- 1CEN, University of Hamburg, Hamburg, Germany
- 2German Meteorological Service, Deutscher Wetterdienst (DWD), Hamburg, Germany
- 3Federal Maritime and Hydrographic Agency, Hamburg, Germany
The Baltic and North Seas Climatology (BNSC) presented here is a new climatology calculated solely from marine in situ observations. Created in cooperation between University of Hamburg (UHH), Federal Maritime and Hydrographic Agency [Bundesamt für Seeschifffahrt und Hydrographie (BSH)] and German Meteorological Service [Deutscher Wetterdienst (DWD)], the BNSC is an update of the KLIWAS (“Klimawandel und Wasserstraßen”) climatology for the North Sea and is extended to the Baltic Sea. A thorough quality control, the reduction of the temporal sampling error and spatial and temporal averaging were applied to the observations, yielding time series of gridded fields of atmospheric and hydrographic parameters in the region of the Baltic, the North Sea and adjacent regions of the North Atlantic. The atmospheric subset of the BNSC consists of time series of monthly mean gridded fields of 2 m air and dew point temperature and air pressure at sea level for the period 1950–2015 on a horizontal 1° × 1° grid. Climatological fields are provided as well. The hydrographic part of the BNSC comprises the variables water temperature and salinity on 105 depth levels for the time interval 1873–2015. The grid boxes' edge length is 0.25° in both zonal and meridional direction. Monthly and annual mean fields are provided as well as decadal monthly mean fields. To create homogenous fields, the method of objective analysis was applied to the fields of decadal means. Furthermore, an extensive sensitivity study was carried out to assess the sensitivity of the data product to the amount of observational data. The BNSC introduced here is compared to several different data products: three reanalyses (ERA-Interim, ERA-40 and COSMO-REA6), the corresponding KLIWAS product and meteorological station data for the atmospheric part. The hydrographic subset is compared to the KLIWAS climatology, the BALTIC ATLAS and the Baltic Sea Physical Reanalysis Product. The BNSC data product allows studying of climate variability but also holds the chance to validate regional numerical climate simulations, which makes it a valuable reference data set. The BSNC is freely available via the website of University of Hamburg's Integrated Climate Data Center.
Introduction
In comparison to other oceanic regions, the Baltic and the North Sea is very well-sampled with respect to both marine and meteorological observations. Different platforms (voluntary observing ships [VOS], buoys (drifting and stationary), research vessels, fire ships etc.) contribute to the wealth of in situ observations that help to assess the conditions of the ocean and the atmosphere and to analyze changes in those two important components of the climate system. Numerical modeling studies in climate science aim at reproducing complex and dynamic processes in order to predict future climate change. Regional climate models, however, depend on observational data as a reference and validation, as was stated in the goals of both the KLIWAS project (Kofalk et al., 2010) and the project “Network of Experts” (both founded by the German Ministry of Transport and Digital Infrastructure). The KLIWAS project (Climate Water Navigation: Impacts of Climate Change on Waterways and Navigation) looked for the effects of climate change on navigation on coastal waterways and on coastal protection infrastructure by running regional climate models. In this project, a gridded climatological reference data set over the North Sea was required to evaluate the quality of hindcast runs of these models. Since there were no sufficient climatologies for the North Sea region in the form of a long term data set, such a reference data set based on in situ observations was created for meteorological and hydrographic parameters on a compatible grid: the KLIWAS North Sea Climatology [KNSC (Bersch et al., 2013, 2016) (hydrographic part) and (Sadikni et al., 2013, 2018) (atmospheric part)]. In order to serve as a validation reference, the input data for these data sets had to be homogenized with respect to quality as well as spatial and temporal dimensions.
Similar data products exist for the North and Baltic Sea: The first to mention that includes atmosphere is Korevaar (1990), a climatology of the North Sea for the time period 1961–1980 based on observations from ships and lightvessels, that was best at that time. The atmospheric North Sea climatology for 1981–1990 by Michaelsen et al. (1998) could be seen not only as an update of the previous, but as well as a precursor of the KNSC, as it, too, was produced in a cooperation between the DWD and the University of Hamburg. Janssen et al. (1999) created a hydrographic climatology of monthly mean fields for both marginal seas based on observational data from 1900 to 1996; (Feistel et al., 2008) provide a time series of monthly mean fields of various marine and atmospheric parameters up to 2005 for the Baltic Sea. A similar data product, but solely focussed on hydrographic parameters, was produced by Núñez-Riboni and Akimova (2015). But in contrast to the BNSC data product presented in this paper, these products do not cover the entire North and Baltic Seas for meteorological and hydrographic parameters on a compatible grid.
Comparison between the atmospheric KNSC data product and the output of regional climate models was performed by Bülow et al. (2014). The hydrographic part of KNSC was used in an intercomparison study between various ocean models in Pätsch et al. (2017). A comprehensive assessment of the atmospheric KNSC and global reanalysis products was done by Schade et al. (2018). Here, the global reanalyses NCEP-1 (Kalnay et al., 1996), ERA-40 (Uppala et al., 2005), ERA-Interim (Dee et al., 2011), MERRA (Rienecker et al., 2011), and 20CRv2 (Compo et al., 2011) were used.
The idea of the data product presented here is to pursue the concept of a combined meteorological-hydrographical data product realized in the KLIWAS project by creating the KNSC data product, to update the climatologies for the ocean and the atmosphere with recent observational data and to extend the data product to the Baltic Sea.
The data and methods applied in this work are described in section Data and Methods. The sources of data of the atmospheric part of the BNSC (hereafter referred to as BNSCatm) and changes in the creation procedure of the BNSCatm data product with respect to the creation of KNSC are briefly described in section Atmospheric BNSC.
The data and methods applied for the calculation of the hydrographic BNSC data product (hereafter referred to as BNSChydr) are elaborately described in section Hydrographic BNSC.
In contrast to the KNSC data product, a sensitivity analysis is applied to the BNSC data products which assesses the sensitivity of the data product with respect to the chosen base of observational data. The description of this study can be found in section Hydrographic BNSC.
Additionally, both BNSC data products are compared to several other data products. A description of the data used and the methods applied is provided in section Comparison With Other Data Products.
The description of the data products themselves (BNSCatm and BNSChydr), the outcome of the sensitivity study and the comparison with the other data products is presented in section Results. It is followed by a summary and an outlook (section Summary and Outlook). The data availability and description of the data files and the provided variables are listed in section Data Availability and Description.
Data and Methods
Overview
The BNSC data product bases on marine observational data in the longitudinal range of 15°W to 31°E. The lower limit of the latitudinal range is set to 47°N; the upper limit is 66°N for the Baltic and 65°N for the North Sea region. The data product consists of temporally averaged fields of the following atmospheric and hydrographic parameters:
• sea level air pressure (SLP)
• air temperature at 2 m height (AT)
• dew point temperature at 2 m height (DP)
• sea water temperature (T)
• sea water salinity (S)
The latter two are available as 3-D fields.
The box size of the horizontal grids is set to:
• 1° × 1° for BNSCatm.
• 0.25° × 0.25° for BNSChydr
The different temporal resolutions and periods are:
• for BNSCatm, monthly and climatological monthly for 1950–2015
• for BNSChydr, monthly, annual and decadal monthly for 1873–2015.
Atmospheric BNSC
Sources of Data
The atmospheric part of this climatology (BNSCatm) is produced with marine in situ observations originating from the Marine Data Center of the DWD, which maintains an extensive climatological archive of national and international weather data gathered by vessels and buoys (see https://www.dwd.de/EN/ourservices/marine_data_center/maritimesdatenzentrum.html, last access May 14th 2019). The data collection of this archive started in the middle of the nineteenth century and is ongoing. Most of these observations come from ships and buoys and are recorded using a variety of methods, including manual observations at specific times as well as automated transmission of measurements that were taken with a high frequency, which is described in more detail by Schade et al. (2013) and Sadikni et al. (2018). All data are checked using the DWD high quality control (HQC) procedure to ensure the maximum degree of reliability before they are added to the archive. These procedures do not only check the individual observation but also implement checks on a sequence of observations from a specific observation platform in order to identify data errors of location and their time series consistency, as is described by Sadikni et al. (2018) in their section 2.a. Even though parts of the data that are included in this archive are a subset of ICOADS, checking all data in the same way with the same method was the main argument to use it for this study. Only data with flags “C” (climatologically right), “D” (analysis consistent), “E” (temporally consistent), “F” (internally consistent), “G” (spatially consistent, or “H” (manually consistent) are used in this study. This leads to about 31 million quality-controlled sets of meteorological in situ observations in the BNSC region for the period January 1950 to December 2015. Due to a relatively small amount of available data in the years before 1950, the period starts later than for the hydrographical part. The data used here can be considered as atmospheric observations near sea level at meteorological standard heights, as is sea level for air pressure and 2 m for the air and dew point temperatures.
Data Processing
For the calculation of the climatology, it has to be taken into account, that the observational data are not equally distributed in space or time. Therefore, measures have been taken to homogenize the data. Since the creation of the atmospheric part of the KNSC and the atmospheric BNSC data product do not differ substantially, the reader is referred to the detailed description in Sadikni et al. (2018).
Only observations at standard observation times (0, 6, 12, and 18 UTC) are used, like it was done for the KNSC climatology in order to reduce possible sampling biases caused by the high frequency of observations by automated measurements. The selected quality controlled observations are checked for duplicates, which is an additional test compared to the precursor KNSC.
In the data, there are fewer observations for the dew point temperature than for the other two parameters. The number of observations was increased by calculating dew point temperature from air temperature and relative humidity, unless it was directly measured. This method adds several hundred values to dew point temperature data.
The further procedure is carried out in nearly the same way as described by Sadikni et al. (2018). In the first step, the data is sorted into grid boxes of one degree edge length for each month of each year, to obtain a time series of monthly means. The 1° × 1° spatial resolution is coarser than that of the hydrographic part, but is chosen due to the low data density to get statistically meaningful results for each grid box.
The air and dew point temperature have distinct diurnal and annual cycles, and therefore a sampling error can occur due to an unbalanced temporal data distribution. Corrections for the hours of the day, and as well the day of a month were calculated to shift the values to the middle of the day and month, respectively. As this is done on a monthly basis, still the annual cycle is kept in the climatology. See Sadikni et al. (2018) for a detailed description of this process. In the first step, for the diurnal cycle correction terms, they were calculated for each month and grid cell as difference between the long-term mean and the 6 h means. A more complex approach was used in the second step for the annual cycle. For each month, the differences between the long-term monthly mean and the daily means were calculated and afterwards, the results for the month were fitted with a polynomial of second order. The difference between the result of the polynomial on a day and the long-term-mean of the month was used as correction term. These corrections were applied to the observations to reduce errors due to the diurnal and annual cycles.
Since the air pressure observations do not show a pronounced mean annual cycle, especially in the northern region, and no diurnal cycle, a different method is applied: The number of days within a month without pressure data must not exceed 14 consecutive days to ensure an even data distribution in time. This criterion differs from the previous version, described by Sadikni et al. (2018), in which data averages over 6 day windows were used, where 4 of 5 windows had to be covered with data, which means a maximum possible data gap of 16 days. In the KNSC, the averages of the 6 day windows were used for monthly mean values, resulting in temporally strongly smoothed results, and in small standard deviations, compared to reanalysis data for example. This is the reason for this new approach for temporal correction of air pressure data.
