- 1WSL-Institute for Snow and Avalanche Research SLF, Davos, Switzerland
- 2School of Architecture, Civil and Environmental Engineering, École Polytechnique Fédérale de Lausanne, Lausanne, Switzerland
- 3Karlsruhe Institute of Technology, Institute of Meteorology and Climate Research, Atmospheric Environmental Research, Garmisch-Partenkirchen, Germany
The energy balance of an alpine snow cover significantly changes once the snow cover gets patchy. The local advection of warm air causes above-average snow ablation rates at the upwind edge of the snow patch. As lateral transport processes are typically not considered in models describing surface exchange, e.g., for hydrological or meteorological applications, small-scale variations in snow ablation rates are not resolved. The overall model error in the hydrological model Alpine3D is split into a contribution from the pure “leading edge effect” and a contribution from an increase in the mean air temperature due to a positive snow-albedo feedback mechanism. We found an overall model error for the entire ablation period of 4% for the almost flat alpine test site Gletschboden and 14% for the Wannengrat area, which is located in highly complex terrain including slopes of different aspects. Terrestrial laser scanning measurements at the Gletschboden test site were used to estimate the pure “leading edge effect” and reveal an increase in snow ablation rates of 25–30% at the upwind edge of a snow patch and a total of 4–6% on a catchment scale for two different ablation days with a snow cover fraction lower than 50%. The estimated increase of local snow ablation rates is then around 1–3% for an entire ablation period for the Gletschboden test site and approximately 4% for the Wannengrat test site. Our results show that the contribution of lateral heat advection is smaller than typical uncertainties in snow melt modeling due to uncertainties in boundary layer parameters but increases in regions with smaller snow patch sizes and long-lasting patchy snow covers in the ablation period. We introduce a new temperature footprint approach, which reproduces a 15% increase of snow ablation rates at the upwind edge of the snow patch, whereas observations indicate that this value is as large as 25%. This conceptual model approach could be used in hydrological models. In addition to improved snow ablation rates, the footprint model better represents snow mask maps and turbulent sensible heat fluxes from eddy-covariance measurements.
Introduction
Modeling an alpine snow-cover is a challenging issue especially in the melting season when the snow cover becomes patchy (Essery et al., 2006; Fujita et al., 2010; Mott et al., 2011, 2013). Accurate modeled snow ablation rates are of special interest for e.g., practitioners in hydropower generation (Schaefli et al., 2007) or flood prevention (Wever et al., 2017) especially in the late snow ablation period. Snow and hydrological models are typically forced by single point meteorological measurements, which are interpolated to the grid of the digital elevation model. Turbulent fluxes in the atmosphere are often calculated with Monin-Obukhov similarity theory based on this interpolated meteorological data. Therefore, snow and hydrological models do not include lateral transport of sensible and latent heat in the atmosphere (Marks and Dozier, 1992; Marks and Winstral, 2001; Pellicciotti et al., 2008; Schlögl et al., 2016). Hence, the influence of the upward heat flux caused by local advection of warm air from the bare ground toward snow-covered areas is not considered and the development of a stable internal boundary layer is underestimated in the models. The effect of lateral transport of sensible heat on snow ablation rates on the catchment scale can be neglected as long as the snow cover remains continuous. However, when the snow cover fraction decreases in the later stages of the ablation period, the local effect of lateral heat transport becomes important (Marsh and Pomeroy, 1996) and snow ablation rates increase at the upwind edge of the snow patch (Liston, 1999; Pomeroy et al., 2003). This leads to an underestimation of the available energy in the model for melting snow and few studies compensate for this effect by using parametrizations in hydrological models (Granger et al., 2006). The physical basis for parametrizations of the lateral heat advection flux was developed in the 1970s (Weisman, 1977), estimated as a function of the fetch distance (Liston, 1995) and refined in the boundary layer integration approach (Granger et al., 2002). Some studies (e.g., Helgason and Pomeroy, 2012; Harder et al., 2017) determined the local advection of sensible heat based on high resolution temperature profile measurements using thermocouples. These measurements are rare but crucial to calibrate model parametrizations. Contrary, eddy-covariance measurements over patchy snow covers are more frequent, but limited by large path lengths of the measurement devices which are not suitable to measure turbulence very close to the snow surface. However, measured turbulent sensible heat fluxes directly over the snow surface are recommended in order to assess the complex nature of heat-exchange processes over patchy snow-covers (Mott et al., 2016). As constant flux layers over patchy snow covers cannot develop to sufficient depth, turbulent sensible heat fluxes measured far away from the snow surface are not useful to assess snow ablation rates as the measurement is strongly influenced by the upwind air flow (Mott et al., 2017).
In this study, we present a conceptual model approach to account for the local advection of sensible heat in order to improve the model performance in the late stage of the ablation period. The strength of this approach is the purely analytical origin which avoids introducing empirical coefficients in the model. We develop adapted footprint estimations (Schuepp et al., 1990) in order to resolve the spatial variability of near-surface air temperatures and turbulent sensible heat fluxes. This fundamental theory was originally deployed for eddy-covariance measurements revealing the origin of the measured turbulence as a function of the measurement height, atmospheric stability and wind speed. As we do not measure two-dimensional near-surface air temperature fields in this study, the conceptual model approach is compared with two-dimensional snow ablation rates recorded with a terrestrial laser scanner.
This paper is organized as follows. In section Methods, study site, measurements, model setup and the footprint approach are introduced. In section Results, we assess the limitations of the Alpine3D model with respect to the calculation of snow ablation rates, the model error due to missing lateral transport processes, the model performance after applying the footprint approach with respect to turbulent sensible heat fluxes, snow mask maps and snow ablation rates and finally a sensitivity analysis to transfer estimated results from our study site to different scales. In section Discussion, the overall model error by neglecting lateral transport processes is discussed and major results of this study are summarized in section Conclusion.
Methods
Study Site
Our study site is located in the upper Dischma valley in the Swiss Alps near Davos. The investigated Gletschboden area (approximately 1 km south of Dürrboden) is almost flat with an extent of around 500 × 400 m (Figure 1).
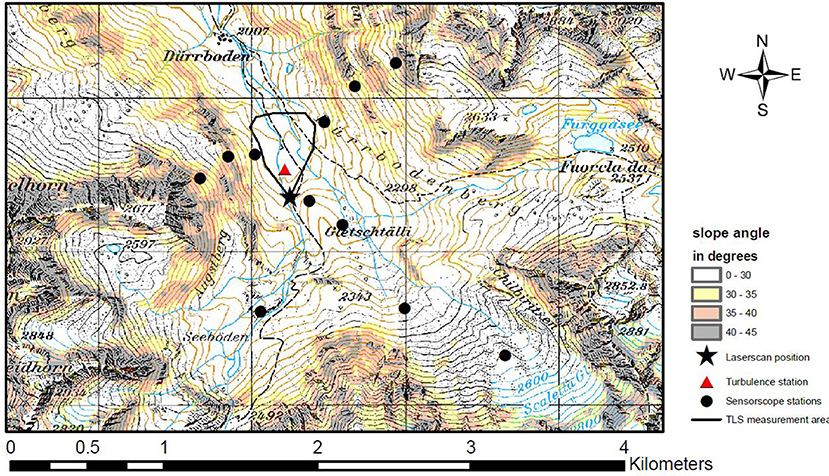
Figure 1. Measurement setup at the Gletschboden area in the upper Dischma valley. Mobile meteorological stations (black circle), Terrestrial laser scanning position (black asterisk), turbulence station (red triangle) and the laser scanner measurement area (solid black line) are shown.
Earlier studies during an extensive field campaign lead to several publications focusing on valley-scale meteorology in the 1980s (Egger, 1983; Hennemuth, 1986). More recent studies (Lehning et al., 2006; Bavay et al., 2009) were conducted in the Dischma valley focusing on hydrological questions and climate change. Additionally, the valley has been used to investigate the influence of locally varying radiation on runoff as a function of catchment size (Comola et al., 2015) and runoff temperatures (Gallice et al., 2016). An extensive field campaign, the Dischma experiment, has been conducted in the Dischma valley from 2014 to 2016 focusing on small-scale snow atmosphere interactions over patchy snow-covers in the late stage of the ablation period (Mott et al., 2017) and assessing small-scale variations in the atmospheric flow field in the surroundings of a steep rock wall and its influence on snow accumulation (Gerber et al., 2017).
Experimental results from the Gletschboden area are transferred to different horizontal scales in section Sensitivity Analysis by using artificial snow distributions for an idealized flat test site, which is described in detail in the companion paper. Additionally, earlier data from the Wannengrat field site (Mott et al., 2015) are re-analyzed.
Mesurements
Terrestrial Laser Scanning
We recorded snow ablation maps with a terrestrial laser scanner (TLS, Riegl-VZ6000) at the Gletschboden area (Figure 1). The laser scanner position is located approximately 30 vertical meters above the Gletschboden area at a northerly exposed slope. In total 44 measurement sets have been conducted in three consecutive years 2014–2016 (2014: 13 measurements; 2015: 17 measurements; 2016: 14 measurements). The laser scanner system has a single-point measurement frequency of 300 kHz and a beam divergence of 0.007°. This set-up allows a horizontal resolution of approximately 0.01 m in 100 m distance to the scanner position. One scan of the Gletschboden area lasts approximately 15 min. The travel time from the laser scanner toward the surface is recorded and afterwards converted into a point cloud of distances. 5 reflectors located at the Gletschboden area and in the closer surroundings were additionally scanned during each measurement to transform the point cloud from the scanner own coordinate system into Swiss coordinates. We tested the accuracy of the laser scanner measurement by repeating measurements from the same scan position and found a mean absolute error (MAE) of 0.5 ± 0.2 cm. Therefore, the small-scale variability of snow ablation rates is measured with high accuracy. Additionally, orthophotos have been created by using pictures recorded from the laser scanner in order to provide snow mask maps. Snow and bare ground can be distinguished by the color information of the orthophoto. Cells with blue band information greater than 175 were categorized as snow and all cells with values smaller or equal 175 were categorized as bare ground. The complete dataset can be found on ENVIDAT (http://dx.doi.org/10.16904/envidat.25).
