- 1Key Laboratory of Regional Climate-Environment for Temperate East Asia, Institute of Atmospheric Physics, Chinese Academy of Sciences, Beijing, China
- 2University of Chinese Academy of Sciences, Beijing, China
Wetlands are complex and diverse ecosystems, substantially contributing to natural capital. Projected variations in natural and anthropogenic scenarios are likely to alter wetland dynamics by substantially impacting the hydrological and ecosystem functions. This study focuses on monitoring the probable impacts of land use change and El Niño Southern Oscillation (ENSO) variations on wetlands dynamics by analyzing the magnitude and extent of global wetland inundation trends during the study period. A microwave dataset was used to study the trends and interannual variability of surface inundation extent of global lakes, rivers, reservoirs, and wetland classes. Between 1995 and 2015, the average rate of increase in surface inundation extent of global waterbodies and wetlands of 7029.6 km2 per year occurred with the average inundation of 2.9 million km2. Whereas, 1998–2004, 2005–2009, and 2010–2015 have shown significant inter-annual variabilityduring 21 years of study period. The maximum contribution of 3.06 million km2 inundation has been recorded during the strong El Niño year of 2010. Swamps flooded forests and coastal wetlands has shown the most significant increase in surface inundation extent. Our results showed a positive lag correlation between Niño 3.4 sea surface temperature (SST) anomalies and surface inundation of different Global Lakes and Wetland classes. Moreover, lakes, rivers, reservoirs, and wetlands have revealed varying responses to different anthropogenic drivers like cropland (CL), natural vegetation (NV), and urban land (UL). CL, NV and barren land (BL) buffered region has presented strong negative connection with coastal inundation extent. Swamps demonstrated strong positive correlation with urban-land and coastal with shrub/grass land cover type. Through this study the extent of impact projected by climatic oscillations and anthropogenic drivers to water bodies and wetlands can be analyzed for well-informed conflict management and decision-making practices for minimizing the human driven impact on natural water systems.
Introduction
Wetlands, the ecosystem known for its natural recycling strength, contributes in controlling and distribution of the nutrient loads, with the extensive ability to support biodiversity greater than that of tropical rainforests (Raisin et al., 1999; Jones et al., 2009; Ramsar Convention on Wetlands, 2015). Wetlands are natural resources which should not only be considered as ecosystems imperative for the biogeochemical cycles but also for contributing to the global social and economic capital (Ozesmi and Bauer, 2002). Open water bodies like lakes alone cannot be considered for defining wetlands rather wetlands are the land features defined with seasonal, sporadic or permanent water saturated soils and are hydrological buffers to global landscape (Agarwal and Garg, 2009; Department of Water, 2009; Junk et al., 2013; Meng et al., 2016). The criterion for defining wetlands rather stays ambiguous but according to most stated sources wetlands are natural or human made geographical landscapes with temporary or permanent presence of marshes, fens, peatlands or water, either in static or flowing state (National Research Council, 1995; Lehner and Doll, 2004; Ramsar Convention on Wetlands, 2013). Although wetlands secure a very small percentage of earth’s surface, still their contributions to sustain the earth processes is critical (Birkett et al., 1998; Erwin, 2009; Kayranli et al., 2010).
Wetlands with larger stocks of mineral and organic matter provides suitable environment for methanogenesis emitting methane as a byproduct (Zhu et al., 2014; Zhang et al., 2017). The significance of this ecosystem is embedded into its unique property of both as carbon source and sink under varying environmental and meteorological conditions (Erwin, 2009). Wetlands signify and distinguish its contribution to ecosystems services by contributing a net total of 12% to the global carbon pool (Saunois et al., 2016). Wetland ecosystems are considered to be both ecologically significant and vulnerable due to the climatic changes observed globally and are considered robust ecosystems because of their contribution to earth’s methane budget (Lee and Yeh, 2009; Mitsch et al., 2013; Zhu et al., 2014). Moreover, the contribution of this ecosystem to climate forcing has been reflected, attributing to the complex biogeochemical processes, highlighting their vital impact on radiative feedback, and forcing (Dlugokencky et al., 2011; Mitsch et al., 2013; Zhu et al., 2014; Hu et al., 2017; Vizza et al., 2017).
The global climate is undergoing variations which is ascribed to natural phenomenon or anthropogenic interferences (Guler and Dural, 2007). The observed changes in the climate systems around the globe has illustrated that the increase of extreme events will project strong impact on different regions of the world through intensified El Niño and La Niña, flooding and drought occurrences (Prigent et al., 2007; Junk et al., 2013). Although, El Niño Southern Oscillations (ENSO) occurs every 2–7 years with followed by warm and cool episodes, El Niño and La Niña, which interchangeably causes drastic variations in global precipitation (Holmgren et al., 2001). These variations in precipitation have shown strong correlation with sea surface temperature (SST) that is associated with ENSO (Mercier et al., 2002).
El Niño Southern Oscillations substantially influences the weather system not just in oceanic atmosphere but also in terrestrial ecosystems through controlling large scale atmospheric circulations (Dai and Wigley, 2000; Trenberth and Caron, 2000). The inter-decadal changes in pacific forcing affects the western and eastern pacific regions and bridges ENSO with climatic variations causing coupling effects of SST and precipitation fields (Chen and Kumar, 2002; Jia and Ge, 2017). The mechanism of radiative control of precipitation can be considered as the basic mechanism behind operating the atmospheric vapor pressure contributing to the rainfall which eventually contributes to the radiative cooling and convective heating, explained by earlier researchers (Trenberth and Caron, 2000). Wetland ecosystems reveal complex terrestrial temporal phasing with ENSO which contributes to the radiative control as higher methane emissions enters the atmosphere followed by excessive precipitation during La Niña periods (Hodson et al., 2011; Pandey et al., 2017; Zhang et al., 2018). The tidal salt marshes and coastal wetlands within a close vicinity of the sea are highly susceptible to sea level rise and coastal cyclones because of global changes (Klemas, 2011).
The human pressure can alter or inhibit ecosystems functioning ability as much as to reduce the biodiversity richness down to 31.7% (Newbold et al., 2015). Inferences from numerous studies have suggested that both the tidal and non-tidal wetland ecosystems will undergo changes due to climatic variations, natural radiative forcing mechanism along with anthropogenic disturbances underlined by lifestyles, livelihoods, structure of economy, and policy (Burkett and Kusler, 2000). The extensive land use practices targeting the global wetland and surrounding areas includes the excessive advancement of agricultural, grazing, and water management for fish farming industry (Verhoeven and Setter, 2010).
Ecosystems that are interlinked through complex pathways like lakes, rivers, reservoirs, and wetlands makes it difficult to comprehend the maximum impact by individual stimuli, natural, or anthropogenic. Hence, the lack of validation exists due to undefined and antecedent conditions like integrated watersheds, synergistic valuation of different hydrologic conditions (hydroperiods) leading to heterogeneity in results (Winter and Rosenberry, 1998). There has been gaps identified in the existing research and studies exhibiting the need to develop techniques that can be used for wetlands baseline inventory, assessment and monitoring at spatio-temporal scales with the global shift in natural oscillations (MacKay et al., 2009). Moreover, the knowledge and research on the global conversion of wetland area to other land use classes has been inadequate and inconsistent (MacKay et al., 2009; van Asselen et al., 2013).
Numerous studies have been conducted based on remote sensing both at regional and global scale to analyze the wetlands changes (Pham-Duc et al., 2017; Aires et al., 2018; Wohlfart et al., 2018; Li et al., 2019; Powell et al., 2019). Microwave datasets have an advantage of not being affected by the cloud cover with higher sensitivity to detect inundation changes (Schroeder et al., 2015). The synthetic aperture radar (SAR) is beneficial for distinguishing between forested wetlands and uplands forests (Klemas, 2011).
This research aims to address the science base behind the interannual variability in global inundation trends over the years. It not only pertains to monitor the changing wetlands globally but digging deep into the reasons of how the changes in natural oscillations like ENSO and anthropogenic drivers like land use change will affect the natural dynamics of wetlands.
Materials and Methods
Datasets
Global Lakes and Wetlands Dataset
The database global lakes and wetlands dataset – level 3 (GLWD), considered as a baseline, shows good agreement with the regional and global distribution of global lakes, and wetland extent. It also entails the ground truth data for global wetland sites and encompasses most of the Ramsar sites (Lehner and Doll, 2004). The GLWD has been developed and validated based on 7 different ground inventories, registries as well as analogous and digital maps covering the spatial extent of the water bodies, making it a spatially consistent, and reliable (Lehner and Doll, 2004). It is still widely used by scientists and researchers for global and regional studies (Aires et al., 2018; Ishizawa et al., 2019; Li et al., 2019).
The detailed geographically distribution of GLWD classes has been mapped in Figure 1A. Among the 12 features classes, 50–100% wetlands, and 25–50% wetlands are found in the north western hemisphere (25°N to 80°N). The wetland complex (10°S to 37°N latitude) mainly covers the Tibetan Plateau, north east of Himalayas and South Asia and South–East Asia, and intermittent wetlands are majorly concentrated between 40°S and 60°N. The arbitrary distribution of lakes, rivers, reservoirs, freshwater floodplain, and coastal wetlands is all around the globe. Some of the classes are geographically distributed within specific latitudes. Swamps flooded forests are concentrated around Amazon and Congo Basin of Central Africa (20°S to 10°N brackish wetlands are concentrated around Middle Eastern countries and southeast Australia (40°S to 50°N) and bogs fens and mires are mostly located around Hudson Bay in the north west and Lena and Ob in northeastern Russia (45°N to 75°N).