In the next step, it is ensured that, if possible, there is a sufficient number of values per grid box for averaging. For this purpose, a threshold for the number of observations per grid point is set for each parameter. This threshold is 20 observations for the temperatures and 500 observations for the pressure, which are the same values as in the KNSC (Sadikni et al., 2018). In the first step, if the number of observations in the grid box is below this threshold value, the observations of the eight surrounding grid boxes are added to the average. If the number of observations is still smaller than the threshold, then, in the second step, the data of the next 16 environment boxes are added for the averaging. Thus, observations of up to 24 surrounding grid boxes can contribute to the result of the center grid box. The results of these calculations are time series of monthly mean fields of the parameters on the one-degree grid.
From these monthly mean fields, the BNSC climatology is calculated for the 30-year periods 1951–1980, 1961–1990, 1971–2000, and 1981–2010. For this, the mean values of the fields for each month of the respective period are computed. A grid box gets no value if it had no value in 1/6 of the time, which is 6 or more months. For the longtime climatology 1950–2015, due to the long time series of 66 years, the criterion is slightly less strict and needs a value in at least 4/5 of the time steps.
Hydrographic BNSC
Sources of Data
Observed temperature and salinity profiles from different data sources are the base of the BNSC data product. The sources of observational data are:
• WOD (World Ocean Data Center, Boyer et al., 2013)
• DOD (Deutsches Ozeanografisches Datenzentrum, Germany, www.bsh.de)
• IOW (Leibniz Institute for Baltic Sea Research Warnemünde, Germany,
• ICES (International Council for Exploration of the Sea, www.ices.dk)
• IMGW-PIB (The Institute of Meteorology and Water Management – National Research Institute, Poland, www.imgw.pl)
• NIOZ (Royal Netherlands Institute for Sea Research, www.nioz.nl)
• BODC (British Oceanographic Data Center, www.bodc.ac.uk)
• SCANFISH (SCANfish data, provided by BSH, www.bsh.de)
• CTD_DK (Danish National Marine Monitoring Data, Bioscience, Aarhus University - http://Mads.dmu.dk)
• ARGO (International ARGO-Project, www.ifremer.fr).
The authors do not claim the list of sources of data to be complete. By the time starting working on BNSChydr, the data sources listed above were the ones the authors were aware of. Considering the creation of marine climatologies a process, future versions may not only include future observational data but also data sources not included yet, as, for example, the Baltic Environmental Database (BED, http://nest.su.se/bed/), the Copernicus Marine Environment Monitoring Service, the SeaDataNet program and the database compiled within the international project “The Year of the Gulf of Finland 2014” (see “The Gulf of Finland assessment., 2016” in the reference list for more details).However, the expansion to more data sources has always to be considered with respect to the cost-value ratio: there is already a strong overlap between some of the above listed data sources. Janssen et al. (1999) also found a great intersection between the ICES/DOD data bases (which they chose for their data product) and other data sources. Thus, new data sources might contribute some observations not included yet but, at the same time, each new data source also adds workload to the data processing procedure which is explained in the following.
Data Processing
The observational data are processed in several consecutive steps described in detail in the following.
Duplicate Profiles
Incorporation of several data sources in the research of observational data includes intersection of different sources of data, e.g., profiles contained in the WOD data base are also provided by ICES. Duplicate profiles, however, would bias the result of the BNSC. Therefore, it is necessary to exclude the duplicate profiles in the further data processing. The numbers of remaining profiles after identification of duplicates are listed in Table 1, sorted by the different data sources.
To get an overview of the distribution of the observations, a time series (1873–2015) of the frequency of observed profiles per year is displayed in Figure 1A. In the period between 1873 and 1882, there are only 1–6 profiles recorded in the whole area. In general, the number of observations is rather low until approximately 1950. The periods of the First and Second World War can clearly be recognized by depressions in the observational frequency. From 1950 on, the observation rate moderately rises to a maximum in the 1990s. For the year 1989, the number of profiles exceeds the value of 30,000 profiles and sticks out. Closer analysis revealed, that most of the profiles in this year can be found in only five 0.25° × 0.25° boxes close to the German North and Baltic Sea coast.
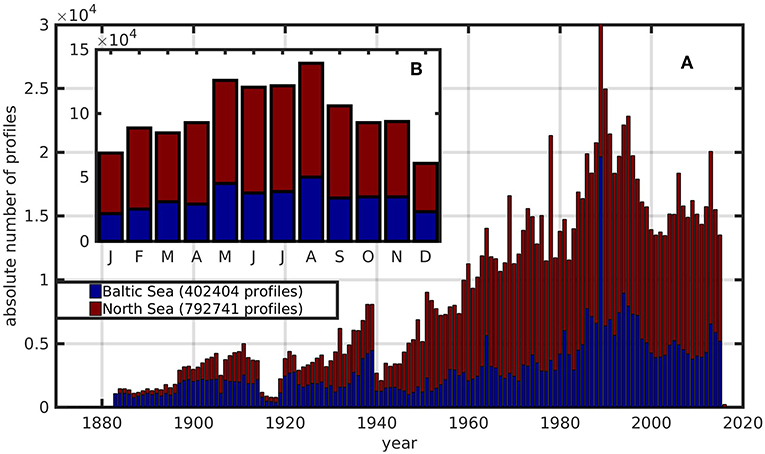
Figure 1. Annual (A) and monthly (B) frequency of marine observational profiles for the BNSC area of the North and Baltic Sea (1873–2015).
Additionally, Figure 1B shows the distribution of the observed profiles in the 12 months of the year. It can clearly be seen that the observational frequency is higher in the summer than in the winter months. In Figure 2, this distribution is horizontally resolved; a distinctly higher data density can be seen in the months May-September than in the rest of the year. This is most obvious in the area of the Baltic and the central North Sea. A rather low number of observations characterizes the outer regions of the BNSC area. With respect to the data coverage as a function of depth (not shown) it can be stated, that the data density is highest at the surface and that a low data density characterizes the deep areas of the BNSC region (i.e., especially the North Atlantic).
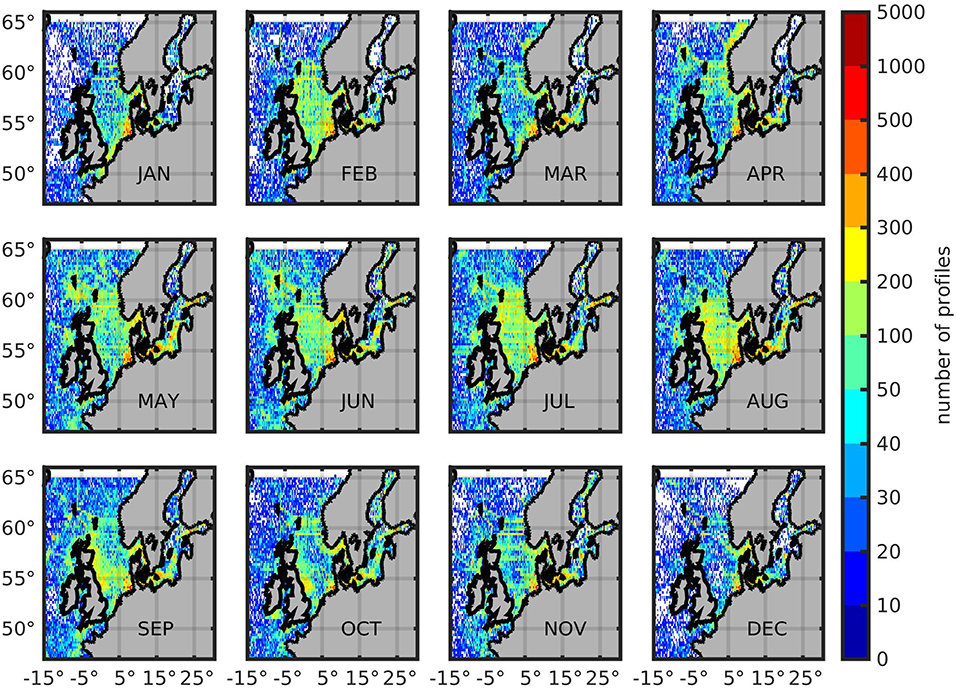
Figure 2. Absolute monthly frequency of marine profiles, 1873–2015, box size 0.25° × 0.25°, ∑ = 1,194,475 profiles.
Quality Control
Erroneous observations have to be filtered out during the creation of the climatology. Here, we follow the data quality control strategy implemented for the automated quality control of the global hydrographic archive for the compilation of the WOCE-Argo global hydrographic climatology (Gouretski, 2018). The number of profiles in the BNSC region (after sorting out duplicates) accounts to more than 1 million and reveals that a check of all these observations with respect to their quality is only possible with an automatic procedure.
The quality control consists of eight different single checks described in the following.
1. Crude range check
In general: Temperature and salinity in the North and Baltic Sea have a characteristic range. Temperature values below −2°C and above 25°C and salinity values above 38 PSU can certainly be regarded as erroneous for the regarded area. Those values are flagged by this check.
Depth dependent: Additionally, this check is based on the frequency distributions of temperature and salinity as a function of depth, and based on this, defines characteristic value ranges for single depth levels. The purpose of this check is to reject crude outliers, which passed the overall range check for temperature and salinity.
2. Maximum observed depth
Each instrument or station type has a characteristic maximum depth level to which observations are recorded. If this value is exceeded in a profile, all values are flagged. Information about the instrument or station type, if available, is stored in the meta data.
3. Constant value
If a profile shows a certain number (N) of consecutive constant values in a defined depth interval, those values will be flagged. The number N depends on the instrument type.
4. Spikes
Spikes are an unnatural change of the parameter value from one depth level to another and therefore have to be flagged. For this purpose, parameter-specific threshold values defining a spike are taken into account as well as the distance between the consecutive depth levels regarded for this check.
5. Vertical gradient
Ranges for the vertical parameter gradient are defined. For this purpose, corresponding frequency distributions are created based on the observational data and ranges for the vertical gradient are defined at a set of levels. For neighboring depth levels with observed parameter values, a vertical gradient can be calculated. In case this lies outside the vertical gradient range for this depth level, both observations are flagged.
6. Number of extrema
The procedure aims at checking the shape of the vertical profile. The number of local extrema (for T and S separately) is found for each observed profile. If the number of identified extrema is more than three, all observed values for this profile are flagged. The extrema are taken into account only if the difference between the parameter values at the neighbor minimum and maximum points exceed predefined tolerances. The tolerances for these differences do not depend on depth and are set to 0.5°C and 0.5 PSU for temperature and salinity.
7. Comparison with digital bathymetry
For this check the digital bathymetry GEBCO2 (Weatherall et al., 2015) is used. If the digital bathymetry indicates solely “land” near the hydrographic station, it is assumed that the position of that hydrographic station is erroneous and all observed values of the profile are flagged. If the digital bathymetry indicates the presence of “sea” points in a certain vicinity of the profile position, the last depth level is compared to the maximum bottom depth among the “sea” points. In case the last observed depth level is deeper than the bottom depth (including a tolerance value), all observed values below the “digital bottom” are flagged.
