- 1Biomedical Engineering Laboratory, School of Electrical and Computer Engineering, National Technical University of Athens, Athens, Greece
- 2Unit of Medical Technology and Intelligent Information Systems, Department of Material Science and Engineering, University of Ioannina, Ioannina, Greece
- 3Department of Neurology, Medical School, University of Ioannina, Ioannina, Greece
- 4Department of Biomedical Research, Institute of Molecular Biology and Biotechnology, Foundation for Research and Technology - Hellas, Ioannina, Greece
This review focuses on virtual coaching systems that were designed to enhance healthcare interventions, combining the available sensing and system-user interaction technologies. In total, more than 1,200 research papers have been retrieved and evaluated for the purposes of this review, which were obtained from three online databases (i.e.,PubMed, Scopus and IEEE Xplore) using an extensive set of search keywords. After applying exclusion criteria, the remaining 41 research papers were used to evaluate the status of virtual coaching systems over the past 10 years and assess current and future trends in this field. The results suggest that in home coaching systems were mainly focused in promoting physical activity and a healthier lifestyle, while a wider range of medical domains was considered in systems that were evaluated in lab environment. In home patient monitoring with IoT devices and sensors was mostly limited to activity trackers, pedometers and heart rate monitoring. Real-time evaluations and personalized patient feedback was also found to be rather lacking in home coaching systems and this is the most alarming find of this analysis. Feasibility studies in controlled environment and an ongoing active research on Horizon 2020 funded projects, show that the future trends in this field are aiming to close the loop with automated patient monitoring, real-time evaluations and more precise interventions.
Introduction
Coaching systems are on a surge of increasing popularity for both every day and medical applications. The primary driving forces for advancement have been: (a) the enormous growth of access to cheaper smart devices, including smartphones, smartwatches, activity trackers, and in home sensing devices (1), (b) the prevalence of design and development focusing on seamless interconnectivity among them (i.e., Internet of Things, IoT) (2), (c) technology acceptance, as people are becoming increasingly aware and familiar with the added benefits of the functionality that is provided (3), (d) increasing reliable internet access, especially through wireless smartphone connectivity, and (e) the continuous increase in mobile and edge processing power, which raises the possibilities of what can be practically achieved (4). Fitness training and activity monitoring was the killer application that brought massive attention and demand in the field, highlighting the need for more data gathering (i.e., better sensing technologies) and most importantly, the need for advanced data interpretation algorithms and personalized user feedback based on these data (i.e., precise coaching). Machine learning, artificial intelligence and advanced data analytics are the major contributors for making more sense from the sensing data. In the medical and healthcare field, coaching systems have been more relevant for chronic disease patient management and disease modifying behavioral changes, in an attempt to guide and improve intervention outcomes (5).
Virtual coaching is an emerging field in the healthcare domain, which is primarily aiming at improving the personalized user-system interaction. This is crucial for promoting patient engagement and compliance, both of which are necessary for achieving long-term behavioral changes and adaptation of a healthier life-style (6). A virtual interface (e.g., virtual environments, virtual, or augmented reality) can be more effective in creating a more enjoyable experience for the end user, while offering the opportunity to create bounding conditions between virtual objects or human-like avatars. The later can significantly affect the quality of the user-system interaction and adherence to intervention (7). Virtual coaching helps in creating a more immersive experience, which in turn makes patients being more focused in setting and achieving higher goals, instead of following a more lightly-hearted approach.
Virtual coaching can be also most affective in the ends of the age spectrum; children, adolescents, and younger adults on the one end and elders on the other. The first are typically the most aware regarding the latest technological trends and most actively willing to be exposed to new and enticing approaches, including virtual scenarios and gamified coaching solutions. To some extent this is also true for older adults on the other end, but virtual coaching is primarily beneficial for the ease of interpretation it can provide and the enhanced guidance in every step of the intervention. Considering that aging is directly associated with the majority of the neurological disorders that lead to cognitive impairment, coaching strategies for this population group are most often limited in terms of the level of complexity that can be supported (8). The usefulness of the straightforward interactions of a virtual system are also beneficial for young patient populations, who experience equally challenging cognitive and learning difficulties (e.g., Autism spectrum disorders) (9, 10).
Previous review studies in the field of virtual coaching have been conducting focusing on systems that promote self-care (11), physical activity and rehabilitation (12), managing insomnia (13), self-tracking and e-coaching for healthy lifestyles (14), conversational agents and avatars in psychology (15). The main objective of this review is to examine the current status and trends of virtual coaching systems in healthcare, with an emphasis on systems that provide automated patient evaluations and appropriate feedback through virtual environments based on the information being gathered. The primary outcome of this study is to showcase automated coaching systems, use cases of active patient monitoring solutions based on sensing data from IoT devices for advanced feedback, the inclusion of automated real-time evaluations of sensor data (e.g., posture and gait analysis as a subject is moving), and the initiation of appropriate intervention from the virtual coach based on this information (e.g., update instructions, dynamically guide and educate the patient, showcase correct movement when an inappropriate one is detected, etc.). A literature review over the past 10 years is performed to assess recent advancements in the adaptation of such novel technologies for virtual coaching systems that are designed to operate independently in home (i.e., uncontrolled environment), and to investigate future trends based on in lab patient monitoring and coaching technologies that are still under evaluation in feasibility-level studies.