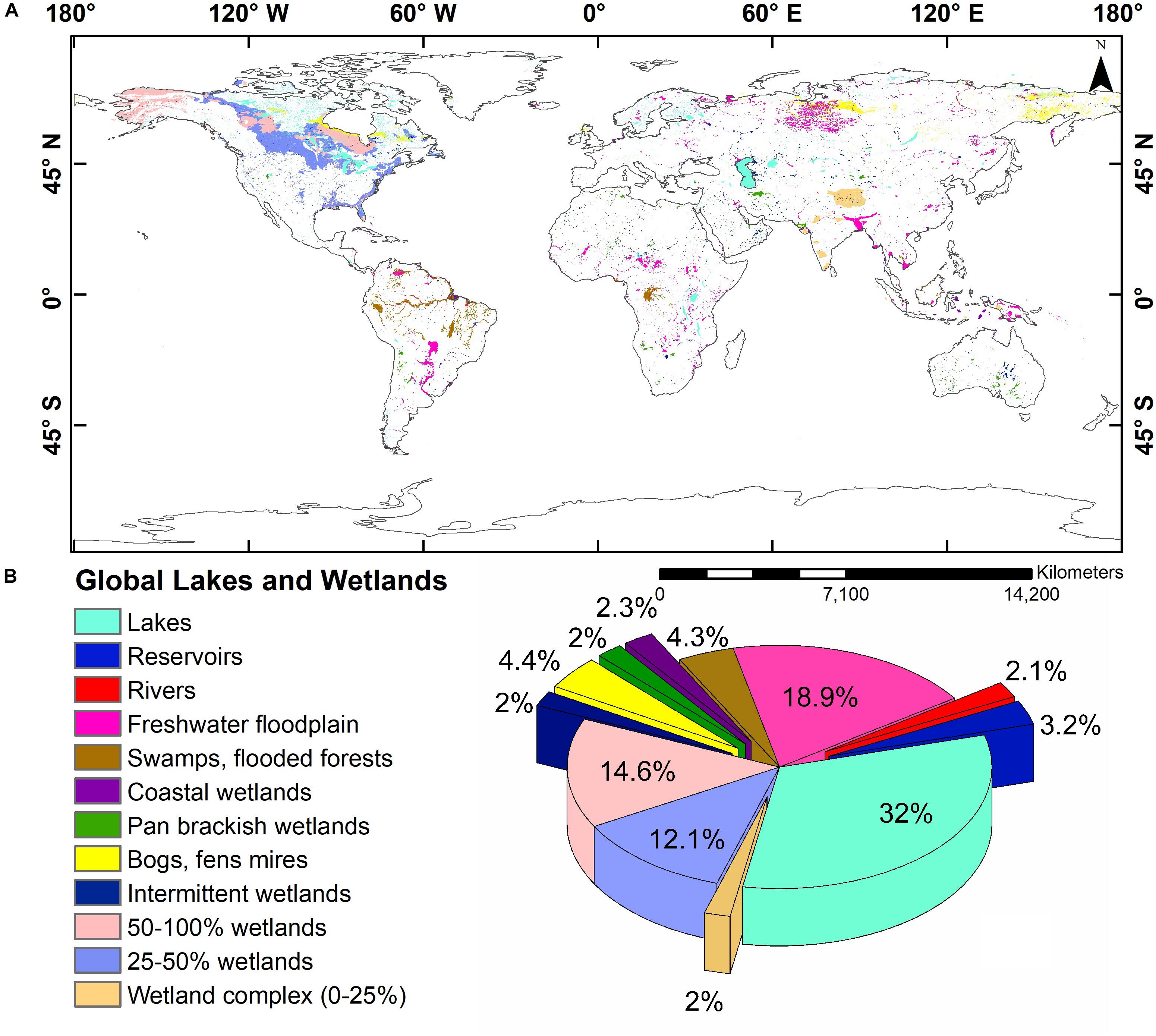
Figure 1. (A) Spatial distribution of Global Lakes and Wetlands – GLWD 3 (Lehner and Doll, 2004). (B) Average percentage contribution of GLWD classes from Surface Water Microwave Product Series (SWAMPS) data to global surface inundation between 1995 and 2015.
Fractional Surface Water – SWAMPS
The active and passive microwave satellite datasets are considered to be appropriate for the analysis of wetland and surface inundations extent (Schroeder et al., 2015; Poulter et al., 2017) as the sensitivity of microwave datasets is higher for liquid water. Surface water microwave product series (SWAMPS) covering the globe is used in this study which has been obtained from Alaska Satellite Facility Distributed Active Archive Center (ASF DAAC). This fully dynamics mixture dataset was developed by a research team based on the evaluation of spatial and temporal accuracies of fractional surface water (FW) (Schroeder et al., 2015) through comparing and combining different satellite products for this dataset. The product demonstrated good agreement with the independent inventories for global domain. The SWAMPS time series consisted of dataset over 1995–1999 and 2000–2015 with bimonthly and daily temporal resolution, respectively. The SWAMPS time series data is based follows EASE Grid 2.0 Coordinate system, with a spatial resolution of ∼25 km. The new version of the data has been improved through reducing the anomalous retrievals contributed by coastal, arid and semi–arid regions (Schroeder et al., 2015).
Land Use Land Cover Dataset
For the land use change assessment of global lakes and wetlands European Space Agency’s Climate Change Initiative dataset “Land Cover CCI” has been utilized (ESA, 2017) with an annual temporal resolution of 24 years (1992–2015) and spatial resolution of 300 m. This dataset has been compiled by utilizing 5 different observation systems in order to combine their global daily reflectance for the production of this long–term land use cover data (ESA, 2017). Originally this dataset was classified into 6 main classes (agriculture, forest, grassland, wetland, settlement, and others) with further division into sub classes. The maps showed a total number of 37 classifications categories based on United Nations Land Cover Classification System (UNLCCS). The different satellite products time series that have been used in the production of this dataset includes MERIS SR, AVHRR SR, PROBA–V SR, Annual LC, NDVI LS, and waterbody (ESA, 2017).
Niño 3.4 Sea Surface Temperature (SST)
Numerous indices have been developed and utilized by scientists to study the natural oscillations related to ENSO events like Oceanic Niño Index (ONI), Multivariate ENSO Index (MEI), Niño 3, Niño 4, and Niño 3.4 SST. Niño 3.4 region influences the atmospheric vapor pressure hence rendering the global precipitation patterns (Trenberth and Caron, 2000; Trenberth, 2011; Adler et al., 2017). For this study, Niño 3.4 SST has been used to calculate Niño 3.4 sea surface temperature anomaly (SSTA) (NOAA/NWS/CPC, 2005). The Niño 3.4 SST basically provides the SST values for the region (Niño 3 – 4) located between Pacific region marked for maximum perturbations dominated due to the variability caused by Hadley and Walker circulations defining into ENSO events. For this study, utilizing the Niño 3.4 SST values, we have calculated the Niño 3.4 SSTA for the period of 1995 to 2015 based on a 2-month running average. The ENSO episodes were defined by the conspicuous 5 or more continuous months with values ≥+0.5°C and ≤–0.5°C anomalies for El Niño and La Niña, respectively. Based on this, 87, 83, and 82 months out of the 252 months were ranked as El Niño, La Niña and ENSO neutral months.
Data Analysis
The surface water microwave product series time series data was initially procured in the EASE Grid 2.0 Coordinate system which was then adjusted according to the geographic coordinate system in order to achieve consistency in the datasets for accuracy in analysis and results. The mean values of the daily composites were considered to calculate the monthly values from the respective bimonthly and daily time series data for the required analysis. All the analysis has been performed at a global scale to focus on the inter–annual variability in surface inundation extent of different waterbodies and wetlands. We considered the wetland classes as identified by GLWD (Lehner and Doll, 2004). The subset raster files generated from GLWD were later used for plotting the monthly and yearly surface inundation changes and trends observed in individual wetland types. The spatial interannual variability has been plotted through applying the standard deviation function on maximum inundation fraction based on yearly values of 21 years data (1995–2015) from the SWAMPS. The maximum inundation fraction was used for plotting the interannual variability and spatial trend to ignore the seasonal inundation variations at higher latitudes for distinctive global map plots. The standard deviation function has been applied to present interannual variability of inundation. The spatial interannual anomaly trend values have been calculated by using the pixel by pixel linear regression.
A monthly correlation analysis of global inundation variations has been performed with Niño 3.4 SSTA natural oscillations ENSO. The statistical method used to analyze the extent of fluctuations in inundation patterns in different wetland classes affected by ENSO and land use change was computed through Pearson’s correlation coefficient technique. The Pearson’s correlation coefficient is used for the parametric measurement of the linear relationship between two variables in order to show the statistical evidence of any linearity found within the given variables. For the analysis, the starting month considered for Niño 3.4 was December of 1994 for performing 2- and 1-month lag correlation, respectively, against monthly inundations anomaly values for each class. The reason for applying 1- and 2-month lag correlation was due to the delayed response of terrestrial ecosystems to ENSO signal (Chen and Kumar, 2002). A total of 252 monthly anomalies in 21 years (1995–2015) of has been used to compute this correlation.
The statistical method used to analyze the extent of fluctuations between inundation patterns in different wetland classes separately with Niño 3.4 SSTA and land use changes has been computed through Pearson’s correlation. The starting month considered for Niño 3.4 SST was December of 1994 for performing 2- and 1-month lag correlation, respectively, against monthly inundation anomaly values for each GLWD class.
Land use change detection has been performed in order to analyze the land use changes and land conversion rates between each GLWD class and anthropogenic drivers from initial and final year (1995–2015) of study period. The SWAMPS dataset has been utilized to calculate the interannual inundation extent in different GLWD classes whereas, the land use change detection of the global wetland area has been performed using the Wetland Class from the land use land cover (LULC) dataset of ESA-CCI for the 21 years but the global wetland area change detection for 21 years has been done using the Wetland Land Cover class extracted from the ESA-CCI dataset.
After resampling and reclassifying the LULC dataset, 10 km buffered regions were created around each wetland class for quantifying the land use change rate in each year during 21 years (1995–2015). The land use considered for change detection included, NV, cropland (CL), wetlands (WL), Shrubs/grasslands (SL/GL), urban land (UL), and barren land (BL). While the correlation coefficients between NV, CL, SL/GL, UL, and BL interannual area values and surface inundation extent for each of the respective GLWD classes was calculated to analyze the variations in anthropogenic drivers with inundation variations of global lakes, rivers, reservoir, and wetlands.
