- 1Department of Bioscience, Arctic Research Centre, Aarhus University, Aarhus, Denmark
- 2Department of Earth, Ocean, and Atmospheric Science, Florida State University, Tallahassee, FL, United States
- 3School of Engineering, Aarhus University, Aarhus, Denmark
- 4Research Group Geomicrobiology, Department of Marine Biogeochemistry, GEOMAR Helmholtz Centre for Ocean Research Kiel, Kiel, Germany
- 5Aquatic Biology, Department of Bioscience, Aarhus University, Aarhus, Denmark
- 6Greenland Climate Research Centre, Greenland Institute of Natural Resources, Nuuk, Greenland
- 7Department of Estuarine and Delta Systems, NIOZ Royal Netherlands Institute of Sea Research and Utrecht University, Yerseke, Netherlands
- 8Centre for Earth Observation Science, University of Manitoba, Winnipeg, MB, Canada
The ecological impacts of meltwater produced by icebergs and sea ice in the waters around Greenland are poorly understood, due in part to limited observations. Current field sampling methods are resource and labor-intensive, and not without significant risk. We developed a small, unoccupied, and robotic platform to retrieve ice samples, while simultaneously eliminating safety risks to scientists and their support infrastructure. The IceDrone consists of a modified commercial hexcopter that retrieves ice samples. We describe the design requirements, construction, and testing of the IceDrone. IceDrone's capabilities were validated in the laboratory and during a field test in January 2019 near Nuuk (southwest Greenland). IceDrone retrieved samples in hard and dry glacial ice in harsh winter conditions. The field test led to modifications in the drilling head design and drilling process that enable it to retrieve samples in thin sea ice. All design files and software are provided in an attempt to rapidly enhance our collective understanding of ice-ocean interactions while improving the safety and productivity of field sampling campaigns.
1. Introduction
Anthropogenic climate change has resulted in a significant reduction in Arctic sea ice cover (Notz and Stroeve, 2016) and in accelerated mass loss from the Greenland Ice Sheet (GrIS) (Bamber et al., 2018; Wood et al., 2018). Arctic sea ice thickness has decreased significantly (Stroeve et al., 2012; Lindsay and Schwieger, 2015) and reductions in the thickness and extent of sea ice coverage, as well as melting of seasonal sea ice, can impact sea ice algae and phytoplankton productivity in Arctic seas and subsequent energy transfer to higher trophic levels (Leu et al., 2011; Harada, 2016; Assmy et al., 2017). Sea ice studies have been conducted by researchers on ice floes (Assmy et al., 2017) or suspended from icebreakers in baskets (Eicken et al., 2009) and both options present significant logistical challenges and safety risks. The ecological impacts reductions in sea ice thickness and extent on marine ecosystems are poorly understood due, in part, to limited observations. With the increase in thinner first-year and newly formed sea ice, as observed during the last decades (Laliberté et al., 2016), there is a basic need for sampling in thin sea ice (5–15 cm) without compromising safety. Sampling from boats and using remotely operated vehicles is possible (Lund-Hansen et al., 2018), but these platforms can disturb sea ice algae and areal coverage is still limited.
Icebergs can contain terrigenous sediments (Azetsu-Scott and Syvitski, 1999; Death et al., 2006; Smith et al., 2007, 2013; Mugford and Dowdeswell, 2010; Bigg, 2016), with considerable variability in concentration and distribution within a given iceberg, and in size fraction. These sediments are released into the marine environment as icebergs melt and may be an important source of iron, which can enhance marine primary productivity (Raiswell et al., 2008, 2016; Lin et al., 2011; Shaw et al., 2011; Bigg, 2016; Duprat et al., 2016; Hopwood et al., 2017, 2019; Hawkings et al., 2018). Glacial meltwater has also been shown to contain nutrients and silica, which may also enhance primary productivity (Azetsu-Scott and Syvitski, 1999; Meire et al., 2016). Thus, characterizing iceberg sediment and nutrient concentrations will aid in understanding the response of the marine ecosystem to iceberg meltwater fluxes, as well as understanding benthic sediment records of ice-rafted debris that are used to estimate iceberg distributions in paleoclimate scenarios (Death et al., 2006).
The total number of Arctic iceberg samples analyzed is not known, but a literature survey suggests that the number is 100–200. The relatively small number of samples is understandable when the logistical and safety aspects of sample collection are considered. Iceberg samples have been retrieved using helicopters (Diemand, 1984; Gagnon and Gammon, 1997), and from small boats, either by chipping pieces off with an ice axe (Hawkings et al., 2018) or by collecting floating pieces of glacial ice from the water (Hopwood et al., 2016, 2017; Meire et al., 2016). Helicopters have also been used to deploy GPS trackers (Sutherland et al., 2014; Jones and Gudmundsson, 2015; Larsen et al., 2015; Sulak et al., 2017) and wave motion sensors (Lever et al., 1991). Icebergs pose a significant safety risk as they can disintegrate and/or roll with little to no warning (Lewis and Bennett, 1984; Macayeal et al., 2011; Heller et al., 2019). Similarly, submerged ice may break off undetected as a result of the buoyancy-driven “foot-loose” mechanism (Wagner et al., 2014).
Thus, ice sample collection presents significant logistical challenges, expenses, and safety risks. Even under ideal weather conditions, resources like boats, helicopters, snow mobiles, and sampling baskets are in high demand during field expeditions. In an attempt to enhance our collective understanding of iceberg meltwater and sea ice algae, and their respective roles in primary production, while simultaneously reducing risks to scientists and their support vessels, we developed a novel robotic ice sampling platform. This paper describes the design, construction, and field validation of the IceDrone, which is a student-built ice sampling system that is mounted on an unmanned aerial vehicle (UAV, or “drone”). The IceDrone consists of a commercial hexcopter that is modified to retrieve ice samples. The remainder of this manuscript is organized as follows. In section 2 we summarize the operational requirements that guided the design process and describe the major components of the IceDrone. The results of laboratory and field tests are presented in section 3. The performance of IceDrone is evaluated in section 4 and design upgrades are described. We conclude in section 5.
2. Materials and Methods
2.1. Design Requirements
The long-term goal is the development of an autonomous robotic ice sampling system and we focus here on the first step of adapting an existing drone platform to retrieve ice samples. Here we describe the operational requirements for the commercial drone system and the ice sampling system. Both systems must be capable of operating in Arctic conditions, which include air temperatures of ±15°C, high relative humidity, rain/snow, and wind speeds up to 10 ms−1 (Table 1).
2.1.1. Drone Requirements
The requirements outlined in Table 1 were used to evaluate commercial drone systems. Briefly, the drone must be able to: (1) take off from a small boat; (2) fly a distance of 0.1–1 km to an iceberg; (3) land on the iceberg; (4) collect an ice sample; (5) return to the boat. Given that the drone will operate from a boat and land on an iceberg, the drone must be able to take off and land from translating, rotating, pitching, rolling, and heaving surfaces and on uneven surfaces. For testing and field sampling, the drone size and weight must adhere to the respective regulations in Denmark and Greenland. The drone must be portable, for transport on commercial aircraft, and capable of operating from small workboats. The airframe must be capable of integrating the ice sampling system and the drone must provide sufficient lift to carry the custom payload and the ice sample. The drone system must include GPS and altitude-hold flight modes, fail safes, and redundant sensors. The drone must include a high resolution stabilized camera, both to monitor drilling operations and to collect still imagery to construct three-dimensional models of the icebergs using photogrammetry software (Crawford et al., 2018). The remote controller used by the operator must display critical flight data (GPS quality, battery level, and flight mode) and live video.
2.1.2. Ice Sampling System Requirements
Ice samples have been collected using a coring system (Talalay, 2016b) or, in the case of icebergs, by chipping off pieces with an ice axe (Hawkings et al., 2018). While humans can easily handle an ice axe and collect the fragments, this task is difficult for a robotic system. Ice sampling system requirements are outlined in Table 1. The ice sampling system must be able to retrieve at least 0.5 kg of ice and should be installed without permanent modification to the drone in under an hour. The sampling system should be operated by the remote controller used to fly the drone but the sampling system should operate independently from the drone's power distribution system and flight controller. Thus, the sampling system should take inputs from the drone's flight controller while providing its own power and processor. The sampling system should be capable of carrying and releasing a small GPS tracker, in the form of either an Expendable Iceberg Tracker (Carlson et al., 2017) or a real-time iceberg tracker (Carlson and Rysgaard, 2018). The sampling system should provide a warning if the iceberg rolls and should provide sufficient buoyancy in the event of an emergency water landing or to permit recovery in the event of a crash.
