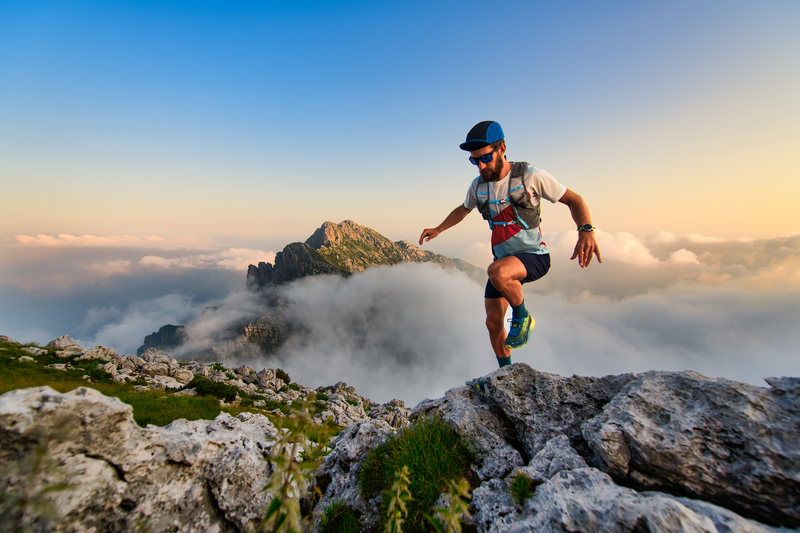
94% of researchers rate our articles as excellent or good
Learn more about the work of our research integrity team to safeguard the quality of each article we publish.
Find out more
ORIGINAL RESEARCH article
Front. Earth Sci. , 03 April 2020
Sec. Interdisciplinary Climate Studies
Volume 8 - 2020 | https://doi.org/10.3389/feart.2020.00090
This article is part of the Research Topic Climate, Land Use, and Fire: Can Models Inform Management? View all 8 articles
Variations in global patterns of burning and fire regimes are relatively well measured, however, the degree of influence of the complex suite of biophysical and human drivers of fire remains controversial and incompletely understood. Such an understanding is required in order to support current fire management and to predict the future trajectory of global fire patterns in response to changes in these determinants. In this study we explore and compare the effects of four fundamental controls on fire, namely the production of biomass, its drying, the influence of weather on the spread of fire and sources of ignition. Our study area is southern Australia, where fire is currently limited by either fuel production or fuel dryness. As in most fire-prone environments, the majority of annual burned area is due to a relatively small number of large fires. We train and test an Artificial Neural Network’s ability to predict spatial patterns in the probability of large fires (>1,250 ha) in forests and grasslands as a function of proxies of the four major controls on fire activity. Fuel load is represented by predicted forested biomass and remotely sensed grass biomass, drying is represented by fraction of the time monthly potential evapotranspiration exceeds precipitation, weather is represented by the frequency of severe fire weather conditions and ignitions are represented by the average annual density of reported ignitions. The response of fire to these drivers is often non-linear. Our results suggest that fuel management will have limited capacity to alter future fire occurrence unless it yields landscape-scale changes in fuel amount, and that shifts between, rather than within, vegetation community types may be more important. We also find that increased frequency of severe fire weather could increase the likelihood of large fires in forests but decrease it in grasslands. These results have the potential to support long-term strategic planning and risk assessment by fire management agencies.
Fires in vegetation are controlled by four fundamental constraints: the production of biomass, its subsequent drying, the influence of weather on the spread of fire and sources of ignition (Archibald et al., 2009; Bradstock, 2010; Moritz et al., 2012). These constraints can be characterised as switches, all of which must be on for landscape fire to occur (Bradstock, 2010). Different fire regimes are characterised by differences in the proportion of time that each factor is ‘switched on’, with wildfire occurrence effectively limited by the factor least frequently switched on (‘the limiting switch’). The four factors are in turn a function of biophysical (e.g., climatic, edaphic, topographic, and vegetation variations) and anthropogenic influences, such as population density, land clearing and management practises (McKenzie and Kennedy, 2012; Giglio et al., 2013; Bistinas et al., 2014; Chuvieco et al., 2014). The strength and direction of such influences on fire varies substantially across biomes, climate types and continents, resulting in significant global, continental and regional scale variations in fire and fire regime patterns (Chuvieco et al., 2008; Archibald et al., 2013; Giglio et al., 2013; Pausas and Ribeiro, 2013). While such variations in the emergent global patterns of burning and fire regimes are relatively well measured, the degree of influence of the complex suite of biophysical and human drivers of fire remains controversial and incompletely understood (Bowman et al., 2011; Marlon et al., 2013; McWethy et al., 2013). A detailed understanding of the sensitivity of fire to potential changes in anthropogenic and biophysical determinants of fire is therefore needed to support fire management and predict the future trajectory of global fire patterns.
Numerous studies have attempted to account for the influence of key climatic, vegetation, and human influences on fire via conventional statistical approaches. For example, temperature, precipitation, water availability, atmospheric dryness, and vegetation type have been related to area burned in either univariate or multivariate, linear modelling approaches (e.g., Krawchuk et al., 2009; Williams et al., 2015; Nolan et al., 2016; Hoyos et al., 2017; Syphard et al., 2017). The influence of measures of population density, land clearing and agricultural activities have been explored using similar approaches, either independently or in concert with climatic and vegetation influences (Chuvieco et al., 2008; Archibald et al., 2013; Bistinas et al., 2014). Derived statistical models of this kind have been incorporated in a variety of coupled dynamic global vegetation and fire models and used to predict both contemporary and future patterns of fire and fire emissions (Aldersley et al., 2011; Kloster et al., 2012).
Despite the insights produced by these approaches, the comparative sensitivity of fire to the full range of determinants (i.e., fuel production, dryness, fire weather, and ignitions) is uncertain at a macro-scale (sub-continental to global). Until the relative sensitivity of fire to each of these determinants is known, it is difficult to ultimately predict how area burned and resultant fire regimes may respond to climatic and human changes. For example, while changes in climate may have caused area burned to increase as a function of increasing dryness in recent decades in some forested ecosystems (e.g., Bradstock et al., 2014; Abatzoglou and Williams, 2016; Holden et al., 2018), there is recognition of negative feedbacks such as lowered biomass production (e.g., Turco et al., 2018; Trauernicht, 2019) or positive feedbacks changed ignition patterns stemming from warming and drying climatic conditions (Mariani et al., 2018).