Methods
Literature Search Overview
Within this section, the methodology for conducting the scoping review is detailed. More specifically, starting from the research question “Which is, nowadays, the level of validity and maturity of virtual coaching systems and how close are we to fully automated closed-loop systems?,” the methodology proposed in Arksey and O'Malley (16) was followed. The main concept of the present study refers to virtual coaching systems within the medical arena, which collect in an automated way data from the end user (e.g., motion data, speech, gestures, etc.) and, through an inference mechanism, provide feedback related to the scope of each system. Ideally, the coaching systems should be adjustable to each user (i.e., personalized feedback) and be designed to dynamically adapt to its sensing input data, user feedback or environmental conditions. In addition, this review will showcase the use of real-time evaluations and coaching feedback, as this functionality is necessary for more advanced smart virtual coaching systems. Real-time refers to the system's ability to be constantly aware of both the user's condition and its environment, dynamically evaluate his/her actions, behavior, movement along with different environmental factors (i.e., real-time evaluations), and being able to timely respond to such changes as they are being registered with appropriate interventions (i.e., real-time user feedback), to ensure compliance, correct system use as intended, and provide motivation.
The collection of the relative studies was limited to a time range from January 2010 and until April 2020, utilizing three online databases; PubMed, Scopus, and IEEE Xplore. Within this context, a panel of three experts chose the most relevant keywords, which were used for querying the aforementioned databases. The selected keywords were “virtual coach,” “virtual coaching,” “virtual trainer,” “virtual therapist,” “virtual nurse,” “virtual intervention,” “persuasive system,” “virtual reality coaching,” “augmented reality coaching,” “assistive robotics coaching,” “avatar coaching,” “e coach,” and “robotic coaching,” and were used to produce the search terminology for this review, by considering all possible variations. Using these keywords, appropriate search queries were formulated, according to the specifications of each database. It should be noted that the literature review for virtual coaching systems was focused on the medical and clinical domain. In addition, the research papers had to be written in English in order to be included in this review. The type of publication was not considered as a limitation, and all studies that were either published in international journals or conference proceedings were included. The next steps involved the identification of the relevant studies and screening of the search results using a set of exclusion criteria as described below.
Study Exclusion Criteria
After collecting the literature, three of the authors (i.e., KT, VT and DG) independently screened the titles and the abstracts of all papers, aiming to apply a set of specific exclusion criteria. These criteria involved four main pillars:
• Domain: Only coaching systems relating to clinical and medical topics were considered. Thus, coaching systems in the area of e.g., sports were excluded.
• End users: Systems should target users who face a morbidity. Thus, any other target group (e.g., medical professionals) were excluded from the next phase.
• Scope: The main scope of the presented system should be active virtual coaching. Thus, systems in the domain of telehealth, e-learning, etc. were excluded. Also, systems that did not include any automated inference and feedback mechanism were excluded. The absence of real-time evaluations or real-time coaching feedback was not considered as a factor for excluding a study. Finally, review papers were also excluded.
• Validity: Systems with no evaluation/validation study were excluded. In addition, studies reporting findings of coaching systems that were tested on different subject populations than their intended end users were also excluded (e.g., if a post-stroke rehabilitation system was evaluated with healthy individuals).
The review panel met three times, in order to discuss the exclusion criteria and form a consensus on the next steps. During the last meeting, a test among the authors was conducted by randomly selecting ten research papers, from the dataset created in the previous phase. Each author had to exclude all papers of the sample provided that met one or more of the exclusion criteria. All authors pointed out the exact same studies for exclusion. All studies that passed the exclusion criteria were forwarded to the next phase, where a full-text review was conducted, as discussed in the following section.
Results
Selected Studies Overview
The literature search process from the three databases resulted in a total of 1,266 research papers. From these studies, 71.2% of them were retrieved by the Scopus database, 17.3% from the PubMed database, and 11.5% from the IEEE Xplore database. The steps of the screening methodology are presented in Figure 1, along with the number of studies that were excluded in each step. The first step consisted of removing duplicate records of common studies that were found across the three databases. All unique studies remaining were then considered in the initial title and abstract-based screening following the proposed exclusion criteria (i.e., section Study Exclusion Criteria). At this state, the main exclusion criteria applied involved the domain and the scope criteria. Full text assessment of the remaining papers was then performed. Each one of the three authors involved assessed a total of 50 papers, thus a subset was reviewed more than once to control for inconsistences in the revision process among the panel. At this state, the most relevant exclusion factor was validity, as many studies included only preliminary methods and results, or presented coaching systems without any evaluation results (e.g., studies at pre-pilot state), or systems that were evaluated with subjects different to the intended patient populations.
