:1481. doi: 10.3390/w11071481
Chen, B., Li, H., Cao, X., Shen, W., and Jin, X. (2015). Vegetation pattern and spatial distribution of NDVI in the yarlung zangbo river basin of China. J. Desert Res. 35, 120–128. doi: 10.7522/j.issn.1000-694X
Chen, H., and Sun, J. (2018). Projected changes in climate extremes in China in a 1.5°C warmer world. Int. J. Climatol. 38, 3607–3617. doi: 10.1002/joc.5521
Chen, S., Gan, T., Tan, X., Shao, D., and Zhu, J. (2019). Assessment of CFSR, ERA-Interim, JRA-55, MERRA-2, NCEP-2 reanalysis data for drought analysis over China. Clim. Dyn. 53, 737–757. doi: 10.1007/s00382-018-04611-1
Chen, X., Mei, M., Ding, Y., Chao, Q., Song, Y., Wu, Q., et al. (2015). The impacts of climate change on several major projects in China. Progr Inquisit Mutat Climat. 11, 337–342. doi: 10.3969/j.issn.1673-1719.2015.05.007
Dai, A. (2011). Drought under global warming: a review. Wiley Interdiscip. Rev. Clim. Chang. 2, 45–65. doi: 10.1002/wcc.81
Ding, J., Yang, T., Zhao, Y., Liu, D., Wang, X., Yao, Y., et al. (2018). Increasingly important role of atmospheric aridity on tibetan alpine grasslands. Geophys. Res. Lett. 45, 2852–2859. doi: 10.1002/2017GL076803
Dong, Y., Chen, H., Yu, D., and Li, C. (2012). “Satellite observations on agricultural adaptation to drought in southwestern China,” in 2012 First International Conference on Agro-Geoinformatics (Shanghai: IEEE), 1–4. doi: 10.1109/Agro-Geoinformatics.2012.6311704
Feng, S., and Fu, Q. (2013). Expansion of global drylands under a warming climate. Atmos. Chem. Phys. 13, 10081–10094. doi: 10.5194/acp-13-10081-2013
Fu, C., and Wang, Q. (1992). The definition and detection of the abrupt climatic change. Sci. Atmos. Sin. 16, 482–493.
Greve, P., Orlowsky, B., Mueller, B., Sheffield, J., Reichstein, M., and Seneviratne, S. I. (2014). Global assessment of trends in wetting and drying over land. Nat. Geosci. 7, 716–721. doi: 10.1038/ngeo2247
Han, X., Zuo, D., Xu, Z., Cai, S., and Gao, X. (2018). Analysis of vegetation condition and its relationship with meteorological variables in the yarlung zangbo river basin of China. Proc. Int Assoc. Hydrol. Sci. 379, 105–112. doi: 10.5194/piahs-379-105-2018
Hao, Z., Hao, F., and Singh, V. P. (2016). A general framework for multivariate multi-index drought prediction based on Multivariate Ensemble Streamflow Prediction (MESP). J. Hydrol. 539, 1–10. doi: 10.1016/j.jhydrol.2016.04.074
Herrera, D., and Ault, T. (2017). Insights from a new high-resolution drought atlas for the Caribbean spanning 1950 to 2016. J. Clim. 30, 7801–7825. doi: 10.1175/JCLI-D-16-0838.1
Huang, S., Leng, G., Huang, Q., Xie, Y., Liu, S., Meng, E., et al. (2017). The asymmetric impact of global warming on US drought types and distributions in a large ensemble of 97 hydro-climatic simulations. Sci. Rep. 7:5891. doi: 10.1038/s41598-017-06302-z
Huang, S., Wang, L., Wang, H., Huang, Q., Leng, G., Fang, W., et al. (2019). Spatio-temporal characteristics of drought structure across China using an integrated drought index. Agric. Water Manage. 218, 182–192. doi: 10.1016/j.agwat.2019.03.053
Ji, L., and Peters, A. J. (2003). Assessing vegetation response to drought in the northern Great Plains using vegetation and drought indices. Remote Sens. Environ. 87, 85–98. doi: 10.1016/S0034-4257(03)00174-3
Jiang, L., Jiapaer, G., Bao, A., Kurban, A., Guo, H., Zheng, G., et al. (2019). Monitoring the long-term desertification process and assessing the relative roles of its drivers in Central Asia. Ecol. Indic. 104, 195–208. doi: 10.1016/j.ecolind.2019.04.067
Kelly, C., Ferrara, A., Wilson, G. A., Ripullone, F., Nolè, A., Harmer, N., et al. (2015). Community resilience and land degradation in forest and shrubland socio-ecological systems: evidence from Gorgoglione, Basilicata, Italy. Land Use Pol. 46, 11–20. doi: 10.1016/j.landusepol.2015.01.026