2.2. The IceDrone
Here we describe the basic design and construction of the IceDrone. Technical drawings, design files, and software can be downloaded from Carlson et al. (2019) and an outreach video can be viewed here: https://vimeo.com/323408072.
2.2.1. IceDrone
IceDrone is built around a DJI Matrice 600 Pro (M600) hexcopter, which was selected because it satisfies all the requirements in Table 1. The M600 has been used in many applications because of its ease of use, lift capacity, endurance, portability, redundancy, and customizability (Boehmler et al., 2018; Li et al., 2018; Arroyo-Mora et al., 2019). The M600 features folding propellers and arms and triple redundant GPS and inertial motion units (IMUs). We selected DJI's Zenmuse X3 camera and 3-axis gimbal, which stabilizes the camera and can pan and tilt to monitor drilling operations. The Zenmuse X3 features a 12.4 megapixel sensor that can capture ultra high definition 4K video and jpeg still images and its use in photogrammetry applications has been previously demonstrated (Han et al., 2017). The DJI CrystalSky Display is used to monitor the live video feed and drone status data. The M600 remote controller expansion kit provides eight additional knobs that can be mapped to control drilling system functions.
2.2.2. Initial Ice Sampling System
The first drill was constructed from a 250 mm long aluminum tube with an inner diameter of 70 mm and a thickness of 10 mm, resulting in a total internal volume of 0.93 L (Figure 2). The aluminum tube fits inside a 3D printed spiral sleeve that removes ice cuttings (Figure 2). The drilling head is also 3D printed, to reduce weight and cost, and features a modified wood auger center drill bit to assist in starting the drill autonomously (Figure 2). The drilling head uses three sets of cutting teeth at an angle of 40° (Figure 3) that were constructed of hardened stainless steel and coated in PVD to increase hardness and abrasion resistance. Cutting teeth were staggered to reduce icing (Figure 3; Talalay, 2016a). The drill (Figure 2) can be installed and removed easily and permits the use of an extraction tool to remove the ice sample (Figure 4). A 3,600 KV brushless motor with a 67:1 gear ratio maintains a drill speed of 645 RPM in air. The brushless motor is powered by a 3S 4,000 mAh lithium polymer (LiPo) battery and all components are protected by appropriate fuses.
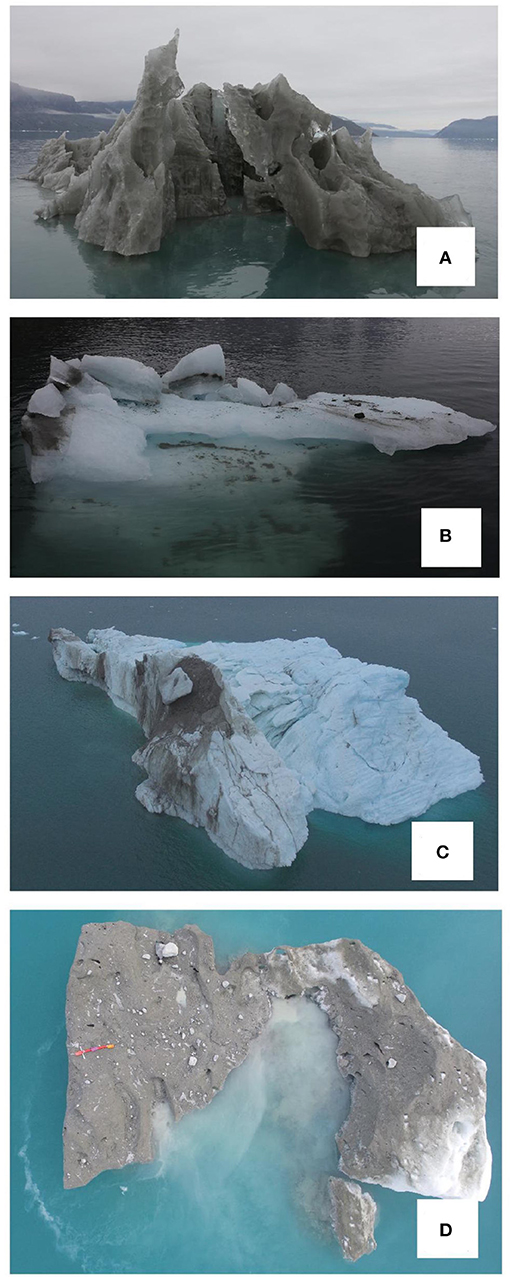
Figure 1. Icebergs and their sedimentation patterns take on different forms in Greenland: (A) Fine-grained sediment frozen into the entire visible iceberg sail; (B) Narrow bands of fine-grained sediment and larger debris; (C) A freshly calved iceberg shows a sediment layer from the top of its parent glacier; (D) A melting, sediment-laden bergy bit with larger stones and debris. Its turbid meltwater plume is also visible.
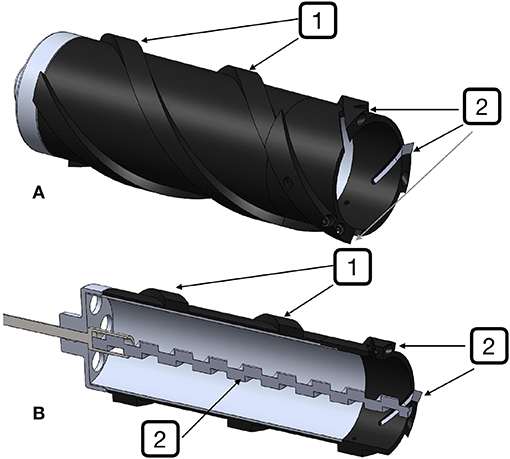
Figure 2. (A) A rendering of the final drill design. (B) A cutaway that shows the aluminum cylinder and the center drill. The following components are labeled: 1. The spiral sleeve; 2. cutting teeth; center drill.
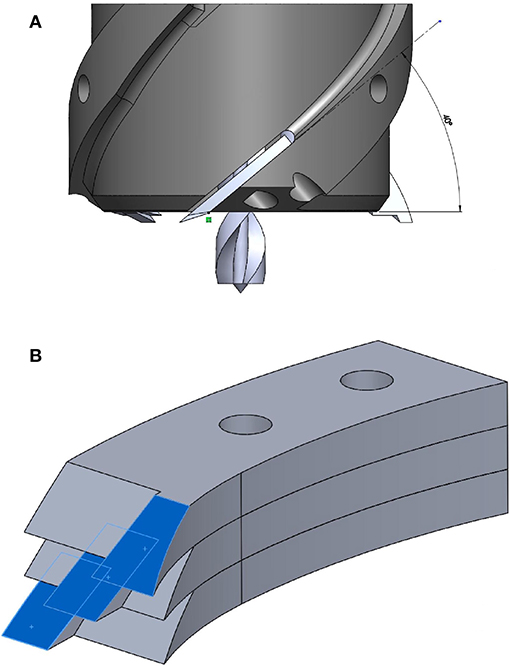
Figure 3. (A) The 3D-printed cutting head features three 40° cutting teeth. (B) The cutting teeth are staggered to reduce icing.
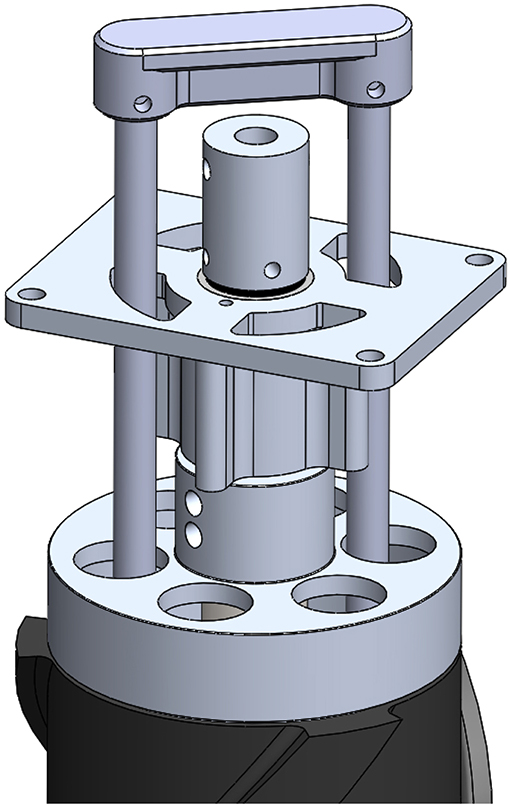
Figure 4. An extraction tool uses two aluminum rods that fit through holes at the top of the drill to remove the ice sample.