Eddy Covariance Measurements
Eddy covariance measurements have been conducted at the Gletschboden test site in the ablation periods of three consecutive years 2014-2016. A vertical setup of three 3-D ultrasonic anemometers were installed at the Gletschboden area using a DA-600 Kaijo Denki for the lowermost measurement (0.3 m), using a CSAT3 (Campbell Scientific, Inc.) for the measurement in 2 m above the snow and a Young sonic in 3.2 m above the snow. Note, that the height of the measurement varied during the three ablation periods. The post-processing of the eddy covariance measurements includes despiking, rotating of the coordinate system and a frequency response correction, which is described in detail by Mott et al. (2017). The complete dataset can be found on ENVIDAT (http://dx.doi.org/10.16904/10).
Models
The physics-based surface process model Alpine3D has been used to simulate snow melt processes. Alpine3D is a spatially distributed, three-dimensional (atmospheric) model for analysing and predicting dynamics of snow-dominated surface processes in mountainous topography. It includes modules for snow cover (SNOWPACK), vegetation and soil, snow transport, radiation transfer and runoff which can be enabled or disabled on demand (Lehning et al., 2006).
Snow ablation rates from laser scanner measurements were compared with Alpine3D snow ablation rates at the Gletschboden test site. The model is forced by data from 10 mobile meteorological stations which are located in the near surrounding of the simulated test site and have been installed specifically for this study. Meteorological fields (air temperature and wind velocity) are interpolated to a 1 x 1 m horizontal grid by inverse distance weighting. Turbulent fluxes were calculated with the Monin-Obukhov bulk formulation (Blanc, 1987), using the univariate stability correction developed in Schlögl et al. (2017) and an aerodynamic roughness length over snow of 0.007 m. Note that the aerodynamic roughness length over bare ground is larger than over snow. In 2015, incoming longwave radiation is measured (instead of parametrized as in 2014 and 2016) at one full energy balance station installed at the Gletschboden area close to the turbulence station. Alpine3D is initialized with measured snow depth distributions from laser scanner measurements exemplarily for several days in the ablation period.
As Alpine3D is limited to pointwise simulating the vertical exchange between the ground and the atmosphere and does not include lateral transport of heat and moisture in the atmosphere except if the drifting and blowing snow module is switched on (Groot Zwaaftink et al., 2011, 2013), the small- scale variability in snow ablation rates during patchy snow covers could not be resolved (Mott et al., 2013). Note that lateral transport calculations in Alpine3D are based on external meteorological fields, which are very expensive to obtain and therefore not a common setup for hydrological simulations. Very high resolution three-dimensional atmospheric simulations are discussed in a companion paper. We conceptualize the effect of lateral heat advection on snow ablation rates, and introduce a footprint approach in the following subsection.
Footprint Approach
Flux footprints have been analytically calculated in the 1990s by solving the diffusion equation (Schuepp et al., 1990). They proposed that the flux footprint can be calculated by considering the cumulative normalized contribution to flux measurements (CNF): . This fundamental theory was deployed for eddy-covariance measurements revealing the origin of the measured turbulence as a function of the measurement height, atmospheric stability and wind speed. For neutral conditions, the flux contribution (FC) is the derivate of the CNF with respect to the upwind fetch distance xL and shown for two different measurement heights (Figure 2):
where U is the wind speed, z is the measurement height, is the friction velocity and k is the von Kàrmàn constant. Note, that the dimension of the flux contribution is [m−1], whereas the CNF (after integrating over the fetch distance) is dimensionless.
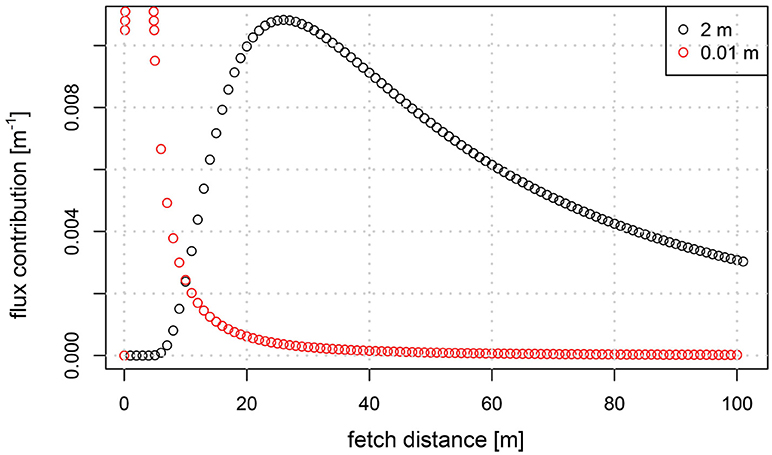
Figure 2. Flux contribution (FC) [m−1] as a function of the fetch distance for a measurement height of 2 m (black) and a theoretical measurement height of 0.01 m (red). In this example the wind velocity is 1 m s−1 and the friction velocity is 0.1 m s−1.
The footprint approach suggested is a conceptual model to describe the effect of lateral heat advection on snow ablation rates and not meant to represent a rigorous integration of the lateral transport equation, describing the effects on the sensible heat flux. Granger et al. (2002) attempt such a more rigorous integration, which requires more data than available in most (including our) practical cases. The footprint approach is adapted to calculate the fetch dependent near-surface air temperatures (instead of turbulent sensible heat fluxes) for a height of z = 0.01 m above the surface in Alpine3D. We chose z = 0.01 m to obtain a strictly monotonic decreasing flux contribution for a horizontal resolution of 1 m of the model grid, despite the fact that footprints decrease very close to the snow/bare ground transition (Figure 2). Thus, the flux contribution is largest for the smallest fetch distance (1 m). The strictly monotonic decreasing flux contribution ensures that the additional energy from lateral transport processes is largest at the snow/bare ground transition and gradually decreases further inside of the snow patch. A (theoretical) decrease in the horizontal resolution from 1 m to e.g., 0.01 m could lead to a non-strictly monotonic decreasing flux contribution (see Figure 2). For those situations, the height z needs to be adjusted to obtain a strictly monotonic decreasing flux contribution. The adapted footprint approach uses surface temperatures from Alpine3D as model input. To our knowledge, footprints for (surface) temperatures have not been developed yet, but separate scalar footprints exist, where concentrations instead of vertical fluxes are used to solve the diffusion equation (Schmid, 1994; Kljun et al., 2002). The maximum contribution of scalar footprints to the sensor/target pixel is located further upwind than for flux footprints. However, we used flux footprints instead of scalar footprints as a model for our weighting functions, as surface temperatures can be treated in a similar way as vertical fluxes. As a first approximation, Monin-Obukhov bulk formulation suggests that vertical fluxes of sensible heat are linearly dependent on the temperature difference between the surface and the air above. Vertical fluxes of sensible heat are therefore also linearly dependent on surface temperatures differences under the assumption of a constant air temperature and wind velocity at the reference height (z = 2 m). This assumption of a constant air temperature and wind velocity field at the reference height is only valid for small and flat test sites as the Gletschboden area and cannot be made for larger distances e.g., for larger snow patches. The calculation of the near-surface air temperature field (TA(0.01 m)xy) contains three main analysis steps, which are explained in the following and were conducted for each pixel ( )xy in the model domain and each time step of the model:
1. 30-min mean wind direction and the variation of the wind direction are estimated from the measurements at the eddy-covariance tower. We assume that the measured wind direction at the eddy-covariance tower is spatially homogenous inside the model domain. This assumption is a necessary requirement as only one meteorological station is available inside the model domain. However, a constant mean wind direction is a reasonable approximation of the true atmospheric conditions for our small and flat test site. Note that this approximation cannot be made for larger catchments. We define an upwind sector based on the wind direction and variation of the wind direction for each pixel in the model domain and each analysis time step. The width of the upwind sector corresponds to the standard deviation of the 30-min wind direction. Modeled surface temperatures from Alpine3D are used as input for the temperature footprint approach. All surface temperature pixels outside the upwind sector are not considered, whereas the surface temperature pixels inside the upwind sector are averaged as a function of the fetch distance (TS(xL)xy) (Figure 3c).
2. The flux contribution (Equation 1) is calculated for each time step and assumed to be equal for the entire model domain (Figure 2, red line). Measured wind speeds at the eddy-covariance tower and modeled friction velocity from Alpine3D are used to determine the flux contribution.
3. Finally, averaged surface temperatures , which are a function of the fetch distance xL, have been multiplied with the flux contribution function FC0.01(xL) (Equation 1) and integrated over the fetch distance (Equation 2).
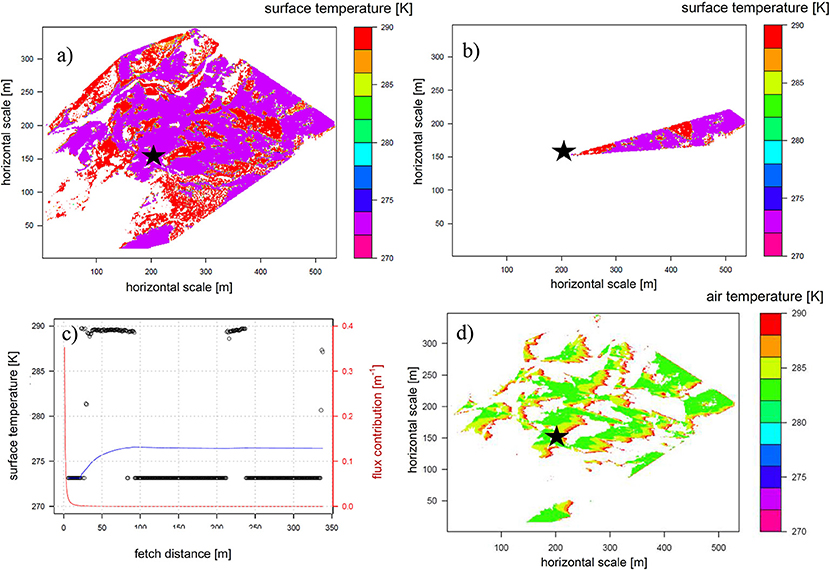
Figure 3. Stepwise explanation of the temperature footprint approach for one time step (21 May 2014, 12 UTC): (a) Alpine3D modeled surface temperatures [K] at the Gletschboden area. (b) Upwind sector shown exemplarily for one pixel (x = 200, y = 150, black star). (c) Averaged surface temperatures as a function of the fetch distance for a chosen pixel (black points), [K] (blue line) (both left y-axis) and flux contribution [m−1] (red line, right y-axis). (d) Near-surface air temperatures after the application of the temperature footprint approach [K] for the Gletschboden area.