In this study we explore and compare the effects of all four fundamental controls on fire across temperate regions of southern Australia, representative of ecosystems where long-term fire activity is currently limited by either fuel production or fuel dryness (Boer et al., 2016). We use a relatively long-term (i.e., circa. 40 years) chronology of mapped fire records, which provides deeper temporal resolution (e.g., double the length) of fire compared with many studies based on the remote sensing archive. We focus on large fire probability because large fires typically account for the bulk of area burned and thus the structure of fire regimes (Reed and McKelvey, 2002; Malamud et al., 2005; Boer et al., 2008; Cui and Perera, 2008). Large fires also are often associated with major human and environmental impacts, such as loss of life and property in southern Australia (Gill, 2005) and elsewhere (Stephens et al., 2014). An understanding of the joint influences of the major controls on large fire activity therefore has the potential to inform management and provide a basis for predicting the future of risks to assets and environments as a function of climatic, environmental and human changes. We specifically ask:
• Are the controls on large fire probability consistent with expectations that biomass/fuel production, fuel dryness, ambient weather, and ignitions act as limiting constraints (i.e., when proxies of all four of these influences are concurrently examined)?
• How is large fire probability in two major pyromes, forests and grasslands, related to geographic variation in proxies of these four fundamental determinants?
• What are the long-term implications that emerge from a formal understanding of these influences, in terms of management and climatic and human change?
The study area is the southern Australian states of NSW (including the Australian Capital Territory), Victoria, South Australia and Tasmania, and the southwest corner of Western Australia (Figure 1). These regions were selected as they have long-term records of agency-mapped and validated fire history (>40 years). All other areas of Australia have focused on remote sensing methods to develop fire histories and do not span the same temporal range. The study covers 515,800 km2 of southern Australia incorporating 60 bioregions (Hutchinson et al., 2005) ranging from arid to alpine ecosystems. Elevation ranges from 0 to 2,000 m above sea level. Climates vary widely across the study region with mean annual precipitation ranging from 112 to 3,250 mm/year and mean annual temperatures ranging from 6 to 24°C (Australian Bureau of Meteorology1). Extant fire regimes in the study area span a wide range of frequencies (typically falling into groups of mostly every 5–20 years or every 20–100 years), intensities (from 0 to 100 kW m–1 to > 50,000 kW m–1) and dominant fire seasons (spring-summer to summer-autumn) (Murphy et al., 2013).
Figure 1. Study area, response variable and predictors. Study area (A) comprises southern Australian states and territories including southwest Western Australia. Response variable (B) is areas with a maximum burned area greater than 50% (1,250 ha). Predictors are biomass (C), dryness (D), fire weather (E), and ignitions (F). Predictor units are: (C) steady state litter or grass biomass in t ha–1; (D) proportion of the fire season where PET > precipitation; (E) proportion of the fire season where FFDI > 50; (F) number of ignitions per fire season.
We divided the study area into two basic pyromes based on dominant fuel types: grass or litter. The study area was partitioned into litter or grass fuel types using a data set consisting of field observations of fuel and vegetation attributes from 113 sites across Australia (Murphy et al., 2019) which shows a strong negative relationship between the maximum grass fuel percentage (i.e., the total fine dead fuel mass) and local tree cover (%) (Boer et al., 2016). We used this relationship to identify tree cover values associated with high grass fuel percentage (>50%, based on the 90th percentile of all grass fuel observations). Focusing on southern Australia (N = 40), the threshold was determined to be 38.5%, above which we classed the fuel type as litter and below which we classed it as grass.
Fire history datasets were obtained from fire agencies in Western Australia, South Australia, Victoria, New South Wales and Tasmania (Table 1). These datasets are typically polygons of area burnt by wildfire for the period 1975–2014 inclusive. For the purpose of analysis, the study area was divided into a regular 5 km grid using the Albers Equal Area projection (n = 20,632). Within each 5 × 5 km grid cell, we calculated the area burnt per year by wildfire and used these data to calculate the maximum area burnt over the 40 year period. To represent large fire occurrence, we created a binary variable where 1 represented a cell with a maximum burned area greater than 1,250 ha (i.e., half of the grid cell size) and 0 represented cells with no fire or with maximum burned area less than 1,250 ha during the entire study period. Fires included in this threshold account for 83% of the total maximum annual area burned. Note that by this definition it is possible for a single fire event to be represented as a large fire in multiple grid cells.
Environmental data were sourced to represent the four fundamental controls on fire activity (Table 1). Restricting the analysis to proxies of each of these controls, rather than a large pool of potentially relevant predictors, allowed for an explicit analysis of their role as determinants of area burned. Biomass was estimated separately for litter and grass systems. Litter biomass was modelled using established relationships between steady state surface fine fuel load, mean annual temperature and mean annual rainfall for the period 1990–2009 (Hijmans et al., 2005; Thomas et al., 2014). Biomass in grass-dominated fuel systems was estimated using a water balance and plant growth model, which integrates satellite imaging, grass biomass observations and climate data, for the period 2000–2009 (Carter et al., 2003). Dryness and weather measures focus on the austral fire season of spring and summer, when the majority of fire activity in this region takes place (Russell-Smith et al., 2007; Murphy et al., 2013; Williamson et al., 2016). We define fuel dryness as the proportion of the fire season where monthly potential evapotranspiration (PET) exceeds precipitation for the period 1990–2009. This measure of dryness, along with a measure of productivity, explained a large fraction of the variation (adj R2: 0.89) in maximum fire activity in forested and grassy systems in Australia (Boer et al., 2016).
We define fire weather as the proportion of the fire season where the McArthur Forest Fire Danger Index (FFDI) exceeds 50. FFDI is a measure of the difficulty of fire suppression that incorporates temperature, relative humidity, wind speed and a drought factor based largely on recent rainfall (McArthur, 1967; Noble et al., 1980). FFDI was calculated with the drought factor fixed (at 10) in order to separate the effects of ambient weather and recent dryness (Bradstock et al., 2009). On this adjusted scale, a value of 50 is indicative of extreme conditions, with the vast majority of property loss from major fires in Australia occurred during times when FFDI was above 50 (Blanchi et al., 2010). FFDI was calculated from maximum daily temperature, minimum daily relative humidity and mean daily wind speed for the period 1990–2009 (Jeffrey et al., 2001; McVicar et al., 2008). The proportion of the fire season with days over 50 was calculated from this daily dataset. Ignitions were represented by the frequency of ignitions per fire season as reported in the Australian Incident Reporting Standard from 2001 to 2012 (AFAC, 2012). While these represent the best available ignition data for the study area, as with similar ignition datasets in other countries they are subject to a range of limitations including missing data and uncertainty in location and cause (Collins et al., 2015; Costafreda-Aumedes et al., 2017). Wind speed (original scale 1 km), litter biomass (250 m), ignitions (points) and burned area (polygons) were re-sampled to the 5 km grid. Biomass and ignition data were log transformed for modelling purposes because they were skewed. Using these transformed variables, the strongest correlations were between biomass and dryness in forests (−0.88; Supplementary Figure 1), dryness and fire weather in grasslands (0.67) and fire weather and ignitions in grasslands (Supplementary Figure 2; see Supplementary Figures 3 and 4 for untransformed correlation matrices).