The standard landing gear were removed and custom landing gear were mounted to the airframe using 3D printed clamps (Figure 5). The custom landing gear system includes hollow axle stepper motors and flotation for emergency water landings. The stepper motors move the drone and ice sampling system up and down to control the drilling depth. The stepper motors were easy to implement and permit high precision movement. The landing gear were stabilized using triangular frames, mounted on top and bottom (Figure 5). The ice sampling system can also release an iceberg tracker using a solenoid actuator. The rolling alarm uses an inclinometer and a LED indicator that is placed in the field of view of the camera, which alerts the operator if the iceberg's orientation changes. The brushless drilling motor, stepper motors, release mechanism, and rolling alarm are controlled by LabView VIs running on a National Instruments myRIO. The aforementioned components are installed on a custom aluminum mounting plate along with the camera (Figure 6). Figure 7 shows a rendering of the drone and drilling system and the completed prototype during buoyancy testing.
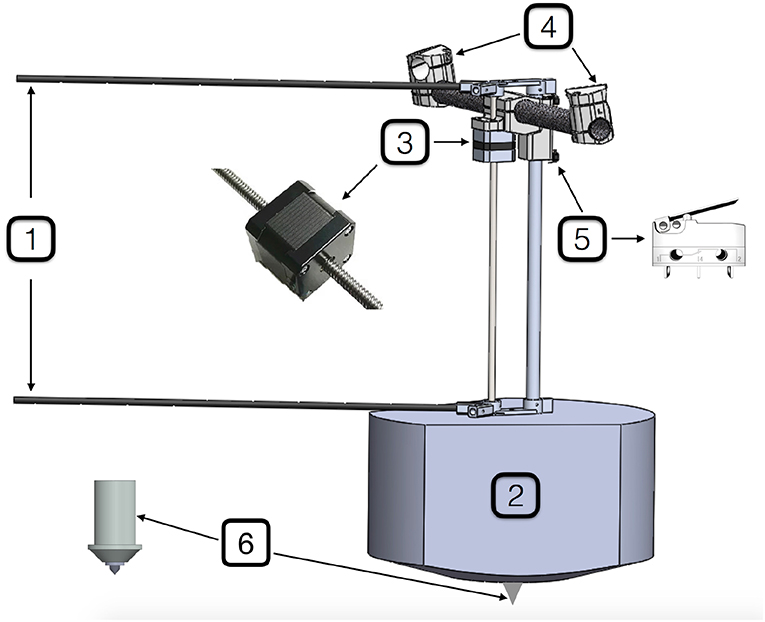
Figure 5. The custom landing gear with the following components labeled: 1. carbon fiber stabilizing rods; 2. foam flotation; 3. hollow axle stepper motors move the drill system up and down threaded lead screws; 4. 3D printed clamps used to attach the landing gear to the drone; 5. Microswitches that define the vertical range of motion; 6. stainless steel spikes.
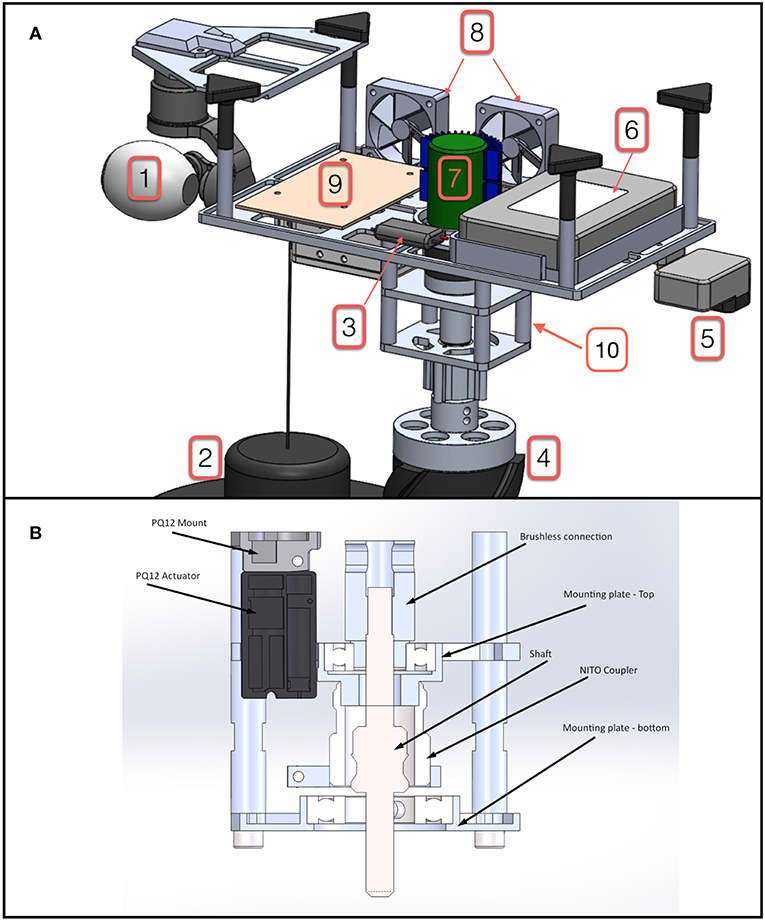
Figure 6. (A) The mounting plate for the electronics with the following components labeled: 1. Zenmuse X3 camera; 2. GPS tracker suspended from release mechanism; 3. electronic speed controller for the brushless motor; 4. drill (Figure 2); 5. optional thermal camera mount; 6. myRIO; 7. brushless motor; 8. cooling fans; 9. Bracket for 3S 4000 mAh LiPo battery; 10. Mounting plate for brushless motor connection to the drill shaft. (B) Cross-sectional view of the quick-release mechanism that uses a NITO coupler that is moved by a linear actuator.
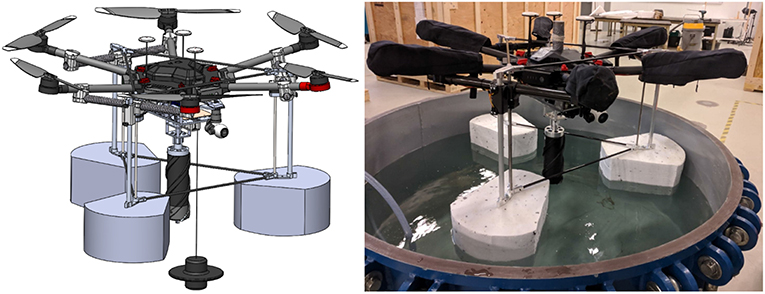
Figure 7. (Left) A rendering of the final design. (Right) The completed prototype during buoyancy testing.
Blocks of ice were used for laboratory testing. During initial tests, the weight of the drone and batteries was replicated using a 4 kg weight. The weight of the final design was 18 kg. Additional tests were conducted with the entire drone and drilling system after the final cutting head design was selected and mounted on the drone. Tests successfully retrieved intact ice samples that weighed slightly more than 500 g. Tests revealed that downward motion followed by brief upward motion drilling was more effective. The tests also revealed that the coring system operated at 590 RPM under load and that the stepper motors were capable of lifting 32 kg. Applying ski wax to the outer spiral sleeve helped to prevent icing.
2.3. Sample Analysis
IceDrone was validated in the field in Godthåbsfjord, near Nuuk, in early January 2019. The drone and the drilling system operated in adverse weather conditions, which included air temperatures down to −15°C, wind speeds in excess of 12 ms−1, and snow. A 1.6 × 1.6 m landing platform was constructed from plywood and secured to the stern of a small workboat from the Greenland Institute of Natural Resources.
2.3.1. Iceberg Analysis
The iceberg sample was melted in the laboratory for analysis of δ18O and δ2H isotopes, nutrients (nitrate, silicate, and phosphorous) and iron species. The isotopic compositions of the samples were analyzed with a Cavity Ringdown Spectrometer, L2130-i Isotopic H20 (Picarro Inc., USA). Six injections were taken from each sample and three were excluded in order to remove any residual results from the previous sample. Vapor content, δ2H, and δ18O values were calculated relative to standards. Four standards were measured at the beginning and end of the sample set. The external standards used to calibrate the results were Vienna Standard Ocean Water 2 (VSMOW2) and Standard Light Antarctic Precipitation 2 (SLAP2). Concentrations of nitrate, phosphate and silicate were determined on a Smartchem200 (AMS Alliance) auto-analyzer following procedures as outlined in Wood et al. (1967) for and , Murphy and Riley (1962) for , and Koroleff (1983) for determination of H4SiO4.