Konings, A. G., Williams, A. P., and Gentine, P. (2017). Sensitivity of grassland productivity to aridity controlled by stomatal and xylem regulation. Nat. Geosci. 10, 284–288. doi: 10.1038/ngeo2903
Lesk, C., Rowhani, P., and Ramankutty, N. (2016). Influence of extreme weather disasters on global crop production. Nature 529, 84–87. doi: 10.1038/nature16467
Lewinska, K., Ivits, E., Schardt, M., and Zebisch, M. (2016). Alpine forest drought monitoring in South Tyrol: PCA based synergy between scPDSI data and MODIS derived NDVI and NDII7 time series. Remote Sens. 8:639. doi: 10.3390/rs8080639
Li, B., Yu, Z., Liang, Z., and Acharya, K. (2014). Hydrologic response of a high altitude glacierized basin in the central tibetan plateau. Glob. Planet. Change 118, 69–84. doi: 10.1016/j.gloplacha.2014.04.006
Li, B., Zhou, W., Zhao, Y., Ju, Q., Yu, Z., Liang, Z., et al. (2015). Using the SPEI to assess recent climate change in the yarlung zangbo river basin, South Tibet. Water 7, 5474–5486. doi: 10.3390/w7105474
Li, H., Liu, L., Shan, B., Xu, Z., Niu, Q., Cheng, L., et al. (2019a). Spatiotemporal variation of drought and associated multi-scale response to climate change over the Yarlung Zangbo River Basin of Qinghai-Tibet Plateau, China. Remote Sens. 11:1596. doi: 10.3390/rs11131596
Li, X., He, B., Quan, X., Liao, Z., and Bai, X. (2015). Use of the Standardized Precipitation Evapotranspiration Index (SPEI) to characterize the drying trend in southwest China from 1982–2012. Remote Sens. 7, 10917–10937. doi: 10.3390/rs70810917
Li, X., Liu, L., Li, H., Wang, S., and Heng, J. (2019b). Spatiotemporal soil moisture variations associated with hydro-meteorological factors over the yarlung zangbo river basin in Southeast Tibetan Plateau. Int. J. Climatol. 40, 188–206. doi: 10.1002/joc.6202
Li, X., You, Q., Ren, G., Wang, S., Zhang, Y., Yang, J., et al. (2019c). Concurrent droughts and hot extremes in northwest China from 1961 to 2017. Int. J. Climatol. 39, 2186–2196. doi: 10.1002/joc.5944
Liu, J., Xu, Z., Zhao, H., Peng, D., and Zhang, R. (2018a). Spatiotemporal variation of extreme precipitation events in the yarlung zangbo river basin from 1973 to 2016, China. Mountain Res. 36, 750−764. doi: 10.7522/j.issn.10000240.2018.0070
Liu, J., Yao, Z., and Chen, C. (2007). Evolution trend and causation analysis of the runoff evolution in the yarlung zangbo river basin. J. Nat. Res. 122, 471–477. doi: 10.11849/zrzyxb.2007.03.017
Liu, J., Zhang, W., Deng, C., and Nie, N. (2018b). Spatiotemporal variations of snow cover over yarlung zangbo river basin in tibet from 2000 to 2014 and its response to key climate factors. J. Glaciol. Geocryol. 40, 643–654. doi: 10.7522/j.issn.1000-0240.2018.0070
Liu, L., Niu, Q., Heng, J., Li, H., and Xu, Z. (2019). Transition characteristics of the dry-wet regime and vegetation dynamic responses over the yarlung zangbo river basin, southeast qinghai-tibet plateau. Remote Sens. 11:1254. doi: 10.3390/rs11101254
Liu, L., Xu, Z., and Huang, J. (2012). Spatio-temporal variation and abrupt changes for major climate variables in the Taihu Basin, China. Stoch. Environ. Res. Risk Assess. 26, 777–791. doi: 10.1007/s00477-011-0547-8
Liu, M., Xu, X., Xu, C., Sun, A. Y., Wang, K., Scanlon, B. R., et al. (2017). A new drought index that considers the joint effects of climate and land surface change. Water Resour. Res. 53, 3262–3278. doi: 10.1002/2016WR020178
Liu, T., Zhang, X., Xiong, S., Qin, X., and Yang, X. (2002). Glacial environments on the tibetan plateau andglobal cooling. Quat. Int. 97, 133–139. doi: 10.1016/S1040-6182(02)00058-7
Liu, X. (2015). Analysis of the Meteorological and Hydrological Charateristics in the Yarlung Zangbo River Basins. Beijing: Tsinghua University.