The final subset of the 41 studies selected was then categorized into three classes depending on the targeted evaluation environment and equipment used: (i) home-based systems (56.1%), (ii) lab/hospital-based systems (36.6%), and (iii) systems including assistive robotics (7.3%). Figures 2, 3 present a quantitative schematic representation of the selected papers. More specifically, Figure 2 presents the distribution of papers over time and also separated per class (i.e., home-based, lab/hospital-based, assistive robotics). An increasing trend in the number of studies per year, and therefore virtual coaching systems developed, can be observed within the last decade, and in fact 75.6% of the 41 studies included were published during the second half of the decade. This is a strong indicator that during the next few years, the number of virtual coaching systems will likely further increase. The number of studies per clinical/medical domain is presented in Figure 3, also separated per class.
In Home Virtual Coaching Systems
Table 1 presents remote virtual coaching systems that were designed and developed to operate at in home environments, as these are prime candidates to showcase the trends and advances in developing state-of-the-art automated models, which are capable of taking advantage of IoT solutions and cloud-edge computing interactions. There are 9 studies presenting coaching systems that focused on promoting and improving the levels of physical activity, with 5 of them focusing on young and middle-aged adults (19, 20, 22, 23, 25), 3 targeting physical activity motivation for the elder population (21, 26, 27), and one study focusing on families with overweight children (24). These applications consisted the typical use case for including continuous active subject monitoring with wearable sensing technology. Systems aiming at reducing the risk of developing type 2 diabetes and weight loss by increasing the level of physical activity in prediabetes subjects and overweight children, respectively, had also implemented continuous monitoring technologies in their intervention programs (24, 33). In most cases, the level of activity was determined by the number of daily steps, while the virtual coaches provided motivational content to help users reach their targeted goals as set during the intervention, in an attempt to keep them engaged in active exercise scheduling, motivate behavioral and lifestyle changes (i.e., especially toward healthier dietary habits) and increase adherence.
Insomnia was found to be another common domain for healthcare coaching systems. All interventions relied on conversational systems, delivering personalized advice either through animated coaching avatars (28, 30), or through automated text dialogs (i.e., chatbots) (29). None of these coaching systems included active sleep monitoring and analysis, but relied on self-reported sleep diaries and user information. Availability of sensor-based sleep tracking technologies however is on the rise as it is currently supported by the majority of commercial smart watch manufacturers (e.g., Garmin, Fitbit, Withings, Samsung, Polar, etc.), and such systems could find their way in similar studies in the future, as sleep tracking algorithms continue to mature. The same means of user-coach interaction was used for the two systems that were developed to assist with smoking cessation in Veterans (32) and young smoking adults (31). A similar system based on interactions with virtual human avatars was also developed to develop a self-management program that could assist older women with overactive bladder symptoms, improving quality of life and perceived symptoms severity (37). The primary focus of these systems was to raise awareness and promote behavioral changes for a more healthy lifestyle overall, a concept that led to holistic approaches with conversational agents that targeted the promotion of physical activity, healthy diet and effective stress coping as in the study of (25).
Real-time feedback on the other hand was included in only a few studies. Baez et al., created a virtual gym environment to address both aspects of physical training and social user interactions between older adults, and used wearable sensors to measure adherence by tracking exercise completion (27). Rehabilitation training consisted another medical domain where real-time feedback was provided to the user, for exercise quality evaluation through tracking posture and body movement during home-based physiotherapy programs for patients after total knee arthroplasty (18). More common was the use of heart rate monitoring and cardiac-control during exercise. Coaching systems that were guiding the level of activity based on heart rate monitoring for improved rehabilitation were developed and tested for patients with myocardial infarction (17), and patients diagnosed with Parkinson's disease (36). Focusing on younger adults, Segerståhl and Kukkonen, developed a system that could be used to guide exercise execution and keep the users within a certain cardiac level (i.e., training intensity level), using a wearable heart rate monitoring device (21). A wearable heart rate sensor was also implemented in another system that targeted virtual interventions for subjects with social anxiety disorders using virtual health agent and characters to simulate stressful immersive environments, where patients could train to improve their social behavior skills (38). Finally, in a more specialized medical application, Wu et al. developed a prototype coaching system that could assist powered wheelchair users to take full advantage of the provided powered seating functions (e.g., tilt in space, backrest recline, leg rest elevation), following clinically recommended adjusting protocols to maintain seating stability and prevent prolonged-seating complications (39).