Results
Temporal Inundation Variations in Global Wetlands
The average input toward the global total of surface water contributed by open waterbodies and wetland classes during the study period of 21 years has been displayed in Figure 1B. The maximum contribution to the surface inundation has been made by global lakes (32%) followed by freshwater floodplains (18.9%), 50–100% wetlands (14.6%), and 25–50% wetlands (12%). These observed values have shown greater extent of deviations when compared with the wetlands class contributions given in the baseline dataset of GLWD.
The 21 years regression coefficient (r2) value of 0.45 (p < 0.001) calculated for total GLWD area (Figure 2) suggests an overall increase in surface inundation trend. Figure 2 illustrates a significant increase in global wetland inundation over the years (slope = 7029.6 km2), along with the cyclic declining trend observed during the 3 episodes conspicuous between 1998 and 2004 (r2 = 0.76, slope = –9247; p < 0.01), 2005 to 2009 (r2 = 0.75, slope = –15013; p < 0.1), and 2010 to 2014 (r2 = 0.49, slope = –28324; p < 0.5) in surface inundation, repeating every 5–7 years. Furthermore, a sharp increase has been observed during 2010, marking it as the highest contributing year to wetland surface inundation during 21 years of study period.
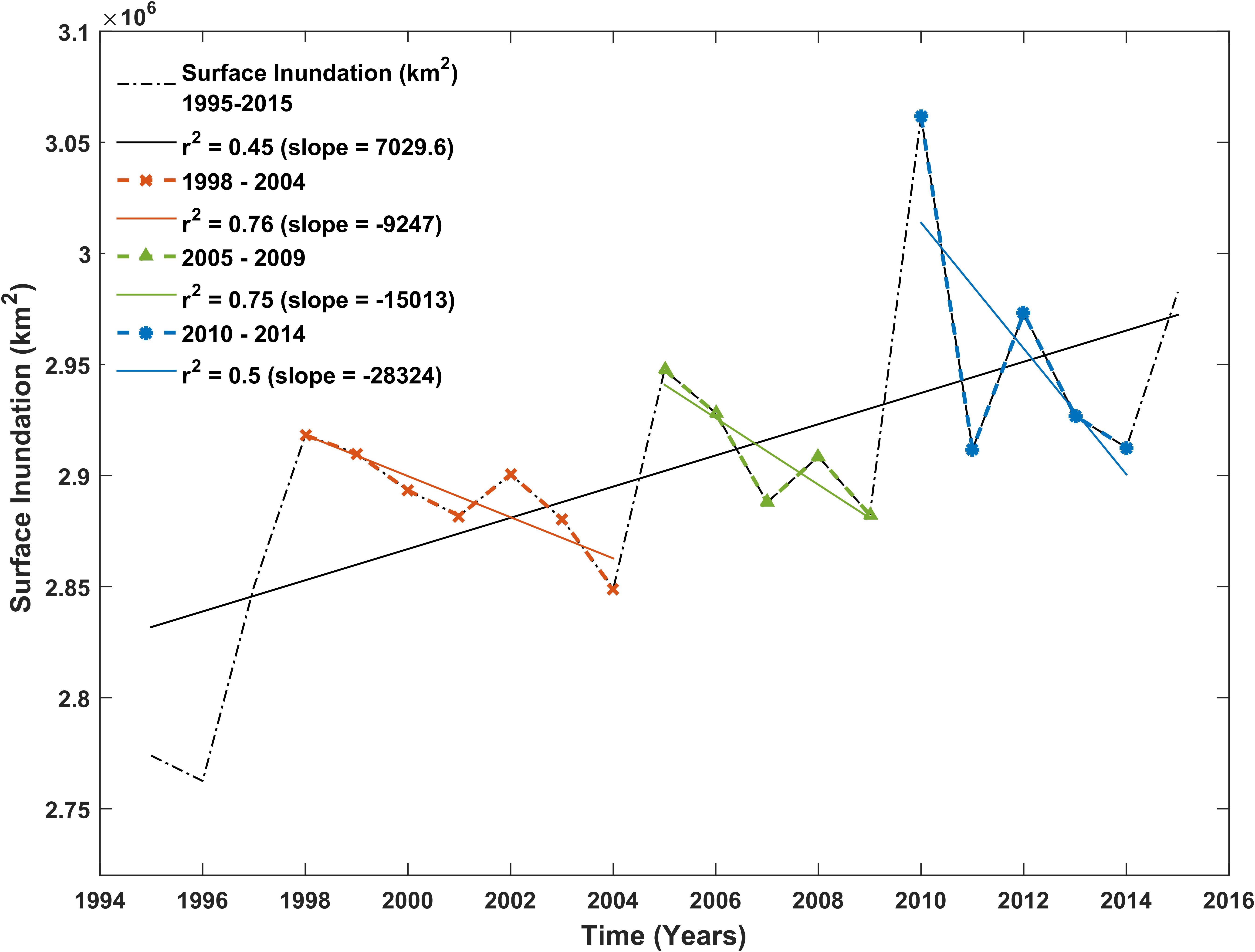
Figure 2. Temporal trends in global surface inundation extent (km2) variability from 1995 to 2015 (black). Three periods of declining trend were identified through 1998–2004 (orange), 2005–2009 (green), and 2010–2015 (blue). The open water bodies like lakes and reservoirs and wetland classes of swamps and coastal wetlands have shown the most significant increase in inundation trend.
The rate of change in surface area inundation of different GLWD classes has been shown in Table 1. Considering the average rate of change for inundation in different wetland classes 50–100% (∼28.9%) and 25–50% wetlands (∼23.3%), in north western hemisphere, have shown high contribution to the surface waters but with a non-significant R2 value. The wetland complex has shown a steep increase after 1999 as illustrated in Figure 3 while rivers, reservoirs, swamps, and coastal wetlands have shown exponential gains in inundated areas. Lakes, pan brackish wetlands, 50–100% and 25–50% wetlands presented minor increases in surface inundation. Whereas, freshwater floodplains and bogs fens and mires had shown no significant changes. The only class that has shown decreasing surface inundation trend is intermittent wetland.
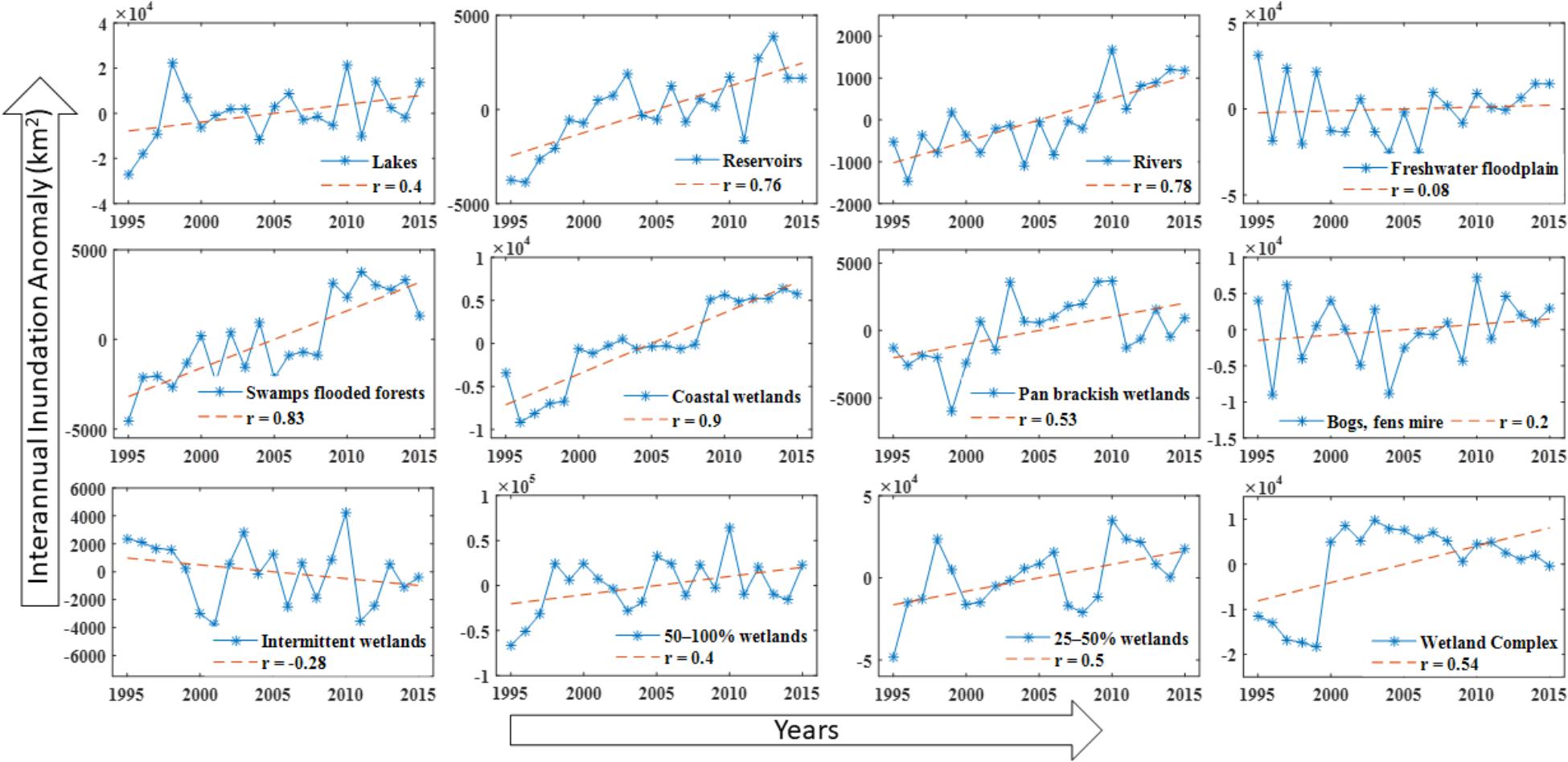
Figure 3. Temporal interannual trends in global surface inundation variability from 1995 to 2015 illustrating significant increments in global reservoirs, rivers, swamps flooded forests, and coastal wetlands.