8. Local climatology
This check tests if the observed parameter values are in a local climatological range. The climatological median value is determined regionally based on the observations that are not flagged by any of the previous quality checks. The median value and the acceptable value range are derived from the frequency distribution of the parameter values. It has to be stressed that the skewness of the frequency distribution is taken into account when defining the parameter range, so that for example in regions of strong horizontal gradients (for example salinity in estuaries) not too many true high, respectively, low values are flagged, see Vandervieren and Hubert (2004). The local parameter range is defined as follows:
where Q1 and Q3 define the location of the first and third quartile, respectively, of the frequency distribution. Consequently, the difference Q3 − Q1 corresponds to the interquartile range. MC, the “medcouple”-value, is a measure for the skewness of the distribution. For more details on this, see Brys et al. (2003).
The quality control is performed separately, on the one hand, for the parameters salinity and temperature and on the other hand, since the North and Baltic Sea differ strongly in their hydrographic characteristics, for the two regions. The longitudinal segregation line is set at 55.8°N in the transition between Kattegat and Belt Sea and splits the entity of observational profiles into 792,741 for the North and 402,404 for the Baltic Sea.
Figure 3 shows the results of the quality control: the fraction of profiles flagged by the quality control is shown here in spatially horizontal resolution. The numerically summarized results of the quality control for the two regions and parameters are given in Table 2.
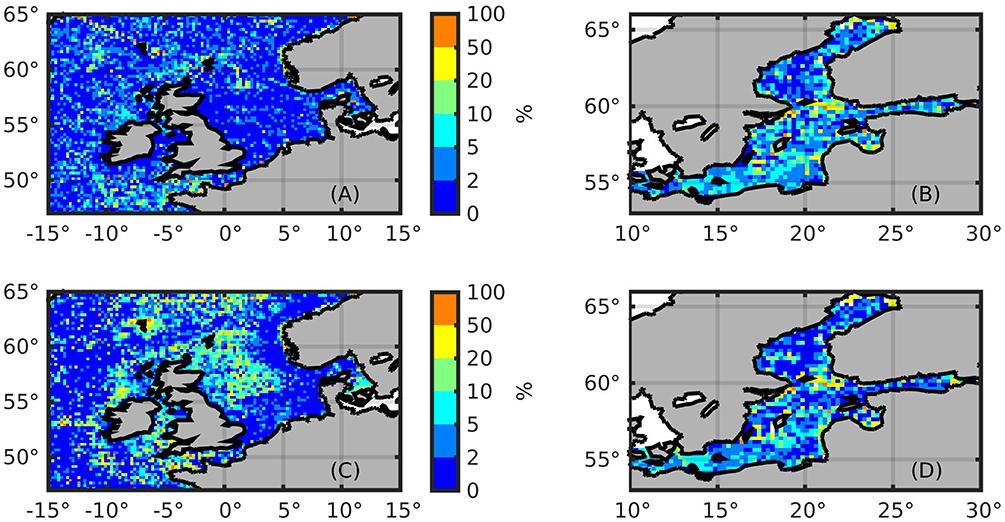
Figure 3. Results of the quality control for water temperature [North Sea (A), Baltic Sea (B)] and salinity [(North Sea) (C), (Baltic Sea) (D)]: spatial distribution of percentage of flagged profiles, box size 0.25° × 0.25°.
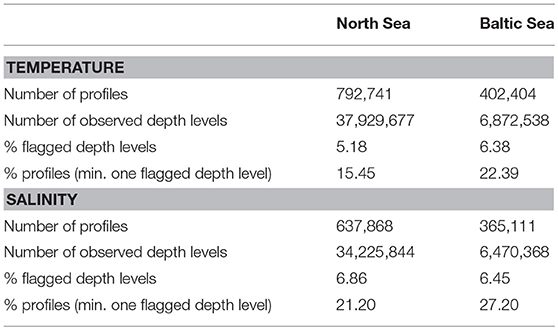
Table 2. Results of the quality control for the North and the Baltic Sea and the two parameters temperature and salinity: summary.
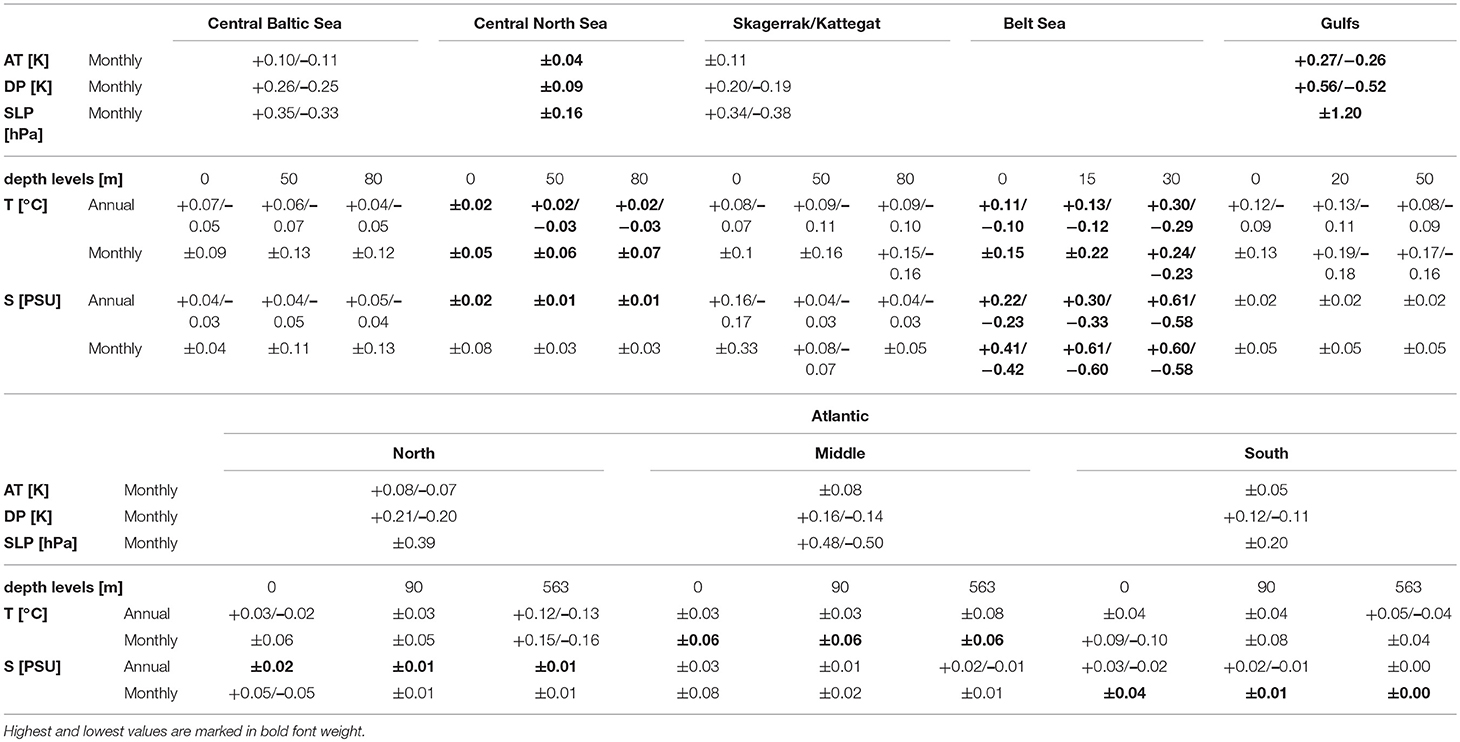
Table 3. Results of the sensitivity study: temporal mean differences between the spatial mean of the reference run (full data collection) and the upper (positive) and lower (negative) envelope formed by the spatial mean of the 100 runs with 90% randomly chosen observations.
Interpolation on Standard Depth Levels and Horizontal Grid
The temperature and salinity observations, as provided by the different data sources, are on observed depth levels. To allow a further processing, a spatially vertical interpolation on common standard depth levels is necessary. The distribution of those depth levels is determined as follows: in the upper region of the North and Baltic Sea: to a depth of 50 m, the difference between the depth levels is chosen to be 5 m. Further down, the difference between the two consecutive levels increases steadily by 1 m. Altogether, there are 105 different depth levels from 0 m to almost 5,000 m depth; the maximum distance (between the last and second last depth levels) accounts for 99 m.
The interpolation is performed following the procedure described by Reiniger and Ross (1968).
The applied horizontal grid has an edge length of 0.25° both in meridional and in zonal direction.
Correction of the Temporal Sampling Error
The observational data are available in temporal irregular distribution, see also inset in Figure 1B. Simple temporal averages of those observations are biased by periods with a high data density. Therefore, the irregular temporal distribution of the observations has to be taken into account and the observations must be adjusted accordingly before averaging. With respect to the annual mean, a single observation of parameter P, Pobs, can be described as the sum of three terms:
where Plt denotes the long-term mean, Pinterann stands for the part corresponding to the interannual variability and finally, Pseas expresses the seasonally variable fraction. It is this last term in combination with the temporal irregular sampling of the observations that would lead to the above mentioned bias if a simple arithmetic mean was applied to the original observations in order to receive an annual mean. The solution for this problem is to eliminate the influence of the seasonal variability on the observations and subtract the last term Pseas from Pobs, This allows the calculation of unbiased annual averages independent on the temporal distribution and number of observations. The adjustment is performed on the basis of a depth dependent long-term mean annual cycle that is derived from the observational data for each grid box separately. The long-term mean annual cycle is expressed as a polynomial (11th order) that is fitted to the observations in a certain area including the respective grid box b. This daily resolved fit for temperature (T) and salinity (S) observations is given in the following two equations.
The annual cycle does not have to be considered on all depth levels, but only in the upper layers with a distinct seasonal signal. For the creation of the BNSC, an adjustment is performed down to a depth of 200 m. In preparation of creation of the time series of annual mean values (am), the procedure is applied as follows: daily anomalies of the long-term mean annual cycle with respect to the long-term annual mean are calculated based on the polynomial fit and are referred to as the adjustment terms; in the following shown exemplarily for temperature T:
These adjustment terms form a set of 365 values. Each single observed value has a corresponding adjustment term, depending on the calendar day of the observation. The adjustment term is subtracted from the observed value.
In case of the monthly mean values, it is not the seasonal variability that could lead to a bias, but the intra monthly variability. Consequently, ly, the correction is applied to the observed values in preparation of creation of the time series of monthly mean values (mm) as follows: the long-term mean annual cycle is split into 12 sections, according to the months of a year. For each single section, a long-term monthly mean value is estimated and the corresponding daily anomalies are calculated, yielding for each month m an individual set of adjustment terms, exemplarily shown here for temperature T:
In contrast to the adjustment term for the creation of the annual mean, only the days corresponding to the respective month m are considered here, denoted by dm. Then, the adjustment term corresponding to the calendar day of observation is subtracted from the observed value.
Creation of Mean Values
Temporal mean averages are composed calculating the arithmetic mean of the corrected observational data in each box. Monthly and annual mean values are calculated. It has to be stressed, that boxes lacking observations are left empty.
Horizontally Interpolated Fields
Based on the fields of box averages (monthly and annual mean values), horizontally interpolated fields are composed, aiming at closing the gaps between populated grid boxes. The applied procedure is the method of optimal interpolation (also known as optimal analysis). It was introduced by Gandin (1965) and since then has been widely used in different hydro-meteorological applications, for instance for the World Ocean Circulation Experiment Climatology (Gouretski and Koltermann, 2004). A vast literature exists about the usage of the optimal interpolation, but we leave this beyond the scope to this paper and only crudely outline the optimal interpolation method below.