We used Artificial Neural Networks (ANN), a useful tool for dealing with complex non-linear relationships in environmental systems (Lek and Guegan, 1999; Gevrey et al., 2003). Wildfires provide numerous examples of modelling problems where the explicit form of the relationship between key variables is not known, thus making them ideal subjects for the use of ANNs (Vasilakos et al., 2009). The use of neural nets in wildfire research dates back over 20 years (Vega-Garcia et al., 1996) and is now widely applied along with other machine learning approaches on topics including fire weather (Lagerquist et al., 2017), fire severity mapping (Harris and Taylor, 2017; Collins et al., 2018) and wildfire prediction (Dutta et al., 2013; Gray et al., 2018).
We fitted single-layer-hidden-layer neural networks using the nnet package (Venables and Ripley, 2002) in R-statistical program v3.4.4 (R Development Core Team, 2018). The response was the occurrence of a large fire within a cell as a binary variable. Due to the low proportions of cells in which large fires occurred (11% forests, 0.6% grasslands), 0 values were down weighted to balance data sizes. Probabilities presented are therefore relative probabilities not absolute probabilities. We used a k-fold cross validation approach where data were randomly split into 10 groups, a model was built on 90% of the data and the remaining 10% were used for model validation, with the process repeated 10 times. Reproducibility was achieved by using the same randomly chosen initial seed. Model prediction accuracy was measured using the area under the curve (AUC) of the receiver operating characteristic (ROC) plot (Hanley and McNeil, 1982), averaged over the 10 folds. AUC values range from 0.5 to 1, where 0.5 implies random prediction and 1 represents perfect prediction. Model performance was considered poor at AUC values below 0.7, moderate at AUC values between 0.7 and 0.9 and strong at AUC values above 0.9 (McCune and Grace, 2002).
In environments of litter-dominated fuels, the fitted neural networks predicting the influence of the four controls on large fire probability in forests had an average AUC value of 0.70 across the 10 folds. The relationship between large forest fire probability and biomass was positive at steady state litter fuel load values up to about 24 t ha–1 and negative above that (Figure 2A). The relationship between large forest fire probability and dryness was positive and close to linear (Figure 2B). The relationship between fire weather and the probability of large forest fires was positive and resembled a logarithmic growth curve, though with broad confidence envelopes (Figure 2C). The relationship between large fire probability and ignition was highly non-linear (Figure 2D). For ignition rates >∼0.1 ignitions per fire season, there was no relationship with large fire occurrence but at the lowest level (<∼0.01 ignitions per fire season), fire probability increased exponentially though with high uncertainty. Between ∼0.01 and ∼0.1 ignitions per fire season there was a weakly negative relationship between ignitions and the probability of large forest fires.
Figure 2. Predicted response of large fire probability (1975–2014) in forests to each of the four major determinants of fire: biomass (A), dryness (B), fire weather (C), and ignitions (D). X-axis units are: (A) steady state litter fuel load in t ha–1; (B) proportion of the fire season where PET > precipitation; (C) proportion of the fire season where FFDI > 50; (D) number of ignitions per fire season. Dotted lines show 95% confidence interval.
In environments of grass-dominated fuels, the average AUC value across the 10 folds for fitted neural networks in grasslands was 0.80. The relationship between large grass fire probability and biomass was negative and close to linear (Figure 3A). The relationship between dryness and large grass fire probability was moderately positive, increasingly strongly at high dryness values and then decreasing equally sharply at the very highest dryness values (Figure 3B). Conversely, at low fire weather values there was a strongly positive relationship with fire probability but this was negative at moderate values and then remained stable at the highest fire weather values (Figure 3B). Similar to forest fires but at a much lower threshold (∼0.005 vs 0.1), the lowest ignition rates were associated with high probabilities of large grass fires, but within large confidence envelopes. Above these rates the probability of large fires was insensitive to further increases in ignition rate (Figure 3D). Forests and grasslands had markedly different distributions of the four proxies: biomass and ignition rates were much higher in forests, while grasslands were dryer and had much more severe fire weather conditions (Figures 2, 3).
Figure 3. As for Figure 2, but for grasslands. X axis units are: (A) mean annual grass biomass in t ha–1; (B) proportion of the fire season where PET > precipitation; (C) proportion of the fire season where FFDI > 50; (D) number of ignitions per fire season.
The relationship between the four fundamental controls of fire and the probability of large fires that was produced by the derived artificial neural network model broadly conformed to first principles and published evidence. In forests, dryness and fire weather were positively related to the probability of large fires. However, most other relationships between individual determinants and large fire probability in both forests and grasslands were more complex. The models fitted here build on the understanding developed from other modelling studies of the drivers of large fires and the relative importance of biomass and dryness in global fire activity. Our results are generally consistent with Meyn et al. (2007), who found that decreasing fuel moisture (increasing dryness) was important in promoting fire in a wide range of forests and other biomass-rich, rarely dry vegetation types, and Krawchuk and Moritz (2011), who found that mesic areas where biomass is relatively abundant experienced more fire activity as fuels dried, as indexed by soil moisture. Kelley et al. (2019) identified some forests (though not all) where fire regimes have shifted consistent with this relationship between fire activity and fuel moisture trends.