The air temperature increase ΔTA(0.01 m)xy due to lateral heat transport processes is described in Equation 2 and is always positive, as the mean surface temperature of the entire model domain in the late ablation period is always larger as the freezing point temperature ΔTAf = 273.15 K.
Near-surface air temperature fields TA(0.01 m)xy are calculated for the late ablation period by adding ΔTA(0.01 m)xy to the interpolated standard Alpine3D air temperature field in 2 m above the surface (TA(2.0 m)xy) (Equation 3):
Afterwards, near-surface air temperature fields TA(0.01 m)xy are used to calculate turbulent sensible heat fluxes at the surface with the Monin-Obukhov bulk formulation. Note that this approach is strongly sensitive to an accurate albedo of the bare ground and accurate information on the spatial distribution of the snow cover at time of peak accumulation.
The different analysis steps of the temperature footprint approach are exemplarily shown for one time step (21 May 2014, 12 UTC) and one pixel (x = 200, y = 150) (Figure 3). Modeled surface temperatures over snow are typically at the freezing point and typically much larger over bare ground during noon (Figure 3a). The upwind sector is defined in Figure 3b, based on an almost southern wind direction (171°) with a 30-min variation in the wind direction of 10°. The median of surface temperatures (inside the upwind sector) as a function of the fetch distance from the target pixel (x = 200, y = 150) indicate two snow-free areas upwind of the target pixel (Figure 3c, black dots). The first snow-free area is located 25–75 m away from the target pixel and extremely influences TA(0.01 m)200, 150, shown by the increase of (Figure 3c, blue line) from 273.15 to 276 K in the area of enhanced surface temperatures. The second snow-free area is located 210-230 m away from the target pixel. As the flux contribution in this area is almost 0, large surface temperatures from the snow-free ground do not influence TA(0.01 m)200, 150, shown by a constant (Figure 3c, blue line) between 210-230 m fetch distance. The term is equal to 276.45 K, which implies an air temperature increase of 3.3 K due to the advection of warm air from the bare ground toward the target pixel. Finally, the near-surface air temperature field in 0.01 m above ground for all pixels in the model domain is shown in Figure 3d.
In absence of near-surface air temperature measurements, we evaluate the performance of our footprint approach by comparing simulated and measured snow ablation rates (section Local Snow Ablation Rates) and simulated and measured snow mask maps (section Snow Mask Maps).
To analyse the model performance of the conceptual model approach with measured turbulent sensible heat fluxes at one specific point, the measurement height was chosen to be the mid-level height of the eddy-covariance measurements (2.0 m). We recalculated modeled surface turbulent sensible heat fluxes from Alpine3D (instead of the modeled surface temperatures) (section Turbulent Sensible Heat Fluxes). The analysis of this model performance contains the same analysis steps as for the temperature footprint, but is based on modeled surface turbulent sensible heat fluxes in Alpine3D:
Results
Limitation of Alpine3D Snow Ablation Rates
As long as the snow cover fraction (SCF) is larger than 90%, Alpine3D sufficiently captures daily snow ablation rates (Figure 4). Both, daily snow ablation rates recorded from the scanner and snow ablation rates from Alpine3D simulations show on average 0.05 m day−1 snow ablation. We found almost no systematic bias and a mean absolute error of 0.02 m day−1 in the Alpine3D simulation. However, the spatial variability in measured snow ablation rates is around one order of magnitude larger than for the snow ablation rates in Alpine3D.
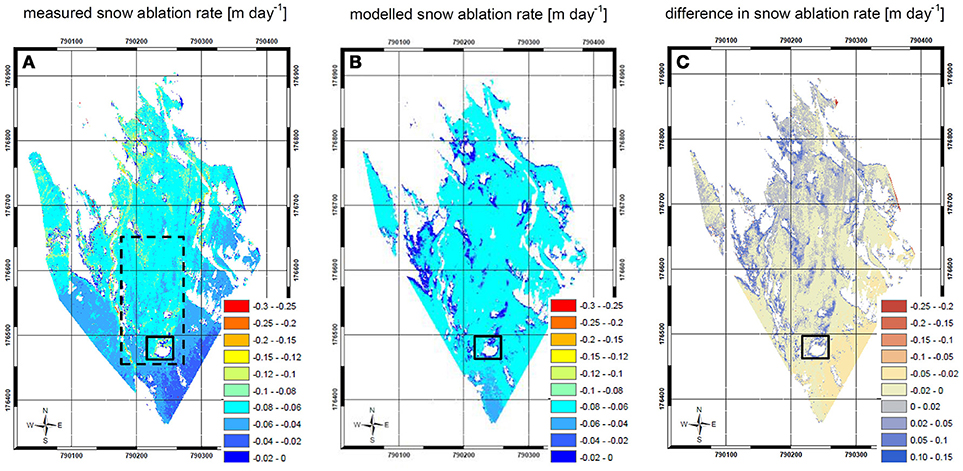
Figure 4. Snow ablation rates [m day−1] for the 06 May 2014 at the Gletschboden area in the upper Dischma valley. (A) Terrestrial laser scanning measurements, (B) Alpine3D model results, (C) model-measurement. The dashed box in (A) shows the location of the investigated area of Figure 5.
Even in this early stage of the ablation period, local heat advection is observed around the snow-free area below the toe of the northern exposed slope (marked by the black rectangle in Figure 4). Enhanced snow ablation rates were recorded with the laser scanner in the close vicinity of snow-free areas, which are not resolved in the model (Figure 4). This spatio-temporal dynamic in the melt-out of the snow cover is mainly a result of the end of winter snow distribution that is typically characterized by shallow snow covers at ridges and deep snow-covers in local depressions. Ridges become snow-free first and the near-surface atmosphere over snow-free areas at ridges is heated more than over persistent snow patches in local depressions, as the albedo of the bare ground is lower than the albedo of snow. Warmer air above the snow-free surface is efficiently transported toward the edge of the snow patches for high wind velocities, resulting in enhanced snow ablation rates at the upwind edge of the snow patches (Figure 4A). Stable internal boundary layers develop at the border between bare ground and snow due to changes in surface temperatures and grow along the fetch (Garratt, 1990; Mahrt and Vickers, 2005; Mott et al., 2011, 2016). Mott et al. (2017) showed that decoupling of the near-surface atmospheric layers from the surrounding warmer air is favored by the development of stable internal boundary layers. The complex interaction between the atmospheric boundary layer and the heterogeneous land-surface is therefore not resolved in our traditional model approach, as Alpine3D calculates the energy balance for each pixel separately based on the assumption of a boundary layer in equilibrium. This approach does not account for spatially varying boundary layer fields.
Above-Average Snow Ablation Rates at the Upwind Edge of Snow Patches
We assessed the increase of measured snow ablation rates at the upwind edge of the snow patch by analysing snow ablation rates from the laser scanner as a function of the fetch distance exemplarily for 1 day (21 May 2014) in the late ablation period 2014 with an already patchy snow cover (SCF = 40%) (Figure 5). For this example, we measured a mean snow height change of approximately 0.09 m day−1 at eight selected snow patches during a southern flow with a mean wind speed of 5 m s−1. On the upwind edge of these snow patches, the maximum snow ablation rates increase to 0.11 m, which corresponds to 25% larger snow ablation rates at the upwind edge due to local warm air advection. Additionally, we analyzed a second ablation day (25 May 2014) in the late ablation period 2014 during a southern flow with a SCF = 20%. We found locally 30% larger snow ablation rates at the upwind edge of the snow patch. In both experimental cases, local warm air advection influences snow ablation rates over a fetch distance of 5 m downwind the leading edge, which is in agreement with (Mott et al., 2011). However, this distance increases up to 15 m with increasing wind speed (Mott et al., 2011). As the development of separate snow patches did not occur in the ablation period 2015 due to a long-lasting strict snow line and 2016 was determined by a rapid decrease of the snow cover fraction (Mott et al., 2017; Appendix A), the investigation above could only be provided for the two ablation days in 2014.
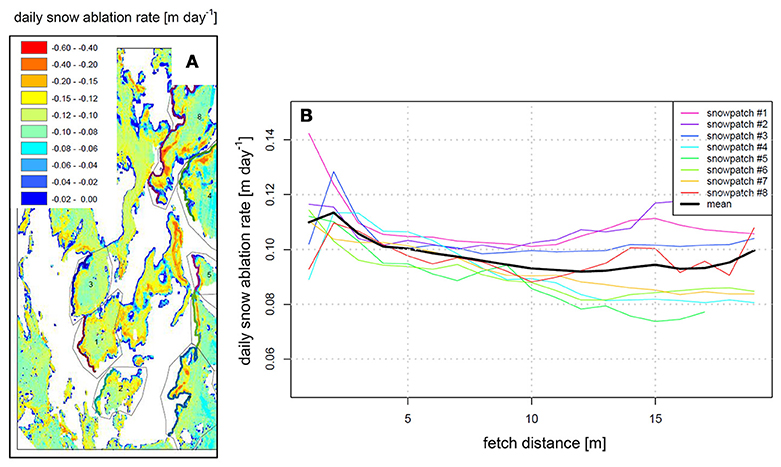
Figure 5. Snow ablation rates [m day−1] for 21 May 2014 at the Gletschboden test site. The dashed box in Figure 4A shows the location of the investigated area. (A). Eight different snow patches were analyzed to assess the daily snow ablation as a function of the fetch distance (B).