In this method, for the arbitrary point (o) the interpolated parameter value Fo is represented as the sum of the parameter first guess value, Go, and the weighted sum of the parameter deviations from the first guess at N observation locations (i):
The optimal weights wo are defined by the spatial correlation structure of the analyzed field. Generally, the optimal interpolation is preferred when the true correlation function can be accurately estimated; otherwise, other methods can provide comparable results. In many applications, the isotropic Gaussian (bell shaped) correlation function C (r) is used with the e-folding correlation length scale:
where r denotes the horizontal spatial distance and R being the correlation length scale.
As noted by Sokolov and Rintoul (1999), the intrinsic correlation length scale for the optimal interpolation will be dictated more by the size of the data-void region than by the actual estimate.
The BNSC region is characterized by strong variations in data density with the central part (central North Sea and Skagerrak, Kattegat, Belt Sea) being much better sampled than the adjacent Atlantic regions and the Gulfs of the Baltic Sea. As a trade-off, we used the e-folding correlation scale of 166 km in all our calculations. The interpolated fields produced by the optimal interpolation procedure may be considered as the result of applying a filter to the data. The optimal interpolation produces a spatial average of the data where smoothing length scales are in dependent on the data configuration, with the small scale oscillations being filtered uniformly, resulting in interpolated fields with homogeneous statistics. In data-poor regions, the optimal interpolation relaxes to the first-guess field.
It needs to be taken into account that the interpolation errors are higher for the data poor time periods. Especially in the starting years of the BNSC time series, the spatial coverage is very low, however. The same refers to greater depths. In the following, the number of populated boxes on each depth level is analyzed and set into relation to the maximum number of possibly populated (“wet”) boxes. The maximum coverage accounts to a little more than 14%; large areas in time and depth, however, show values of 5% and less. Based on this analysis and taking into account the frequency of observations as a function of time (see Figure 1), it was chosen to perform interpolation on all depth levels in monthly resolution for the period 1950–2015. Additionally, the spatial coverage can be improved when box-averaged fields for wider time-windows (e.g., several years) are used. For a time window of 10 years from 1955 on, the maximum value of horizontal coverage improves to more than 50% and large areas show more than 20% horizontal coverage. Still, the coverage in the deeper layers remains rather poor. A monthly resolution is applied to the standard depth levels of up to 101 m. For greater depths, the annual mean is applied.
To summarize:
• Before 1950, the spatial coverage is not sufficient to produce reasonable interpolated fields.
• The merging of box averages in a time window of 10 years yields a satisfying spatial coverage. For this reason, the interpolated fields are created based on decadal mean monthly and annual mean values of the box averages. The following decades are chosen for this:
• 1956–1965,
• 1966–1975,
• 1976–1985,
• 1986–1995,
• 1996–2005,
• 2006–2015.
The seasonal signal only reaches to a certain depth. For the BNSC, the standard depth level of 101 m marks the depth to which decadal monthly box averages are taken as a basis; for greater depths, the decadal annual mean is applied.
Sensitivity Analysis
The BNSC box averages, i.e., the core of the data product, is sensitive to the available observations. A lack of some observations might alter the data product as well as additional observations becoming available in future. To estimate the influence of the observational basis on the data product, a sensitivity analysis is performed.
The sensitivity analysis is applied to all parameters for the time periods 1950–2015 and 1960–2015 for the atmospheric and the hydrographic part, respectively.
The analysis works with fields of anomalies as deviations from fields of long-term mean values. Based on the box averages of the atmospheric and the hydrographic data product, long-term climatological monthly mean values are calculated by temporally averaging over the available box values in the complete respective period. As a reference for the sensitivity analysis, the anomaly fields are then calculated as the difference between the long-term mean fields and fields of box averages of the BNSC product. Additionally, anomaly fields are calculated that are not based on the entity but on only 90% of the BNSC observations. The reduction of observations is applied randomly and repeated 100 times. In the following, each 10%-reduction and the subsequent box averaging is referred to as a “run.”
Furthermore, the BNSC region is divided into eight sub-regions:
• the Atlantic part of the BNSC is divided into a
• northern (north of 60°N),
• a southern (south of 53°N) and
• a middle part;
• the central North Sea,
• the Skagerrak/Kattegat area (east of 8°E and north of 56°N),
• the Belt Sea
• the central Baltic Sea (east of 13°E and south of 59°N, including the Gulf of Riga),
• and finally the two greater gulfs of the Baltic Sea (north of 59°N).
It has to be mentioned that the Skagerrak/Kattegat and the Belt Sea area are regarded separately only for the hydrographic parameters; for the atmospheric variables, those two regions are treated as one.
For each of the above mentioned runs, the anomaly fields are averaged horizontally in each of these eight (seven) sub-regions. The same is applied to the anomaly fields of the reference. This yields time series for each run, which form a kind of “hose” around the time series of the reference, with an upper and a lower envelope.
The distance of the envelopes from the reference, however, critically depends on the number of runs that are performed. To illustrate that the chosen number of 100 runs is sufficient, the envelope-reference distances are investigated with respect to their dependency on the number of runs. The envelope as a function of the number of runs (not shown) reveals that both the distance to the lower and the upper envelope increases considerably from one to about 10 runs but experiences a rather low augmentation after the number of runs exceeds 60. With even more runs, the distances converge to a maximum. The respective negative maximum for the lower envelope and the positive maximum for the upper envelope define the measure for the minimum number of required runs: it is the number of runs corresponding to a value of 95% of the maximum of the respective envelope. For all the three atmospheric parameters in all seven regions it shows, that the minimum number of required runs lies between 70 and 80.
For the hydrographic parameters, envelopes exist around the time series of reference anomalies on each depth level and also here a clear convergence of the envelope distances can be seen for all regions, both parameters and the two different temporal resolutions. This confirms that the number of 100 runs is sufficient.
Comparison With Other Data Products
The BNSCatm is compared to different data products. These include the KNSC, three different reanalyses and station data. In addition, the BNSCatm was compared with available temperature data from Schmager et al. (2008), and with 30-year means 1981–2010 from ICOADS.
KNSCatm, Re-analyses and Station Data
The BNSC is compared to the previous project, the KNSC (Sadikni et al., 2018) to identify changes in the new version. The KNSC has the same spatial and temporal resolutions as BNSC, and covers the period from 1950 to 2010.
The sea level pressure is compared to three different reanalysis products. The first two re-analyses are ERA40 and ERA-Interim, both from ECMWF, and are analyzed for the overlapping time period 1979–2001. ERA-40 (Uppala et al., 2005) has a 125 km spatial resolution and the original data was uploaded in 6 h values. ERA-Interim (Berrisford et al., 2011; Dee et al., 2011) is the following project, which analyzes data from 1979 on with constant updates to the present. It has a spatial resolution of 79 km and a temporal resolution of 6 h. The climatologies of the variables temperature and sea level pressure of BNSCatm are also compared to ERA-Interim over the time period from 1981 to 2010. Additionally, the BNSC is compared to the COSMO-REA6 reanalysis (Bollmeyer et al., 2015) from the DWD and the University of Bonn. COSMO-REA6 was calculated for the time period 1995 to 2015 and has a spatial resolution of 6 km and an hourly temporal resolution. For the comparison, only monthly means are used. Before comparing the field, it is always interpolated onto the coarser grid.
The compared station data are time series of sea level pressure of 10 different stations along the German coast. The data was provided by the DWD (www.dwd.de/cdc). For comparison, the nearest grid cell of the ERA-40 reanalysis, respectively, the BNSCatm, is used.
In most comparisons, the monthly means are calculated and then a difference time series is created. Afterwards, these difference time series are averaged over the whole time period, single month or seasons. For the comparison of climatologies 1981–2010, a climatology for the same period is calculated from ERA Interim and this is monthly subtracted from the BNSCatm climatology.
14 Areas From Climatology of the Baltic Sea and ICOADS
The BNSCatm was compared to available air and dew point temperature data in the Climatology of the Baltic Sea based on the ICOADS-Data Set (1951–2000) by Schmager et al. (2008). They provide monthly means of air and dew point temperature for 14 areas in the Baltic Sea. For the same areas and period, means were calculated for each month from the monthly means of air and dew point temperature of the BNSCatm.
The hydrographic subset of the BNSC is compared to three data products:
• the precursor of the BNSC, the KNSCv2 data set for the North Sea region (Bersch et al., 2016)
• the BALTIC ATLAS (Feistel et al., 2008) for the Baltic Sea region.
(the latter two are based on in situ observations).
• the BALTIC SEA PHYSICAL REANALYSIS PRODUCT (Axell and Liu, 2016).
All three data products together with the applied comparison methods are described in the following.
KNSCv2
The precursor of the data product presented here, the KLIWAS North Sea climatology (KNSCv2, Bersch et al., 2016) is used for comparison with time series of box-averages of the BNSC data product. The KNSCv2 product comes on altogether 179 depth levels on a horizontal grid with a box length of 0.5° (zonal) and 0.25° (meridional). It covers the period 1873–2013. For the comparison, the BNSC box averages in both annual and monthly resolution are used. Concerning the spatial resolution, BNSC is adapted to KNSCv2 by horizontally averaging the two BNSC boxes corresponding to one KNSCv2 box. BNSC values are linearly interpolated on KNSCv2 depth levels. In case of spatial and temporal correspondence, absolute differences are computed.
BALTIC ATLAS
The time series of monthly mean values for the Baltic Sea (BALTIC: Monthly Time Series 1900–2005, Feistel et al., 2008) is used for the comparison with the monthly BNSC box averages of temperature and salinity. The BALTIC grid has an edge length of 1° and regularly spaced (10 m) depth levels. For the comparison, the BNSC product is horizontally averaged over the 16 boxes that correspond to one BALTIC box. Additionally, the BNSC values are linearly interpolated on the BALTIC depth levels. Absolute differences are then computed for every spatial and temporal correspondence between BALTIC and BNSC in the period 1900–2005.
Baltic Sea Physical Reanalysis Product
The BALTIC SEA PHYSICAL REANALYSIS PRODUCT, hereafter referred to as BSRA, provides, among others, temperature and salinity fields in monthly resolution. The horizontal grid has an edge length of 5 km. BSRA has 50 irregularly spaced depth levels with a distance of 4 m in the upper and a maximum distances of 40 m in the lower part of the water column. The period covered by the BSRA product is 1989–2015.
For the comparison, the BSRA boxes corresponding to one BNSC box are spatially averaged and subsequently linearly interpolated to the BNSC depth levels. Absolute differences between the two data products are then computed.
The BNSCatm data products are available from https://icdc.cen.uni-hamburg.de/1/daten/atmosphere/bnsc-met/
and should be cited as
Jahnke-Bornemann, Annika; Sadikni, Remon (2018). Baltic and North Sea Climatology meteorological part (Version 2.0). World Data Center for Climate (WDCC) at DKRZ. https://doi.org/10.1594/WDCC/BNSClim_meteo_v2
The BNSChydr data products are available from
https://icdc.cen.uni-hamburg.de/1/daten/ocean/bnsc/
and should be cited as
Hinrichs, Iris; Gouretski, Viktor (2018). Baltic and North Sea Climatology hydrographic part (Version 1.0). World Data Center for Climate (WDCC) at DKRZ. https://doi.org/10.1594/WDCC/BNSClim_hydro_v1.0
Free download as well as visual exploration of the data product is possible here.