Spatial Distribution of Global Inundation Trend
The spatial variability observed during 21 years (1995–2015) of inundation fraction has been mapped in Figure 4. The highest variability has been observed in the small regions of south-west of China, Central Asia, Middle East, and North Africa. The coastal areas have shown a distinct interannual variability in most regions of the globe while moderately high variability is presented over Central Asia, Eastern Europe, parts of Canada and North America, Eastern Australia, Saheli region, and south-western Indian region.
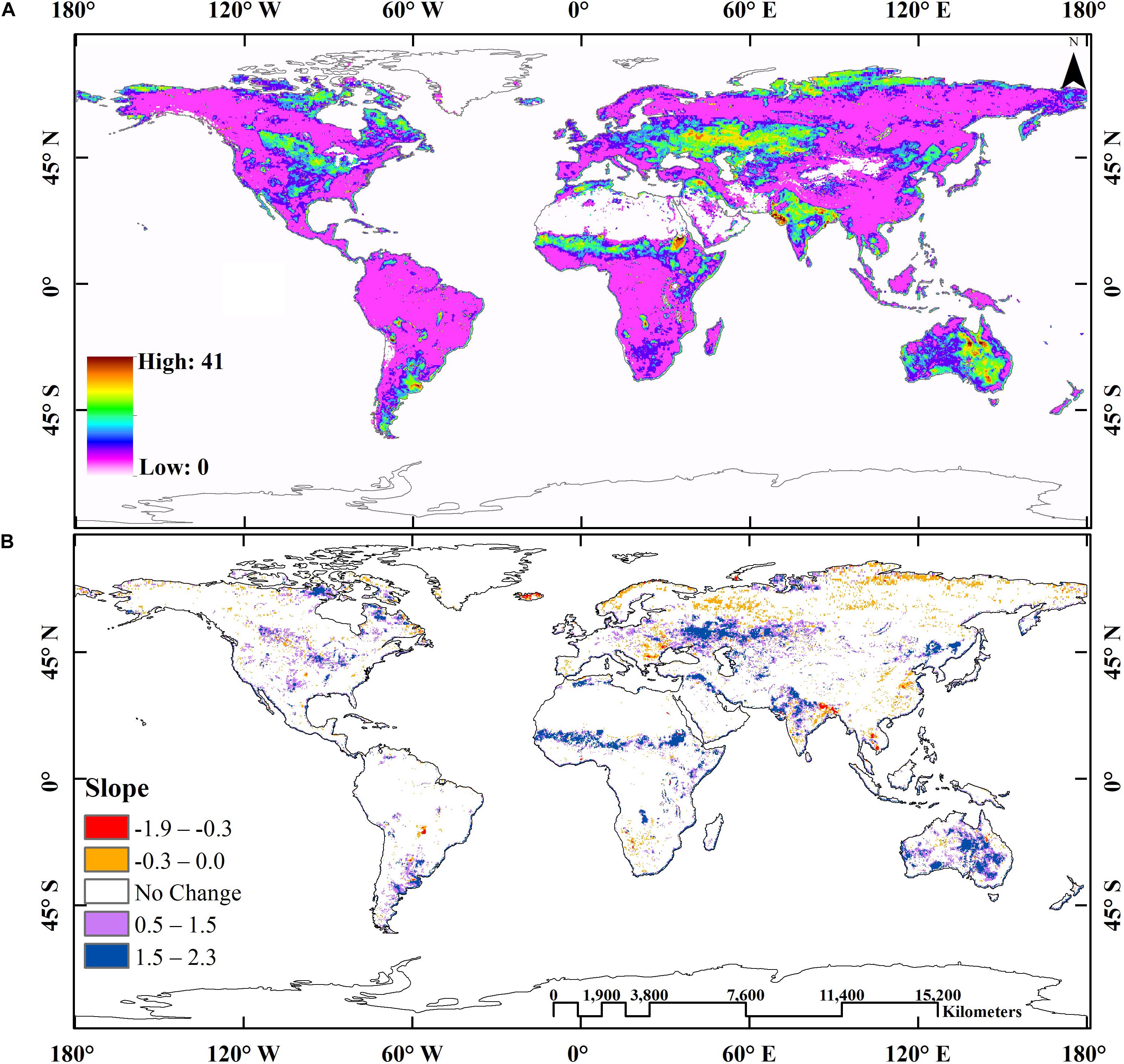
Figure 4. (A) Global distribution of interannual variability during 1995–2015 (fractional percentage) from SWAMPS dataset indicating the extent of deviation (standard deviation of maximum inundation fraction) observed in global inundation patterns. (B) Interannual maximum surface inundation anomaly trend (with the slope values presenting fractional percentage/year) during 1995–2015 from SWAMPS dataset.
From the spatial trend analysis (Figure 4B), the maximum increasing trend (p < 0.05) has been presented by 25–50% wetlands (24.7%), swamps (18%), and freshwater floodplain (18%) while the maximum decreasing trend has also been observed in 25–50% wetlands (34%) and wetland complex (20.5%). The most significant decrease (p < 0.05) in the interannual anomaly inundation values has been revealed by 25–50% wetlands (35%) and wetland complex (21%) conversely 25–50% wetland also contributed to the highest increase (p < 0.05) in inundation anomaly values along with swamps flooded forests and freshwater floodplain.
Impacts of Niño 3.4 SSTA on Inundation Variability
The global inundation variability has shown positive correlations with Niño 3.4 SSTA values for La Niña months; the correlation coefficients computed for the monthly anomalies of global surface inundation extent are ∼0.19 (p < 0.01), ∼0.36 (p < 0.01), and ∼0.40 (p < 0.01) for 0-, 1- and 2-months led by Niño 3.4 SSTA, respectively.
The correlation coefficient of Niño 3.4 SSTA values with ENSO Neutral phase (82 months) and El Niño (87 months) and La – Niña (83 months) for the study period has been presented in Table 2. The maximum value for surface inundation observed has been contributed by the year of 2010 which has also been the year of strong El Niño episode through various ENSO indexes. Based on our results, 9 out of 12 GLWD classes revealed significant positive correlation with La Niña episodes while 2 classes revealed significantly negative correlation with El Niño months. The highest correlation coefficient found for swamps flooded forest and wetland complex was for no lag and 2-month lag with Niño 3.4 SSTA during El Niño episodes, respectively.
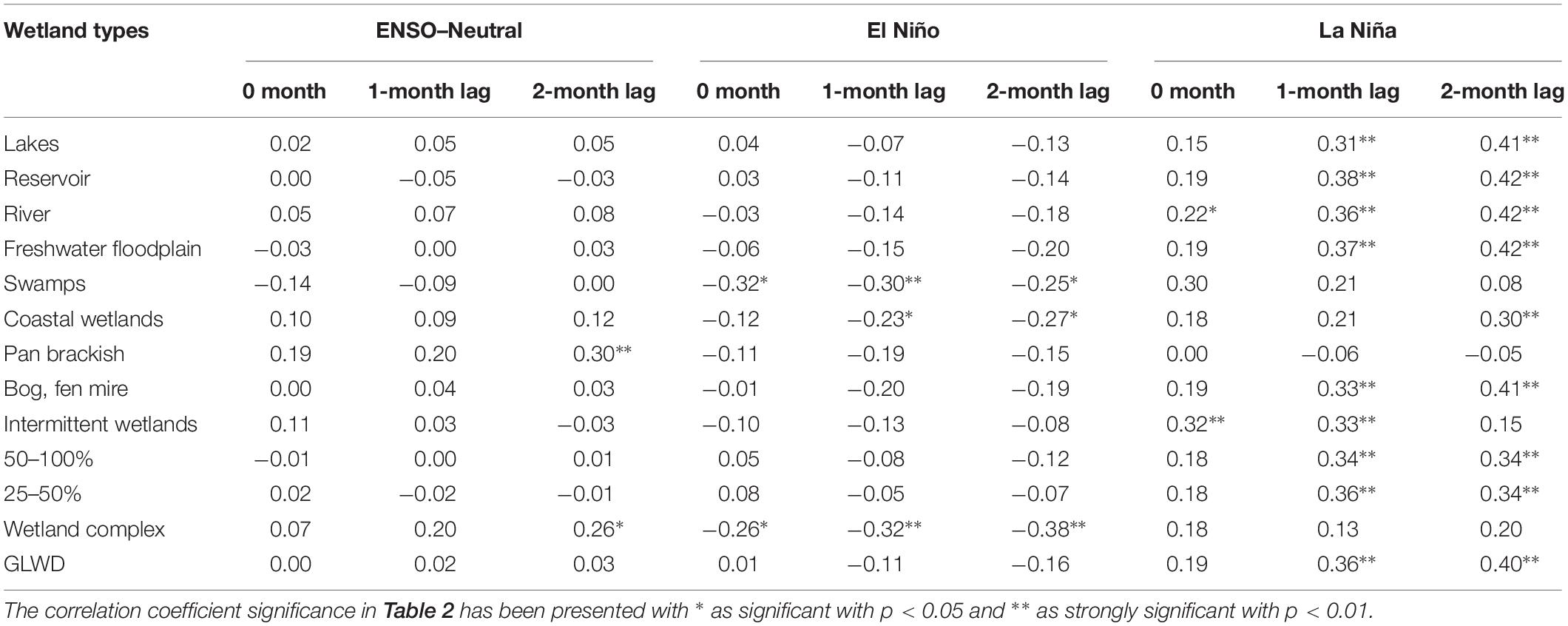
Table 2. Correlation of Global lakes and wetland classes with monthly Niño3.4 SSTA along with El Niño and La Niña months from 1995 to 2015.
The GLWD classes that have shown significant positive correlation coefficients during La Niña months are lakes, reservoirs, rivers, freshwater floodplain among open water bodies and coastal, bog fens mire, 50–100% and 25–50% wetland types with 2-month difference led by Niño 3.4 SSTA. While, intermittent wetland has presented significantly positive correlation with no lag and 1-month lag period during La Niña episodes. Whereas, the pan brackish wetland was the only type to have shown significant positive correlation during ENSO Neutral months with a 2-lag period of Niño 3.4 SSTA conversely showing no significant correlation with El Niño or La Niña episodes.