A sequential Fe extraction was performed to determine the Fe content and speciation. Fe was extracted from a 10 ml melted sample, first for 1 h with 0.5 M HCl (bioavailable fraction) and then for 24 h at 70°C in 6 M HCl (crystalline fraction). All extraction steps were performed under anoxic conditions (100 % N2 atmosphere) to avoid changes in Fe redox speciation during extraction due to O2. After both extraction steps, the samples were centrifuged for 15 min at 5,000 × g (Hermle 7300). Then, 200 μl of the supernatant was stabilized in 800 μl of 1 M anoxic HCl. Fe(II) and total Fe concentrations were determined by the spectrophotometric Ferrozine assay in triplicate with a spectrophotometric plate reader (FlashScan 550; Analytic, Jena, Germany). For calculation of Fe(III) concentrations, the concentration of Fe(II) was subtracted from the total Fe concentration.
2.3.2. Sea Ice Sample Analysis
Six sea ice samples were collected from land-fast ice in a shallow bay near the iceberg sampling site. The samples were sliced in half and each half was melted, filtered and analyzed for chlorophyll-a following Lund-Hansen et al. (2016).
3. Results
3.1. Field Validation
3.1.1. Iceberg
Several grounded icebergs were found north of Nuuk in shallow embayments, however, most were irregularly shaped and did not offer a suitable landing zone. One grounded iceberg with a single pinnacle appeared to have a suitable landing area. The top of the pinnacle was >10 m high and was, therefore, evaluated using a Phantom 4 drone, which revealed what appeared to be a suitable landing area. IceDrone landed on the top of the pinnacle and settled into a shallow bowl-shaped depression (Figure 8). A 0.8 kg sample was retrieved and was later processed for nutrients and iron (Figure 8).
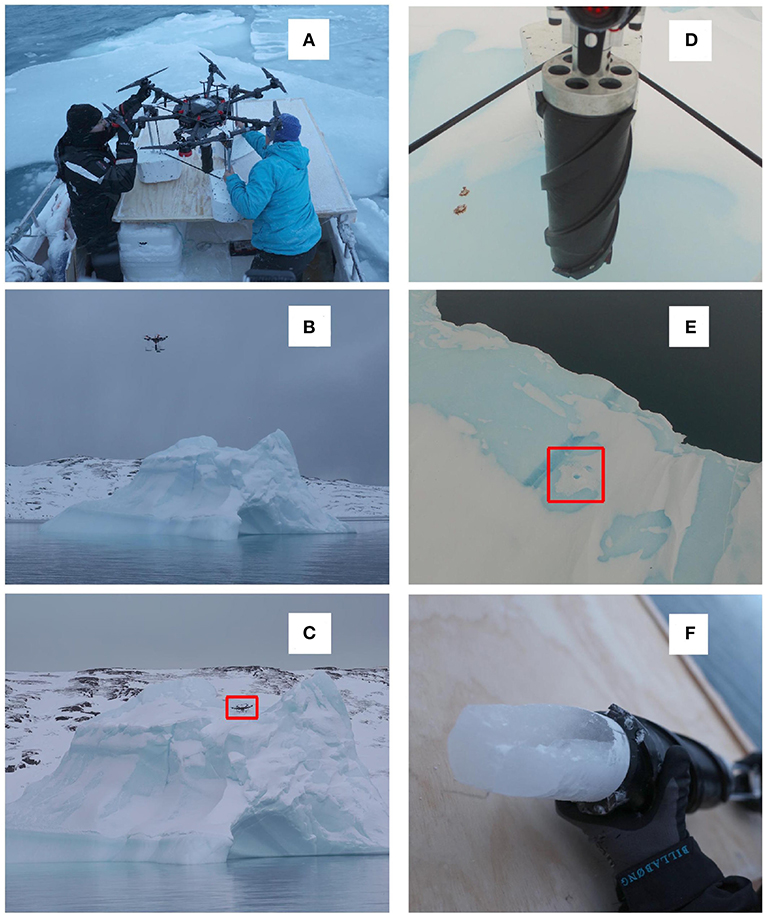
Figure 8. (A) Preflight checks on the plywood landing platform (those pictured are coauthors; image used with their consent). (B) IceDrone approaching the iceberg and preparing to land. (C) IceDrone landed on top of the iceberg (red square). (D) IceDrone drilling into the top of the iceberg. (E) The hole (red square) was clearly visible after takeoff. (F) Removing the ice sample using the extraction tool.
After the sample was retrieved, IceDrone acquired a combination of nadir-looking and oblique still images of the iceberg by flying around the iceberg several times to ensure adequate overlap of the images. Additional images were acquired from the landing platform as the boat completed several circuits around the iceberg. In total, 223 drone images were acquired, along with 60 images from a Canon 5DSR Mk III camera equipped with a 24 mm lens. Out of 283 images, 230 were aligned in Agisoft Photoscan Pro (version 1.4.5) and these images were processed to create a high-resolution 3D model of the iceberg (Figure 9). The images and the 3D model of the iceberg do not reveal any sediment. The imagery component of the field sampling strategy is more relevant for icebergs with strong spatial heterogeneity (e.g., Figures 1B,C). Given that this iceberg was grounded well inside a shallow embayment in winter, a GPS tracker was not deployed.
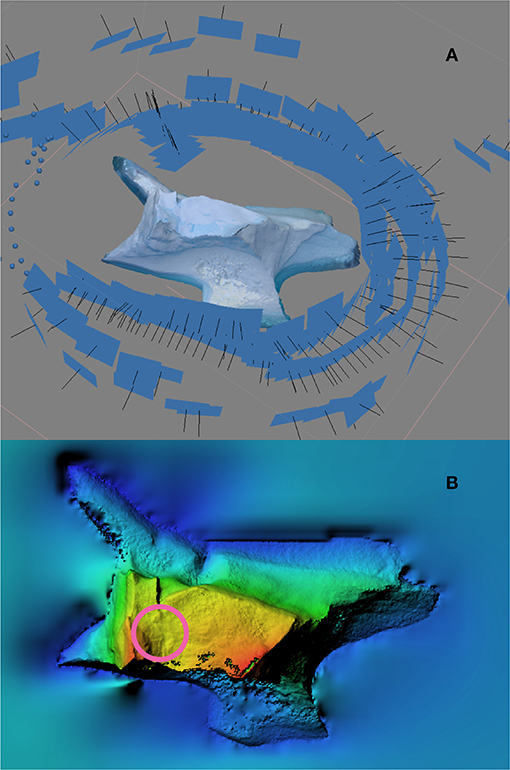
Figure 9. (A) A high resolution 3D model constructed from 230 images that were processed in Agisoft Photoscan. The locations of aligned images are indicated by the blue rectangles. (B) The digital elevation model (DEM) produced by Photoscan with the sampling site indicated by the pink circle. Drone-generated DEMs could be used to select a suitable landing site in future autonomous versions of IceDrone.
Based on triplicate readings, the iceberg sampled had δ18O and δ2H values of −26.33 ± 0.08‰ and −198.17 ± 0.59‰, respectively. Nutrient analysis of the same iceberg sample showed nitrate concentrations of 1 μM, whereas ammonium (<0.03 μM), phosphate (<0.06 μM) and silicate (<0.2 μM) were below detection limits. In the 0.5 M HCl (bioavailable) fraction Fe(II) was below detection limit and Fe(III) at 4 μM. In the 6 M HCl (crystalline) fraction Fe(II) was at 7 μM and Fe(III) at 51 μM.
3.1.2. Bergy Bit
A second sampling test was attempted on a grounded bergy bit. The initial orientation of the bergy bit provided a relatively flat and level landing area that was free of obstructions and covered in light snow. IceDrone landed and began drilling (Figure 10A). The tide was ebbing during this time and the reduction in water level changed the buoyant force on the bergy bit and it rolled to a new stable orientation (Figure 10B). The change in orientation occurred rapidly and without warning. Luckily, the drill held IceDrone in place and it was able to take off from the inclined surface.
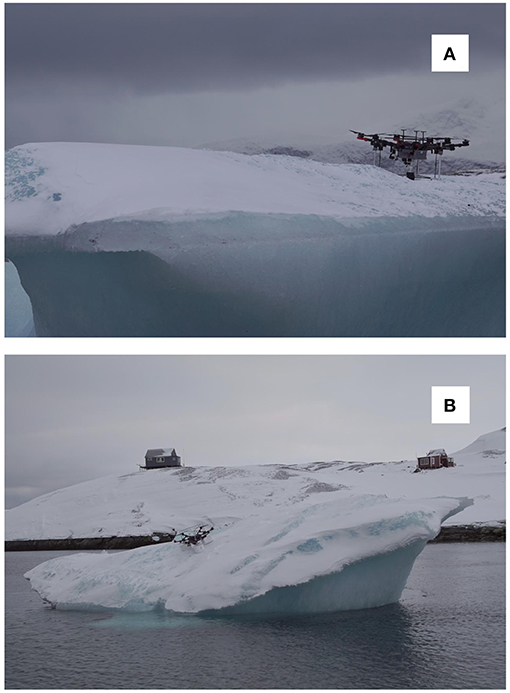
Figure 10. (A) IceDrone drilling on a grounded bergy bit. (B) IceDrone held in place by the drill after the bergy bit rolled.