After first submission of this manuscript, an update of the BNSChydr product has taken place. The authors recommend to use the update, which can be found under the same above given link. Until no new DOI has been assigned, the above given citation remains. All analysis presented in this paper are based on BNSChydr v1.0.
The spatial dimensions of the BNSC data products are described in Table 4.
The BNSC data product itself is complemented by information about the distribution of sea and land in the BNSC region in order to be able to distinguish between the two spheres.
For BNSChydr and BNSCatm, there are two NetCDF-files available with the same dimensions as described in Table 4. They consist of a binary field, which determines whether a grid box corresponds to water (value = 1) or land (value = 0). The distinction between water and land was made based on the highly spatially resolved GEBCO bathymetry (Weatherall et al., 2015) by estimating the fraction of GEBCO water boxes in one BNSC box. The threshold value for distinction wass set to 0.9 for BNSChydr and to 0.6 for BNSCatm for this purpose. This means that more than 10% (40%) for BNSChydr (BNSCatm) water fraction determine the BNSC box to be water. In addition, the land sea fraction for each grid box is also provided.
Table 5 lists the file names of the BNSC data product and gives an overview about the nomenclature of the file names in the individual data products.
Finally, Table 6 gives an overview and short explanation of the different variables stored in the .nc-files.
Results
As all results for the BNSC can be found under https://icdc.cen.uni-hamburg.de/projekte/bnsc-project/, we only show some chosen examples of the results.
BNSCatm
General Results
The longtime climatology 1950–2015, from which the months January and July are shown in Figure 4 for the three atmospheric parameters, exhibits an acceptable spatial coverage for all months, with poor data coverage just in the north of the North Sea, and the Gulf of Bothnia, as well as west of Ireland.
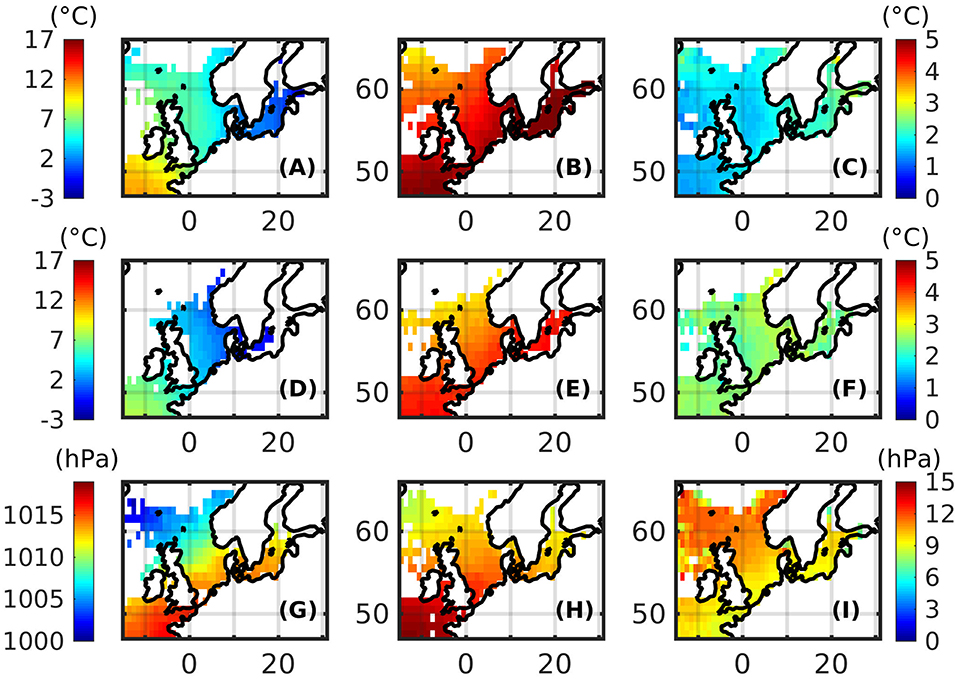
Figure 4. BNSCatm Climatology for 1950 to 2015: means of the parameters 2 meter air temperature (A,B), 2 m dew point temperature (D,E), and sea level pressure (G,H) for the months January (A,D,G) and July (B,E,H) and the mean standard deviation of each parameter over the whole period (C,F,I). Boxes with insufficient data coverage are white.
General climatological characteristics are well represented by the BNSC. The values in the air temperature and dew point temperature climatology range from −2 to19°C and −5 to 20°C, respectively. The results for January and July are shown in Figures 4A,B for mean air temperature and (D) and (E) for mean dew point temperature. As expected, the temperatures are higher in summer than in winter and have a tendency to decrease from south to north. The mean intra monthly standard deviations are smaller in summer than in winter and the values are in the range of 1 to 4 K for both parameters. Mean values of the intra monthly standard deviations over all months are shown for air temperature in Figure 4C and dew point in Figure 4F.
The values of the air pressure climatology range between 1000 and 1019 hPa and are higher in summer than in winter with a tendency to decrease from southeast to northwest. The results for mean air pressure in January and July are shown in Figures 4G,H. The standard deviation has higher values in winter than in summer and ranges between 6 and 16 hPa in the monthly data. It is smallest in the south of Great Britain and largest in the north west over the open water of the Atlantic Ocean. A mean of the standard deviations of the climatology product over all months is shown in Figure 4I.
The four 30-years climatologies (not shown) exhibit a temporal evolution of data distribution, where the largest data gaps exist in the 1951–1980 climatology for all parameters, and best spatial coverage has the climatology for the period 1961–1990. It can be suggested that the poorer data coverage in the early period is due to the setup of a monitoring network after the Second World War, whereas the gaps in later periods are due to changes in the measurement technologies, like replacements of old drifting buoys, less manual observations from ships, but an increasing number of automated observations. The dew point temperature has fewer observations than the other two parameters and therefore larger data gaps. The climatologies for all parameters show the expected behavior over the year.
Sensitivity Study
The sensitivity study yields regional fluctuation ranges caused by the random reduction of the basis of observations by 10%. As a result, the fluctuation ranges are listed in Table 3 (first three rows for BNSCatm) for the regions defined in section Sensitivity analysis. The values shown here are based on monthly mean fields and are the temporal mean differences between the spatial mean of the reference run (full data collection) and the upper and the lower envelope. For all three atmospheric BNSC parameters, the central North Sea exhibits the lowest and the region of the Gulfs of Finland and Bothnia the highest fluctuation ranges (marked with bold font weight in the table). This is most probably a direct consequence of the sampling density, which is very high in the central North Sea and rather low in the two greater gulfs of the Baltic Sea, also due to seasonal ice coverage.
In most of the seven regions of the sensitivity study, the fluctuation ranges of all parameters exhibit a temporal evolution (not shown). Except for the region of the two gulfs and the central Baltic Sea, the fluctuation ranges are significantly higher in the period 1950–1960 than from 1960 onward for the BNSCatm data product: The fluctuation ranges of the earlier period are about two to seven times the ranges of the later period. This is probably also caused by the poor data base in the earlier periods.
The climatological mean fields of BNSCatm are also subjected to this analysis, but the results are not listed in Table 3. For the 30-year climatological monthly mean fields the fluctuation ranges are in general of similar magnitude as the values for the time series of the monthly mean field. Similar to the findings for the time series of the monthly mean fields, a difference in the fluctuation ranges can be seen between the first 30-year-window (1951–1980) and the other three time periods. Lessons to be learned from the sensitivity analysis are that the data set of BNSCatm is more stable in the period from 1960 onward and in the later 30-year climatologies than before 1960 and in the first climatology from 1951 to 1980.
Comparison With KNSC
For the purpose of comparison, monthly averages of the KNSC period from 1950 to 2010 are subtracted from those of the BNSC, resulting in a difference time series.
For the mean air pressure, the largest differences between BNSC and KNSC seem to occur in areas and at times that have fewer observations than the surroundings. The differences in the extended winter months from December to March are in the interval −1.5 to 2 hPa. In the summer months, the deviations are slightly smaller in the interval −0.5 to 0.7 hPa. Due to the changes in the procedure, that were described in Data processing, the differences between BNSC and KNSC are quite large and the BNSC standard deviation is larger in most grid boxes. These differences are smaller in the summer than in the winter months and in the range −1 hPa to 15 hPa.
The differences in air temperature climatology are very small and caused by the changes to the input data. The changes are of the order of a tenth of a degree for means as well as for standard deviations. Changes in the input data caused changes smaller than 1 K in the dew point temperature climatology as well, that are spatially and temporally statistically distributed.
Comparison With Reanalyses
The time series of the monthly mean values of the BNSCatm parameters 2 m air temperature and air pressure are compared with the corresponding parameters from the ERA-Interim reanalysis (Dee et al., 2011). For this purpose, in the first step, the ERA Interim data was transformed to the BNSC grid. Then climatologies for the period 1981–2010 of air pressure and air temperature are calculated from ERA-Interim data and compared with the corresponding climatology of the BNSCatm.
Figure 5 shows the results for the mean air pressure difference between BNSCatm and ERA-Interim climatologies for January (A) and July (B). The mean monthly differences are in the interval −2.2 hPa (November) to +2.7 hPa (February). In winter they are positive in most parts of the open sea, but negative close to most coastlines except for the Danish coast, the Skagerrak and Kattegat. This means that, especially during winter, the ERA-Interim reanalysis has higher pressure values near most of the coasts than the BNSCatm. A possible reason is that the BNSCatm only contains averaged data from observations above sea, whereas the reanalysis has grid boxes, where results above sea were mixed with land data that had higher pressure values. On the other hand, ERA-Interim has slightly lower pressure values over open water than BNSCatm and there are particularly large deviations in the north, where the number of observation data is lower. So it can be assumed that this is caused by sampling errors of the BNSCatm, especially as the largest differences tend to occur at the edges of the data field.
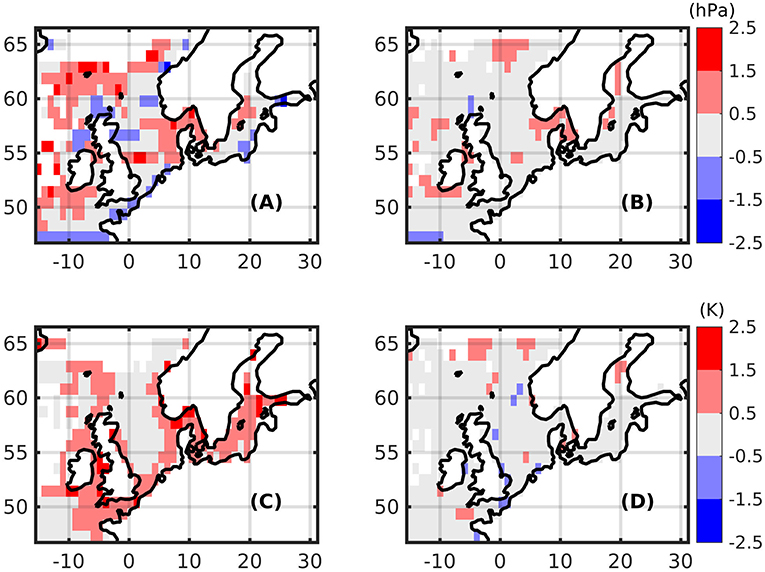
Figure 5. Difference between BNSC and ERA Interim Climatology 1981–2010 for mean sea level pressure (A,B) and air temperature (C,D) for the months January (A,C) and July (B,D).