Liu, X., Ren, L., Yuan, F., Xu, J., and Liu, W. (2012). Assessing vegetation response to drought in the Laohahe catchment, North China. Hydrol. Res. 43, 91–101. doi: 10.2166/nh.2011.134
Liu, X., Zhu, X., Pan, Y., Li, S., Liu, Y., and Ma, Y. (2016). Agricultural drought monitoring: progress, challenges, and prospects. J. Geogr. Sci. 26, 750–767. doi: 10.1007/s11442-016-1297-9
Liu, Z., Yao, Z., Huang, H., Wu, S., and Liu, G. (2014). Land use and climate changes and their impacts on runoff in the yarlung zangbo river basin, China. Land Degrad. Dev. 25, 203–215. doi: 10.1002/ldr.1159
Luo, C., Bao, X., Wang, S., Zhu, X., Cui, S., Zhang, Z., et al. (2015). Impacts of seasonal grazing on net ecosystem carbon exchange in alpine meadow on the tibetan plateau. Plant Soil 396, 381–395. doi: 10.1007/s11104-015-2602-6
Lv, L., Liu, X., Zhou, H., and Wu, L. (2013). Analysis on the change trend of the annual runoff in the middle-lower yarlung zangbo river. Yellow River 35, 27–29. doi: 10.3969/j.issn.1000-1379.2013.05.009
Mann, H. B. (1945). Non-parametric tests against trend. Econometrica 13, 245–259. doi: 10.2307/1907187
Martínez-Fernández, J., González-Zamora, A., Sánchez, N., and Gumuzzio, A. (2015). A soil water based index as a suitable agricultural drought indicator. J. Hydrol. 522, 265–273. doi: 10.1016/j.jhydrol.2014.12.051
Martínez-Fernández, J., González-Zamora, A., Sánchez, N., Gumuzzio, A., and Herrero-Jiménez, C. M. (2016). Satellite soil moisture for agricultural drought monitoring: assessment of the SMOS derived soil water deficit index. Remote Sens. Environ. 177, 277–286. doi: 10.1016/j.rse.2016.02.064
Massmann, A., Gentine, P., and Lin, C. (2019). When does vapor pressure deficit drive or reduce evapotranspiration? J. Adv. Model. Earth Syst. 11, 3305–3320. doi: 10.1029/2019MS001790
McKee, T. B., Doesken, N. J., and Kleist, J. (1993). “The relationship of drought frequency and duration to time scales,” in Eighth Conference on Applied Climatology (Anaheim, CA: American Meteorological Society).
Mishra, A. K., and Singh, V. P. (2010). A review of drought concepts. J. Hydrol. 391, 202–216. doi: 10.1016/j.jhydrol.2010.07.012
Mishra, A. K., and Singh, V. P. (2011). Drought modeling-A review. J. Hydrol. 403, 157–175. doi: 10.1016/j.jhydrol.2011.03.049
Novick, K. A., Ficklin, D. L., Stoy, P. C., Williams, A. C., Bohrer, G., Oishi, A. C., et al. (2016). The increasing importance of atmospheric demand for ecosystem water and carbon fluxes. Nat. Clim. Chang. 6:1023. doi: 10.1038/nclimate3114
Palmer, W. C. (1965). Meteorological Drought. Washington, DC: US Department of Commerce Weather Bureau.