We found that the relationship between biomass and large fire probability in forests was initially positive but became negative at higher values. In contrast, increasing biomass in grasslands tended to be associated with decreased risk of large fires, perhaps because regions with low biomass are more sensitive to substantial but rare precipitation pulses that promote fuel build-up and continuity (O’Donnell et al., 2011). The relationship between dryness and large fire probability was positive for all dryness values in forests, but was positive in grasslands only up to values of ∼90% of the fire season having PET exceeding precipitation. Above this value of 90%, the relationship between dryness and large fires in grasslands was negative, suggesting that conditions conducive to extreme fuel dryness in grasslands may be insufficient for the extensive biomass growth required to support large fires. The positive relationship between fire weather and probability of large fires in forests is consistent with a number of studies globally spanning many forest types (Stavros et al., 2014; Rodrigues et al., 2019). In contrast, for grass-dominated fuels, modelled decreases in large fire probability with increasing fire weather severity suggest a possible association between the high FFDI experienced for extended periods in arid areas due to high temperatures and low humidity, and biomass levels insufficient to support large fire (King et al., 2013; Supplementary Figure 2). Further, grass fuels by their nature are well aerated and dry quickly relative to litter fuels, which are horizontal and packed against the soil surface thereby retaining more moisture. The model used here is limited in its ability to capture the contrasting and potentially interacting effects between biomass, dryness and fire weather, three fundamental determinants of large fire probability, in environments of grass-dominated fuels. In both grassy and forested systems, the probability of large fires was highest at very low ignition rates, albeit with considerable uncertainty around probability estimates. Above very low ignition rates, increasing ignition rates did not increase the probability of large fires. This pattern may reflect biases in the locality of ignitions, which tend to have highest probabilities near densely populated areas where large fires are less likely e.g., the wildland urban interface around towns and cities (Faivre et al., 2014; Collins et al., 2015; Clarke et al., 2019). Our analysis cannot deal with spatial issues of this kind and if we assume that the ignition relationship primarily reflects population density effects, then large fire probability is essentially insensitive to variation in ignitions, once this population size effect is notionally removed. The relationships we found between large fire probability and the fundamental controls on fire were derived at different scales in forests and grasslands and thus are not strictly equivalent. Biomass values and ignition rates were much higher in forests than in grasslands, whereas dryness and fire weather values were much higher in grasslands than forests.
In this modelling study we did not attempt to explain seasonal or inter-annual variability in fire activity in terms of corresponding temporal variability in each fundamental determinant of fire (Abatzoglou et al., 2018; Kelley et al., 2019). Nevertheless, because we used multi-decadal fire and predictor records, the relationships implicitly reflected this variation. Our model also integrated information at large spatial scales across southern Australia within two broad vegetation categories, grasslands and forests. Major structural and climatic variation exists within each of these categories. For example, the model does not distinguish between different woody fuel types (e.g., woodland, dry forest, wet forest) and therefore the results reflected the entire sub-continental domain of woody fuel types, rather than the responses specific locations or regions. An extension of this modelling approach to address monthly to seasonal timescales and variation in vegetation structure may yield insights into the sensitivity of large fire probability to its fundamental determinants at a level potentially more relevant to fire managers. Further research could more directly explore the potential interactions between human effects, such as vegetation clearing/modification or infrastructure patterns, on each of the four primary determinants of fire probability, as done in other studies (Bistinas et al., 2014; Kelley et al., 2019) or the consider alternative proxy(s) for each determinant. A range of empirical relationships have been derived and could be used for this purpose, such as links between weather and fuel moisture (Meyn et al., 2007; Resco de Dios et al., 2015) or fire weather and ignition (Penman et al., 2013).
In forests, the sensitivity of large fire probability to biomass suggests the potential to decrease burned area by reducing fuel load through management, consistent with evidence from empirical and modelling studies across southern Australia (Boer et al., 2009; Price et al., 2015). The modelled relationship between biomass and large fire probability is positive from ∼10 to 24 t ha–1 and implies that reducing fuel load from 24 to 16t ha–1 leads to a reduction in large fire probability of about 50%, from 0.8 to 0.4. However, this averages across many vegetation types and ignores finer scale processes such as the location and rate of prescribed burning. For example, Cirulis et al. (2019) found that a 50% reduction in burnt area was possible at prescribed burning treatment rates of 10% p.a. in forests in the Australian Capital Territory, but that the same treatment rate would result in just a 20% decrease in burnt area for forests in the southeast of Tasmania. Further, there are limits to the risk reduction available through fuel management, due to cost and resource constraints, prevailing weather conditions, smoke effects on human health (e.g., Borchers Arriagada et al., 2019; Gazzard et al., 2019) and other factors such as potential negative impacts of unseasonal fire on plant populations via early or late season burning (Miller et al., 2019).
Climate change may alter fuel loads through changing temperature and rainfall patterns or through potential fertilisation effects of increased atmospheric carbon dioxide, but the magnitude of changes projected for this region (Thomas et al., 2014; Clarke et al., 2016) is generally lower than that required to significantly alter fire probability, based on the relationships we found between biomass and large fire probability in forests. While there have been relatively few studies of climate change impacts explicitly addressing fuel moisture, they suggest the potential for future increases in fuel dryness in many areas (Matthews et al., 2012; Liu, 2017). Our results indicate that increased dryness under climate change could potentially increase probability of large fires in forests, but have little effect (or even negative effects at very high dryness values) on large fire probability in grass-dominated fuels (Boer et al., 2016). The unprecedented burnt area of the 2019–2020 forest fires in eastern Australia, characterised by extreme preceding dryness, are consistent with this (Boer et al., 2020; Nolan et al., 2020). Our results suggest potentially opposing implications of projected increases in the severity of fire weather under climate change (Clarke and Evans, 2019; Dowdy et al., 2019). In forests, increasing fire weather could lead to higher probability of large fires, although this does not factor in potential shifts in seasonality (Miller et al., 2019). In contrast, our results indicated that in grasslands, increased severity of fire weather could decrease the probability of large fires (potentially indirectly via reduced biomass), at least for areas experiencing extreme fire danger conditions for more than ∼5% of the fire season. Our results indicate potentially complex effects of human populations on ignitions and large fire probability that need to be further unpacked in order to understand future changes in human populations and land use.
Our findings suggest that changes between, rather than within, vegetation communities, may have the greater potential to alter existing fire regimes. Over the domain of dryness and fire weather present in forests, shifts from litter-dominated to grass-dominated fuels may lead to a decrease in fire probability, even as risk in both systems would rise in response to increasing dryness or fire weather (Figure 4). Hence shifts from litter-driven forest systems to grass systems may be accompanied by fundamental changes in the prevailing fire regime and the relative importance of the four determinants (Bowman et al., 2013; Halofsky et al., 2013; Jiang et al., 2013; Boer et al., 2019). While increased dryness and fire weather both acted to increase large fire risk in forests, the other two determinants (biomass growth and dryness) had generally opposing effects in environments dominated by grass fuels. Such changes would have significant implications for not only fire management, but other factors such as biodiversity and carbon emissions. These interpretations assume a stationary fire-climate relationship, an assumption that is not tested here.
Figure 4. Potential future trajectory of large fire risk in currently forested areas. Shifts from litter-dominated to grass-dominated fuels may lead to a decrease in fire probability, even as risk in both systems would rise in response to increasing dryness or fire weather.