A similar comparison on a monthly basis for sea level pressure was done for ERA-40 (Uppala et al., 2005) and ERA-Interim for the time period from 1979 to 2001, as well as with COSMO-REA6 (Bollmeyer et al., 2015) for the time period from 1995 to 2015 (not shown). The comparison between sea-level pressure in BNSCatm, ERA-Interim and ERA-40 climatology showed mean monthly differences in the interval of −1.5 hPa and 1.5 hPa. The comparison with COSMOS-REA6 showed mean monthly differences in the interval of −1 hPa to 1 hPa overall mean difference. All reanalyses show similar patterns as described above, with negative values at the coast and slightly positive values at open water. The differences in winter are higher compared to differences in summer.
One possible explanation for the average lower pressures of the re-analyses over open water is the “fair weather bias,” which means that ships avoid the bad weather conditions associated with low pressures, and therefore there are fewer measurements in these situations. These low pressure values are therefore missing in the mean values of the BNSCatm, but are included in the re-analyses. On the coasts, on the other hand, the re-analyses seems to have on average too high pressure values, which is probably again due to mixed land/sea grid boxes in the re-analyses.
The mean 2 m air temperature difference between the climatologies of BNSCatm and ERA Interim is shown in Figure 5 for January (C) and July (D). The differences are between −2.1 K (May) and 8 K (December), but this occurs only at a single box at the Norwegian coast, most differences are below 2 K. The average air temperatures of the BNSCatm are higher during winter on the coasts and especially over the Baltic Sea than in the ERA-Interim data set. This is a well-known effect (Schade et al., 2013), as in ERA-Interim, during winter the cooler temperatures of the land points are mixed with the warmer ocean. During summer, this effect occurs in the opposite direction, but in this case the temperature differences between warm land and cold sea are smaller than in winter, since land is a worse heat storage. Again, the differences north of the UK are most likely related to a sampling error caused by the lower number of observations in this region, and most extreme values occur at the edges of the data field as well.
Comparison With Station Data
Data from coastal measurements are not included in the calculation of the BNSCatm, Therefore, these data can be used to investigate the differences between BNSCatm and reanalysis data at the coasts. The monthly mean of the measurements at a station and in the nearest grid cell in the BNSCatm and ERA-40 is compared for the overlapping time periods. Figure 6 shows the station names (A) and the mean difference of monthly means of BNSCatm and ERA-40 to the measurements (B). In most cases, the mean values of both BNSCatm and ERA-40 are lower than the values of the measurements. At the North Sea coast, the differences between BNSCatm and measurements are smaller than those between the reanalysis and the measurements. At the Baltic Sea coast, the reanalysis is closer to the observations than the BNSC. Nevertheless, these differences of both products lie within the range of uncertainty, as they are also due to the fact that we compared means over an area to a point measurement and that the areas of the corresponding grid cells were completely over sea and not over the coast.
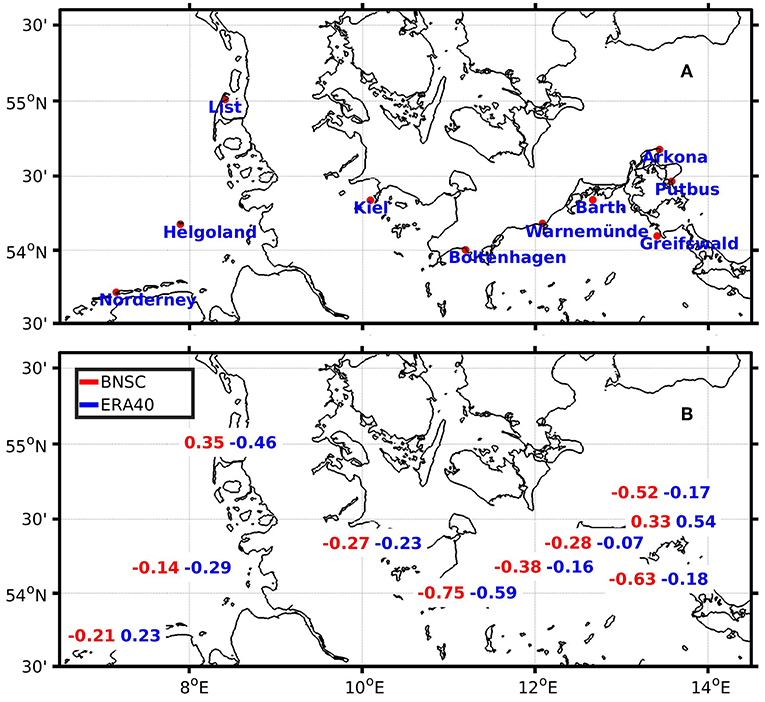
Figure 6. (A) Station names, (B) Mean Difference of monthly mean sea level pressure [hPa] in measurements to closest grid cell in BNSCatm (red) and ERA-40 (blue).
Comparison With Area Means and ICOADS
The 14 results for the different areas showed in general excellent conformity with each other, only small differences of up to 2°C in winter for some areas in the North. One explanation is, as we know, that these are the months and areas with lowest data density. A direct comparison of the BNSCatm 30-year climatology 1981–2010 with the ICOADS 30-year mean 1981–2010 (available online at https://icoads.noaa.gov/data.icoads.html) confirms this assumption. In principle, the data sets show similar patterns and values for all atmospheric parameters and months. However, the ICOADS data set has a much coarser resolution on a 2 × 2 degree grid. The ICOADS product is a simple monthly mean of all available data, that includes everything without checking the quality and number of data per grid point. In the averaged 30-year data product, ICOADS exhibits obvious artifacts in areas of low data density that are not in the BNSCatm. Sadikni et al. (2018) found similar results when KNSC was compared to ICOADS. This shows the importance of careful quality checks and averaging methods that take several aspects like diurnal cycles and data density into account.
BNSChydr
The data products for the hydrographic part of the BNSC are available as time series of monthly and annual mean values on standard depth levels for the parameters temperature and salinity. There are three different kinds of data products
1. Time series of box averages (temporal mean values over all observations in a 0.25° × 0.25° grid boxes in 1 year, respectively 1 month)
2. Decadal box averages (based on 1)
3. Interpolated fields (based on 2).
In the following, those three data products are described in more detail.
Time Series of Box Averages
As described in section Creation of mean values, box averages of temperature and salinity are calculated for the BNSC region. This yields a time series of annual and monthly mean values for the period 1873–2015. Grid boxes that either do not contain any observation or solely observations that do not pass the quality control, are left empty. As an example, Figure 7 shows the annual mean values at the surface for the year 2000. Additionally, the corresponding standard deviations and the number of observations contributing to the mean value are shown for temperature and salinity.
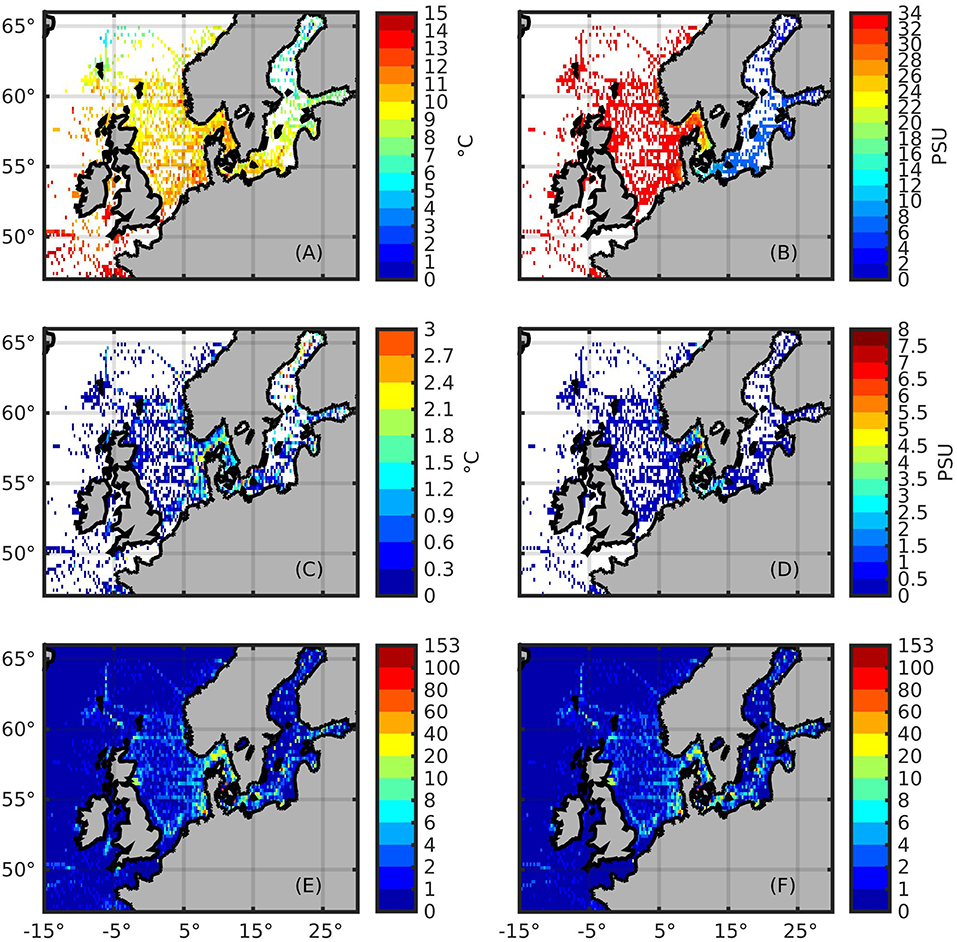
Figure 7. Sea surface: annual mean values of both BNSChydr parameters [temperature: (A) and salinity: (B)] for the year 2000, together with the respective standard deviation (C,D) and the number of observations (E,F).
Concerning the distribution of populated boxes as a function of depth and time (not shown) it can be stated that deeper water layers (below approximately 1,000 m) show only very few populated boxes until the beginning of the 1950s, in salinity as well as in temperature. After that, the data density increases (see also Figure 1) and also the deeper layers are populated.
Decadal Box Averages
For the decades determined in section Creation of mean values, a temporal mean over the box averages is created together with the corresponding standard deviation. Every populated box is considered for this. An example, the decadal temperature mean of August, is shown in Figure 8A at 10 m depth for the decade 1976–1985.
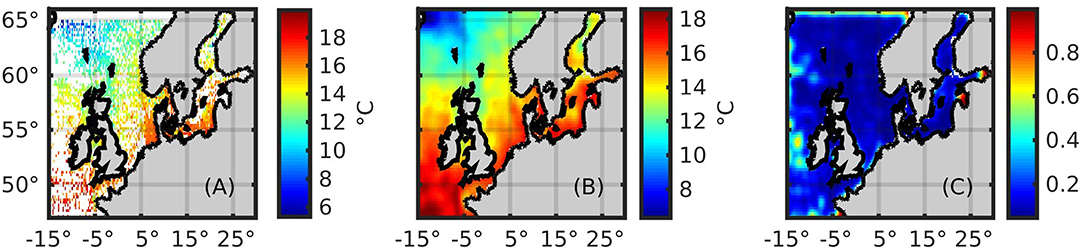
Figure 8. TEMPERATURE, August 1976–1985, depth = 10 m, decadal monthly mean values as box averages (A), interpolated field (B) (both in °C) and the corresponding relative interpolation error (C, dimensionless).