Coaching Systems for Hospital/Lab Environment
This section includes 15 studies showcasing virtual coaching systems that were designed and evaluated in controlled hospital or lab environments, or virtual coaching systems that were designed for in home environment, but were evaluated in hospital/lab settings. As it shown in Table 2, 11 of these studies consisted of preliminary system evaluations focusing on feasibility and technology acceptance, performing single supervised experimental sessions in small patient populations. The primary focus of the virtual coaching systems that were tested was physical rehabilitation and psychological training for subjects with cognitive impairment, phobias, or mental disorders. Since the experiments were conducted under medical supervision, the clinical use cases extended to patients with more severe physical, mental or cognitive deficits, who were commonly excluded from studies and systems targeting in home evaluations, as the intervention could lead to uncontrolled and hard to predict adverse events. As expected, technologies for continuous subject monitoring could not be properly evaluated under these experimental conditions and, thus, none of these studies reported such measurements. On the other hand, real-time evaluations were extensively integrated in the proposed systems and tested in these studies, with “live” user feedback functionality being the key differentiating factor compared to the in home systems presented in the previous section.
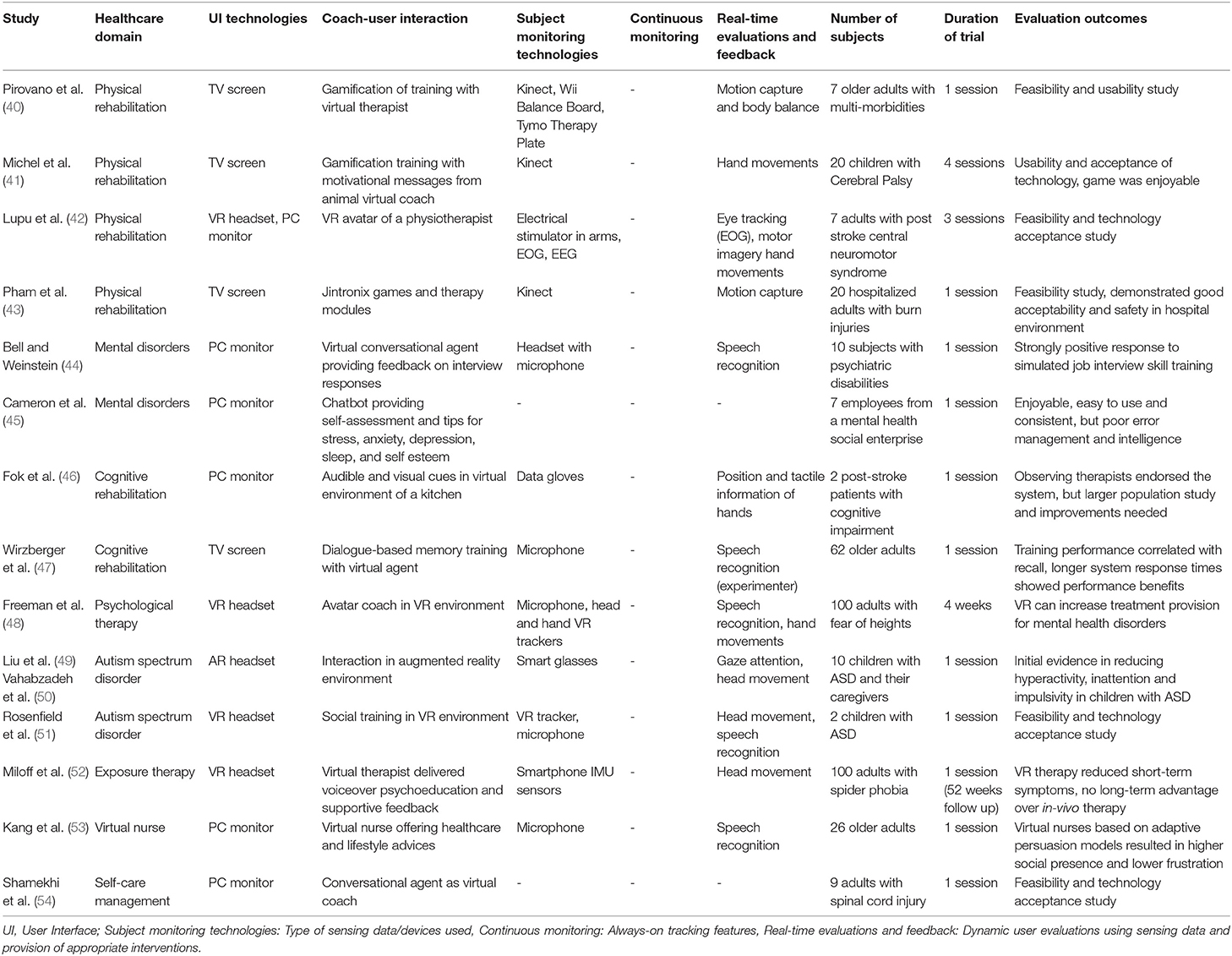
Table 2. Coaching systems that were evaluated in controlled laboratory or clinical environment under direct supervision.