This study has the potential for further development and application by fire management agencies, not just because large fires are a significant issue, but also because the modelling approach and results we have developed begin to quantify the links between the fundamental biophysical determinants of fire and key outcomes such as probability of large fires. Such an approach, with further refinements such as seasonal analyses, may help to improve short-term (e.g., seasonal forecasting, emergency warnings) and long-term (projection of climate change impacts) management of fire to achieve core objectives such as risk reduction for people and property and maintenance of ecosystem processes and services. This will ultimately contribute to a broader agency understanding of climate change vulnerability and impacts, and greater societal resilience and ability to co-exist with fire (Moritz et al., 2014; McWethy et al., 2019).
Fire history datasets are in a publicly accessible repository: www.data.gov.au. Ignition data was obtained from NSW Rural Fire Service and access requests should be directed to webmaster@rfs.nsw.gov.au. WorldClim data is available at: https://www.worldclim.org/. Wind speed data is available at www.csiro.au. AussieGrass and SILO climate data are available at www.longpaddock.qld.gov.au.
TP, MB, GC, JF, OP, and RB contributed conception and design of the study. TP, MB, OP, and HC contributed data preparation and analysis. RB, TP, and MB wrote sections of the manuscript. HC wrote the first draft of the manuscript. All authors contributed to manuscript revision, read and approved the submitted version.
OP’s salary was provided by the NSW Rural Fire Service. MB was partly financially supported by the Bushfires and Natural Hazards Cooperative Research Centre.
The authors declare that the research was conducted in the absence of any commercial or financial relationships that could be construed as a potential conflict of interest.
Thank you to the fire agencies in Western Australia, South Australia, Victoria, New South Wales, the Australian Capital Territory and Tasmania for providing fire history and ignition data. This manuscript was developed through a series of workshops at the Creswick campus of the University of Melbourne.
The Supplementary Material for this article can be found online at: https://www.frontiersin.org/articles/10.3389/feart.2020.00090/full#supplementary-material
Abatzoglou, J. T., and Williams, A. P. (2016). Impact of anthropogenic climate change on wildfire across western US forests. Proc. Natl. Acad. Sci. U.S.A. 113, 11770–11775. doi: 10.1073/pnas.1607171113
Abatzoglou, J. T., Williams, A. P., Boschetti, L., Zubkova, M., and Kolden, C. A. (2018). Global patterns of interannual climate-fire relationships. Global. Change Biol. 24, 5164–5175. doi: 10.1111/gcb.14405
AFAC (2012). Australian Incident Reporting Standard (AIRS), Instructions for Incident Reporting. Melbourne: Australasian Fire and Emergency Service Authorities Council.
Aldersley, A., Murray, S. J., and Cornell, S. E. (2011). Global and regional analysis of climate and human drivers of wildfire. Sci. Total Environ. 409, 3472–3481. doi: 10.1016/j.scitotenv.2011.05.032
Archibald, S., Lehmann, C. E. R., Gomez-Dans, J. L., and Bradstock, R. A. (2013). Defining pyromes and global syndromes of fire regimes. Proc. Natl. Acad. Sci. U.S.A. 110, 6442–6447. doi: 10.1073/pnas.1211466110
Archibald, S., Roy, D. P., van Wilgen, B. W., and Scholes, R. J. (2009). What limits fire? An examination of drivers of burnt area in Southern Africa. Global Change Biol. 15, 613–630. doi: 10.1111/j.1365-2486.2008.01754.x
Bistinas, I., Harrison, S. P., Prentice, I. C., and Pereira, J. M. C. (2014). Causal relationships versus emergent patterns in the global controls of fire frequency. Biogeosciences 11, 5087–5101. doi: 10.5194/bg-11-5087-2014
Blanchi, R., Lucas, C., Leonard, J., and Finkele, K. (2010). Meteorological conditions and wildfire-related house loss in Australia. Int. J. Wildland Fire 19, 914–926. doi: 10.1071/wf08175
Boer, M., Bowman, D., Murphy, B., Cary, G., Cochrane, M., Fensham, R., et al. (2016). Future changes in climatic water balance drive transformational shifts in Australian fire regimes. Environ. Res. Lett. 11:065002.
Boer, M. M., Bradstock, R. A., Gill, A. M., Sadler, R. J., and Grierson, P. F. (2008). Spatial scale invariance of southern Australian forest fires mirrors the scaling behaviour of fire-driving weather events. Landscape Ecol. 23, 899–913.
Boer, M. M., Resco De Dios, V., and Bradstock, R. (2020). Unprecedented burn area of Australian mega forest fires. Nat. Clim. Change 10, 171–172. doi: 10.1038/s41558-020-0716-1
Boer, M. M., Resco De Dios, V., Stefaniak, E. Z., and Bradstock, R. A. (2019). A hydroclimatic model for the distribution of fire on Earth. Biogeosci. Discuss. doi: 10.5194/bg-2019-441
Boer, M. M., Sadler, R. J., Wittkuhn, R., McCaw, L., and Grierson, P. F. (2009). Long-term impacts of prescribed burning on regional extent and incidence of wildfires – evidence from fifty years of active fire management in SW Australian forests. Forest Ecol. Manag. 259, 132–142.
Borchers Arriagada, N., Horsley, J. A., Palmer, A. J., Morgan, G. G., Tham, R., and Johnston, F. H. (2019). Association between fire smoke fine particular matter and asthma-related outcomes: systematic review and meta-analysis. Environ. Res. 179:108777. doi: 10.1016/j.envres.2019.108777
Bowman, D., Murphy, B., Boer, M., Bradstock, R., Cary, G., Cochrane, M., et al. (2013). Forest fire management, climate change and the risk of catastrophic carbon losses. Front. Ecol. Environ. 11:66–68. doi: 10.1890/13.WB.005
Bowman, D. M. J. S., Balch, J., Artaxo, P., Bond, W. J., Cochrane, M. A., D’Antonio, C. M., et al. (2011). The human dimension of fire regimes on Earth. J. Biogeogr. 38, 2223–2236. doi: 10.1111/j.1365-2699.2011.02595.x
Bradstock, R. (2010). A biogeographic model of fire regimes in Australia: current and future implications. Global Ecol. Biogeogr. 19, 145–158.
Bradstock, R., Penman, T., Boer, M., Price, O., and Clarke, H. (2014). Divergent responses of fire to recent warming and drying across south-eastern Australia. Global Change Bio. 20, 1214–1228. doi: 10.1111/gcb.12449
Bradstock, R. A., Cohn, J., Gill, A. M., Bedward, M., and Lucas, C. (2009). Prediction of the probability of large fires in the Sydney region of south-eastern Australia using components of fire weather. In. J. Wildland Fire 18, 932–943.