3.1.3. Sea Ice
Multiple attempts were made to retrieve sea ice samples in land-fast ice in shallow embayments near Nuuk (Figure 11). The drilling head design lacked core dogs (also called “core catchers”) or a collet to retain the sample, and once seawater entered the drill the ice sample slipped out and was collected manually. Six sea ice samples 25–30 cm in length were retrieved by hand, after they slipped from the drill, and analyzed for chlorophyll-a. As expected, chlorophyll values were low near the top of the ice, ranging from 0 to 0.4 μg chl L−1. Chlorophyll values near the bottom of the sea ice ranged from 0.14 to 1.84 μg chl L−1.
4. Discussion
4.1. Iceberg Analyses
IceDrone retrieved complete ice samples in every laboratory test and succeeded in retrieving a sample from an iceberg during a field test in Greenland. The iceberg isotope samples collected by IceDrone compare with previous samples collected by hand in Godthåbsfjord (Rysgaard, unpublished). In total 200 measurements of δ18O were collected from icebergs in Godthåbsfjord in 2012, ranging from −30.04 to −17.91‰ with a median of δ1818O = −28‰. In addition, a similar number of δ2H measurements exist from icebergs sampled by hand in the fjord ranging from −218.47 to −147.56‰ with a median of δ2H = −218.47‰. Nutrient samples from icebergs in Godthåbsfjord reveal large variability in dissolved silicate concentrations ranging from below the detection level in clear ice samples up to 18 μM in debris-rich ice samples (Meire et al., 2016). Nitrate and phosphate levels in glacier ice have previously been detected at 1 μM (Wadham et al., 2016) and up to 0.35 μM (Hawkings et al., 2016), respectively. The amount of bioavailable Fe(III) that we measured is similar to what was previously found in iceberg samples from around the world, which was reported to be around 2.7–17 μM (Raiswell et al., 2016; Hopwood et al., 2017). The more crystalline Fe phases were found to vary globally between 17 and 64 μM (Raiswell et al., 2016), which also fits with our 6 M HCl extractable Fe(III) fraction.
4.2. Initial Performance Evaluation
IceDrone demonstrates great potential to increase our understanding of iceberg and sea ice inputs to the upper ocean but it is a prototype that was designed and built by undergraduate students under tight time and budgetary constraints. Here we list issues that were identified during the initial tests that took place near Nuuk in January 2019. These issues led to hardware and software improvements that were implemented by a second group of undergraduate students as part of their mechatronics curriculum and these are described in sections 4.3–4.4.
4.2.1. Sample Retrieval
Sea ice samples slipped from the drill and had to be retrieved by hand. Adding core dogs or a collet would also allow IceDrone to retain samples from thin sea ice, an environment that is unsafe for researchers.
4.2.2. Quick Release
IceDrone's initial design did not feature a quick release mechanism so it could not disengage or jettison the drill if it became stuck or in the event of an iceberg rolling emergency. Drill sticking is a problem for ice coring operations in general (Talalay, 2016a) and a release mechanism would allow IceDrone to free itself, which could enable unattended sampling and land-based operations, as a boat would no longer be necessary to retrieve the system if the drill stuck.
4.2.3. Drone Orientation
The rolling bergy bit (Figure 10) highlights the risk of iceberg instability and the need for an improved autonomous response. The initial solution used an LED that simply alerted the operator and future versions of IceDrone could include an autonomous response that is initiated if the iceberg rolls past a threshold, after which point the drill would be released, allowing the drone to take off quickly and autonomously.
4.2.4. Camera
The camera feed from the drone was helpful in monitoring drilling operations but vibrations sometimes overloaded the gimbal motors, which made it respond erratically to pitch and pan commands from the controller. Vibration dampeners should be installed on the gimbal mount to eliminate this problem.
4.2.5. Sediment and Firn
High sediment loads and larger debris can damage or break cutting teeth (Cao et al., 2015) and IceDrone has yet to be tested under these conditions. Similarly, drilling is only possible on ice that is free of any appreciable snow and/or firn layer. While this is a limitation, drilling through firn remains a general challenge for the ice sampling community (Talalay, 2016a).
4.2.6. Landing and Sampling Site Selection
The size of the Matrice 600 limits sampling to icebergs with relatively flat surfaces with minimum dimensions of ~2 × 2m. As a result, icebergs with complex geometries or steep slopes (e.g., Figure 1A) cannot be sampled using the IceDrone. During the field tests reported here, sampling sites were selected visually by the drone operator for testing purposes only and the landing on the iceberg was carried out using the live video feed. The emphasis was on testing the drilling system and, as a result, drone imagery of the iceberg was only acquired after an ice sample was successfully retrieved. Following Jouvet et al. (2019), the drone imagery could have been acquired first and used to construct a digital elevation model (DEM; Figure 9B) of the iceberg to aid in landing site selection. For our test iceberg, photogrammetric reconstruction of the iceberg geometry was simplified by the fact that the iceberg was grounded and photogrammetry-based site selection may be more complicated when targeting drifting icebergs (Crawford et al., 2018). Future iterations of IceDrone could include onboard image processing algorithms that can autonomously select scientifically interesting sampling sites and then rank them based on the presence/absence of obstacles and the slope of the surrounding surface. We also note that autonomous landing site selection on uneven and unprepared terrain remains an active area of research (e.g., Forster et al., 2015; Stolz et al., 2018; Cheng et al., 2019; Pestana et al., 2019) and was well-beyond the scope of the undergraduate student projects featured here. Stereoscopic first-person-view (FPV) goggles may be a more practical short-term solution (e.g., Smolyankskiy and Gonzalez-Franco, 2017) to enable semi-autonomous operations.
4.3. Hardware Improvements
4.3.1. Long-Range Communications
An XBee SX 868 MHz transceiver was added for long-range communications. While this issue was not encountered during the field validation, the upgrade will permit the drone to telemeter critical status data over ranges up to 14.5 km. The 868 MHz operating frequency also does not interfere with the drone's 2.4 GHz datalink.
4.3.2. Current Sensor
A current sensor was installed on the positive lead between the drill battery and the ESC to measure the current load on the drill. These current measurements are used by the upgraded software to automate the drilling process (see section 4.4). A Polulu distributed board based on the Allegro ACS711EX hall-effect current sensor is used here. The maximum current observed during the field validation was 14 A DC. The Polulu board provides a current rating of ±31 A DC and a voltage range of 100 Volts DC, which affords a suitable safety margin of 2.2 for current spikes during motor startup and stall conditions while providing constant measurement capabilities (Carlson et al., 2019).
4.3.3. Electronics Box
An electronics box was designed to house the main circuit board, the myRIO, the XBee module, the electronic speed controller (ESC) and the LiPo battery for the DC-motor that drives the drill (Figure 12). The box also serves as a mount for the DC-motor and drill suspension and the Zenmuse X3 camera. The design of the electronics box focused on ease of access, weatherproofing, and mechanical endurance. The electronics box is made from nylon (PA 2200) and is manufactured by selective laser sintering (SLS) 3D printing, which offers very few design limitations and rapid print times.
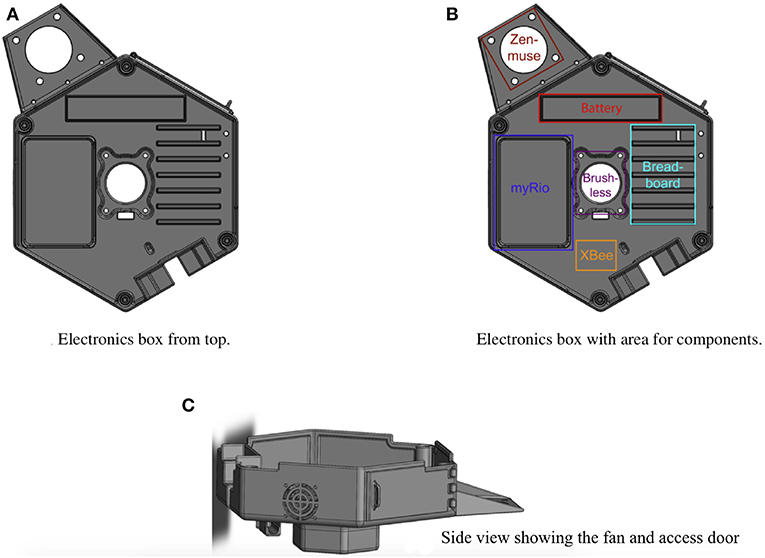
Figure 12. The improved electronics box is constructed SLS nylon, which is lightweight and durable. (A) Top view; (B) top view with mounting areas for components labeled. (C) Side view with the vent for the cooling fan shown. The access door is to the right of the fan vent.