Virtual reality (VR) wearable headsets were introduced into different medical domains, along with more advanced movement tracking and speech recognition systems. Freeman et al. used immersive VR environments to enhance psychological therapy interventions for adults with height phobias by simulating different scenarios of high altitude (48), while subjects with spider phobia were also introduced to a VR system that provided exposure therapy and educational material to help them cope with their fear (52). Systems offering social training for children with Autism Spectrum Disorders (ASD) (51), and physical rehabilitation for post-stroke patients (42) have also been evaluated, showing positive outcomes of the feasibility and acceptability of the immersive VR technology in medical coaching applications. Augmented reality (AR) devices in the form of smart glasses were also tested with children suffering from ASD, as a means to assist them in directing their attention toward a human companion during conversation scenarios, and promote social activities (49, 50).
As it is shown in Table 2, the controlled environment and the supervised experiments allowed researchers to evaluate more complex sensing and monitoring technologies. Virtual coaching and gamification of physical rehabilitation training with advanced movement tracking and real-time head and body movement evaluations were implemented using commercially available devices, including Microsoft's Kinect, Nintendo's Wii platform and its peripherals (40, 41, 43). In addition, in the cases where VR was also part of the intervention, head, and hand movement tracking was also performed using the included proprietary trackers of the commercial VR systems, or the integrated IMU sensor if a smartphone attached to a head-mounted device was used instead. Eye tracking through commercial smart glasses was also implemented in AR systems targeting children with ASD, in order to train them to direct their attention toward a fellow conversational companion and engage more in social activities (49, 50).
Despite being in a more laboratory state of technology readiness, custom tracking solutions were less common and only a couple of studies invested in alternative solutions. In a proof of concept study targeting cognitive rehabilitation, a sensing glove was utilized to test feasibility of a more realistic hand and finger movement tracking system with tactile feedback, while performing an intervention focusing on virtually simulated everyday tasks (e.g., meal preparation), showing mixed results in post-stroke patients (46). In another system for post-stroke rehabilitation developed by Lupu et al., biosignal analysis was used for eye movement tracking and motor imagery training, using electrophysiological data captured while recording patient's electrooculogram (EOG) and electroencephalogram (EEG) signals (42). As it shown by their inclusion in a single study, the use of such technologies for medical applications is currently in its infancy state, as the acquisition of biosignals can only be implemented under direct medical supervision and assistance.
Systems supporting natural speech recognition and text semantics analysis in the form of automated conversational agents either through virtual avatars of chatbots, respectively, were also found to gain research attention for coaching purposes. They were primarily used in systems targeting patients with mental and cognitive disorders, offering healthcare and motivational coaching, in order to help them overcome phobias, mental health care, and train in social activities (44, 45, 47, 53). Speech recognition was commonly used to complement systems with immersive VR, in order to provide an enhanced user-system interaction experience (48, 51).
Assistive Robotics in Coaching
This section describes the use of external assistive technologies and robotic-based interventions as part of the coaching process. As expected, these systems were evaluated under supervised conditions in lab environment, but are distinguished by the studies reported in section Coaching Systems for Hospital/Lab Environment above, since the added robotic devices enhance the realism of the interventions, especially when it is complemented with automated audio feedback and speech recognition capabilities. Robotic rehabilitation systems could enhance the sensing input for the patients, providing force and haptic feedback, while interactions with actual robotic coaches and older adults that were used in socially assistive robotic systems, were found to be useful in improving social, physical, and cognitive training programs. The later was shown to be very effective in a recent study, where a commercial robot (i.e., NAO robot) was used as a coach to assist and provoke social interactions in older adults with and without cognitive impairment (55). The robotic coach was designed to provide one-on-one coach-user interaction, as well as multi-user and coach interactions, with individualized activity management and dynamically adaptive behavior for long-term engagement and was very received during its initial testing.
Then, there were also two feasibility evaluation studies that focused on robotic physical rehabilitation. Despite targeting different age population groups and medical conditions, both studies resulted in similar findings; showcasing the proposed coaching systems as useful training tools to complement the existing approaches in rehabilitation therapy. Chiang et al., used force feedback from two haptic devices to enhance real-time user-system interactions in non-immersive VR environments, training children and adolescents with upper limb disabilities to improve performance in activities of daily living, showing high level of satisfaction when using virtual rehabilitation systems (56). An arm exoskeleton that provided gravity-compensation in arm movements and dynamically adapted feedback in non-immersive VR environment was also tested for personalized rehabilitation in post-stroke patients with severely affected motor functionality (57). A closed-loop system that facilitated unsupervised motor learning providing adjustable level of supported depending on each patient's motor potential, and continuous visual feedback of a virtual arm was proposed to complement the perception of natural movement and improve the quality and range of the functional restoration.