The local increase of snow ablation rates at the upwind edges contribute with 4% (SCF = 40%, 21 May 2014) and with 6% (SCF = 20%, 25 May 2014) to the total daily snow ablation rate of the entire catchment. The rather smaller contribution to the total snow ablation of the entire Gletschboden area in comparison with the enhanced snow ablation directly at the upwind edge of the presented snow patch is a result of the fetch distance distribution. As an example, for a given snow cover distribution at the Gletschboden area with a SCF = 40% only 30% of the snow-covered pixels have a smaller fetch distance than 5 m and are directly affected by local heat advection. A large fraction of snow-covered pixels further inside the snow patches is not affected by the leading edge effect (Figure S1). Thus, the contribution of local heat advection to the mean snow ablation on a catchment scale strongly depends on the fetch distance distribution. The fetch distance distribution itself is heavily dependent on the mean snow patch size. As the Gletschboden area is characterized by a rather homogeneous terrain, snow patches are relatively continuous with a mean snow patch size of approximately 30 x 30 m for SCF = 40 and 20 x 20 m for SCF = 20%.
The contribution of local heat advection to the mean snow ablation is expected to increase for regions with more heterogeneous surface characteristics, driving smaller snow patch sizes. Our calculations suggest that this increase in the contribution of local heat advection to the mean snow ablation rates can reach up to 12% for a mean patch size of 10 x 10 m and converges to the measured increase in snow ablation rates at the leading edge of 25–30% in the limiting case of a snow patch sizes smaller than 4 x 4 m (not shown). We found above-average snow ablation rates in comparison with snow ablation rates further inside the snow patch. However, the effect of local heat advection contributing to snow ablation additionally includes an increase in mean air temperatures and larger snow ablation rates further inside the snow patch, which are not directly affected by the “leading edge effect” but originated from a positive snow-albedo feedback and dirt or debris on the snow surface. Above-average snow ablation rates further inside the snow patch (in comparison with a continuous snow cover) cannot be shown from our laser scanner measurements, but are analyzed in the companion paper. In this study, near-surface air temperature fields were calculated with the non-hydrostatic atmospheric model ARPS for different snow cover fractions and used as input for the hydrological model Alpine3D, in which turbulent heat fluxes were calculated with the Monin-Obukhov bulk formulation. Numerical results reveal that above-average snow ablation rates further inside of the snow patch (in comparison to a continuous snow cover) are sensitive to varying snow cover fractions and wind velocities, but do not depend on the mean snow patch size.
Representation of Snow Patches in Alpine3D Before the Footprint Correction
The representation of the spatial and temporal dynamics of snow patches in Alpine3D is assessed in the late ablation period. The model performance was statistically analyzed by introducing a contingency table. The false alarm rate (model predicts snow, although the area is snow-free in the observation) is of special interest, as this model error can be related to the missing lateral heat transport. The proportion correct (correctly forecasted pixels), miss rates and false alarm rates are calculated for two ablation periods 2014 and 2015 as a function of the snow cover fraction (Figure 6). The model was initialized with the peak of winter snow distribution recorded with the scanner mid of April at a horizontal grid resolution of 1 m.
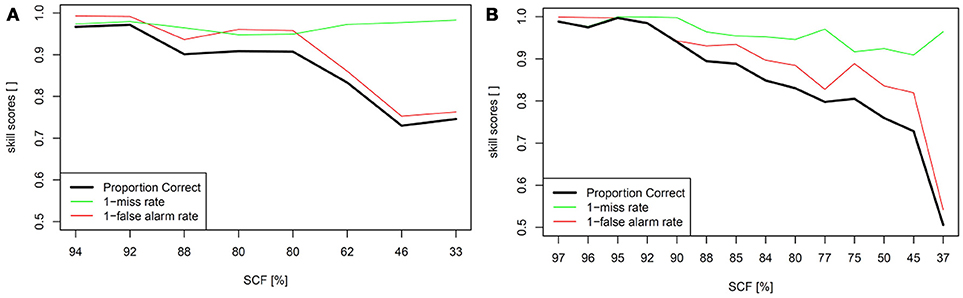
Figure 6. Skill score for 2014 (A) and 2015 (B) as a function of different snow cover fractions (SCF) for the Gletschboden area. Correctly forecasted pixels (black) and the error due to misses (green) and false alarm (red) are shown.
In both years, the proportion correct decreases with a decreasing fraction of snow. The proportion correct is above 0.95 as long as the snow cover is still continuous and decreases to 0.7 for SCF > 50%. In 2015, the values even decrease toward 0.5 for a fraction of snow smaller than 50%. This decrease in the proportion correct is mainly caused by the error due to the false alarm rates. A significant part of the false alarm rates is the result of the missing lateral transport of heat and moisture. Uncertainties in the parametrization of the incoming longwave radiation and uncertainties in the aerodynamic roughness length of snow and applied stability corrections are also assumed to contribute to differences in measured and modeled snow-cover distribution (Schlögl et al., 2017).
We assume that other external error sources (e.g., the small-scale spatial variability of the radiation or wind velocity in the catchment) can be neglected, as the investigated catchment is almost flat. However, both external error sources need to be considered in complex terrain, where turbulent sensible heat fluxes are strongly determined by slope winds.
Resolving Small-Scale Variations in Snow Ablation Rates by Applying the Temperature Footprint Approach
Near-surface air temperatures are recalculated with the temperature footprint approach for six analysis days (20 May 2014 – 25 May 2014) by initializing the model with the measured laser scanner snow distribution from 19 May 2014. We chose an initial snow distribution very close to the starting date of the analysis period instead of an initial snow distribution at the peak of winter in mid-April. This set-up better represents the location and size of the snow patches for the starting date of the analysis period and prevents a bias of around 20% in the correct model prediction of the snow patches by initializing the model with a snow cover distribution in mid-April, mainly caused by the false alarm rate (Figure 6A).
Near-surface air temperatures are exemplarily shown for the 20 May 2014 12 UTC (Figure 7 upper panels). Standard Alpine3D air temperatures over snow show almost no spatial variability (ΔTA = 0.1 K) (Figure 7A). After applying the temperature footprint approach however, near-surface air temperatures at a height of 0.01 m above the surface at the upwind edge of the snow patches are larger compared to further inside the snow patch (ΔTA = 6.1 K) (Figure 7B). For this specific example a southern flow leads to larger near-surface temperatures and larger snow ablation rates at the southern edge of the snow patch. For other days (e.g., 06 May 2014; Figure 4) we observed larger daily snow ablation rates in all directions around the snow patch caused by changing diurnal wind directions. The changing diurnal wind directions at the Gletschboden area are a typical phenomenon in larger valleys, where anabatic winds during noon follow katabatic winds in the morning (Hennemuth, 1986; Mott et al., 2017). By applying the temperature footprint approach, we were able to provide snow ablation maps where the small-scale variation as observed in the laser scanner measurements could be resolved.
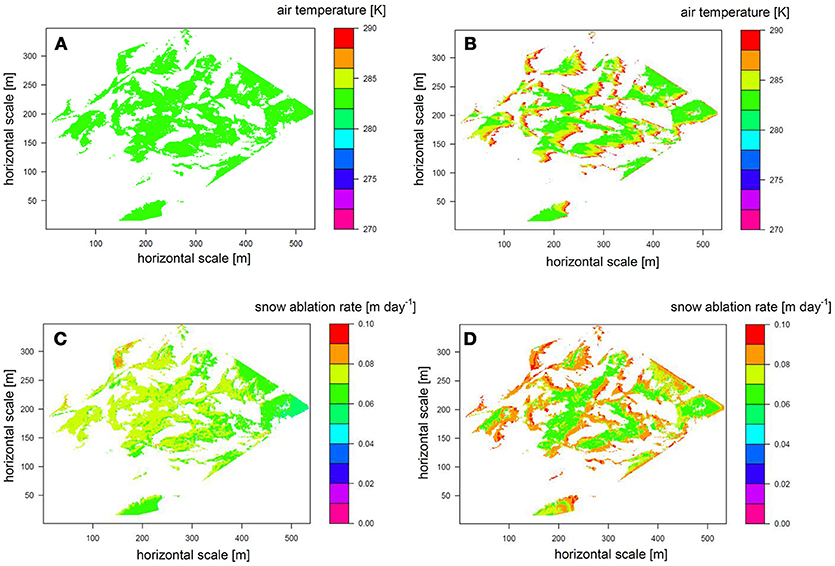
Figure 7. Near-surface air temperatures [K] (top panels) for the Gletschboden area for the 20 May 2014 12 UTC as input in Alpine3D before applying the temperature footprint approach (A) and after applying the temperature footprint approach (B). Modeled snow ablation rate [m day−1] for the Gletschboden area for the 20 May 2014 (bottom panels) before applying the temperature footprint approach (C) and after applying the temperature footprint approach (D).
In the following we analyse the increase of the mean near-surface air temperature over snow-covered pixels by applying the temperature footprint approach (Figure S2). The air temperature increase is highly correlated with the surface temperature of the adjacent bare ground. The adjacent bare ground is heated after sunrise, which leads to an increase in values of . Values of increase from 6 K (for a SCF = 40%) to 14 K (for a SCF = 20%) as a function of the snow cover fraction at noon. We found a 24 h mean value of K for six analysis days (20 May 2014 – 25 May 2014), with a mean wind velocity of 4 m s−1 and a mean surface temperature of the adjacent bare ground of 282 K. The strong sensitivity of the temperature footprint approach to surface temperatures of the adjacent bare ground and fraction of snow is further discussed in section Sensitivity Analysis for an idealized test site.
Model Performance of the Footprint Approach
We analyzed the model performance of Alpine3D before and after applying the footprint approach with respect to turbulent sensible heat fluxes for a special point and two-dimensional snow mask maps and snow ablation rates.