The hexagonal shape of the box matches the geometry of the drone's frame and makes the overall design visually appealing while simplifying integration. The interior of the box accommodates the electronic components and access holes simplify wire routing for external components. A hinged door allows the battery to be changed easily. The box also features a vent for the fan to prevent the electronic components from overheating, as the stepper motor drivers and the DC-motor produce significant amounts of heat during operation. A finite element model study was performed to ensure that the box can withstand the torque applied by the drill motor (Carlson et al., 2019).
4.3.4. Landing Gear
The landing gear are constructed of expanded polystyrene (EPS) foam with spikes (Figure 5). The height of the EPS foam was reduced from 200 to 120 mm to accommodate the weight of the drone and drilling system (18 kg) with a safety factor of 1.5, which equates to an effective weight of 27 kg. The spikes were redesigned to use stainless steel track and cross country spikes. The spikes are glued in a 3D printed shaft using medium strength Loctite, which allows the spike to detach if it freezes to the ice.
4.3.5. Quick Release
The quick release uses a NITO coupler, which is commonly used for hose attachments in compressed air systems. The NITO coupler was chosen because of its thoroughly tested and proven design. The drill shaft design was modified to ensure that the shaft can transfer torque from the brushless motor to the drill and secure the drill to the drone while also detaching quickly. The shaft modifications include a hexagonal tip that ensures a tight, but sliding fit, with the brushless connector on the motor (Figure 6B). The mid-section of the shaft is milled to match the geometry of the male NITO coupler fitting, which allows the shaft to be secured to the corresponding female NITO coupler, which is fixed to the drone. An Actuonix PQ12 linear actuator moves the female coupler, which allows the shaft to be secured and jettisoned, if necessary. M8 threading at the bottom of the shaft is used to connect the drill.
4.3.6. Drill Design
A new drill design modifies the drill shaft (see section 4.3.5), the length of the drill, the inner and outer diameters, and the angle and number of cutting teeth. The new design also includes features aimed at retaining ice samples, which include a single one-way vent on the top of the sleeve that is closed by a rubber membrane and core dogs near the bottom of the sample. The outer spiral jacket now uses a hollow construction from SLS printed nylon to save weight. SLS nylon also offers improved elasticity at sub-zero temperatures.
The cutting teeth on the drill head are now secured using countersunk screws, which simplifies the geometry from a straight chamfer to a variable filet. The clearance between the drill head and each cutting tooth has been increased to avoid material jamming. The drill head also features a spring-loaded core dogs, which grab and break off the ice sample. This spring-loading forces the core dog to skate along the ice sample as it enters the drill body. Once the drill is retracted, the sharpened upper edge of the tooth engages with the ice sample, and as the retraction continues, drives the tooth further into the sample, severing it from the iceberg. Once retrieved, cut-outs on the core dogs enable the operator to swivel them out to release the ice sample.
The field validation (see section 3.1) revealed that the PVD coating wore off with minimal use and the cutting teeth and center drill were spotted with corrosion from salt incursion. The cutting teeth and chipmunk teeth are constructed from direct metal laser sintering (DMLS) printed titanium, which features enhanced wear resistance and allows for custom geometries. The center drill bit is now constructed from a DMLS printed titanium tip and a machined aluminum shaft.
4.3.7. LiDAR
Three Benewake TFMINI Micro LiDAR modules were installed on each end of the triangular frame. The LiDAR modules have a range of 12 m and are used to assess the iceberg surface before landing and to track the progress of drilling activities. The LiDAR distances during drilling are displayed in the form of a progress bar on the ground station display.
4.3.8. Microswitches
Microswitches are used during drilling to indicate when one of the limits of the lead screws has been reached (Figure 5). When a microswitch is pressed, the stepper motor has reached either the top or the bottom of the landing gear frame and needs to stop. The micro switch sends a signal through LabVIEW to the corresponding Big Easy Driver, pulling the enable pin high, which causes the stepper to stop. In the original design, the microswitches were not weatherproof and the wires were routed externally. The microswitches are now covered by 3D printed shields that also enclose their wiring.
4.4. Software Improvements
4.4.1. LiDAR
The LiDAR data are read through UART with LabVIEW. Bad readings are identified and discarded. The current draw from the drill is also read using LabVIEW and these data are combined with the LiDAR data and microswitches to automate the drilling process (Figure 13). The drone operator simply indicates that the drone has landed using one of the switches on the remote controller, which initiates the automated drilling process. The drilling process operates the drill at maximum RPM while moving the stepper motors downward until a resistance threshold is met, or until the drilling process is complete, which is indicated by the microswitches (Figure 13). If the resistance threshold is met before drilling is complete, the steppers raise the drill until resistance decreases below the threshold and then downward motion resumes until drilling is complete, or the resistance threshold is met again. When all bottom microswitches are activated the “triangle wobble” process begins, whereby one stepper is raised at a time in a clockwise fashion that acts to snap the ice sample free. Afterwards, all stepper motors move upwards until all top microswitches are activated, at which point the drill motor stops.
5. Conclusions
We demonstrated the design, construction, and testing of IceDrone, an open source and robotic ice sampling platform that was developed around a commercial, off-the-shelf DJI Matrice 600 Pro hexcopter. Laboratory and field tests showed that IceDrone can autonomously retrieve and hold shallow ice samples in glacial ice. Attempts to retrieve samples from thin sea ice were not totally successful, but should be possible with some minor modifications to the design, as described in the Discussion. Thus, IceDrone has demonstrated the potential for unmanned aerial drone systems to aid in ice sampling operations and the prototype demonstrated here paves the way for the development of more capable and fully autonomous systems. IceDrone enhances safety by eliminating the need to operate near icebergs. IceDrone may also increase productivity on research vessels where logistical resources like small boats and helicopters are in high demand, and, even when they are available, their use is strongly weather-dependent for obvious safety reasons. While IceDrone was originally developed with icebergs in mind, it may also be used to obtain samples from other potentially hazardous environments, such as thin sea ice and on glaciers and should considered for use in large-scale monitoring programs (e.g., Druckenmiller et al., 2009; Straneo et al., 2019).
Data Availability Statement
The design files and software required to replicate the IceDrone system are publicly available via Mendeley Data at: http://dx.doi.org/10.17632/jx7b5t4f6j.1.
Author Contributions
DC provided the minimum operating requirements for the IceDrone. JP and MJ were responsible for design and construction, and they were supervised by CM. JP and MJ installed the drilling system in the field and CM flew the drone. LL-H, SR, and LM provided the expertise with ice drilling and field logistics. LL-H analyzed the sea ice samples. KL performed the iron analyses. MH, ST, JL, FT, and AF upgraded the IceDrone design based on feedback from the field tests. DC organized the field campaign, analyzed the drone imagery, and wrote the manuscript. All authors contributed to the experimental design and to drafting the manuscript.
Conflict of Interest
The authors declare that the research was conducted in the absence of any commercial or financial relationships that could be construed as a potential conflict of interest.
Acknowledgments
We would like to thank the Danish Technological Institute for sponsoring the SLS and DMLS 3D printed parts. We thank Caspar Haarløv for making the IceDrone outreach video and the Lundbeck Foundation for funding his participation in the expedition. We gratefully acknowledge the contributions of the Arctic Research Centre (ARC) at Aarhus University and the Greenland Institute of Natural Resources. We would especially like to thank Flemming Heinrich for making our January 2019 field validation a success. This work is a contribution to the Arctic Science Partnership (ASP) http://asp-net.org.
References
Arroyo-Mora, J. P., Kalacska, M., Inamdar, D., Soffer, R., Lucanus, O., Gorman, J., et al. (2019). Implementation of a UAV-hyperspectral pushbroom imager for ecological monitoring. Drones 3:12. doi: 10.3390/drones3010012
Assmy, P., Fernández-Méndez, M., Duarte, P., Meyer, A., Randelhoff, A., Mundy, C., et al. (2017). Leads in Arctic pack ice enable early phytoplankton blooms below snow-covered sea ice. Sci. Rep. 7:40850. doi: 10.1038/srep40850
Azetsu-Scott, K., and Syvitski, J. P. (1999). Influence of melting icebergs on distribution, characteristics and transport of marine particles in an east Greenland fjord. J. Geophys. Res. 104, 5321–5328.