Discussion
As Figure 3 suggests, 17 studies of the total of 41 included in this review of virtual coaching systems dealt with either physical rehabilitation or physical activity and motivation of healthier lifestyle choices. This was also found to be the most prominent field for using sensing devices as 15 out of the 17 systems included patient monitoring, especially with the capacity to capture level of exercise with activity trackers, accelerometers, and pedometers, and relevant body movement using depth cameras for precise posture and skeleton tracking, or even haptic and exoskeleton devices. Systems for motivation of physical activity could also be more easily implemented in larger patient populations as they did not engage specific and detailed real-time evaluations and relied mostly on user's self-reported input. Coaching systems for mental disorders and cognitive rehabilitation were also found to gain research attention in the past few years, but, as shown in Table 2, their applications were limited only within supervised testing conditions and have not yet been transported to independent, in home use scenarios. In addition, another notable remark refers to the under-representation of clinical conditions, like Parkinson's disease, cardiovascular diseases, ASD, diabetes, and orthopedics for which technology-aided virtual coaching systems should have a substantial impact to quality of life and everyday activities.
Integration of new technologies for in home coaching systems was also found to be rather limited, which is an alarming outcome for the field, overall. For example, immersive VR and AR interaction solutions were not implemented in systems that targeted in home use cases, and were rather only found in controlled lab environment. This is an indicator, however, that these technologies are currently being meticulously tested, and mass adoption in the future is to be expected. Especially as they continue to improve in terms of supported functionality, tracking accuracy and lower costs. The same could be applied to assistive robotics, as positive results from the studies reported in Table 3 suggest that the robotic-based training has great potential to be used as a way to enhance existing interventions in occupational therapy. Although wide spread adoption is more challenging than VR and AR technologies, there is already evidence that the novelty factor that accompanies assistive robotic interventions, can be very beneficial in specific medical conditions such as stroke rehabilitation (58).
Subject monitoring and sensing technologies being used in coaching systems seem to be highly dependent on technology readiness levels of the various IoT devices. The level of physical activity as estimated through step tracking is found to be the most common means, which can be explained considering that it is nowadays a mature technology (i.e., in terms of both hardware and software), with increased availability and reduced associated costs, as competing commercial wearable devices pushed advancements in this field. Microsoft's Kinect was another landmark device that enabled advanced functionality in coaching systems, as it provided unobtrusive monitoring of whole body movement and more precise user-system interaction with upper extremities. Despite being discontinued at consumer level, its practicality allowed even non-highly tech educated people to familiarize with this technology and has inspired researchers to develop medical devices and systems that integrate similar functionality, especially in rehabilitation interventions (18, 59). Heart rate monitoring is another field where IoT devices have reached a level of functional robustness and monitoring accuracy that justified their widespread use for clinical use cases in coaching systems (Table 1). A similar transition in monitoring technology is currently being materialized following the advancements in smartwatches, which should be shown in more precise physical activity and sleep monitoring and enhanced evaluation feedback. This is the first link that will enable the development of personalized and precise monitoring systems that can seamlessly provide continuous patient tracking in their everyday life environment and living conditions.
The second important link in the chain of more advanced coaching systems is the feedback that they can provide to the user. As the distinction of in home and in lab systems in the results section revealed, the transition from offline and semi-real evaluation of user behavior to real-time feedback for in home environment is currently in its early stages (Table 1), despite the widespread use cases of real-time evaluation functionality under controlled, supervised conditions (Table 2). The findings in Table 2 however, are indicative of the future trends in the evaluation feedback of coaching systems, and the necessity to close the loop with automated and more precise real-time evaluations. This would ultimately allow the available clinical personnel to effectively supervise larger patient populations in their medical domain of expertise, and increase access to better healthcare services in rural areas.
The increased practical functionality that the improved monitoring technologies offer (section Coaching Systems for Hospital/Lab Environment), could define a new generation of coaching systems that are able to revolutionize patient perspective, as “smart” systems become progressively aware of user's environment, activities and behavior, improving the quality of user-system interactions and eventually adherence to the intervention protocols. A closed-loop coaching system that can dynamical adjust to each patient's needs, evaluate their behavior and actions in real-time, and intervene accordingly offers a holistic approach in motor and cognitive training and rehabilitation address. This will set a crucial milestone in coaching systems enabling the continuity of healthcare between subsequent visits with medical professionals.
The lack of holistic coaching systems was also acknowledged by the European Commission, which led to several recent projects responding to the H2020 call SC1-PM-15-2017—Personalized coaching for well-being and care of people as they age. The call tried to address the deployment of radically new solutions for personalized virtual coaching systems, building upon intelligent ICT environments, access to relevant physiological, and behavioral data and new forms of accessible interaction based on tangible user interaction concepts. Currently, these projects are evaluating the different approaches with the targeted aging population:
HOLOBALANCE1 introduces a new personalized platform for virtual coaching, motivation, and empowerment of older citizens with balance disorders. The coaching part is realized with a holographic surrogate physiotherapist and augmented reality games, along with easy to use wearable sensors in an interoperable platform design (60).