Carter, J., Bruget, D., Hassett, R., Henry, B., Ahrens, D., Brook, K., et al. (2003). “Australian grassland and rangeland assessment by spatial simulation (Aussiegrass),” in Science for Drought, Proceedings of the National Drought Forum, Vol. 2003, eds R. Stone and I. Partridge (Brisbane: Queensland Department of Primary Industries), 152–159.
Chuvieco, E., Aguado, I., Jurdao, S., Pettinari, M. L., Yebra, M., Salas, J., et al. (2014). Integrating geospatial information into fire risk assessment. Int. J. Wildland Fire 23, 606–619. doi: 10.1071/WF12052
Chuvieco, E., Giglio, L., and Justice, C. (2008). Global characterization of fire activity: toward defining fire regimes from Earth observation data. Global Change Biol. 14, 1488–1502. doi: 10.1111/j.1365-2486.2008.01585.x
Cirulis, B., Clarke, H., Boer, M., Penman, T., Price, O., and Bradstock, R. (2019). Quantification of inter-regional differences in risk mitigation from prescribed burning across multiple management values. Int. J. Wildland Fire doi: 10.1071/WF18135
Clarke, H., and Evans, J. P. (2019). Exploring the future change space for fire weather in southeast Australia. Theor. Appl. Climatol. 136, 513–527. doi: 10.1007/s00704-018-2507-4
Clarke, H., Gibson, R., Cirulis, B., Bradstock, R. A., and Penman, T. D. (2019). Developing and testing models of the drivers of anthropogenic and lightning-caused ignition in southeastern Australia. J. Environ. Manage. 235, 34–41. doi: 10.1016/j.jenvman.2019.01.055
Clarke, H., Pitman, A. J., Kala, J., Carouge, C., Haverd, V., and Evans, J. P. (2016). An investigation of future fuel load and fire weather in Australia. Clim. Change 139, 591–605. doi: 10.1007/s10584-016-1808-9
Collins, K. M., Price, O. F., and Penman, T. D. (2015). Spatial and temporal patterns of fire ignitions in southeastern Australia. Int. J. Wildland Fire 24, 1098–1108.
Collins, L., Griffioen, P., Newell, G., and Mellor, A. (2018). The utility of random forests for wildfire severity mapping. Remote Sens. Environ. 216, 374–384.
Costafreda-Aumedes, S., Comas, C., and Vega-Garcia, C. (2017). Human-caused fire occurrence modelling in perspective: a review. Int. J. Wildl. Fire 26, 983–998. doi: 10.1071/WF17026
Cui, W., and Perera, A. H. (2008). What do we know about forest fire size distribution, and why is this knowledge useful for forest management? Int. J. Wildland Fire 17, 234–244. doi: 10.1071/WF06145
Dowdy, A. J., Ye, H., Pepler, A., Thatcher, M., Osbrough, S. L., Evans, J. P., et al. (2019). Future changes in extreme weather and pyroconvection risk factors for Australian wildfires. Sci. Rep. 9:10073. doi: 10.1038/s41598-019-46362-x
Dutta, R., Aryal, J., Das, A., and Kirkpatrick, J. B. (2013). Deep cognitive imaging systems enable estimation of continental-scale fire incidence from climate data. Sci. Rep. 3:3188. doi: 10.1038/srep03188
Faivre, N., Jin, Y., Goulden, M. L., and Randerson, J. T. (2014). Controls on the spatial pattern of wildfire ignitions in Southern California. Int. J. Wildland Fire 23, 799–811. doi: 10.1071/WF13136
Gazzard, T., Walshe, T., Galvin, P., Salkin, O., Baker, M., Cross, B., et al. (2019). What is the ‘appropriate’ fuel management regime for the Otway Ranges, Victoria, Australia? Developing a long-term fuel management strategy using the structured decision-making framework. Int. J. Wildland Fire doi: 10.1071/WF18131
Gevrey, M., Dimopoulos, L., and Lek, S. (2003). Review and comparison of methods to study the contribution of variables in artificial neural network models. Ecol. Model. 160, 249–264. doi: 10.1016/S0304-3800(02)00257-0
Giglio, L., Randerson, J. T., and van der Werf, G. R. (2013). Analysis of daily, monthly, and annual burned area using the fourth-generation global fire emissions database (GFED4). J. Geophys. Res. Biogeosci. 118, 317–328. doi: 10.1002/jgrg.20042
Gill, A. M. (2005). Landscape fires as social disasters: an overview of ‘the bushfire problem’. Glob. Environ. Change B 6, 65–80.
Gray, M. E., Zachmann, L. J., and Dickson, B. G. (2018). A weekly, continually updated dataset of the probability of large wildfires across western US forests and woodlands. Earth Syst. Sci. Data 10, 1715–1727. doi: 10.5194/essd-10-1715-2018
Halofsky, J. E., Hemstrom, M. A., Conklin, D. R., Halofsky, J. S., Kerns, B. K., and Bachelet, D. (2013). Assessing potential climate change effects on vegetation using a linked model approach. Ecol. Model. 266, 131–143. doi: 10.1016/j.ecolmodel.2013.07.003
Hanley, J. A., and McNeil, B. J. (1982). The meaning and use of the area under a receiver operating characteristic (ROC) curve. Radiology 143, 29–36.
Harris, L., and Taylor, A. H. (2017). Previous burns and topography limit and reinforce fire severity in a large wildfire. Ecosphere 8:e02019. doi: 10.1002/ecs2.2019
Hijmans, R. J., Cameron, S. E., Parra, J. L., Jones, P. G., and Jarvis, A. (2005). Very high resolution interpolated climate surfaces for global land areas. Int. J. Climatol. 25, 1965–1978.
Holden, Z. A., Swanson, A., Luce, C. H., Jolly, W. M., Maneta, M., Oyler, J. W., et al. (2018). Decreasing fire season precipitation increased recent western US forest wildfire activity. Proc. Natl. Acad. Sci. U.S.A. 115, E8349–E8357. doi: 10.1073/pnas.1802316115
Hoyos, N., Correa-Metrio, A., Sisa, A., Ramos-Fabiel, M. A., Espinosa, J. M., Restrepo, J. C., et al. (2017). The environmental envelope of fires in the Colombian Caribbean. Appl. Geogr. 84, 42–54. doi: 10.1016/j.apgeog.2017.05.001
Hutchinson, M. F., McIntyre, S., Hobbs, R. J., Stein, J. L., Garnett, S., and Kinloch, J. (2005). Integrating a global agro-climatic classification with bioregional boundaries in Australia. Global Ecol. Biogeogr. 14, 197–212.