Turbulent Sensible Heat Fluxes
Modeled turbulent sensible heat fluxes are sufficiently simulated for SCF > 60%, but once the snow cover gets patchy and the fetch distance over snow decreases, measured turbulent sensible heat fluxes in 2 m above the surface are typically directed upwards, whereas modeled surface turbulent sensible heat fluxes are typically directed downwards (Figure 8A). This difference is mainly caused by the development of a stable internal boundary layer over snow patches, which is not resolved in the model. As turbulent fluxes are calculated with Monin-Obukhov similarity theory, which is exclusively applicable for constant flux layers extending to the first grid point in the atmosphere or the level of meteorological measurements, Monin-Obukhov similarity theory is typically not applicable over patchy snow covers.
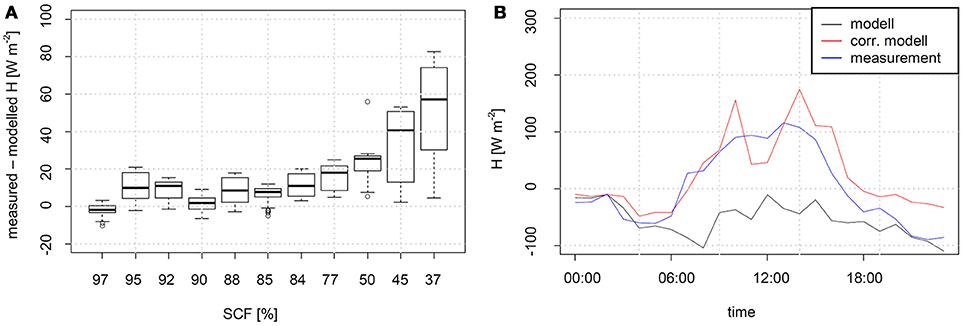
Figure 8. Difference between modeled surface turbulent sensible heat fluxes and measured turbulent sensible heat fluxes in 2 m above the surface [W m−2] at the eddy-covariance tower as a function of snow cover fraction (SCF) [%] (A). Turbulent sensible heat fluxes (H) for 18 May 2015 (SCF = 37%) at the eddy-covariance tower (B). The eddy-covariance measurement in 2 m above the surface is shown in blue, Alpine3D surface turbulent sensible heat flux is shown in black, turbulent sensible heat flux in 2 m above the surface after applying the flux footprint approach is shown in red.
The model performance of turbulent sensible heat fluxes for a single point could be significantly improved by applying the flux footprint approach (Figure 8B). Modeled turbulent sensible heat fluxes after applying the flux footprint approach represent the flux in 2 m above the surface and are compared against eddy-covariance measurements at the same height. For this example, the measurement height is located above the height of the stable internal boundary layer and the unstable boundary layer in upwind direction of the snow patch is taken into account by applying the flux footprint approach. Hence, model results after applying the flux footprint approach are more accurate to measured turbulent heat flux at the eddy-covariance tower than modeled surface turbulent sensible heat fluxes with Monin-Obukhov similarity theory. This example clearly demonstrates that an eddy-covariance measurement height of 2 m above the surface in the late ablation period is located to far from the surface in order to get information on turbulent heat exchange above the melting snow surface.
Snow Mask Maps
Snow mask maps have been analyzed before and after applying the temperature footprint approach (Figure 9) to test the improvement in representing snow patches in Alpine3D. Alpine3D was run in this specific example for 2 days (21–22 May 2014), initialized with the snow distribution of the laser scanner measurement from 21 May 2014. We choose this short period of 2 days in order to ensure no bias in mean snow ablation rates between model and laser scanner measurement. By initializing Alpine3D with snow distributions at the peak of winter mid of April, the proportion correct for the Gletschboden area is below 75% at end of May (Figure 6A). As this skill score is strongly dependent on different boundary layer parameters (e.g., the aerodynamic roughness length of snow or the stability correction) and correct input radiation, the improvement in Alpine3D model simulations by applying the temperature footprint approach would be masked by those uncertainties.
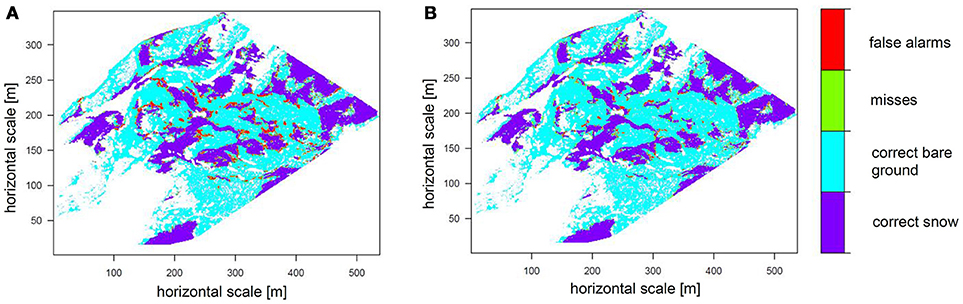
Figure 9. Snow mask map for the Gletschboden area for the 22 May 2014. Model correctly provides snow (dark blue), model correctly provides bare ground (light blue). False alarms are shown in red, misses are shown in light green. White colors indicate no data. Snow mask maps are shown without the temperature footprint approach (A) and with the temperature footprint approach (B).
The improvement in the model performance is clearly visible by comparing Figures 9A,B and is analytically expressed by calculating the false alarm rates. False alarm rates in the late ablation period are around 6% before applying the temperature footprint approach and could be decreased to around 3% after applying the temperature footprint approach. Hence, we were able to improve the Alpine3D model performance with respect to the size and location of snow patches in an Alpine catchment.
Local Snow Ablation Rates
Comparisons of measured and of simulated snow ablation rates with and without using the footprint approach are shown in Figure 10. Homogeneous standard Alpine3D snow ablation rates without applying the temperature footprint approach (Figure 4) do not resolve the enhanced snow ablation rates on the upwind edge of the snow patch (Figure 10A). Enhanced snow ablation rates at the upwind edge of the snow patch could be partly resolved by applying the temperature footprint approach. The improved representation of snow ablation rates at the upwind edge of the snow patches could also be observed for measured snow ablation rates above 0.15 m day−1 (b). While modeled standard Alpine3D snow ablation rates stay constant at measured snow ablation rates around 0.15 m day−1, snow ablation rates additionally increase after applying the temperature footprint approach. The Pearson correlation coefficient between measured and modeled snow ablation rates could be increased from 0.73 (before applying the temperature footprint approach) to 0.85 after the application of the temperature footprint approach for the two ablation days in 2014.
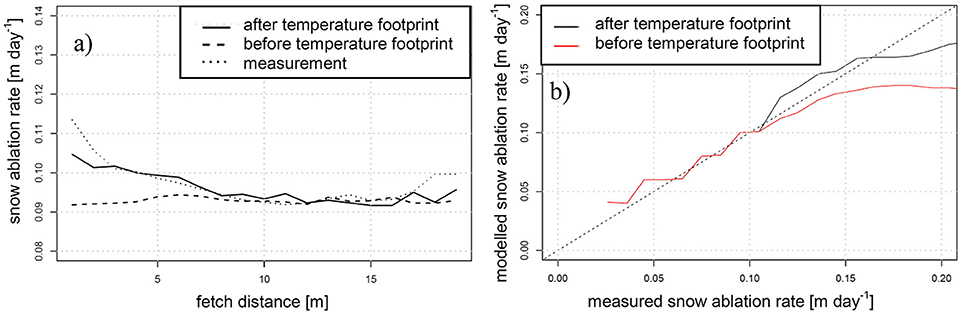
Figure 10. Snow ablation rate for the 21 May 2014 [m day−1] as a function of the fetch distance [m] (A) for the terrestrial laser scanning measurement and the model results with and without applying the temperature footprint approach. Snow ablation rate [m day−1] for the model results (with and without applying the temperature footprint approach) (y-axis) as a function of the measured snow ablation (x-axis) (B).
Sensitivity Analysis
We analyse the sensitivity of major results from the temperature footprint approach by creating artificial snow cover distributions with varying fraction of snow from 5-95% and a varying number of snow patches from 1 to 36. Additionally to varying snow cover distributions, the horizontal scale of the domain and meteorological conditions (soil temperature and wind velocity) are modified (Table 1) and compared with results from the Gletschboden area (K). In summary, we modified 5 parameters in order to assess the differences in enhanced air temperature by the temperature footprint approach .
The value of is equal to 3.3 K using all default values in Table 1 and increases from 0.2 K to 5.4 K by varying the fraction of snow from 95% to 5% as long as the remaining four parameter were not modified. The values of for the modification of the other parameters are shown in Table 1. In summary, four parameters are strongly sensitive to modifications with a similar spread in values of from 1-5 K, except the modification of the number of snow patches. Modifying more than one parameter could lead to much larger values of than 5 K. A large number of snow patches and a small fraction of snow lead to values of up to 10 K (Figure S3). Increasing the horizontal scale of the model domain lowers values of , as the snow patch size increases and a larger percentage of snow-covered pixels is not affected by lateral transport of sensible heat (Figure S4). High wind velocities and high surface temperatures of the adjacent bare ground lead to large values of (Figure S5).
Discussion
We discuss the overall model error caused from neglecting lateral transport processes as found for the Gletschboden area and compare it to a second test site, which has been analyzed previously (Mott et al., 2015). The split of the overall model error in a contribution from the pure “leading edge effect” and a contribution from an increase in the mean air temperature due to a positive snow-albedo feedback is maintained (Table 2). We presented the contribution of enhanced snow ablation from the pure “leading edge effect” at the upwind edge, for an entire catchment and for an entire catchment and entire ablation period by using snow ablation rates further inside the snow patch as a reference. Based on the analysis of high-resolution snow ablation data of two ablation days at the Gletschboden test site, the local advection of warm air causes 25–30% more snow ablation at the upwind edge of a single snow patch and contributes 4–6% to the total snow ablation of the entire catchment. 4–6% additional snow ablation due to local warm air advection at the patch boundaries has been found for the specific snow distributions observed at the Gletschboden test site for the two analysis days late in the ablation period. The snow cover at the Gletschboden test site in the ablation period remains continuous for around 80% of the ablation period and the snow cover fraction rapidly decreases afterwards (Figure 11A). Normalized snow depth ablation rates are almost constant throughout the ablation period and slightly increase toward the end of the ablation period. Based on this information, we found almost no contribution of the pure effect of local heat advection to snow ablation in the first 80% of the ablation period, as the snow cover remains continuous. In the late ablation period (last 20%) the contribution of the local heat advection to snow ablation steadily increases from 4% (SCF = 40%) to 6% (SCF = 20%) and finally up to 25–30% for a snow patch size smaller than 4 × 4 m. By integrating over the entire ablation period, the consideration of the pure “leading edge effect” increases the total seasonal snow ablation approximately 1–3%. Note that numbers are valid for the Gletschboden area and may only be transferable to regions with a similar snow patch size distribution.