Bamber, J. L., Tedstone, A. J., King, M. D., Howat, I. M., Enderlin, E. M., van den Broeke, M. R., et al. (2018). Land ice freshwater budget of the Arctic and North Atlantic Oceans: 1. Data, methods, and results. J. Geophys. Res. Oceans 123, 1827–1837. doi: 10.1002/2017JC013605
Bigg, G. R. (2016). Icebergs: Their Science and Links to Global Change. Cambridge: Cambridge University Press.
Boehmler, J. M., Lorìa-Salazar, S. M., Stevens, C., Long, J. D., Watts, A. C., Holmes, H. A., et al. (2018). Development of a multispectral albedometer and deployment on an unmanned aircraft for evaluating satellite retrieved surface reflectance over Nevada's Black Rock Desert. Sensors 18:3504. doi: 10.3390/s18103504
Cao, P., Yang, C., Chen, Y., Chen, B., and Talalay, P. (2015). Experimental study of the drilling process in debris-rich ice. Cold Regions Sci. Technol. 120, 138–144. doi: 10.1016/j.coldregions.2015.10.001
Carlson, D. F., Boone, W., Meire, L., Abermann, J., and Rysgaard, S. (2017). Bergy bit and melt water trajectories in Godthabsfjord (SW Greenland) observed by the expendable ice tracker. Front. Mar. Sci. 4:276. doi: 10.3389/fmars.2017.00276
Carlson, D. F., Pasma, J., Jacobsen, M. E., Holm, M. H., Thomsen, S., Lillethorup, J. P., et al. (2019). Design files and LabVIEW VIs for the IceDrone. doi: 10.17632/jx7b5t4f6j.1. Available online at: https://data.mendeley.com/datasets/jx7b5t4f6j/1
Carlson, D. F., and Rysgaard, S. (2018). Adapting open-source drone autopilots for real-time iceberg observations. MethodsX 5, 1059–1072. doi: 10.1016/j.mex.2018.09.003
Cheng, H.-W., Chen, T.-L., and Tien, C.-H. (2019). Motion estimation by hybrid optical flow technology for UAV landing in an unvisited area. Sensors 19:1380. doi: 10.3390/s19061380
Crawford, A. J., Mueller, D., and Joyal, G. (2018). Surveying drifting icebergs and ice islands: deterioration detection and mass estimation with aerial photogrammetry and laser scanning. Rem. Sens. 10:575. doi: 10.3390/rs10040575
Death, R., Siegert, M. J., Bigg, G. R., and R.Wadley, M. (2006). Modelling iceberg trajectories, sedimentation rates and meltwater input to the ocean from the Eurasian Ice Sheet at the Last Glacial Maximum. Palaeogeogr. Palaeoclimatol. Palaeoecol. 236, 135–150. doi: 10.1016/j.palaeo.2005.11.040
Diemand, D. (1984). Iceberg temperatures in the North Atlantic-theoretical and measured. Cold Regions Sci. Technol. 9, 171–178.
Druckenmiller, M., Eicken, H., Johnson, M., Pringle, D., and Williams, C. (2009). Toward an integrated coastal sea-ice observatory: System components and a case study at Barrow, Alaska. Cold Regions Sci. Technol. 56, 61–72. doi: 10.1016/j.coldregions.2008.12.003
Duprat, L. P. A. M., Bigg, G. R., and Wilton, D. J. (2016). Enhanced Southern Ocean marine productivity due to fertilization by giant icebergs. Nat. Geosci. 9, 219–221. doi: 10.1038/ngeo2633
Eicken, H., Gradinger, R., Salganek, M., Shirasawa, K., Perovich, D., and Leppäranta, M. (2009). Field Techniques in Sea Ice Research. University of Alaska Press. Available online at: https://epic.awi.de/id/eprint/40808/
Forster, C., Faessler, M., Fontana, F., Werlberger, M., and Scaramuzza, D. (2015). “Continuous on-board monocular-vision-based elevation mapping applied to autonomous landing of micro aerial vehicles,” in 2015 IEEE International Conference on Robotics and Automation (ICRA) (Seattle, WA), 111–118.
Gagnon, R., and Gammon, P. (1997). In situ thermal profiles and laboratory impact experiments on iceberg ice. J. Glaciol. 43, 569–582.
Han, Y.-G., Yoo, S. H., and Kwon, O. (2017). Possibility of applying unmanned aerial vehicle (UAV) and mapping software for the monitoring of waterbirds and their habitats. J. Ecol. Environ. 41:21. doi: 10.1186/s41610-017-0040-5
Harada, N. (2016). Review: Potential catastrophic reduction of sea ice in the western Arctic Ocean: its impact on biogeochemical cycles and marine ecosystems. Glob. Planet. Change 136, 1–17. doi: 10.1016/j.gloplacha.2015.11.005
Hawkings, J., Wadham, J., Tranter, M., Telling, J., Bagshaw, E., Beaton, A., et al. (2016). The Greenland Ice Sheet as a hot spot of phosphorus weathering and export in the Arctic. Glob. Biogeochem. Cycles 30, 191–210. doi: 10.1002/2015GB005237
Hawkings, J. R., Benning, L. G., Raiswell, R., Kaulich, B., Araki, T., Abyaneh, M., et al. (2018). Biolabile ferrous iron bearing nanoparticles in glacial sediments. Earth Planet. Sci. Lett. 493, 92–101. doi: 10.1016/j.epsl.2018.04.022
Heller, V., Chen, F., Brühl, M., Gabl, R., Chen, X., Wolters, G., et al. (2019). Large-scale experiments into the tsunamigenic potential of different iceberg calving mechanisms. Sci. Rep. 9:861. doi: 10.1038/s41598-018-36634-3
Hopwood, M., Cantoni, C., Clarke, J., Cozzi, S., and Achterberg, E. (2017). The heterogeneous nature of Fe delivery from melting icebergs. Geochem. Perspect. Lett. 3, 200–209. doi: 10.7185/geochemlet.1723
Hopwood, M., Carroll, D., Dunse, T., Hodson, A., Holding, J., Iriarte, J., et al. (2019). Review article: how does glacier discharge affect marine biogeochemistry and primary production in the Arctic? Cryosphere Discuss. doi: 10.5194/tc-2019-136. [Epub ahead of print].
Hopwood, M. J., Connelly, D. P., Arendt, K. E., Juul-Pedersen, T., Stinchcombe, M. C., Meire, L., et al. (2016). Seasonal changes in Fe along a glaciated Greenlandic fjord. Front. Earth Sci. 4:15. doi: 10.3389/feart.2016.00015
Jones, D., and Gudmundsson, G. (2015). Tracking B-31 iceberg with two aircraft-deployed sensors. Nat. Hazards Earth Syst. Sci. 15, 1243–1250. doi: 10.5194/nhess-15-1243-2015
Jouvet, G., van Dongen, E., Lüthi, M., and Vieli, A. (2019). In-situ measurements of the ice flow motion at Eqip Sermia glacier using a remotely controlled UAV. Geosci. Instr. Methods Data Syst. doi: 10.5194/gi-2019-6. [Epub ahead of print].
Koroleff, F. (1983). “Determination of nutrients,” in Methods of Seawater Analysis, eds K. Grasshoff, M. Erhardt, and K. Kremling (Verlag Chemie), 125–187.
Laliberté, F., Howell, S., and Kushner, P. (2016). Regional variability of a projected sea ice-free Arctic during the summer months. Geophys. Res. Lett. 43, 256–263. doi: 10.1002/2015GL066855
Larsen, P.-H., Overgaard Hansen, M., Buus-Hinkler, J., Harnvig Krane, K., and Sønderskov, C. (2015). Field tracking (GPS) of ten icebergs in eastern Baffin Bay, offshore Upernavik, northwest Greenland. J. Glaciol. 61, 421–437. doi: 10.3189/2015JoG14J216
Leu, E., Søreide, J., Hessen, D., Falk-Petersen, S., and Berge, J. (2011). Consequences of changing sea-ice cover for primary and secondary producers in the European Arctic shelf seas: timing, quantity, and quality. Prog. Oceanogr. 90, 18–32. doi: 10.1016/j.pocean.2011.02.004
Lever, J., Klein, K., Mitchell, D., and Diemand, D. (1991). Wave-induced iceberg motion. Cold Regions Sci. Technol. 20, 11–23.