To be able to estimate the representativeness of the temporal mean for the respective decade, further statistics are made available together with the decadal monthly mean and the corresponding standard deviation. This is, on the one hand, the number of years that went into the decadal monthly mean with the maximum number consequently accounting to 10. On the other hand, information about the coverage of the time window of the decade is provided. For this purpose, an average and corresponding standard deviation are calculated of the years that contribute to the decadal mean. This defines the temporal center and spread of the decadal box average. A decadal monthly mean, for which all of the 10 years contribute, yields a mean value of, for example, 1990.5 (for the decade 1986–1995) and a standard deviation of 3.0.
Interpolated Fields
Based on the decadal box averages, as described in section Horizontally interpolated fields, the interpolated fields of the decadal monthly means are created. An example is displayed in Figure 8B. Besides other statistical parameters that are not explicitly shown here, a relative interpolation error is made available and is shown for the example in Figure 8C. For the definition of the interpolation error, see Gouretski and Koltermann (2004). The relative interpolation error differs between 0 and 1 and should always be considered together with the interpolated field. It can be used to mask field values. One example would be to use only interpolated values that correspond to a relative interpolation error of <0.5.
Sensitivity Study
In contrast to the BNSCatm, the sensitivity study for BNSChydr was done on different depth horizons, thus yielding of up to 105 different fluctuation ranges, depending on the region in focus. For the display of the results, Table 3 is restricted to three exemplary depth levels for each region for temperature and salinity, which were examined in two different temporal resolutions. In general, it can be stated that the fluctuation ranges for temperature and salinity are higher in monthly resolution. Regarding the different regions, the Belt Sea seems to be most sensitive to the observational database not only in salinity but also in temperature. The lowest fluctuation ranges, similar to the results of BNSCatm, are found in the central North Sea for temperature and annual mean salinity. The monthly mean salinity fields are least sensitive to the observational database in the southern region of the Atlantic part of the BNSC region.
Comparison With Other Data Products
The results of the comparison of the monthly mean BNSChydr fields with other data products are summarized in Figure 9. Shown here are the distributions of the relative frequency of the absolute differences between the BNSC and the three other data products, derived as described in section Comparison With Other Data Products. The results show that the mean value of the absolute differences is close to zero for all three comparisons, implying that the data products in general agree well. The spread of the distributions, however, is significantly different between the three data product comparisons: it is least in the comparison with KNSCv2 and highest in the comparison with BSRA.
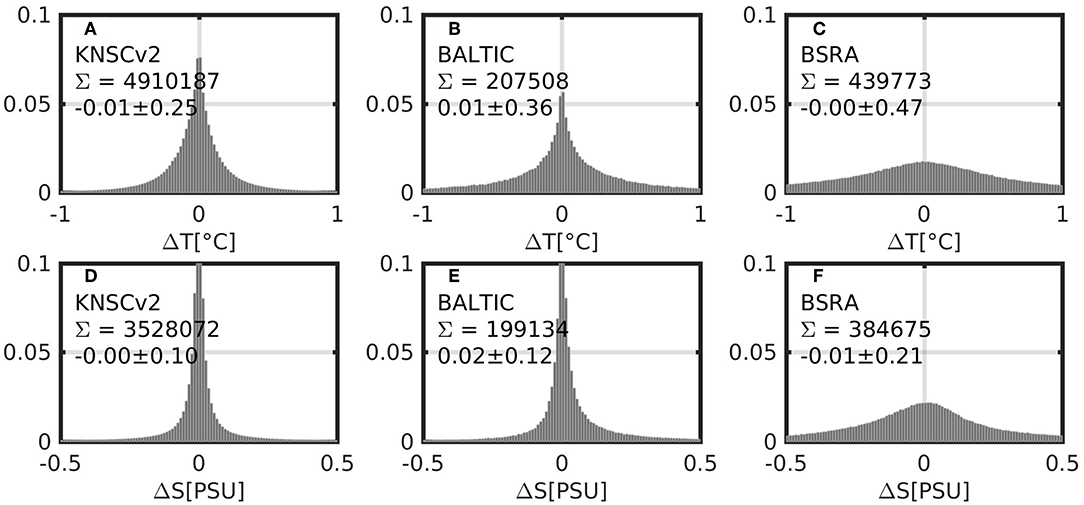
Figure 9. Relative frequency distributions of absolute differences between the BNSC data product and KNSCv2 [temperature: (A) and salinity: (D)], the Baltic ATLAS [temperature: (B) and salinity: (E)] and BSRA [temperature: (C) and salinity: (F)]. Additional information are the number of collocated boxes (i.e., 4910187 in the case of KNSCv2) and the mean and standard deviation of the absolute differences.
Regarding salinity, the highest discrepancies between the data products can be found (not shown) in the transition area between the Baltic and the North Sea, the area of Skagerrak, Kattegat and Belt Sea. This area is characterized by high horizontal salinity gradients, which explains the large differences between the data products.
Except of singularities, no regional emphasis can be found in the comparison of the temperature fields of the data products. However, the differences exhibit a seasonal signal that is most pronounced in the subsurface waters. This implies that the reproduction of the seasonal cycle is different between the BNSC and the other three data products. Intercomparison of BSRA and KNSCv2, however, yields a similar result, which means that the differences in the seasonal cycle cannot be explained by the BNSC alone. Yet, this requires further investigation.
BNSChydr fields in annual resolution were compared only to the KNSCv2- annual fields and show good agreement. The absolute differences are symmetrically distributed around zero with a mean difference of 0.00 and a standard deviation of 0.34°C for temperature, respectively, 0.01 ± 0.10 PSU for salinity.
Summary and Outlook
The main characteristics of the BNSC product are the following:
1. It comprises the entire North and Baltic Seas and includes fields of atmospheric and hydrographic parameters in different temporal resolutions on a compatible grid over a long common period (1950–2015).
2. The temporal sampling error was effectively reduced by appropriate correction methods.
3. There is one uniform quality control procedure for all in situ measurements of the ocean, and one for the atmosphere.
BNSCatm
A new atmospheric climatology of North and Baltic Sea on a one degree grid was created based on marine in situ observations. It contains monthly means of the parameters sea level pressure, 2 meter air temperature, and 2 meter dew point temperature for the period 1950–2015, a climatology for all 12 months of this time period, and monthly climatologies of the periods 1951–1980, 1961–1990, 1971–2000, and 1981–2010. The resulting data set contains mean values as well as standard deviations, and the mean number of observations per grid point.
The present version includes time and area of the KNSC climatology, on the same grid, but some small improvements were applied in the production that caused differences. The differences are largest in air pressure (between −1.5 to 2 hPa) and especially its standard deviation (about −1 to 15 hPa) as this is calculated with a different method. The values of mean air temperature and dew point temperature and associated standard deviations show only small changes of <1 degree compared to the KNSC climatology.
The monthly comparison between BNSCatm and ERA-Interim climatologies for the period 1981–2010 showed for mean pressure differences in the interval −2.2 hPa to +2.7 hPa. The mean 2 m air temperature differences are between −2.1 K and 8 K at a single point at the Norwegian coast, and if that is ignored, the maximum difference is below 2 K. Most extreme values occur at the edges of the data field. A part of these differences can be explained by the so called “fair weather bias,” which means that there are fewer measurements under severe weather conditions. This can cause a bias compared to the reanalysis results, which includes land stations and fills such gaps at sea with modeled data. But this inclusion of the land data leads in the analysis to mixed land/sea boxes that arise on the coasts and tend to have higher values than the BNSC.
The comparison between sea-level pressure in BNSCatm, ERA-Interim and ERA-40 climatology for 1979–2001 showed mean differences in the interval of −1.5 hPa and 1.5 hPa. Additionally the comparison with COSMOS-REA6 for 1995–2015 showed mean deviations between −1 hPa to 1 hPa. Highest absolute differences between the reanalysis and BNSCatm occur in winter and lowest in summer with better agreement over the open water and less good agreement close to the coast.
An intercomparison between BNSCatm, the ERA-40 reanalysis and observations based on station data was carried out. The mean deviations of both BNSCatm and ERA-40 from the observational data are within the range of uncertainty and show a regional pattern with the BNSC being closer to the observations at the North Sea coast and ERA-40 at the Baltic Sea coast.
Lessons to be learned from the sensitivity analysis are that the data set of BNSCatm is more stable in the period from 1960 onward and in the later 30-year climatologies than before 1960 and in the first climatology from 1951 to 1980.
Regular updates of the dataset and an extension of the data set for wind speed and direction are planned for the future.
BNSChydr
The BNSChydr data product presented here consists of gridded 3D-fields in different temporal resolutions (monthly, annual, decadal monthly) and different processing levels (box-averages and horizontally interpolated fields). A comprehensive description of the data used and the methods applied to create the data product are followed by the results of a sensitivity analysis, which estimates, on a regional basis, the sensitivity of the temporal mean fields to the basis of the observational data. The Belt Sea exhibits the highest fluctuation ranges in this respect, in both temperature and salinity, whereas the central North Sea is characterized by a low sensitivity in both parameters.
The comparison with other data products yields good agreement in general, yet further investigations, which are beyond the scope of this paper, are necessary.
In future, it is desirable to continue this work by implementing further and future observations. Based on the entity of quality-controlled observations of T und S, one can also think of creating more aggregated data products with respect to, for example, the ocean's heat content, and stratification or climate indices.
Author Contributions
IH created the hydrographic part of the BNSC, carried out the sensitivity analysis of both the BNSCatm and the BNSChydr, compared the BNSChydr to other data sources, and wrote the corresponding parts of the manuscript. IH is the lead author of this manuscript. AJ-B has created the atmospheric part of the BNSC climatology data set, carried out the analysis of the results, the comparisons with ERA Interim and has therefore written the description of the methodology, and the analysis of the atmospheric part of the data set as well as the comparisons with ERA Interim. AA provided the input data set from DWD for the BNSCatm, participated in meetings for the planning and discussion of the analysis of the climatology, and corrected the manuscript. AG participated in meetings for the planning and discussion of the analysis of the climatology and corrected the manuscript. VG created the hydrographic part of the BNSC. CJ contributed to the analysis of the final product as well as comparison to other products and the writing of the manuscript. BK provided input data for BNSChydr, participated in meetings for the planning and discussion of the analysis of the BNSC data product. JM participated in meetings for the BNSC and corrected the manuscript. RS created most of the predecessor of the atmospheric part of the BNSC, i.e., the KLIWAS North Sea climatology, and revised this manuscript. BT participated in meetings for the planning and discussion of the analysis of the climatology and corrected the manuscript.
Funding
This work was partly funded by the Cluster of Excellence CliSAP (EXC177) Universität Hamburg, funded through the German Science Foundation (DFG). IH received funding for this work from BSH.
Conflict of Interest Statement
The authors declare that the research was conducted in the absence of any commercial or financial relationships that could be construed as a potential conflict of interest.