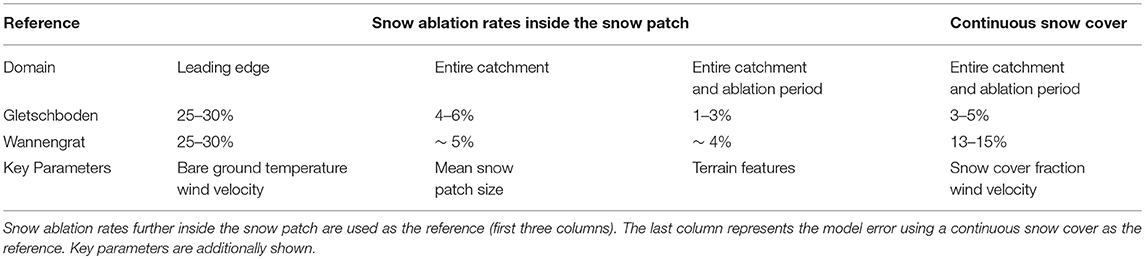
Table 2. Fraction of above-average snow ablation rates [%] relative to snow ablation rates inside the snow patch and relative to a continuous snow cover for the Gletschboden and Wannengrat test site due to lateral transport processes at the leading edge of a snow patch, for the entire catchment and for the entire catchment and ablation period.
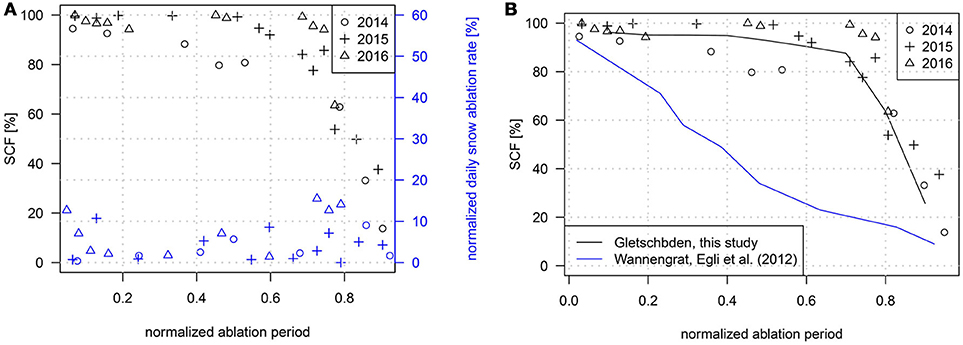
Figure 11. Snow cover fraction (SCF) [%] and normalized daily snow ablation rate [%] as a function of the normalized ablation period for 2014–2016 at the Gletschboden test site (A). SCF [%] as a function of the normalized ablation period for the Gletschboden test site (black) and the Wannengrat test site (blue, Egli et al., 2012) (B). Results are based on terrestrial laser scanning measurements.
Based on existing data sets and model estimations, we could also estimate the leading edge effect, mean snow patch size and the relative duration of patchy snow covers during the ablation period for a second test site. The Wannengrat area (Davos, Switzerland) is more than one order of magnitude larger than the Gletschboden test site and located in highly complex terrain including steep slopes of different aspects. Further information about the Wannengrat area can be found in Egli et al. (2012) and Mott et al. (2011, 2015). For this analysis, we used mean perimeters of the snow patches for different snow-covered areas estimated in Mott et al. (2015) for the Wannengrat area by simply applying a constant melting rate to the snow depth distribution at peak of winter accumulation, which has been shown to yield correct statistics of important parameters such as the snow cover fraction (Egli et al., 2012).
Similar to what we found for the Gletschboden test site, Mott et al. (2011) found up to 25–30% above-average snow ablation rates at the leading edge for the Wannengrat test site. The mean snow patch size during similar snow cover fraction values is slightly larger for the Wannengrat test site than for the Gletschboden test site (SCF = 40%: 30 m Gletschboden vs. 32 m Wannengrat; SCF = 20%: 20 m Gletschboden vs. 25 m Wannengrat). Also similar to what has been calculated for the test site Gletschboden, above-average snow ablation rates decrease from 30% at the leading edge to approximately 5% for the entire Wannengrat catchment for one specific day in the late ablation period.
Patchy snow covers dominate the entire ablation period for the Wannengrat area, where a SCF <50% was observed for the last 70% of the ablation period (Egli et al., 2012; Figure 11B). The Wannengrat test site is characterized by much higher snow depth heterogeneity at peak of winter accumulation than the flatter Gletschboden test site, resulting in a much longer duration of the patchy snow cover phase. By integrating over the entire ablation period, the consideration of the pure “leading edge effect” at the Wannengrat catchment (approximately 4%) is larger than for the Gletschboden area (1–3%), particularly due to a much larger relative duration of patchy snow covers.
However, estimated model errors described above only consider the “leading edge effect.” This local effect does not account for the stronger snow ablation over the entire snow patch area caused by an increase in the mean air temperature due to a positive snow-albedo feedback. This additional effect could not be captured by laser scanner measurements in this study but is assessed in the companion paper by analysing the effect of varying snow cover fractions on near-surface air temperatures calculated with the non-hydrostatic atmospheric model ARPS. Numerical results reveal that mean snow ablation rates of snow patches (also for larger fetch distances than represented by the leading edge effect) are strongly sensitive to a varying fraction of snow and wind velocities and robust to the mean snow patch size.
Based on sensitivity runs performed in the companion paper, we compared mean snow ablation rates for a different fraction of snow to mean snow ablation rates over a continuous snow cover. Mean snow ablation rates for a different fraction of snow in combination with measured relative duration of patchy snow covers at the Wannengrat and the Gletschboden area were used to estimate the overall model error for the entire ablation period.
The overall model error by neglecting lateral transport processes is larger for the Wannengrat area (13–15%) than for the Gletschboden area (3–5%) (Table 2). Larger model errors for the Wannengrat area can be explained by the high sensitivity of numerical results to varying snow cover fraction and the relative duration of a patchy snow cover during the ablation period, which is much larger for the Wannengrat area. At the Gletschboden test site, above-average snow ablation from lateral transport processes can be split in 50% contribution from the pure “leading edge effect” and 50% contribution from the mean air temperature increase from a positive snow-albedo feedback. For the Wannengrat area, the positive snow-albedo feedback is larger than the pure “leading edge effect,” mainly caused by a small relative duration of patchy snow covers within the ablation period.
Conclusion
We analyzed the limited model performance of the physics based surface process model Alpine3D in calculating snow ablation rates over patchy snow covers, as Alpine3D neglects the spatial variability of near-surface air temperatures over patchy snow covers. Snow ablation rates at the upwind edge of a snow patch are distinctly underestimated in the model, as higher near-surface air temperatures due to local heat advection are not resolved. In this study, we applied a temperature footprint approach on near-surface air temperature fields, a technique originally deployed for eddy-covariance flux analysis. This conceptual model approach correctly predicts enhanced snow ablation rates at the upwind edge of snow patches, as recalculated fetch-dependent near-surface air temperatures are enhanced in this region. The analysis of the model performance of the footprint approach with respect to turbulent sensible heat fluxes for a single point and two-dimensional snow mask maps and snow ablation rates show that Alpine3D model results could be significantly improved.
Modeled turbulent sensible heat fluxes over patchy snow covers at 2 m above ground are mostly directed upwards during noon after the application of the flux footprint approach and therefore correspond much better to eddy-covariance measurements. In addition, two-dimensional snow mask maps and local snow ablation rates could be significantly better simulated by applying the temperature footprint approach. However, 25% larger ablation rates at the upwind edge of the snow patch (in comparison with further inside of the snow patch) cannot be completely explained by the implemented model approach (Figure 10A). We were able to explain around 15% (out of 25%) above-average snow ablation rates at the upwind edge with the temperature footprint approach. This underestimation might be partly caused by an enhanced incoming longwave radiation in regions of enhanced air temperatures, as incoming longwave radiation increases with increasing air temperature. Additionally, the advection of latent heat is not resolved in this approach and could contribute to enhanced snow ablation at the upwind edge (Harder et al., 2017).
We analyzed the total model error by neglecting lateral transport processes and separated the total model error in two contributions: The pure “leading edge effect” and a mean air temperature increase from a positive snow-albedo feedback. We compared model errors of the Gletschboden test site with those estimated for the Wannengrat area in highly complex terrain including slopes of different aspects. The total model error for an entire ablation period by neglecting lateral transport processes is larger for the Wannengrat area (approximately 14%) than for the Gletschboden area (approximately 4%) which is explained by the longer patchy snow cover duration at the Wannengrat area. For the Wannengrat area, the increase in the mean air temperature from a positive snow-albedo feedback is larger than the pure “leading edge effect”, whereas both effects show a similar contribution to the overall model error for the Gletschboden area.
In summary, we were able to improve Alpine3D snow ablation rates by applying a temperature footprint approach on near-surface air temperatures over patchy snow covers. Enhanced snow ablation rates at the upwind edge of snow patches due to lateral transport of sensible heat could be resolved. The consideration of small-scale lateral transport processes is a fundamental investigation and complements e.g., a study of (Helbig et al., 2015), who parametrized snow covered areas on larger scales up to 3 km.