Land Use Effects on Global Inundation Trends
The land area that underwent conversion from anthropogenic land use to lakes, rivers, reservoirs, and wetlands and vice versa make ∼10,000 km2 of the global total in which round way conversion between wetlands and NV has majorly contributed. Conversion of wetland areas to BL and shrubland/grasslands to wetlands also made substantial contribution.
Figure 5 presents the spatial illustration of land use change from 1995 to 2015. Based upon 21 years of change detection analysis 0.38% of wetland area from LULC has undergone land conversion globally, accounting for 0.14% gain and 0.24% loss observed in the wetland areas. The percentage conversion of wetland area of 0.24% to other land form, in order of maximum to minimum, has been contribution by NV (48.8%), BL (5.4%), shrub/grass lands (4.1%), CL (3.2%), and UL (0.6%); while the gain of 0.14% of global wetland area has been contributed by NV (28.5%), shrub/grass land (4.9%), CL (3.5%), and BL (1%).
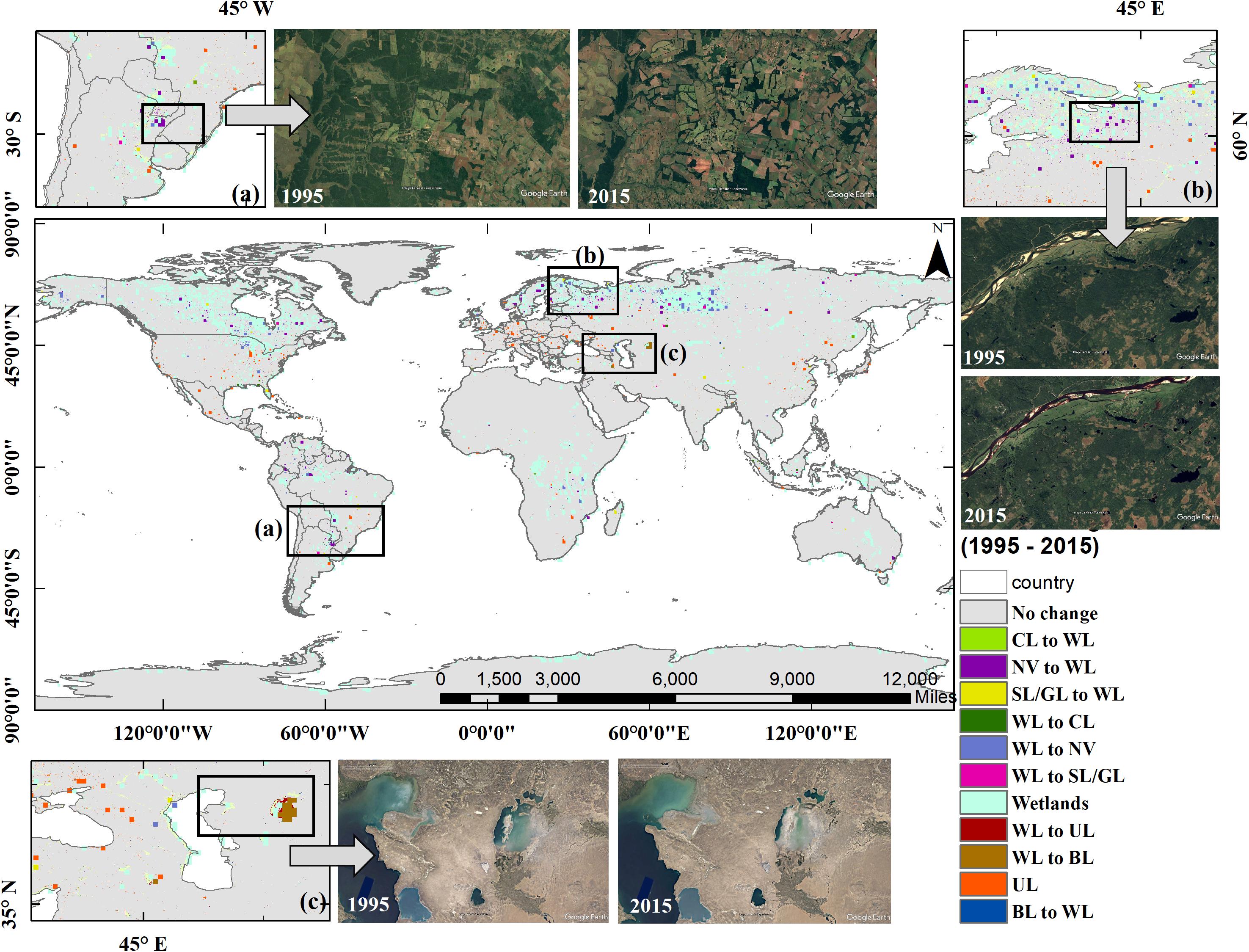
Figure 5. Land use land cover change map illustrating the land transformation between 1995 and 2015; CL to WL (Cropland to Wetland), NV to WL (Natural Vegetation to Wetland), SL/GL to WL (Shrubland/Grassland to Wetland), WL to CL (Wetland to Cropland), WL to NV (Wetland to Natural Vegetation), WL to SL/GL (Wetland to Shrubland/Grassland), WL to UL (Wetland to Urban land), WL to BL (Wetland to Barren land), BL to WL (Barren land to Wetland), Wetlands, and UL (Urban Land). Where the conversion of natural vegetation to wetland has been shown in subplot (a) in the catchment area of the Paraná River located in the south-Central America, and subplot (b) of the catchment region of the Northern Dvina River in the Arkhangelsk Oblast region located in the north of European Russia; while the conversion of wetland area to barren land has been shown in subplot (c) shows the some part of Caspian Sea along with the areal coverage of Aral Sea of Central Asia besides each of the three subplots regions are shown through Google earth images from 1995 and 2015 to highlight the land use change as observed through the satellite imagery. Different wetland classes responded differently to the land use change, depending essentially on the deeper ecological and atmospheric connection between the two. Seven of the classes have shown high correlations (positive/negative) with the land use change drivers within the 10 km radius of each class. The significant correlation coefficients have been presented by rivers and reservoirs from open water bodies and swamps, coastal, 50–100% and wetland complex form the wetland classes. The summary of correlation coefficients for all the land use drivers with different wetland types has been presented in Correlation matrix; Table 3.
Reservoirs, coastal wetlands, and wetland complex has shown strongly significant negative correlation with shrub/grass land (r < –0.75) and swamps and coastal with NV (r > 0.75); while swamps and coastal wetlands has shown high positive correlation coefficients with UL and swamps with CL (r > 0.75), respectively.
Discussion
The surface inundation extent for the global lakes, reservoirs, rivers and wetlands has been calculated along with the interannual variability observed in this study. Our study results have revealed interannual variability in global surface inundation over time. The maximum value has been observed during 2010 and the minimum during 1995 and 1996, followed by an overall increase in interannual inundation trend from 1995 to 2015. Conspicuously, the inundation extent for the year 1998 contributes the highest in the initial 10 years of the study period (1995–2004). The 1998 inundation peak shows good agreement with previous researches while a decreasing trend in inundation extent was found in the subsequent years till 2001 (Prigent et al., 2007).
Our results, based on the global areal coverage of lakes, reservoirs, rivers and wetlands, provides an average global inundation extent of ∼2.9 million km2. Our results agrees well with the recent documented areal observations of global wetland inundation extent of 1.5 to 15 million km2, from minimum to maximum coverage, respectively (Prigent et al., 2001; Zhu and Gong, 2014; Hu et al., 2017; Poulter et al., 2017; Davidson et al., 2018). Our study presented an increment of 2.77 to 2.9 million km2 from 1995 to 2015, respectively, increasing at a rate of ∼0.24% (∼7029.6 km2) per year. Whereas, a previous study reported a 2.72 to 2.77 million km2 increase from 1980 to 2015 (Pekel et al., 2016).
The increasing rate of surface inundation revealed by 11 out of the 12 water bodies can be attributed to natural or anthropogenic drivers few of which includes land conversion, temperature and precipitation variability, ENSO and sea level rise (SLR) (Prigent et al., 2007; Klemas, 2011; Trenberth, 2011; Junk et al., 2013; Tapia-Armijos et al., 2017). Intermittent wetland is the only class to have shown a non-significant decreasing trend while open waterbodies and wetland types presented a substantial increase in the surface inundation extent during 1995–2015.
Swamps and coastal wetlands, with geographical dominance around tropics and subtropics, have shown a substantial increase in the surface inundation. The inundation around tropics can be linked to the increasing precipitation rates around tropics and amazon due to multidecadal warming (Wang et al., 2018). The coastal wetlands have shown the most significant increasing trend among all the global wetlands which can be ascribed to factors like thermal expansion of sea water, decaying of glaciers, ice caps and ice sheets (IPCC, 2007; Cazenave and Llovel, 2010; Church and White, 2011). Previously, a 1.7 mm/year rise in Global Mean Sea Level (GMSL) reported between 1900 and 2009 with an even higher rate projected during the end of 20th century due to thermal expansion and melting of glaciers and ice sheets agreeing with the increase in coastal inundation (Mimura, 2013). The 50–100% and 25–50% wetland, located in the north-western hemisphere, contributed higher percentages to global inundation but with non-significant increasing trends. North America which accounts for half of the permanently inundated surface has gained 17,000 km2 while Asia has been reported to an increase of 71,000 km2 of permanent inundation in the 30 years between 1984 and 2015 (Pekel et al., 2016). Wetland complex, mostly concentrated around South and East Asia mainly over the Tibetan Plateau, has presented a non-significant increasing trend with an increase in lake area reported due to changing weather and climatic phenomenon (Yang and Lu, 2014; Jiang et al., 2017). The middle and higher latitudes along with coastal wetlands have contributed the most to permanently inundated spaces also much of this average increase has been observed after 1997–1998.