Jeffrey, S. J., Carter, J. O., Moodie, K. M., and Beswick, A. R. (2001). Using spatial interpolation to construct a comprehensive archive of Australian climate data. Environm. Model. Software 16, 309–330.
Jiang, X., Rauscher, S. A., Ringler, T. D., Lawrence, D. M., Williams, A. P., Allen, C. D., et al. (2013). Projected future changes in vegetation in western North America in the twenty-first century. J. Clim. 26, 3671–3636.
Kelley, D. I., Bistinas, I., Whitley, R., Burton, C., Marthews, T. R., and Dong, N. (2019). How contemporary bioclimatic and human controls change global fire regimes. Nat. Clim. Change 9, 690–696. doi: 10.1038/s41558-019-0540-7
King, K. J., Cary, G. J., Bradstock, R. A., and Marsden-Smedley, J. B. (2013). Contrasting fire responses to climate and management: insights from two Australian ecosystems. Global Change Biol. 19, 1223–1235. doi: 10.1111/gcb.12115
Kloster, S., Mahowald, N. M., Randerson, J. T., and Lawrence, P. J. (2012). The impacts of climate, land use, and demography on fires during the 21st century simulated by CLM-CN. Biogeosciences 9, 509–525. doi: 10.5194/bg-9-509-2012
Krawchuk, M. A., and Moritz, M. A. (2011). Constraints on global fire activity vary across a resource gradient. Ecology 92, 121–132.
Krawchuk, M. A., Moritz, M. A., Parisien, M. A., Van Dorn, J., and Hayhoe, K. (2009). Global Pyrogeography: the Current and Future Distribution of Wildfire. PLoS One 4:e5102. doi: 10.1371/journal.pone.0005102
Lagerquist, R., Flannigan, M. D., Wang, X., and Marshall, G. A. (2017). Automated prediction of extreme fire weather from synoptic patterns in northern Alberta. Canada. Can. J. For. Res. 47, 1175–1183. doi: 10.1139/cjfr-2017-0063
Lek, S., and Guegan, J. F. (1999). Artificial neural networks as a tool in ecological modelling, an introduction. Ecol. Model. 120, 65–73. doi: 10.1016/S0304-3800(99)00092-7
Liu, Y. Q. (2017). Responses of dead forest fuel moisture to climate change. Ecohydrology 10:e1760. doi: 10.1002/eco.1760
Malamud, B. D., Millington, J. D. A., and Perry, G. L. W. (2005). Characterizing wildfire regimes in the United States. Proc. Natl. Acad. Sci. U.S.A. 102, 4694–4699. doi: 10.1073/PNAS.0500880102
Mariani, M., Holz, A., Veblen, T. T., Williamson, G., Fletcher, M.-S., and Bowman, D. M. J. S. (2018). Climate change amplifications of climate-fire teleconnections in the Southern Hemisphere. Geophys. Res. Lett. 45, 5071–5081. doi: 10.1029/2018GL078294
Marlon, J. R., Bartlein, P. J., Daniau, A. L., Harrison, S. P., Maezumi, S. Y., Power, M. J., et al. (2013). Global biomass burning: a synthesis and review of Holocene paleofire records and their controls. Q. Sci. Rev. 65, 5–25. doi: 10.1016/j.quascirev.2012.11.029
Matthews, S., Sullivan, A. L., Watson, P., and Williams, R. J. (2012). Climate change, fuel and fire behaviour in a eucalypt forest. Global Change Biol. 18, 3212–3223. doi: 10.1111/j.1365-2486.2012.02768.x
McArthur, A. G. (1967). Fire Behaviour in Eucalypt Forests. Forestry and Timber Bureau, Leaflet No. 107. Canberra: Commonwealth of Australia.
McCune, B., and Grace, J. (2002). Analysis of Ecological Communities. Glenden Beach, OR: MJM Software Design.
McKenzie, D., and Kennedy, M. C. (2012). Power laws reveal phase transitions in landscape controls of fire regimes. Nat. Commun. 3, 726. doi: 10.1038/ncomms1731
McVicar, T., Van Niel, T., Li, L., Roderick, M., Rayner, D., Ricciardulli, L., et al. (2008). Wind speed climatology and trends for Australia, 1975-2006: capturing the stilling phenomenon and comparison with near-surface reanalysis output. Geophys. Res. Lett. 35:L20403.
McWethy, D. B., Higuera, P. E., Whitlock, C., Veblen, T. T., Bowman, D. M. J. S., Cary, G. J., et al. (2013). A conceptual framework for predicting temperate ecosystem sensitivity to human impacts on fire regimes. Global Eco. Biogeogr. 22, 900–912. doi: 10.1111/geb.12038
McWethy, D. B., Schoennagel, T., Higuera, P. E., Krawchuk, M., Harvey, B. J., Metcalf, E. C., et al. (2019). Rethinking resilience to wildfire. Nat. Sustainab. 2, 797–804. doi: 10.1038/s41893-019-0353-8
Meyn, A., White, P. S., Buhk, C., and Jentsch, A. (2007). Environmental drivers of large, infrequent wildfires: the emerging conceptual model. Prog. Phys. Geogr. 31, 287–312.
Miller, R. G., Tangney, R., Enright, N. J., Fontaine, J. B., Merritt, D. J., Ooi, M. K. J., et al. (2019). Mechanisms of fire seasonality effects on plant populations. Trends Ecol. Evol. 34, 1104–1117. doi: 10.1016/j.tree.2019.07.009
Moritz, M. A., Batllori, E., Bradstock, R. A., Gill, A. M., Handmer, J., Hessburg, P. F., et al. (2014). Learning to coexist with wildfire. Nature 515, 58–66. doi: 10.1038/nature13946
Moritz, M. A., Parisien, M. A., Batllori, E., Krawchuk, M. A., Van Dorn, J., Ganz, D. J., et al. (2012). Climate change and disruptions to global fire activity. Ecosphere 3:art49. doi: 10.1890/ES11-00345.1
Murphy, B. P., Bradstock, R. A., Boer, M. M., Carter, J., Cary, G. J., Cochrane, M. A., et al. (2013). Fire regimes of Australia: a pyrogeographic model system. J. Biogeogr. 40, 1048–1058.
Murphy, B. P., Prior, L. D., Cochrane, M. A., Williamson, G. J., and Bowman, D. M. J. S. (2019). Biomass consumption by surface fires across Earth’s most fire prone continent. Global Change Biol. 25, 254–268. doi: 10.1111/gcb.14460
Noble, I. R., Bary, G. A. V., and Gill, A. M. (1980). McArthur’s fire-danger meters expressed as equations. Aust. J. Ecol. 5, 201–203.