Council of Coaches2 introduces a radically new virtual coaching concept based on multiple autonomous, embodied virtual coaches, which form together a personal council addressing the needs of older adults in an integrated manner (61).
WellCo3 provides an affective-aware coach that interacts through speech with the user in order to act as a virtually interface among the user and the platform managing the flow of all interactions and empower users in their behavior change process through simulation activities tailored to their current mood (62).
CAPTAIN4 proposes a transparent technology designed to turn the home of the older adult into a ubiquitous assistant, based on a projected augmented reality through use of micro-projectors, contextualized information and instructions on top of the real environment (63).
vCare4 embeds clinical profiles and the pathways to drive the behavior of the virtual coach at home and provide well-elaborated services for tele-rehabilitation in neurology and cardiology (64).
SAAM5 supports the aging population living at home, with a novel and practical emphasis on ambient sensing and learning of user needs and preferences, and effective coaching by leveraging the user's social support networks (65).
EMPATHIC6 proposes multimodal face analytics, adaptive spoken dialogue systems and natural language interfaces along with non-intrusive technologies to extract physiological markers of emotional states in real-time, in order to support dependent aging persons and their caregivers (66).
NESTORE7 leverages on novel ICT technologies for unobtrusive monitoring system, including wearable and environmental sensors, intelligent Decision Support System to provide personalized goals toward wellbeing, and active coaching as conversational agents, embodied in a physical companion to establish affective communication and engage user in personalized coaching activities (67).
Finally, it should be noted that besides the large amount of studies that has been considered during the literature search, it is still very likely that some relevant research papers have not been discovered or missed. From the studies that have been included however, it is clear that the current and near future research trends in virtual coaching systems are pushing to close the loop with advanced patient monitoring, improved virtual user-system interactions and most critically, support for automated feedback and dynamically adjusted precise interventions, based on personalized evaluations from the sensing data.
Conclusion
The systematic evaluation of the literature within this review has shown that virtual coaching systems are gaining momentum, as new monitoring and system-user interaction technologies are being evaluated and their medical applications are expanding in more complex diseases. Technology integration is highly dependent on the current status and supported functionality of IoT sensing devices, with novel patient monitoring and intriguing virtual interfaces becoming increasingly popular in coaching systems, especially in controlled and supervised environments. Transition to fully automated in home coaching systems, however, is proving to be in its early stages and many obstacles still remain before holistic coaching solutions with real-time evaluations and personalized feedback can become widely available for unsupervised in home use. The added value of such systems in terms of effectiveness, technology acceptance, and reduced healthcare costs has been well documented though and proven in proof of concept studies over the past few years. The current research projects that were funded to address these issues are on track to set the foundations for a new generation of coaching systems.
Author Contributions
KT, VT, and DG performed the screening process of the research papers. DF performed a final revision of the final manuscript. All authors contributed equally in the preparation of the manuscript, the formulation of the literature search methodology, the exclusion criteria used for this review, revision of the article, and approved the submitted version.
Funding
This study was supported by the Holobalance project (https://holobalance.eu/) which is funded within the European Union's Horizon 2020 research and innovation programme under grant agreement no. 769574.
Conflict of Interest
The authors declare that the research was conducted in the absence of any commercial or financial relationships that could be construed as a potential conflict of interest.
Footnotes
2. ^https://council-of-coaches.eu/
4. ^https://www.captain-eu.org/
References
1. Farahani B, Firouzi F, and Chakrabarty K. Healthcare IoT. Intelligent Internet of Things: From Device to Fog and Cloud. Firouzi Springer International Publishing. (2020).p. 515–45. doi: 10.1007/978-3-030-30367-9_11
2. Woo MW, Lee J, and Park K. A reliable IoT system for personal healthcare devices. Future Gen Comp Syst. (2018) 78:626–40. doi: 10.1016/j.future.2017.04.004
3. Dimitrov DV. Medical internet of things and big data in healthcare. Healthc Inform Res. (2016) 22:156–63. doi: 10.4258/hir.2016.22.3.156
4. Ojo MO, Giordano S, Procissi G, and Seitanidis IN. A review of low-end, middle-end, and high-end Iot devices. IEEE Access. (2018) 6:70528–54. doi: 10.1109/ACCESS.2018.2879615
5. Knickerbocker JU, Budd R, Dang B, Chen Q, Colgan E, Hung LW, et al. Heterogeneous integration technology demonstrations for future healthcare, IoT, and AI computing solutions. In: 2018 IEEE 68th Electronic Components and Technology Conference (ECTC). (2018) doi: 10.1109/ECTC.2018.00231
6. Kulyk O, op den Akker R, Klaassen R, and van Gemert-Pijnen LJ. Personalized virtual coaching for lifestyle support: principles for design and evaluation. Int J Adv Life Sci. (2014) 6:300.