The average increase in surface inundation extent can be attributed to the intensified global precipitation (Huntington, 2006) resulting from atmospheric teleconnections linked to ENSO cycle as an eventuality to Pacific warming (Dai and Wigley, 2000; Cai et al., 2014). The ENSO induced variability in precipitation patterns can inflict flooding or drought, in turn affecting the global wetland extent through the wet and dry hydroperiods (Prigent et al., 2007). Based on our results, swamp flooded forest geographically spread around tropics showed negative correlation with 0- and 1-month lag period with El Niño months. This result agrees with earlier study demonstrating reduced precipitation in amazon basin during El Niño periods (Latif and Keenlyside, 2009). Wetland complex, another low latitude class, revealed significantly negative 2-month lag correlation with El Niño. The drying of swamps and wetland complex as a result to El Niño periods has been reported earlier for different lower latitudinal regions including amazon basin, Indian, and African continent (Mercier et al., 2002; Kumar et al., 2006; Trenberth, 2011). Despite of the reduced precipitation incurred due to the coupling effect of CO2 and water vapors the atmospheric concentrations of the former translates in wetland methane emissions variability eventually contributing as the feedback mechanism to warming climate (Mercier et al., 2002; Kumar et al., 2006; Trenberth, 2011; Paudel et al., 2016). This s in increased vapor pressure and intensified precipitation rates (Pendergrass and Hartmann, 2014) explaining increased inundation around tropics and sub tropics.
The 8 out of 9 GLWD classes that has shown higher correlations with La Niña episodes for 2- month lag period while only intermittent wetland has shown higher correlation value with 0- and 1-month lag period. The 7 classes (lakes, rivers, reservoirs, freshwater floodplains, bogs fens mire, 50–100%, and 25–50% wetland) revealing positive correlation not entirely but are mainly concentrated around mid to high latitudes. The trade winds administering the atmospheric vapor pressure distribution; governed by SST, has influenced global precipitation patterns leading to wetting of higher latitudes (Trenberth, 2011). The global inundation extent for GLWD has shown significant positive 1- and 2-month lagged correlation with Niño 3.4 SSTA for La Niña months. Excessive ENSO variations are reported to cause intensified fluctuations between the pronounced wet and dry periods whereby the dry periods are expected to a twofold while wet periods to a threefold increased occurrences (Yoon et al., 2015). With the increasing SST, the evapotranspiration increases resulting in ENSO induced precipitation of +0.04 mm/day (Adler et al., 2017). A linear relationship between global precipitation and surface temperature has been established (Allen and Ingram, 2002) while others reported a 3.6% increase in global precipitation for every 1 degree increase in SST (Sugi and Yoshimura, 2004).
Our study results have shown an overall increase in inundated surface extent while the LULC change have shown a reduction (0.24% loss) in wetland area between the initial (1995) and final year (2015) Based on our study results of land use change, the land conversion has been most significant in between NV and wetlands that mostly happened in Eurasian and North America regions; while the conversion of wetland to CL has been dominant over American continent. The conversion of wetlands to BL or shrub/grasslands has been dominant in Europe and Asia where the drying of Aral Sea due to irrigational practices remained most noticeable (Edlinger et al., 2012; Micklin, 2016).
The regions undergoing urban sprawl and land use change also attributes to variability in localized vertical wind motion caused by “urban surface expansion induced warming.” This localized process could intensify or reduce regional precipitation in an around areas with exponential urban growth which can be linked to the increased inundation surfaces around urban centers in most of the wetland classes (Zhao and Wu, 2018). An upsurge in water storage areas, for human and economic use, in an around high population zones has been recorded which also explains the inundation increase around urban areas (Sarma et al., 2001; Tian et al., 2015).
Our study results have shown significant positive correlations with shrub/grass lands which can be attributed to the water holding capacity of different wetlands. As reported, playas surrounded by grass and pasture lands responds positively by having longer water holding capacity as compared with those surrounded by CL; making them more susceptible to anthropogenic disturbances rather than climatic variations (Collins et al., 2014). The wetlands connected with aquifers experiences reduced inundation due to either drying of aquifers or excessive withdrawals for agriculture purposes (Smith et al., 2011). Our results presented grasslands and shrublands conversion to other land has shown agreement with the fragmentation and loss as the surface inundation increased over time (Yu et al., 2017) which could also be associated with overgrazing (Sekercioglu et al., 2011; Huang et al., 2012). Although the restoration measures have shown better yields in grassland areas yet the damage endured by soil ecosystem has inhibited the soil recovery inflicted due to overgrazing and excessive precipitation (Huang et al., 2018). Meanwhile, a reduction in grazing practices over European region resulted in abandonment of fens, seems to have contributed to the shifting of wetlands to NV which agrees to our land conversion results (Middleton et al., 2006).
Coastal wetlands have shown positive correlation with shrubland/grassland which may be attributed to the increased tourism facilities and structures (Camacho-Valdez et al., 2014; Ivajnsic and Kaligaric, 2014). Furthermore, salt water intrusion interlinked with SLR can also be considered a reason for declining NV around the coastal wetlands (Ivajnsic and Kaligaric, 2014; Osland et al., 2018) and for increase in BL around the coastal areas.
Different types of wetlands may respond differently to the stressors like climate change or land use practices. Interannual variability caused by natural oscillations has effects on all types of ecosystems. El Niño events deteriorate mangroves health and also cause damages to alpine forbs and boreal forests (Holmgren et al., 2001). Despite the positive lagged correlation found between surface inundation variations in most of the classes during La Niña months with Niño 3.4 SSTA still it is difficult to state the exact effect of ENSO on different waterbodies and wetlands. Since the distribution of majority of the GLWD classes are not consistent geographically and are studied against the variability of Niño 3.4 SSTA which has a coupling effect on different regions hence this study has only revealed one side of the picture. For better understanding of scientific and physical phenomenon, deeper analytical study is required considering other oceanic and atmospheric variables like Indian Ocean Dipole, temperature, precipitation, evapotranspiration, atmospheric pressure, and wind direction.
Conclusion
The global lakes and wetlands are intricately connected hydrologic system making it difficult to analyze the combined impact of natural ENSO and anthropogenic drivers on these vital ecosystems. ENSO is a complex internally driven oscillation mechanism which can undergo variations by the interruptions caused by natural forcing hence making it difficult to analyze the impact of this mechanism on the global inundation changes. Likewise, the human settlements pose threats to the waterbodies impairing the sources’ quality and quantity hence the study of impacts of natural and human stressors in altering these sensitive yet indispensable ecosystems are crucial.
The spatial and temporal changes have been observed in order to study extent and distribution along with the effects of natural oscillations and anthropogenic activities on the global lakes, rivers, reservoirs and wetlands. The study revealed a per year increase of 7029.6 km2 with an average global inundation extent of 2.9 km2 from 1995 to 2015. All the classes of GLWD has contributed to this global surface inundation increase except for intermittent wetlands. Through this study we have been able relate the coupling episodes of El Niño and La Niña with the global inundation variations. The study highlights that the La Niña months seems to have stronger link with the inundation trends observed in most of the global lakes and wetland classes except for pan brackish, swamps, intermittent wetlands and wetland complex while the later three have shown stronger connection to El Niño periods. Our study also identifies CL, NV, BL, UL, and shrub/grass land as the most significant land use types and land use changes that have shown varying relationship to global lakes and wetlands surface inundation. This study of land use changes can prove to be helpful in implementing of robust land conservation efforts and monitoring for achieving sustainable water consumption.
The lack of connection related to the complex dynamics of natural oscillations and climatic factors can limit our understanding of global changes and its impacts on global lakes and wetlands. For the future research, the aspect of establishing the link between natural oscillations and other climate variables for an improved understanding of the natural processes involved in contributing to the hydrological variations can be considered.
Data Availability Statement
The datasets analyzed in this manuscript are not publicly available. Request to access the datasets should be directed toc2FuYUB0ZWEuYWMuY24=.
Author Contributions
SI designed the study, performed the data analysis, and wrote the paper. XX, GJ, and AZ designed the study, acquired the data, and discussed the results. All authors contributed to the results discussion, manuscript revision, and approval of publish.
Funding
This study was funded by the Strategic Priority Research Program of the Chinese Academy of Sciences, CAS Earth (XDA19030401) and Pioneer Hundred Talents Program of Chinese Academy of Sciences.
Conflict of Interest
The authors declare that the research was conducted in the absence of any commercial or financial relationships that could be construed as a potential conflict of interest.
Acknowledgments
We thank Dr. Kyle C. McDonald and Ms. Kat Jensen for making SWAMPS data available to us for this research. SI would also like to acknowledge the Chinese Academy of Sciences (CAS) and The World Academy of Sciences (TWAS) for awarding the CAS-TWAS President’s Fellowship for this research work.
References
Adler, R. F., Gu, G. J., Sapiano, M., Wang, J. J., and Huffman, G. J. (2017). Global precipitation: means, variations and trends during the satellite era (1979-2014). Surv. Geophys. 38, 679–699. doi: 10.1007/s10712-017-9416-4
Agarwal, R., and Garg, J. K. (2009). Methane emission modelling from wetlands and waterlogged areas using MODIS data. Curr. Sci. 96, 36–40.
Aires, F., Prigent, C., Fluet-Chouinard, E., Yamazaki, D., Papa, F., and Lehner, B. (2018). Comparison of visible and multi-satellite global inundation datasets at high-spatial resolution. Remote Sens. Environ. 216, 427–441. doi: 10.1016/j.rse.2018.06.015
Allen, M. R., and Ingram, W. J. (2002). Constraints on future changes in climate and the hydrologic cycle. Nature 419, 224–232.
Birkett, C., Chanton, J., Dunne, T., Estes, J., Finlayson, M., Fresco, L., et al. (1998). Global Wetland Distribution and Functional Characterization: Trace Gases and the Hydrologic Cycle. Stockholm: Royal Swedish Academy of Sciences.