Penman, H. L. (1948). Natural evaporation from open water, bare soil and grass. Proc. R. Soc. London Ser. A Math. Phys. Eng. Sci. 193, 120–145. doi: 10.1098/rspa.1948.0037
Rawson, H. M., Begg, J. E., and Woodward, R. G. (1977). The effect of atmospheric humidity on photosynthesis, transpiration and water use efficiency of leaves of several plant species. Planta 134, 5–10. doi: 10.1007/BF00390086
Schoppach, R., and Sadok, W. (2012). Differential sensitivities of transpiration to evaporative demand and soil water deficit among wheat elite cultivars indicate different strategies for drought tolerance. Environ. Exp. Bot. 84, 1–10. doi: 10.1016/j.envexpbot.2012.04.016
Schwalm, C. R., Anderegg, W. R. L., Michalak, A. M., Fisher, J. B., Biondi, F., Koch, G., et al. (2017). Global patterns of drought recovery. Nature 548, 202–205. doi: 10.1038/nature23021
Shao, D., Chen, S., Tan, X., and Gu, W. (2018). Drought characteristics over China during 1980-2015. Int. J. Climatol. 38, 3532–3545. doi: 10.1002/joc.5515
Sheffield, J., Wood, E. F., and Roderick, M. L. (2012). Little change in global drought over the past 60 years. Nature 491, 435–438. doi: 10.1038/nature11575
Shen, M., Piao, S., Cong, N., Zhang, G., and Jassens, I. A. (2015). Precipitation impacts on vegetation spring phenology on the tibetan plateau. Glob. Change Biol. 21, 3647–3656. doi: 10.1111/gcb.12961
Shiru, M. S., Shahid, S., Chung, E., and Alias, N. (2019). Changing characteristics of meteorological droughts in Nigeria during 1901–2010. Atmos. Res. 223, 60–73. doi: 10.1016/j.atmosres.2019.03.010
Spinoni, J., Vogt, J. V., Naumann, G., Barbosa, P., and Dosio, A. (2018). Will drought events become more frequent and severe in Europe? Int. J. Climatol. 38, 1718–1736. doi: 10.1002/joc.5291
Teweldebirhan Tsige, D., Uddameri, V., Forghanparast, F., Hernandez, E. A., and Ekwaro-Osire, S. (2019). Comparison of meteorological- and agriculture-related drought indicators across Ethiopia. Water 11:2218. doi: 10.3390/w11112218
Tong, S., Lai, Q., Zhang, J., Bao, Y., and Zhang, F. (2017). Spatiotemporal drought variability on the Mongolian Plateau from 1980–2014 based on the SPEI-PM, intensity analysis and Hurst exponent. Sci. Total Environ. 15, 1557–1565. doi: 10.1016/j.scitotenv.2017.09.121
Van der Schrier, G., Barichivich, J., Briffa, K. R., and Jones, P. D. (2013). A scPDSI-based global data set of dry and wet spells for 1901-2009. J. Geophys. Res. Atmos. 118, 4025–4048. doi: 10.1002/jgrd.50355
Vicente-Serrano, S. M., Beguería, S., and López-Moreno, J. (2010). A multiscalar drought index sensitive to global warming: the standardized precipitation evapotranspiration index. J. Clim. 23, 1696–1718. doi: 10.1175/2009JCLI2909.1
Vicente-Serrano, S. M., Van der Schrier, G., Beguería, S., Azorin-Molina, C., and Lopez-Moreno, J. (2015). Contribution of precipitation and reference evapotranspiration to drought indices under different climates. J. Hydrol. 526, 42–54. doi: 10.1016/j.jhydrol.2014.11.025
Wagner, W., Hahn, S., Kidd, R., Melzer, T., Bartalis, Z., Hasenauer, S., et al. (2013). The ASCAT soil moisture product: a review of its specifications, validation results, and emerging applications. Meteorol. Z 22, 5–33. doi: 10.1127/0941-2948/2013/0399
Wang, H., Vicente-serrano, S. M., Tao, F., Zhang, X., Wang, P., Zhang, C., et al. (2016). Monitoring winter wheat drought threat in Northern China using multiple climate-based drought indices and soil moisture during 2000–2013. Agric. For. Meteorol. 228–229, 1–12. doi: 10.1016/j.agrformet.2016.06.004
Wang, L. (2016). Study on Hydrochemical Characteristics and Its Influencing Factors in YarlungTsangpo River Basin. Beijing: Institute of Geographic Sciences and Natural Resources Research, CAS.