Uncertainties in modeled snow ablation rates are mostly determined by uncertainties in the boundary layer parameters (e.g., roughness length or stability corrections) and the parametrization of the incoming longwave radiation (Schlögl et al., 2016). Monin-Obukhov similarity theory model errors are strongly dependent on the chosen stability correction and significantly contribute to uncertainties in modeled snow ablation rates even over a continuous snow cover (Schlögl et al., 2017). In this study, we showed that Monin-Obukhov similarity theory model errors significantly increase over patchy snow covers. In the late ablation period (SCF < 50%), uncertainties in modeled snow ablation rates are partly determined by the complex interaction between the heterogeneous snow cover and the atmosphere (Mott et al., 2017).
Author Contributions
SS did the fieldwork, analysis and wrote the manuscript. ML and RM conceived and supervised the project, provided guidance, helped with the analysis and revised the manuscript. CF helped with the fieldwork, supervised the project and revised the manuscript.
Funding
The work was funded by Swiss National Science Foundation (Project: Snow-atmosphere interactions driving snow accumulation and ablation in an Alpine catchment: The Dischma Experiment; SNF-Grant: 200021_150146 and Project: The sensitivity of very small glaciers to micrometeorology. P300P2_164644).
Conflict of Interest Statement
The authors declare that the research was conducted in the absence of any commercial or financial relationships that could be construed as a potential conflict of interest.
Acknowledgments
We would like to thank Franziska Gerber, Lisa Dirks, Luis Queno, Christian Sommer, Prisco Frei and Urs Kühne for their help during the field work.
Supplementary Material
The Supplementary Material for this article can be found online at: https://www.frontiersin.org/articles/10.3389/feart.2018.00120/full#supplementary-material
Figure S1. Percentage of snow covered areas with a fetch distance below 5 m (blue) and below 15 m (red) estimated for the Gletschboden area for several different snow cover fractions (SCF) in the ablation period 2014.
Figure S2. Values of [K] (Equation 2; averaged over snow covered pixels) as a function of the local time for 6 different analysis days in the late ablation period 2014.
Figure S3. [K] (Equation 2; averaged over snow covered pixels) as a function of the snow cover fractions (SCF) [%] and the number of snow pixels. Default values were used for the non-modified parameter (Table 1).
Figure S4. [K] (Equation 2; averaged over snow covered pixels) as a function of the horizontal scale [m] of the catchment for several different snow cover fractions (SCF). Default values were used for the non-modified parameter (Table 1).
Figure S5. [K] (Equation 2; averaged over snow covered pixels) as a function of the wind velocity [m s−1] and temperature of the adjacent bare ground [°C]. Default values were used for the non-modified parameter (Table 1).
References
Bavay, M., Lehning, M., Jonas, T., and Löwe, H. (2009). Simulations of future snow cover and discharge in Alpine headwater catchments. Hydrol. Process. 23, 95–108. doi: 10.1002/hyp.7195
Blanc, T. (1987). Accuracy of bulk-method-determined flux, stability, and sea surface roughness. J. Geophys. Res. Atmos. 92, 3867–3876. doi: 10.1029/JC092iC04p03867
Comola, F., Schaefli, B., Rinaldo, A., and Lehning, M. (2015). Scale-dependent effects of solar radiation patterns on the snow-dominated hydrologic response. Geophys. Res. Lett. 42, 3895–3902. doi: 10.1002/2015GL064075.
Egger, J. (1983). Pressure distributions in the dischma valley during field experiment DISKUS. Beitr. Phys. Atmos. 56, 163–176.
Egli, L., Jonas, T., Grünewald, T., Schirmer, M., and Burlando, P. (2012). Dynamics of snow ablation in a small Alpine catchment observed by repeated terrestrial laser scans. Hydrol. Process. 26, 1574–1585. doi: 10.1002/hyp.8244
Essery, R., Granger, R., and Pomeroy, J. (2006). Boundary-layer growth and advection of heat over snow and soil patches: modeling and parameterization. Hydrol. Process. 20, 953–967. doi: 10.1002/hyp.6122
Fujita, K., Hiyama, K., Iida, H., and Ageta, Y. (2010). Self-regulated fluctuations in the ablation of a snow patch over four decades. Water Resour. Res. 46:W11541. doi: 10.1029/2009WR008383
Gallice, A., Bavay, M., Brauchli, T., Comola, F., Lehning, M., and Huwald, H. (2016). StreamFlow 1.0: an extension to the spatially distributed snow model Alpine3D for hydrological modeling and deterministic stream temperature prediction. Geosci. Model Dev. 9, 4491–4519. doi: 10.5194/gmd-9-4491-2016
Garratt, J. R. (1990). The internal boundary layer–A review. Bound. Lay. Meteorol. 50, 171–203. doi: 10.1007/BF00120524
Gerber, F., Lehning, M., Hoch, S. W., and Mott, R. (2017). A Close-ridge small-scale atmospheric flow field and its influence on snow accumulation. J. Geophys. Res. Atmos. 122, 7727–7754. doi: 10.1002/2016JD026258
Granger, R. J., Essery, R., and Pomeroy, J. W. (2006). Boundary-layer growth over snow and soil patches: field observations. Hydrol. Process. 20, 943–951. doi: 10.1002/hyp.6123
Granger, R. J., Pomeroy, J. W., and Parviainen, J. (2002). Boundary-layer integration approach to advection of sensible heat to a patchy snow cover. Hydrol. Process. 16, 3559–3569. doi: 10.1002/hyp.1227
Groot Zwaaftink, C. D., Löwe, H., Mott, R., Bavay, M., and Lehning, M. (2011). Drifting snow sublimation: a high-resolution 3-D model with temperature and moisture feedbacks. J.Geophys.Res. Atmos. 116:D16107. doi: 10.1029/2011JD015754
Groot Zwaaftink, C. D., Mott, R., and Lehning, M. (2013). Seasonal simulation of drifting snow sublimation in Alpine terrain. Water Resour. Res. 49, 1581–1590. doi: 10.1002/wrcr.20137
Harder, P., Pomeroy, J. W., and Helgason, W. (2017). Local scale advection of sensible and latent heat during snowmelt. Geophys. Res. Lett. 44, 9769–9777. doi: 10.1002/2017GL074394
Helbig, N., van Herwijnen, A., Magnusson, J., and Jonas, T. (2015). Fractional snow-covered area parameterization over complex topography. Hydrol. Earth Syst. Sci. 19, 1339–1351. doi: 10.5194/hess-19-1339-2015
Helgason, W., and Pomeroy, J. (2012). Problems closing the energy balance over a homogeneous snow cover during midwinter. J. Hydrometeorol. 13, 557–572. doi: 10.1175/JHM-D-11-0135.1
Hennemuth, B. (1986). Thermal asymmetry and cross-valley circulation in a small Alpine valley. Bound. Lay. Meteorol. 36, 371–394. doi: 10.1007/BF00118338
Kljun, N., Rotach, M. W., and Schmid, H. P. (2002). A three-dimensional backward lagrangian footprint model for a wide range of boundary-layer stratifications. Bound. Layer Meteorol. 103, 205–226. doi: 10.1023/A:1014556300021
Lehning, M., Völksch, I., Gustafsson, D., Nguyen, T. A., Stähli, M., and Zappa, M. (2006). ALPINE3D: a detailed model of mountain surface processes and its application to snow hydrology. Hydrol. Process. 20, 2111–2128. doi: 10.1002/hyp.6204
Liston, G. E. (1995). Local advection of momentum, heat, and moisture during the melt of patchy snow covers. J. Appl. Meteorol. 34, 1705–1715. doi: 10.1175/1520-0450-34.7.1705
Liston, G. E. (1999). Interrelationships among snow distribution, snowmelt, and snow cover depletion: implications for atmospheric, hydrologic, and ecologic modeling. J. Appl. Meteorol. 38, 1474–1487. doi: 10.1175/1520-0450(1999)038<1474:IASDSA>2.0.CO;2
Mahrt, L., and Vickers, D. (2005). Boundary-Layer adjustment over small-scale changes of surface heat flux. Bound. Lay. Meteorol. 116, 313–330. doi: 10.1007/s10546-004-1669-z
Marks, D., and Dozier, J. (1992). Climate and energy exchange at the snow surface in the alpine region of the sierra Nevada. 2. snow cover energy balance. Water Resour. Res. 28, 3043–3054. doi: 10.1029/92WR01483
Marks, D., and Winstral, A. (2001). Comparison of snow deposition, the snow cover energy balance, and snowmelt at two sites in a semiarid mountain basin. J. Hydrometeorol. 2, 213–227. doi: 10.1175/1525-7541(2001)002<0213:cosdts>2.0.co;2
Marsh, P., and Pomeroy, J. W. (1996). Meltwater fluxes at an arctic forest-tundra site. Hydrologic. Process. 10, 1383–1400. doi: 10.1002/(SICI)1099-1085(199610)10:10<1383::AID-HYP468>3.0.CO;2-W
Mott, R., Daniels, M., and Lehning, M. (2015). Atmospheric flow development and associated changes in turbulent sensible heat flux over a patchy mountain snow cover. J. Hydrometeor. 16, 1315–1340. doi: 10.1175/JHM-D-14-0036.1
Mott, R., Egli, L., Grünewald, T., Dawes, N., Manes, C., Bavay, M., et al. (2011). Micrometeorological processes driving snow ablation in an Alpine catchment. Cryosphere 5, 1083–1098. doi: 10.5194/tc-5-1083-2011