Nolan, R. H., Boer, M. M., Collins, L., Resco de Dios, V., Clarke, H., Jenkins, M., et al. (2020). Causes and consequences of eastern Australia’s 2019–20 season of mega-fires. Global Change Biol. 26, 1039–1041. doi: 10.1111/gcb.14987
Nolan, R. H., de Dios, V. R., Boer, M. M., Caccamo, G., Goulden, M. L., and Bradstock, R. A. (2016). Predicting dead fine fuel moisture at regional scales using vapour pressure deficit from MODIS and gridded weather data. Remote Sens. Environ. 174, 100–108. doi: 10.1016/j.rse.2015.12.010
O’Donnell, A. J., Boer, M. M., McCaw, W. L., and Grierson, P. F. (2011). Vegetation and landscape connectivity control wildfire intervals in unmanaged semi-arid shrublands and woodlands in Australia. J., Biogeogr. 38, 112–124.
Pausas, J. G., and Ribeiro, E. (2013). The global fire productivity relationship. Global Ecol. Biogeogr. 22, 728–736. doi: 10.1111/geb.12043
Penman, T. D., Price, O. F., and Bradstock, R. (2013). Modelling the determinants of ignition in the Sydney Basin, Australia: implications for future management. Int. J. Wildland Fire 22, 469–478.
Price, O. F., Penman, T. D., Bradstock, R. A., Boer, M., and Clarke, H. (2015). Biogeographical variation in the potential effectiveness of prescribed fire in south-eastern Australia. J. Biogeogr. 42, 2234–2245.
R Development Core Team (2018). R: A Language and Environment for Statistical Computing. Vienna: The R Foundation for Statistical Computing. Available at: http://www.R-project.org/
Reed, W. J., and McKelvey, K. S. (2002). Power-law behaviour and parametric models for the size-distribution of forest fires. Ecol. Model. 150, 239.
Resco de Dios, V., Fellows, A. W., Nolan, R. H., Boer, M. M., Bradstock, R. A., Domingo, F., et al. (2015). A semi-mechanistic model for predicting the moisture content of fine litter. Agric. For. Meteorol. 203, 64–73. doi: 10.1016/j.agrformet.2015.01.002
Rodrigues, M., Costafreda-Aumedes, S., Comas, C., and Vega-Garcia, C. (2019). Spatial stratification of wildfire drivers towards enhanced definition of large-fire regime zoning and fire seasons. Sci. Total Environ. 689, 634–644. doi: 10.1016/j.scitotenv.2019.06.467
Russell-Smith, J., Yates, C. P., Whitehead, P. J., Smith, R., Craig, R., Allan, G. E., et al. (2007). Bushfires ‘down under’: patterns and implications of contemporary Australian landscape burning. Int. J. Wildland Fire 16, 361–377.
Stavros, E. N., Abatzoglou, J., Larkin, N. K., McKenzie, D., and Steel, E. A. (2014). Climate and very large wildland fires in the contiguous western USA. Int. J. Wildland Fire 23, 899–914. doi: 10.1071/Wf13169
Stephens, S. L., Burrows, N., Buyantuyev, A., Gray, R. W., Keane, R. E., Kubian, R., et al. (2014). Temperate and boreal forest mega-fires: characteristics and challenges. Front. Ecol. Environ. 12:115–122.
Syphard, A. D., Keeley, J. E., Pfaff, A. H., and Ferschweiler, K. (2017). Human presence diminishes the importance of climate in driving fire activity across the United States. Proc. Natl. Acad. Sci. U.S.A. 114, 201713885. doi: 10.1073/pnas.1713885114
Thomas, P. B., Watson, P. J., Bradstock, R. A., Penman, T. D., and Price, O. F. (2014). Modelling litter fine fuel dynamics across climate gradients in eucalypt forests of south-eastern Australia. Ecography 37, 827–837.
Trauernicht, C. (2019). Vegetation—Rainfall interactions reveal how climate variability and climate change alter spatial patterns of wildland fire probability on Big Island. Hawaii. Sci. Total Environ. 650, 459–469. doi: 10.1016/j.scitotenv.2018.08.347
Turco, M., Rosa-Cánovas, J. J., Bedia, J., Jerez, S., Montávez, J. P., Llasat, M. C., et al. (2018). Exacerbated fires in Mediterranean Europe due to anthropogenic warming projected with nonstationary climate-fire models. Nat. Commun. 9:3821. doi: 10.1038/s41467-018-06358-z
Vasilakos, C., Kalabokidis, K., Hatzopoulos, J., and Matsinos, I. (2009). Identifying wildland fire ignition factors through sensitivity analysis of a neural network. Nat. Hazards 50, 125–143.
Vega-Garcia, C., Lee, B. S., Woodard, P. M., and Titus, S. J. (1996). Applying neural network technology to human-caused wildfire occurrence prediction. Applications 10, 9–18.
Williams, A. P., Seager, R., Macalady, A. K., Berkelhammer, M., Crimmins, M. A., Swetnam, T. W., et al. (2015). Correlations between components of the water balance and burned area reveal new insights for predicting forest fire area in the southwest United States. Int. J. Wildland Fire 24, 14–26.
Keywords: wildfire, drivers, biomass, fuel moisture, dryness, fire weather, ignition, Australia
Citation: Clarke H, Penman T, Boer M, Cary GJ, Fontaine JB, Price O and Bradstock R (2020) The Proximal Drivers of Large Fires: A Pyrogeographic Study. Front. Earth Sci. 8:90. doi: 10.3389/feart.2020.00090
Received: 28 October 2019; Accepted: 16 March 2020;
Published: 03 April 2020.
Edited by:
John T. Abatzoglou, University of Idaho, United StatesReviewed by:
Clay Trauernicht, University of Hawaii, United StatesCopyright © 2020 Clarke, Penman, Boer, Cary, Fontaine, Price and Bradstock. This is an open-access article distributed under the terms of the Creative Commons Attribution License (CC BY). The use, distribution or reproduction in other forums is permitted, provided the original author(s) and the copyright owner(s) are credited and that the original publication in this journal is cited, in accordance with accepted academic practice. No use, distribution or reproduction is permitted which does not comply with these terms.
*Correspondence: Hamish Clarke, aGFtaXNoY0B1b3cuZWR1LmF1
Disclaimer: All claims expressed in this article are solely those of the authors and do not necessarily represent those of their affiliated organizations, or those of the publisher, the editors and the reviewers. Any product that may be evaluated in this article or claim that may be made by its manufacturer is not guaranteed or endorsed by the publisher.
Research integrity at Frontiers
Learn more about the work of our research integrity team to safeguard the quality of each article we publish.