Wells, N., Goddard, S., and Hayes, M. J. (2004). A self-calibrating Palmer Drought Severity Index. J. Clim. 17, 2335–2351. doi: 10.1175/1520-0442(2004)017<2335:ASPDSI>2.0.CO
White, D. H., and Walcott, J. J. (2009). The role of seasonal indices in monitoring and assessing agricultural and other droughts: a review. Crop Pasture Sci. 60:599. doi: 10.1071/CP08378
Yamamoto, R., Iwashima, T., Kazadi, S., and Hoshiai, M. (1985). Climatic jump: a hypothesis in climate diagnosis. J. Meteorol. Soc. Jpn. 63, 1157–1160. doi: 10.2151/jmsj1965.63.6_1157
Yang, M., Mou, Y., Meng, Y., Liu, S., Peng, C., and Zhou, X. (2020). Modeling the effects of precipitation and temperature patterns on agricultural drought in China from 1949 to 2015. Sci. Total Environ. 711:135139. doi: 10.1016/j.scitotenv.2019.135139
Yang, Q., Mingxing, L. I., Zheng, Z. Y., and Zhuguo, M. A. (2017). Regional applicability of seven meteorological drought indices in China. Sci. China Earth Sci. 147–162. doi: 10.1007/s11430-016-5133-5
You, Q., Kang, S., Wu, Y., Xu, Y., Zhang, Y., and Huang, J. (2007). Climate change over the yarlung zangbo river basin during 1961-2005. J. Geogr. Sci. 17, 409–420. doi: 10.1007/s11442-007-0409-y
Yu, M., Li, Q., Hayes, M. J., Svoboda, M. D., and Heim, R. R. (2014). Are droughts becoming more frequent or severe in china based on the standardized precipitation evapotranspiration index: 1951–2010? Int. J. Climatol. 34, 545–558. doi: 10.1002/joc.3701
Yuan, W., and Zhou, G. (2004). Theoratical study and research prospect on drought indices. Adv. Earth Sci. 19, 982–991. doi: 10.3321/j.issn:1001-8166.2004.06.016
Zambrano Mera, Y. E., Rivadeneira Vera, J. F., and Pérez-Martín, M. Á. (2018). Linking El niño southern oscillation for early drought detection in tropical climates: the ecuadorian coast. Sci. Total Environ. 643, 193–207. doi: 10.1016/j.scitotenv.2018.06.160
Zargar, A., Sadiq, R., Naser, B., and Khan, F. I. (2011). A review of drought indices. Environ. Rev. 19, 333–349. doi: 10.1139/a11-013
Zeng, Z., Wu, W., Zhou, Y., Li, Z., Hou, M., and Huang, H. (2019). Changes in reference evapotranspiration over Southwest China during 1960–2018: attributions and implications for drought. Atmosphere 10:705. doi: 10.3390/atmos10110705
Zhai, J., Su, B., Krysanova, V., Vetter, T., Gao, C., and Jiang, T. (2010). Spatial variation and trends in PDSI and SPI indices and their relation to streamflow in 10 large regions of China. J. Clim. 23, 649–663. doi: 10.1175/2009JCLI2968.1
Zhai, P., Zhou, B., and Chen, Y. (2018). A review of climate change attribution studies. J. Meteorol. Res. 32, 671–692. doi: 10.1007/s13351-018-8041-6
Zhang, B., AghaKouchak, A., Yang, Y., Wei, J., and Wang, G. (2019a). A water-energy balance approach for multi-category drought assessment across globally diverse hydrological basins. Agric. For. Meteorol. 264, 247–265. doi: 10.1016/j.agrformet.2018.10.010
Zhang, D., Zhang, L., Yang, J., and Feng, G. (2010). The impact of temperature and precipitation variation on drought in China in last 50 years. Acta Phys. Sin. 59, 655–663. doi: 10.7498/aps.59.655