Mott, R., Gromke, C., Grünewald, T., and Lehning, M. (2013). Relative importance of advective heat transport and boundary layer decoupling in the melt dynamics of a patchy snow cover. Adv. Water Res. Vol. 55, 88–97. doi: 10.1016/j.advwatres.2012.03.001
Mott, R., Paterna, E., Horender, S., Crivelli, P., and Lehning, M. (2016). Wind tunnel experiments: cold-air pooling and atmospheric decoupling above a melting snow patch. Cryosphere 10, 445–458. doi: 10.5194/tc-10-445-2016
Mott, R., Schlögl, S., Dirks, L., and Lehning, M. (2017). Impact of extreme land-surface heterogeneity on micrometeorology over spring snow-cover. J. Hydrometeor. 18, 2705–2722. doi: 10.1175/JHM-D-17-0074.1
Pellicciotti, F., Helbing, J., Rivera, A., Favier, V., Corripio, J., Araos, J., et al. (2008). A study of the energy balance and melt regime on Juncal Norte Glacier, semi-arid Andes of central Chile, using melt models of different complexity. Hydrologic. Process. 22, 3980–3997. doi: 10.1002/hyp.7085
Pomeroy, J., Toth, B., Granger, R. J., Hedstrom, N. R., and Essery, R. L. H. (2003). Variation in surface energetics during snowmelt in a subarctic mountain catchment. J. Hydrometeorol. 4, 702–719. doi: 10.1175/1525-7541(2003)004<0702:VISEDS>2.0.CO;2
Schaefli, B., Hingray, B., and Musy, A. (2007). Climate change and hydropower production in the Swiss Alps: quantification of potential impacts and related modeling uncertainties. Hydrol. Earth Syst. Sci. 11, 1191–1205. doi: 10.5194/hess-11-1191-2007
Schlögl, S., Lehning, M., Nishimura, K., Huwald, H., Cullen, N. J., and Mott, R. (2017). How do stability corrections perform in the stable boundary layer over snow? Bound. Lay. Meteorol. 165, 161–180. doi: 10.1007/s10546-017-0262-1
Schlögl, S., Marty, C., Bavay, M., and Lehning, M. (2016). Sensitivity of Alpine3D modeled snow cover to modifications in DEM resolution, station coverage and meteorological input quantities. Environ. Model. Softw. 83, 387–396. doi: 10.1016/j.envsoft.2016.02.017
Schmid, H. P. (1994) Source area for scalars and scalar fluxes. Bound. Layer Meteorol. 67, 293–318. doi: 10.1007/BF00713146
Schuepp, P. H., Leclerc, M. Y., Macpherson, J. I., and Desjardins, R. L. (1990). Footprint Prediction of scalar fluxes from analytical solutions of the diffusion equation. Bound. Lay. Meteorol. 50, 255–373. doi: 10.1007/BF00120530
Weisman, R. N. (1977). Snowmelt: a two-dimensional turbulent diffusion model. Water Res. Res. 13, 337–342. doi: 10.1029/WR013i002p00337
Wever, N., Comola, F., Bavay, M., and Lehning, M. (2017). Simulating the influence of snow surface processes on soil moisture dynamics and streamflow generation in an alpine catchment. Hydrol. Earth Syst. Sci. 21, 4053–4071. doi: 10.5194/hess-21-4053-2017
Appendix
Diverse snow patch development Earlier studies (e.g., Mott et al., 2011) suggest that the snow patch development in an ablation season is similar in different years and snow patches evolve at the same location. We confirm this statement for the ablation periods 2014 and 2016 at the Gletschboden test site, where the snow patch development is similar.
However, we found for our test site that the development of snow patches is distinctly different for the years 2014 and 2015 (Figure A1). The formation of isolated snow patches is predominant in 2014, whereas in 2015 a strict snow line develops from the northern, lower elevated part and the snow cover is influenced by a huge avalanche from the north eastern slope. In the following we tested three hypotheses which could explain the different development:
1. Snow cover at peak accumulation
2. Zero degree level and elevation gradient in snow height during the snow season
3. Turbulent sensible heat fluxes in the ablation period
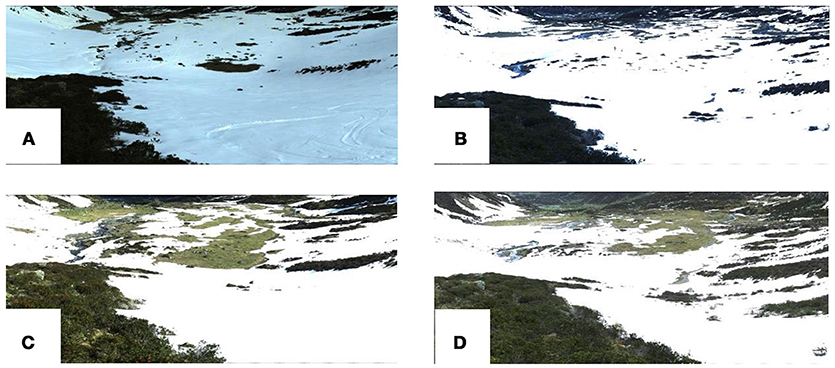
Figure A1. Panoramic images of the Gletschboden area recorded from the scan position for the early ablation period (SCF = 80%) (A,B) and the late ablation period (SCF = 50%) (C,D). Images on the left are recorded in 2014 (08 May 2014: A; 21 May 2014: C) and images on the right are recorded in 2015 (11 May 2015: B; 16 May 2015: D).
We tested if the snow cover at peak accumulation significantly differs between 2014 and 2015 (Figure A2a). The snow height at the beginning of the measurements in April was larger in 2015, but highly correlated with snow heights in 2014 (R2 = 0.91). Despite the large correlation coefficient some very small-scale differences in the snow height have been detected at the slope toe of the northern-exposed slope. Snow could efficiently be transported to the toe of the slope in 2015, resulting in much larger snow heights in this area compared to 2014 (Figure A2b). These small-scale differences in snow heights could be explained by different wind directions during mid-winter snowfall events. Snowfall events in 2014 typically occurred during a southern flow, whereas the wind direction during snowfall events was mainly north in 2015, leading to an efficient snow transport toward the toe of northern-exposed slope (Gerber et al., 2017).
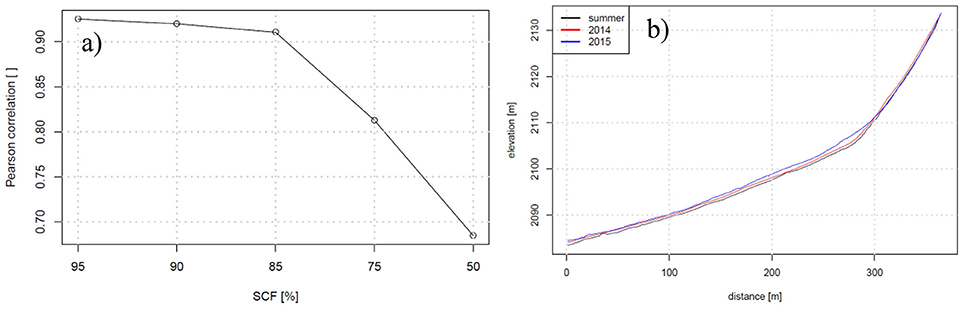
Figure A2. Comparison of the Alpine snow cover at Gletschboden in 2014 and 2015: Pearson correlation coefficient of the snow height of both years as a function the snow cover fraction (a). Cross section of digital elevation model for summer and the time of peak accumulation 2014 and 2015 (b).
We observed a shallow snow pack at the slope toe in 2014 at peak accumulation and a development of a bare ground patch in this area early in the ablation period (see bare ground at the slope toe in Figure A1A). From this early state in the ablation period the Pearson correlation coefficient rapidly decreases to 0.7 during the course of the ablation period which analytically describes the different development of the snow patches (Figure A2a). Hence, snow melting in 2014 is mainly initialized by the snow-free area at the slope toe and develops starting from this area, whereas snow melting in 2015 is mainly initialized by the northern (lower elevated) parts of Gletschboden.
The initialization of snow melting from the lower elevated parts in the north is favored by a strong elevation gradient of the snow height in 2015, which could be partly explained by a long-lasting zero degree level close to the mean elevation of the catchment. The snow height in regions above 2300 m asl was deep at the time of peak accumulation whereas the surface was already snow-free at the same time in 1,700 m asl. The elevation gradient of the snow height was much smaller in 2014.
The third hypothesis tests different local heat advection directions in 2014 and 2015. As the wind direction in the ablation period 2014 and 2015 do not significantly differ (not shown), local heat advection acts similarly on the patchy snow cover. We expect that local heat advection from different directions as a consequence of different wind directions play a minor role in different snow patch developments. This assumption is caused by the fact that wind directions in the entire ablation periods are typically similar.
In summary, the different development of the Alpine snow patches in the Gletschboden area in 2014 and 2015 is mainly caused by a different peak accumulation due to different wind directions during mid-winter snowfall events and due to different elevation gradients of the snow height. This result cannot be generalized to other test sites and ablation periods. There might be years of a very similar snow patch development (as in 2014 and 2016) if the snow cover at peak accumulation, the wind direction in the ablation period and the elevation gradient of the snow height are similar.
Keywords: eddy-covariance measurement, patchy snow cover, snow ablation rate, temperature footprint approach, terrestrial laser scanning, sensible heat flux
Citation: Schlögl S, Lehning M, Fierz C and Mott R (2018) Representation of Horizontal Transport Processes in Snowmelt Modeling by Applying a Footprint Approach. Front. Earth Sci. 6:120. doi: 10.3389/feart.2018.00120
Received: 14 November 2017; Accepted: 30 July 2018;
Published: 08 October 2018.
Edited by:
Thomas Vikhamar Schuler, University of Oslo, NorwayReviewed by:
Danny Marks, Agricultural Research Service (USDA), United StatesJean Emmanuel Sicart, Institut de Recherche pour le Développement (IRD), France
Copyright © 2018 Schlögl, Lehning, Fierz and Mott. This is an open-access article distributed under the terms of the Creative Commons Attribution License (CC BY). The use, distribution or reproduction in other forums is permitted, provided the original author(s) and the copyright owner(s) are credited and that the original publication in this journal is cited, in accordance with accepted academic practice. No use, distribution or reproduction is permitted which does not comply with these terms.
*Correspondence: Sebastian Schlögl, c2ViYXN0aWFuLnNjaGxvZWdsQHNsZi5jaA==