Acknowledgments
The authors would like to thank the Maritime Data Center of the DWD for providing the atmospheric observational data. The hydrographic observational data were obtained from different data sources. Thanks, for providing marine in situ data, to the World Ocean Data Center (WOD), the Deutsches Ozeanografisches Datenzentrum, Germany (DOD), the Leibniz Institute for Baltic Sea Research Warnemünde, Germany (IOW), the International Council for Exploration of the Sea (ICES), the Institute of Meteorology and Water Management – National Research Institute, Poland (IMGW-PIB), the Royal Netherlands Institute for Sea Research (NIOZ), the British Oceanographic Data Centre (BODC), the University of Aarhus, and the International ARGO-Project (www.ifremer.fr). The authors would like to thank Kerstin Jochumsen, Sabine Hüttl-Kabus, Nils Schade, Hartmut Heinrich, (all BSH), Natacha Féry (DWD) and Manfred Bersch (UHH) for support and useful discussions concerning the work presented in this paper. Thanks to Detlef Stammer for the support of this work. This work would not have been possible without the wealth of scientific observations in the North and Baltic Sea region. To take measurements at sea and analyze the samples is often a stressful, yet important, work. The authors are grateful to all the scientists and technicians that were involved in sampling, analyzing and publishing the observational data via the data centers. The authors would like to thank the two reviewers for their valuable and detailed comments which helped to improve the manuscript.
References
Axell, L., and Liu, Y. (2016). Application of 3-D ensemble variational data assimilation to a Baltic Sea reanalysis 1989–2013. Tellus A Dynamic Meteorol. Oceanography 68:24220. doi: 10.3402/tellusa.v68.24220
Berrisford, P., Dee, D., Poli, P., Brugge, R., Fielding, K., Fuentes, M., et al. (2011). The ERA-Interim Archive Version 2.0, ERA Report Series 1. Available online at: http://www.ecmwf.int/en/elibrary/8174-era-interim-archive-version-20
Bersch, M., Gouretski, V., Sadikni, R., and Hinrichs, I. (2013). KLIWAS North Sea Climatology of Hydrographic Data (Version 1.0). World Data Center for Climate (WDCC) at DKRZ. doi: 10.1594/WDCC/KNSC_hyd_v1.0
Bersch, M., Hinrichs, I., Gouretski, V., and Sadikni, R. (2016). Hydrographic climatology of the North Sea and surrounding regions – version 2.0, Center for Earth System Research and Sustainability (CEN), University of Hamburg. Available online at: https://icdc.cen.uni-hamburg.de/daten/ocean/knsc-hydrographic.html
Bollmeyer, C., Keller, J. D., Ohlwein, C., Wahl, S., Crewell, S., Friederichs, P., et al. (2015). Towards a high-resolution regional reanalysis for the European CORDEX domain. Q. J. Roy. Meteor. Soc. 141, 1–15. doi: 10.1002/qj.2486
Boyer, T. P., Antonov, J. I., Baranova, O. K., Coleman, C., Garcia, H. E., Grodsky, A., et al. (2013). “World Ocean Database 2013,” in NOAA Atlas NESDIS 72, Technical Edn., eds S. Levitus and A. Mishonov (Silver Spring, MD), 209. doi: 10.7289/V5NZ85MT
Brys, G., Hubert, M., and Struyf, A. (2003). A Comparison of Some New Measures of Skewness, Heidelberg: Physica-Verlag HD.
Bülow, K., Dietrich, C., Elizalde, A., Gröger, M., Heinrich, H., Hüttl-Kabus, S., et al. (2014). Comparison of Three Regional Coupled Ocean Atmosphere Models for the North Sea Under Today‘s and Future Climate Conditions (KLIWAS Schriftenreihe; KLIWAS-27/2014), Koblenz: Bundesanstalt für Gewasserkunde.
Compo, G. P., Whitaker, J. S., Sardeshmukh, P. D., Matsui, N., Allan, R. J., Yin, X., et al. (2011). The Twentieth Century Reanalysis Project. Q. J. Roy. Meteor. Soc. 137, 1–28. doi: 10.1002/qj.776
Dee, D. P., Uppala, S. M., Simmons, A. J., Berrisford, P., Poli, P., Kobayashi, S., et al. (2011). The ERA-Interim reanalysis: configuration and performance of the data assimilation system. Q. J. Roy. Meteor. Soc. 137, 553–597. doi: 10.1002/qj.828
Feistel, R., Feistel, S., Nausch, G., Szaron, J., Łysiak-Pastuszak, E., and Ærtebjerg, G. (2008). “BALTIC: monthly time series 1900–2005,” in State and Evolution of the Baltic Sea, 1952–2005, eds R. Feistel, G. Nausch, and N. Wasmund (John Wiley & Sons, Inc.), 311–336. doi: 10.1002/9780470283134.ch11
Gandin, L. (1965). Objective Analysis of Meteorological Fields (Leningrad: Gidromet), 1963, [English Translation]. Jerusalem: Israel Program for Scientific Translation.
Gouretski, V. (2018). World ocean circulation experiment – argo global hydrographic climatology. Ocean Sci. 14, 1127–1146. doi: 10.5194/os-14-1127-2018
Gouretski, V. V., and Koltermann, K. P. (2004). “WOCE global hydrograhic climatology,” in Berichte des BSH, 35, Bundesamt für Seeschiffsfahrt und Hydrographie (Hamburg), 52.
Janssen, F., Schrum, C., and Backhaus, J. O. (1999). A climatological data set of temperature and salinity for the Baltic Sea and the North Sea. Deutsche Hydrografische Zeitschrif. 51:5. doi: 10.1007/BF02933676
Kalnay, E., Kanamitsu, M., Kistler, R., Collins, W., Deaven, D., Gandin, L., et al. (1996). The NCEP/NCAR 40-Year reanalysis project. Bull. Am. Meteorol. Soc. 77, 437–472. doi: 10.1175/1520-0477(1996)077<0437:TNYRP>2.0.CO;2
Kofalk, S., Moser, H., Rudolf, B., Heinrich, H., and Heyer, H. (2010). “Facing the impacts on navigation and waterways by climate change: The German Research Program KLIWAS,” 32nd PIANC International Navigation Congress 2010, Vol. 1, PLI-ANC. 134–140.
Korevaar, C. G. (1990). North Sea Climate, XII. Springer: Netherlands. doi: 10.1007/978-94-867009-1982-2
Michaelsen, K., Krell, U., Reinhardt, V., Graßl, H., and Kaufeld, L. (1998). Climate of the North Sea. Deutscher Wetterdienst. Available online at: www.dwd.de
Núñez-Riboni, I., and Akimova, A. (2015). Monthly maps of optimally interpolated in situ hydrography in the North Sea from 1948 to 2013. J. Marine Syst. 151, 15–34. doi: 10.1016/j.jmarsys.2015.06.003
Pätsch, J., Burchard, H., Dieterich, C., Gräwe, U., Gröger, M., Mathis, M., et al. (2017). An evaluation of the North Sea circulation in global and regional models relevant for ecosystem simulations. Ocean Model. 116, 70–95. doi: 10.1016/j.ocemod.2017.06.005
Reiniger, R. F., and Ross, C. K. (1968). A method of interpolation with application to oceanographic data. Deep Sea Res. Oceanograp. Abstr. 15, 185–193. doi: 10.1016/0011-7471(68)90040-5
Rienecker, M. M., Suarez, M., Gelaro, J., Todling, R., Julio Bacmeister, R., Liu, E., et al. (2011). MERRA: NASA's Modern-era retrospective analysis for research and applications. J. Climate 24, 3624–3648. doi: 10.1175/JCLI-D-11-00015.1
Sadikni, R., Bersch, M., Jahnke-Bornemann, A., and Hinrichs, I. (2013). KLIWAS North Sea Climatology of Meteorological Data (Version 1.0), World Data Center for Climate (WDCC) at DKRZ. doi: 10.1594/WDCC/KNSC_met_v1.0
Sadikni, R., Schade, N. H., Andersson, A., Jahnke-Bornemann, A., Hinrichs, I., Gates, L., et al. (2018). The KLIWAS North sea climatology. part I: processing of the atmospheric data. J. Atmos. Oceanic Technol. 35, 111–126. doi: 10.1175/JTECH-D-17-0044.1
Schade, N. H., Heinrich, H., and Rosenhagen, G. (2013). Regional evaluation of ERA-40 reanalysis data with marine atmospheric observations in the North Sea Area. Meteorol. Z. 22, 675–684. doi: 10.1127/0941-2948/2013/0471
Schade, N. H., Sadikni, R., Jahnke-Bornemann, A., Hinrichs, I., Gates, L., Tinz, B., et al. (2018). The KLIWAS north sea climatology. part II: assessment against global reanalyses. J. Atmos. Oceanic Technol. 35 127–145. doi: 10.1175/JTECH-D-17-0045.1
Schmager, G., Fröhle, P., Schrader, D., Weisse, R., and Müller-Navarra, S. (2008). Sea State, Tides, in State and Evolution of the Baltic Sea, 1952-2005: A Detailed 50-Year Survey of Meteorology and Climate, Physics, Chemistry, Biology, and Marine Environment. John Wiley & Sons, Inc. doi: 10.1002/9780470283134.ch7
Sokolov, S., and Rintoul, S. R. (1999). Some remarks on interpolation of nonstationary oceanographic fields. J. Atmos. Oceanic Technol. 16, 1434–1449. doi: 10.1175/1520-0426(1999)016<1434:SROION>2.0.CO;2
The Gulf of Finland assessment. (2016). “The gulf of finland assessment,” in Reports of the Finnish Environment Institute 27/2016, eds M. Raateoja and O. Setälä (Helsinki: Finnish Environment Institute).
Uppala, S. M., KÅllberg, P. W., Simmons, A. J., Andrae, U., Bechtold, V. D. C., Fiorino, M., et al. (2005). The ERA-40 re-analysis. Q. J. Roy. Meteor. Soc. 131, 2961–3012. doi: 10.1256/qj.04.176
Vandervieren, E., and Hubert, M. (2004). “An adjusted boxplot for skewed distributions,” in Proceedings in Computational Statistics 2004, eds J. Antoch. 1933–1940 (Heidelberg: Springer-Verlag).
Keywords: North Sea, Baltic Sea, climatology, reanalysis, in situ data, quality control
Citation: Hinrichs I, Jahnke-Bornemann A, Andersson A, Ganske A, Gouretski V, Jensen C, Klein B, Möller J, Sadikni R and Tinz B (2019) The Baltic and North Seas Climatology (BNSC)—A Comprehensive, Observation-Based Data Product of Atmospheric and Hydrographic Parameters. Front. Earth Sci. 7:158. doi: 10.3389/feart.2019.00158
Received: 19 December 2018; Accepted: 05 June 2019;
Published: 02 July 2019.
Edited by:
Markus Meier, Leibniz Institute for Baltic Sea Research (LG), GermanyReviewed by:
Vladimir Alexeevich Ryabchenko, P. P. Shirshov Institute of Oceanology (RAS), RussiaGermo Väli, Tallinn University of Technology, Estonia
Copyright © 2019 Hinrichs, Jahnke-Bornemann, Andersson, Ganske, Gouretski, Jensen, Klein, Möller, Sadikni and Tinz. This is an open-access article distributed under the terms of the Creative Commons Attribution License (CC BY). The use, distribution or reproduction in other forums is permitted, provided the original author(s) and the copyright owner(s) are credited and that the original publication in this journal is cited, in accordance with accepted academic practice. No use, distribution or reproduction is permitted which does not comply with these terms.
*Correspondence: Iris Hinrichs, iris.hinrichs@uni-hamburg.de