Zhang, Q., Fan, K., Sing, V. P., Song, C., Xue, C., and Sun, P. (2019b). Is Himalayan-Tibetan Plateau “drying”? Historical estimations and future trends of surface soil moisture. Sci. Total Environ. 658, 374–384. doi: 10.1016/j.scitotenv.2018.12.209
Zhang, Q., Kong, D., Singh, V. P., and Shi, P. (2017). Response of vegetation to different time-scales drought across China: spatiotemporal patterns, causes and implications. Glob. Planet. Change 152, 1–11. doi: 10.1016/j.gloplacha.2017.02.008
Zhang, Q., Yu, H. Q., Sun, P., Singh, V. P., and Shi, P. (2019c). Multisource data based agricultural drought monitoring and agricultural loss in China. Glob. Planetary Change 172, 298–306. doi: 10.1016/j.gloplacha.2018.10.017
Zhao, F. F., Xu, Z. X., Huang, J. X., and Li, J. Y. (2008). Monotonic trend and abrupt changes for major climate variables in the headwater catchment of the yellow river basin. Hydrol. Process. 22, 4587–4599. doi: 10.1002/hyp.7063
Zhou, S., Wang, J., Xu, L., Wang, X., Colgan, P. M., and Mickelson, D. M. (2010). Glacial advances in southeastern tibet during late quaternary and their implications for climatic changes. Quat. Int. 218, 58–66. doi: 10.1016/j.quaint.2009.11.026
Zhou, S., Williams, A. P., Berg, A. M., Cook, B. I., Zhang, Y., Hagemann, S., et al. (2019a). Land-atmosphere feedbacks exacerbate concurrent soil drought and atmospheric aridity. Proc. Natl. Acad. Sci. U.S.A. 116, 18848–18853. doi: 10.1073/pnas.1904955116
Zhou, S., Zhang, Y., Park, W. A., and Gentine, P. (2019b). Projected increases in intensity, frequency, and terrestrial carbon costs of compound drought and aridity events. Sci. Adv. 5:u5740. doi: 10.1126/sciadv.aau5740
Zhu, Q., Luo, Y., Xu, Y., Tian, Y., and Yang, T. (2019). Satellite soil moisture for agricultural drought monitoring: assessment of SMAP-derived soil water deficit index in xiang river basin, China. Remote Sens. 11:362. doi: 10.3390/rs11030362
Keywords: drought, climate change, dry-wet regime, spatio-temporal, alpine, Tibetan Plateau
Citation: Niu Q, Liu L, Heng J, Li H and Xu Z (2020) A Multi-Index Evaluation of Drought Characteristics in the Yarlung Zangbo River Basin of Tibetan Plateau, Southwest China. Front. Earth Sci. 8:213. doi: 10.3389/feart.2020.00213
Received: 13 April 2020; Accepted: 20 May 2020;
Published: 24 June 2020.
Edited by:
Xingcai Liu, Chinese Academy of Sciences, ChinaReviewed by:
Xiuping Li, Institute of Tibetan Plateau Research (CAS), ChinaGuangtao Fu, University of Exeter, United Kingdom
Copyright © 2020 Niu, Liu, Heng, Li and Xu. This is an open-access article distributed under the terms of the Creative Commons Attribution License (CC BY). The use, distribution or reproduction in other forums is permitted, provided the original author(s) and the copyright owner(s) are credited and that the original publication in this journal is cited, in accordance with accepted academic practice. No use, distribution or reproduction is permitted which does not comply with these terms.
*Correspondence: Liu Liu, bGl1bGl1QGNhdS5lZHUuY24=