Lewis, J., and Bennett, G. (1984). Monte Carlo calculations of iceberg draft changes caused by roll. Cold Regions Sci. Technol. 10, 1–10.
Li, B., Zhu, Y., Wang, Z., Li, C., Peng, Z.-R., and Ge, L. (2018). Use of multi-rotor unmanned aerial vehicles for radioactive source search. Rem. Sens. 10:728. doi: 10.3390/rs10050728
Lin, H., Rauschenberg, S., Hexel, C. R., Shaw, T. J., and Twining, B. S. (2011). Free-drifting icebergs as sources of iron to the Weddell Sea. Deep Sea Res. II 58, 1392–1406. doi: 10.1016/j.dsr2.2010.11.020
Lindsay, R., and Schwieger, A. (2015). Arctic sea ice thickness loss determined using subsurface, aircraft, and satellite observations. Cryosphere 9, 269–283. doi: 10.5194/tc-9-269-2015
Lund-Hansen, L., Hawes, I., Nielsen, M., and Sorrell, B. (2016). Is colonization of sea ice diatoms faciliated by increased surface roughness in growing ice crystals? Polar Biol. 40, 593–602. doi: 10.1007/s00300-016-1981-3
Lund-Hansen, L., Juul, T., Eskildsen, T., Hawes, I., Sorrell, B., Melvad, C., et al. (2018). A low-cost remotely operated vehicle (ROV) with an optical positioning system for under-ice measurements and sampling. Cold Regions Sci. Technol. 151, 148–155. doi: 10.1016/j.coldregions.2018.03.017
Macayeal, D. R., Abbot, D. S., and Sergienko, O. V. (2011). Iceberg-capsize tsunamigenesis. Ann. Glaciol. 52, 51–56. doi: 10.3189/172756411797252103
Meire, L., Meire, P., Struyf, E., Krawczyk, D. W., Arendt, K. E., Yde, J. C., et al. (2016). High export of dissolved silica from the Greenland Ice Sheet. Geophys. Res. Lett. 43, 9173–9182. doi: 10.1002/2016GL070191
Mugford, R. I., and Dowdeswell, J. A. (2010). Modeling iceberg-rafted sedimentation in high-latitude fjord environments. J. Geophys. Res. 115:F03024. doi: 10.1029/2009JF001564
Murphy, J., and Riley, J. (1962). A modified single solution method for the determination of phosphate in natural waters. Anal. Chim. Acta 26, 31–36.
Notz, D., and Stroeve, J. (2016). Observed Arctic sea-ice loss directly follows anthropogenic CO2 emission. Science 354, 747–750. doi: 10.1126/science.aag2345
Pestana, J., Maurer, M., Muschick, D., Hofer, M., and Fraundorfer, F. (2019). Overview obstacle maps for obstacle-aware navigation of autonomous drones. J. Field Robot. 36, 734–762. doi: 10.1002/rob.21863
Raiswell, R., Benning, L. G., Tranter, M., and Tulaczyk, S. (2008). Bioavailable iron in the Southern Ocean: the significance of the iceberg conveyor belt. Geochem. Trans. 9:7. doi: 10.1186/1467-4866-9-7
Raiswell, R., Hawkings, J. R., Benning, L. G., Baker, A. R., Death, R., Albani, S., et al. (2016). Potentially bioavailable iron delivery by iceberg-hosted sediments and atmospheric dust to the polar oceans. Biogeosciences 13, 3887–3900. doi: 10.5194/bg-13-3887-2016
Shaw, T., Raiswell, R., Hexel, C., Vu, H., Moore, W., Dudgeon, R., et al. (2011). Input, composition, and potential impact of terrigenous material from free-drifting icebergs in the Weddell Sea. Deep Sea Res. II 58, 1376–1383. doi: 10.1016/j.dsr2.2010.11.012
Smith, K. Jr., Sherman, A., Shaw, T., and Sprintall, J. (2013). Icebergs as unique Lagrangian ecosystems in polar seas. Annu. Rev. Mar. Sci. 5, 269–287. doi: 10.1146/annurev-marine-121211-172317
Smith, K. L. Jr., Robison, B. H., Helly, J. J., Kaufmann, R. S., Ruhl, H. A., Shaw, T. J., et al. (2007). Free-drifting icebergs: hot spots of chemical and biological enrichment in the Weddell Sea. Science 317, 478–482. doi: 10.1126/science.1142834
Smolyankskiy, N., and Gonzalez-Franco, M. (2017). Stereoscopic first person view system for drone navigation. Front. Robot. AI 4:11. doi: 10.3389/frobt.2017.00011
Stolz, B., Brödermann, T., Castiello, E., Englberger, G., Erne, D., Gasser, J., et al. (2018). “An adaptive landing gear for extending the operational range of helicopters,” in 2018 IEEE/RSJ International Conference on Intelligent Robots and Systems (IROS) (Madrid), 1757–1763.
Straneo, F., Sutherland, D., Stearns, L., Catania, G., Heimbach, P., Moon, T., et al. (2019). The case for a sustained Greenland Ice Sheet-Ocean Observing System (GrIOOS). Front. Mar. Sci. 6:138. doi: 10.3389/fmars.2019.00138
Stroeve, J., Serreze, M., Holland, M., Kay, J., Malanik, J., and Barret, A. (2012). The Arctic's rapidly shrinking sea ice cover: a research synthesis. Clim. Change 110, 1005–1027. doi: 10.1007/s10584-011-0101-1
Sulak, D. J., Sutherland, D. A., Enderlin, E. M., Stearns, L. A., and Hamilton, G. S. (2017). Iceberg properties and distributions in three Greenlandic fjords using satellite imagery. Ann. Glaciol. 58, 92–106. doi: 10.1017/aog.2017.5
Sutherland, D., Roth, G. E., Hamilton, G., Mernild, S., Stearns, L., and Straneo, F. (2014). Quantifying flow regimes in a Greenland glacial fjord using iceberg drifters. Geophys. Res. Lett. 41, 8411–8420. doi: 10.1002/2014GL062256
P. G. Talalay (ed.). (2016a). “Drilling challenges and perspectives for future development,” in Mechanical Ice Drilling Technology (Singapore: Springer Geophysics, Springer), 259–279.
P. G. Talalay (ed.). (2016b). “Yearly history of ice drilling from nineteeth to the first half of twentieth century,” in Mechanical Ice Drilling Technology (Singapore: Springer Geophysics, Springer), 9–13.
Wadham, J. L., Hawkings, J., Telling, J., Chandler, D., Alcock, J., O'Donnell, E., et al. (2016). Sources, cycling and export of nitrogen on the Greenland Ice Sheet. Biogeosciences 13, 6339–6352. doi: 10.5194/bg-13-6339-2016
Wagner, T. J., Wadhams, P., Bates, R., Elosegui, P., Stern, A., Vella, D., et al. (2014). The “footloose” mechanism: iceberg decay from hydrostatic stresses. Geophys. Res. Lett. 41, 5522–5529. doi: 10.1002/2014GL060832
Wood, E., Armstrong, F., and Rich, F. (1967). Determination of nitrate in seawater by cadmium-copper reduction to nitrate. J. Mar. Biol. Assoc. U.K. 47, 23–31.
Keywords: iceberg, sea ice, sediment, nutrients, iron, drone, Greenland, glacier
Citation: Carlson DF, Pasma J, Jacobsen ME, Hansen MH, Thomsen S, Lillethorup JP, Tirsgaard FS, Flytkjær A, Melvad C, Laufer K, Lund-Hansen LC, Meire L and Rysgaard S (2019) Retrieval of Ice Samples Using the Ice Drone. Front. Earth Sci. 7:287. doi: 10.3389/feart.2019.00287
Received: 11 July 2019; Accepted: 21 October 2019;
Published: 05 November 2019.
Edited by:
Alun Hubbard, Arctic University of Norway, NorwayReviewed by:
Denis Samyn, Royal Museum for Central Africa, BelgiumTom Holt, Aberystwyth University, United Kingdom
Copyright © 2019 Carlson, Pasma, Jacobsen, Hansen, Thomsen, Lillethorup, Tirsgaard, Flytkjær, Melvad, Laufer, Lund-Hansen, Meire and Rysgaard. This is an open-access article distributed under the terms of the Creative Commons Attribution License (CC BY). The use, distribution or reproduction in other forums is permitted, provided the original author(s) and the copyright owner(s) are credited and that the original publication in this journal is cited, in accordance with accepted academic practice. No use, distribution or reproduction is permitted which does not comply with these terms.
*Correspondence: Daniel F. Carlson, ZGFuZmNhcmxzb24mI3gwMDA0MDtnbWFpbC5jb20=