Burkett, V., and Kusler, J. (2000). Climate change: potential impacts and interactions in wetlands of the United States. J. Am. Water Resour. Assoc. 36, 313–320.
Cai, W. J., Borlace, S., Lengaigne, M., van Rensch, P., Collins, M., Vecchi, G., et al. (2014). Increasing frequency of extreme El Nino events due to greenhouse warming. Nat. Clim. Change 4, 111–116. doi: 10.1038/nclimate2100
Camacho-Valdez, V., Ruiz-Luna, A., Ghermandi, A., Berlanga-Robles, C. A., and Nunes, P. A. L. D. (2014). Effects of land use changes on the ecosystem service values of coastal wetlands. Environ. Manag. 54, 852–864. doi: 10.1007/s00267-014-0332-9
Chen, J., and Kumar, P. (2002). Role of terrestrial hydrologic memory in modulating enso impacts in north america. J. Clim. 15, 3569–3585. doi: 10.1175/1520-0442(2003)015<3569:rothmi>2.0.co;2
Church, J. A., and White, N. J. (2011). Sea-level rise from the late 19th to the early 21st century. Surv. Geophys. 32, 585–602. doi: 10.1007/978-94-007-2063-3_17
Collins, S. D., Heintzman, L. J., Starr, S. M., Wright, C. K., Henebry, G. M., and McIntyre, N. E. (2014). Hydrological dynamics of temporary wetlands in the southern great plains as a function of surrounding land use. J. Arid Environ. 109, 6–14. doi: 10.1016/j.jaridenv.2014.05.006
Dai, A., and Wigley, T. M. L. (2000). Global patterns of ENSO-induced precipitation. Geophys. Res. Lett. 27, 1283–1286. doi: 10.1029/1999gl011140
Davidson, N. C., Fluet-Chouinard, E., and Finlayson, C. M. (2018). Global extent and distribution of wetlands: trends and issues. Mar. Freshw. Res. 69, 620–627.
Dlugokencky, E. J., Nisbet, E. G., Fisher, R., and Lowry, D. (2011). Global atmospheric methane: budget, changes and dangers. Philos. Trans. R. Soc. Math. Phys. Eng. Sci. 369, 2058–2072. doi: 10.1098/rsta.2010.0341
Edlinger, J., Conrad, C., Lamers, J. P. A., Khasankhanova, G., and Koellner, T. (2012). Reconstructing the spatio-temporal development of irrigation systems in uzbekistan using landsat time series. Remote Sens. 4, 3972–3994. doi: 10.3390/rs4123972
Erwin, K. L. (2009). Wetlands and global climate change: the role of wetland restoration in a changing world. Wetl. Ecol. Manag. 17, 71–84. doi: 10.1007/s11273-008-9119-1
Guler, M., and Dural, A. B. (2007). Climate change and its effects. J. Environ. Protect. Ecol. 8, 410–417.
Hodson, E. L., Poulter, B., Zimmermann, N. E., Prigent, C., and Kaplan, J. O. (2011). The El nino-southern oscillation and wetland methane interannual variability. Geophys. Res. Lett. 38:L08810.
Holmgren, M., Scheffer, M., Ezcurra, E., Gutierrez, J. R., and Mohren, G. M. J. (2001). El nino effects on the dynamics of terrestrial ecosystems. Trends Ecol. Evol. 16, 89–94. doi: 10.1016/s0169-5347(00)02052-8
Hu, S. J., Niu, Z. G., Chen, Y. F., Li, L. F., and Zhang, H. Y. (2017). Global wetlands: potential distribution, wetland loss, and status. Sci. Total Environ. 586, 319–327. doi: 10.1016/j.scitotenv.2017.02.001
Huang, F., Wang, P., and Zhang, J. J. (2012). Grasslands changes in the Northern Songnen Plain, China during 1954-2000. Environ. Monit. Assess. 184, 2161–2175. doi: 10.1007/s10661-011-2107-6
Huang, L., Shao, Q. Q., Liu, J. Y., and Lu, Q. S. (2018). Improving ecological conservation and restoration through payment for ecosystem services in Northeastern Tibetan Plateau, China. Ecosyst. Serv. 31, 181–193. doi: 10.1016/j.ecoser.2018.04.005
Huntington, T. G. (2006). Evidence for intensification of the global water cycle: review and synthesis. J. Hydrol. 319, 83–95. doi: 10.1016/j.jhydrol.2005.07.003
Ishizawa, M., Chan, D., Worthy, D., Chan, E., Vogel, F., and Maksyutov, S. (2019). Analysis of atmospheric CH4 in canadian arctic and estimation of the regional CH4 fluxes. Atmos. Chem. Phys. 19, 4637–4658. doi: 10.5194/acp-19-4637-2019
Ivajnsic, D., and Kaligaric, M. (2014). How to preserve coastal wetlands, threatened by climate change-driven rises in sea level. Environ. Manag. 54, 671–684. doi: 10.1007/s00267-014-0244-8
Jia, X. J., and Ge, J. W. (2017). Interdecadal changes in the relationship between ENSO, EAWM, and the wintertime precipitation over china at the end of the twentieth century. J. Clim. 30, 1923–1937. doi: 10.1038/srep26888
Jiang, L. G., Nielsen, K., Andersen, O. B., and Bauer-Gottwein, P. (2017). Monitoring recent lake level variations on the tibetan plateau using CryoSat-2 SARIn mode data. J. Hydrol. 544, 109–124. doi: 10.1016/j.jhydrol.2016.11.024
Jones, K., Lanthier, Y., van der Voet, P., van Valkengoed, E., Taylor, D., and Fernandez-Prieto, D. (2009). Monitoring and assessment of wetlands using earth observation: the globwetland project. J. Environ. Manage. 90, 2154–2169. doi: 10.1016/j.jenvman.2007.07.037
Junk, W. J., An, S. Q., Finlayson, C. M., Gopal, B., Kvet, J., Mitchell, S. A., et al. (2013). Current state of knowledge regarding the world’s wetlands and their future under global climate change: a synthesis. Aqua. Sci. 75, 151–167. doi: 10.1007/s00027-012-0278-z
Kayranli, B., Scholz, M., Mustafa, A., and Hedmark, A. (2010). Carbon storage and fluxes within freshwater wetlands: a critical review. Wetlands 30, 111–124. doi: 10.1007/s13157-009-0003-4
Klemas, V. (2011). Remote sensing of wetlands: case studies comparing practical techniques. J. Coast. Res. 27, 418–427.
Kumar, K. K., Rajagopalan, B., Hoerling, M., Bates, G., and Cane, M. (2006). Unraveling the mystery of Indian monsoon failure during El Nino. Science 314, 115–119. doi: 10.1126/science.1131152
Latif, M., and Keenlyside, N. S. (2009). El Nino/Southern oscillation response to global warming. Proc. Natl. Acad. Sci. U.S.A. 106, 20578–20583. doi: 10.1073/pnas.0710860105
Lee, T. M., and Yeh, H. C. (2009). Applying remote sensing techniques to monitor shifting wetland vegetation: a case study of danshui river estuary mangrove communities, Taiwan. Ecol. Eng. 35, 487–496. doi: 10.1016/j.ecoleng.2008.01.007
Lehner, B., and Doll, P. (2004). Development and validation of a global database of lakes, reservoirs and wetlands. J. Hydrol. 296, 1–22. doi: 10.1016/j.jhydrol.2004.03.028
Li, H. Y., Mao, D. H., Li, X. Y., Wang, Z. M., and Wang, C. Z. (2019). Monitoring 40-year lake area changes of the qaidam basin, tibetan plateau, using landsat time series. Remote Sens. 11:343. doi: 10.3390/rs11030343
MacKay, H., Finlayson, C. M., Fernandez-Prieto, D., Davidson, N., Pritchard, D., and Rebelo, L. M. (2009). The role of Earth Observation (EO) technologies in supporting implementation of the ramsar convention on wetlands. J. Environ. Manage. 90, 2234–2242. doi: 10.1016/j.jenvman.2008.01.019
Meng, L., Roulet, N., Zhuang, Q. L., Christensen, T. R., and Frolking, S. (2016). Focus on the impact of climate change on wetland ecosystems and carbon dynamics. Environ. Res. Lett. 11:100201. doi: 10.1088/1748-9326/11/10/100201
Mercier, F., Cazenave, A., and Maheu, C. (2002). Interannual lake level fluctuations (1993–1999) in Africa from topex/poseidon: connections with ocean–atmosphere interactions over the Indian Ocean. Glob. Planet. Change 32, 141–163. doi: 10.1016/s0921-8181(01)00139-4
Middleton, B. A., Holsten, B., and van Diggelen, R. (2006). Biodiversity management of fens and fen meadows by grazing, cutting and burning. Appl. Veg. Sci. 9, 307–316. doi: 10.1111/j.1654-109x.2006.tb00680.x
Mimura, N. (2013). Sea-level rise caused by climate change and its implications for society. Proc. Japan Acad. Ser. B Phys. Biol. Sci. 89, 281–301. doi: 10.2183/pjab.89.281
Mitsch, W. J., Bernal, B., Nahlik, A. M., Mander, U., Zhang, L., Anderson, C. J., et al. (2013). Wetlands, carbon, and climate change. Landsc. Ecol. 28, 583–597.
National Research Council, (1995). Wetlands: Characteristics and Boundaries. Washington, DC: The National Academies Press.
Newbold, T., Hudson, L. N., Hill, S. L. L., Contu, S., Lysenko, I., Senior, R. A., et al. (2015). Global effects of land use on local terrestrial biodiversity. Nature 520, 45–50. doi: 10.1038/nature14324
NOAA/NWS/CPC (2005). “Nino 3.4,” in NOAA/NWS/CPC Indices and Forecasts, ed. NOAA National Weather Service, (Springs, MD: NOAA/NWS/CPC).
Osland, M. J., Feher, L. C., Lopez-Portillo, J., Day, R. H., Suman, D. O., Menendez, J. M. G., et al. (2018). Mangrove forests in a rapidly changing world: global change impacts and conservation opportunities along the Gulf of Mexico coast. Estuar. Coast. Shelf Sci. 214, 120–140. doi: 10.1016/j.ecss.2018.09.006
Ozesmi, S. L., and Bauer, M. E. (2002). Satellite remote sensing of wetlands. Wetl. Ecol. Manag. 10, 381–402.
Pandey, S., Houweling, S., Krol, M., Aben, I., Monteil, G., Nechita-Banda, N., et al. (2017). Enhanced methane emissions from tropical wetlands during the 2011 La Nina. Sci. Rep. 7:45759. doi: 10.1038/srep45759
Paudel, R., Mahowald, N. M., Hess, P. G. M., Meng, L., and Riley, W. J. (2016). Attribution of changes in global wetland methane emissions from pre-industrial to present using CLM4.5-BGC. Environ. Res. Lett. 11.
Pekel, J. F., Cottam, A., Gorelick, N., and Belward, A. S. (2016). High-resolution mapping of global surface water and its long-term changes. Nature 540, 418–422. doi: 10.1038/nature20584
Pendergrass, A. G., and Hartmann, D. L. (2014). Changes in the distribution of rain frequency and intensity in response to global warming. J. Clim. 27, 8372–8383. doi: 10.1175/jcli-d-14-00183.1
Pham-Duc, B., Prigent, C., Aires, F., and Papa, F. (2017). Comparisons of global terrestrial surface water datasets over 15 years. J. Hydrometeorol. 18, 993–1007. doi: 10.1175/jhm-d-16-0206.1
Poulter, B., Bousquet, P., Canadell, J. G., Ciais, P., Peregon, A., Saunois, M., et al. (2017). Global wetland contribution to 2000-2012 atmospheric methane growth rate dynamics. Environ. Res. Lett. 12:094013. doi: 10.1088/1748-9326/aa8391
Powell, M., Hodgins, G., Danaher, T., Ling, J., Hughes, M., and Wen, L. (2019). Mapping wetland types in semiarid floodplains: a statistical learning approach. Remote Sens. 11:609. doi: 10.3390/rs11060609
Prigent, C., Matthews, E., Aires, F., and Rossow, W. B. (2001). Remote sensing of global wetland dynamics with multiple satellite data sets. Geophys. Res. Lett. 28, 4631–4634. doi: 10.1029/2001gl013263
Prigent, C., Papa, F., Aires, F., Rossow, W. B., and Matthews, E. (2007). Global inundation dynamics inferred from multiple satellite observations, 1993-2000. J. Geophys. Res. Atmos. 112:12107.
Raisin, G., Bartley, J., and Croome, R. (1999). Groundwater influence on the water balance and nutrient budget of a small natural wetland in Northeastern Victoria, Australia. Ecol. Eng. 12, 133–147. doi: 10.1016/s0925-8574(98)00059-7
Ramsar Convention on Wetlands (2013). The Ramsar Convention Rue Mauverney 28, CH-1196. Gland: Ramsar Convention on Wetlands.
Ramsar Convention on Wetlands, (2015). Draft Resolution: Call to Action to Ensure and Protect The Water Requirements Of Wetlands for the Present And The Future., 48th Meeting of the Standing Committee. Gland: Convention On Wetlands.
Sarma, V. V., Krishna, G. M., Mailini, H. B., and Rao, K. N. (2001). Land Use/land cover change detection through remote sensing and its climatic implications in the godavari delta region. J. Indian Soc. Remote Sens. 29, 86–91.
Saunois, M., Jackson, R. B., Bousquet, P., Poulter, B., and Canadell, J. G. (2016). The growing role of methane in anthropogenic climate change. Environ. Res. Lett. 11:120207. doi: 10.1088/1748-9326/11/12/120207
Schroeder, R., McDonald, K. C., Chapman, B. D., Jensen, K., Podest, E., Tessler, Z. D., et al. (2015). Development and evaluation of a multi-year fractional surface water data set derived from active/passive microwave remote sensing data. Remote Sens. 7, 16688–16732. doi: 10.3390/rs71215843
Sekercioglu, C. H., Anderson, S., Akcay, E., Bilgin, R., Can, O. E., Semiz, G., et al. (2011). Turkey’s globally important biodiversity in crisis. Biol. Conserv. 144, 2752–2769. doi: 10.1016/j.biocon.2011.06.025
Smith, L. M., Haukos, D. A., McMurry, S. T., LaGrange, T., and Willis, D. (2011). Ecosystem services provided by playas in the high plains: potential influences of USDA conservation programs. Ecol. Appl. 21, S82–S92.
Sugi, M., and Yoshimura, J. (2004). A mechanism of tropical precipitation change due to CO2 increase. J. Clim. 17, 238–243. doi: 10.1175/1520-0442(2004)017<0238:amotpc>2.0.co;2
Tapia-Armijos, M. F., Homeier, J., and Munt, D. D. (2017). Spatio-temporal analysis of the human footprint in South Ecuador: influence of human pressure on ecosystems and effectiveness of protected areas. Appl. Geogra. 78, 22–32. doi: 10.1016/j.apgeog.2016.10.007
Tian, B., Zhou, Y. X., Thom, R. M., Diefenderfer, H. L., and Yuan, Q. (2015). Detecting wetland changes in shanghai, China using FORMOSAT and Landsat TM imagery. J. Hydrol. 529, 1–10. doi: 10.1016/j.jhydrol.2015.07.007
Trenberth, K. E. (2011). Changes in precipitation with climate change. Clim. Res. 47, 123–138. doi: 10.3354/cr00953
Trenberth, K. E., and Caron, J. M. (2000). The southern oscillation revisited: sea level pressures, surface temperatures, and precipitation. J. Clim. 13, 4358–4365. doi: 10.1175/1520-0442(2000)013<4358:tsorsl>2.0.co;2
van Asselen, S., Verburg, P. H., Vermaat, J. E., and Janse, J. H. (2013). Drivers of wetland conversion: a global meta-analysis. PLoS One 8:e81292. doi: 10.1371/journal.pone.0081292
Verhoeven, J. T. A., and Setter, T. L. (2010). Agricultural use of wetlands: opportunities and limitations. Ann. Bot. 105, 155–163. doi: 10.1093/aob/mcp172
Vizza, C., West, W. E., Jones, S. E., Hart, J. A., and Lamberti, G. A. (2017). Regulators of coastal wetland methane production and responses to simulated global change. Biogeosciences 14, 431–446. doi: 10.5194/bg-14-431-2017
Wang, X. Y., Li, X. C., Zhu, J., and Tanajura, C. A. S. (2018). The strengthening of Amazonian precipitation during the wet season driven by tropical sea surface temperature forcing. Environ. Res. Lett. 13.
Winter, T. C., and Rosenberry, D. O. (1998). Hydrology of prairie pothole wetlands during drought and deluge: a 17-year study of the cottonwood lake wetland complex in North Dakota in the perspective of longer term measured and proxy hydrological records. Clim. Change 40, 189–209.
Wohlfart, C., Winkler, K., Wendleder, A., and Roth, A. (2018). TerraSAR-X and wetlands: a review. Remote Sens. 10.
Yang, X. K., and Lu, X. X. (2014). Drastic change in China’s lakes and reservoirs over the past decades. Sci. Rep. 4:6041. doi: 10.1038/srep06041
Yoon, J. H., Wang, S. Y. S., Gillies, R. R., Kravitz, B., Hipps, L., and Rasch, P. J. (2015). Increasing water cycle extremes in California and in relation to ENSO cycle under global warming. Nat. Commun. 6:8657. doi: 10.1038/ncomms9657
Yu, K. F., Lehmkuhl, F., and Falk, D. (2017). Quantifying land degradation in the Zoige Basin, NE tibetan plateau using satellite remote sensing data. J. Mount. Sci. 14, 77–93. doi: 10.1007/s11629-016-3929-z
Zhang, Z., Zimmermann, N. E., Calle, L., Hurtt, G., Chatterjee, A., and Poulter, B. (2018). Enhanced response of global wetland methane emissions to the 2015–2016 El Niño-Southern oscillation event. Environ. Res. Lett. 13:074009. doi: 10.1088/1748-9326/aac939
Zhang, Z., Zimmermann, N. E., Stenke, A., Li, X., Hodson, E. L., Zhu, G. F., et al. (2017). Emerging role of wetland methane emissions in driving 21st century climate change. Proc. Natl. Acad. Sci. U.S.A. 114, 9647–9652. doi: 10.1073/pnas.1618765114
Zhao, D. M., and Wu, J. (2018). Changes in urban-related precipitation in the summer over three city clusters in China. Theoret. Appl. Climatol. 134, 83–93. doi: 10.1007/s00704-017-2256-9
Zhu, P., and Gong, P. (2014). Suitability mapping of global wetland areas and validation with remotely sensed data. Sci. China Earth Sci. 57, 2283–2292. doi: 10.1007/s11430-014-4925-1
Keywords: surface inundation, wetland dynamics, land use land cover changes, remote sensing, El Niño Southern Oscillations
Citation: Ilyas S, Xu X, Jia G and Zhang A (2019) Interannual Variability of Global Wetlands in Response to El Niño Southern Oscillations (ENSO) and Land-Use. Front. Earth Sci. 7:289. doi: 10.3389/feart.2019.00289
Received: 26 August 2019; Accepted: 21 October 2019;
Published: 14 November 2019.
Edited by:
Muhammad Bilal, Nanjing University of Information Science and Technology, ChinaReviewed by:
Md Firoz Khan, University of Malaya, MalaysiaBushra Khalid, International Institute for Applied Systems Analysis (IIASA), Austria
Copyright © 2019 Ilyas, Xu, Jia and Zhang. This is an open-access article distributed under the terms of the Creative Commons Attribution License (CC BY). The use, distribution or reproduction in other forums is permitted, provided the original author(s) and the copyright owner(s) are credited and that the original publication in this journal is cited, in accordance with accepted academic practice. No use, distribution or reproduction is permitted which does not comply with these terms.
*Correspondence: Anzhi Zhang, emhhbmdhbnpoaUB0ZWEuYWMuY24=