7. van Wissen A, Vinkers C, and van Halteren A. Developing a Virtual Coach for Chronic Patients: A User Study on the Impact of Similarity, Familiarity and Realism. Cham: Springer International Publishing (2016). doi: 10.1007/978-3-319-31510-2_23
8. Klimova B, Valis M, and Kuca K. Cognitive decline in normal aging and its prevention: a review on non-pharmacological lifestyle strategies. Clin Intervent Aging. (2017) 12:903–10. doi: 10.2147/CIA.S132963
9. Liu X, and Zhao W. Buddy: a virtual life coaching system for children and adolescents with high functioning autism. In: 2017 IEEE 15th Intl Conf on Dependable, Autonomic and Secure Computing, 15th Intl Conf on Pervasive Intelligence and Computing, 3rd Intl Conf on Big Data Intelligence and Computing and Cyber Science and Technology Congress. (2017). doi: 10.1109/DASC-PICom-DataCom-CyberSciTec.2017.62
10. Zhao W, Liu X, Qiu T, and Luo X. Virtual avatar-based life coaching for children with autism spectrum disorder. Computer. (2020) 53:26–34. doi: 10.1109/MC.2019.2915979
11. Ding D, Liu HY, Cooper R, Cooper RA, Smailagic A, and Siewiorek D. Virtual coach technology for supporting self-care. Phys Med Rehabil Clin N Am. (2010) 21:179–94. doi: 10.1016/j.pmr.2009.07.012
12. Tropea P, Schlieter H, Sterpi I, Judica E, Gand K, Caprino M, et al. Rehabilitation, the great absentee of virtual coaching in medical care: scoping review. J Med Internet Res. (2019) 21:e12805. doi: 10.2196/12805
13. Salvemini AG, D'Onofrio, Ciccone F, Greco A, Tullio A, et al. Insomnia and information and communication technologies (ICT) in elderly people: a systematic review. Med Sci. (2019) 7:70. doi: 10.3390/medsci7060070
14. Lentferink AJ, Oldenhuis H, de Groot KEM, Polstra L, Velthuijsen H, and van Gemert-Pijnen JEWC. Key components in ehealth interventions combining self-tracking and persuasive ecoaching to promote a healthier lifestyle: a scoping review. J Med Internet Res. (2017) 19:e277. doi: 10.2196/jmir.7288
15. Provoost S, Lau HM, Ruwaard J, and Riper H. Embodied conversational agents in clinical psychology: a scoping review. J Med Internet Res. (2017) 19:e151. doi: 10.2196/jmir.6553
16. Arksey H, and O'Malley L. Scoping studies: towards a methodological framework. Int J Soci Res Methodol. (2005) 8:19–32. doi: 10.1080/1364557032000119616
17. Salvi D, Ottaviano M, Muuraiskangas SA, Martínez-Romero C, Vera-Muñoz MF, Cabrera Umpiérrez MT, et al. An m-Health system for education and motivation in cardiac rehabilitation: the experience of HeartCycle guided exercise. J Telemed Telecare. (2018) 24:303–16. doi: 10.1177/1357633X17697501
18. Prvu Bettger J, Green CL, Holmes DN, Chokshi A, Mather RC 3rd, Hoch BT, et al. Effects of virtual exercise rehabilitation in-home therapy compared with traditional care after total knee arthroplasty: VERITAS. a randomized controlled. Trial J Bone Joint Surg Am. (2020) 102:101–9. doi: 10.2106/JBJS.19.00695
19. Segerståhl K, and Oinas-Kukkonen H. Designing personal exercise monitoring employing multiple modes of delivery: implications from a qualitative study on heart rate monitoring. Int J Med Inform. (2011) 80:e203–13. doi: 10.1016/j.ijmedinf.2011.08.011
20. Watson A, Bickmore T, Cange A, Kulshreshtha A, and Kvedar J. An internet-based virtual coach to promote physical activity adherence in overweight adults: randomized controlled trial. J Med Internet Res. (2012) 14:e1. doi: 10.2196/jmir.1629
21. Bickmore TW, Silliman RA, Nelson K, Cheng DM, Winter M, Henault L, et al. A randomized controlled trial of an automated exercise coach for older adults. J Am Geriatr Soc. (2013) 61:1676–83. doi: 10.1111/jgs.12449
22. Friederichs S, Bolman C, Oenema A, Guyaux J, and Lechner L. Motivational interviewing in a Web-based physical activity intervention with an avatar: randomized controlled trial. J Med Internet Res. (2014) 16:e48. doi: 10.2196/jmir.2974
23. Akker HJA, Klaassen R, and Nijholt A. Virtual Coaches for Healthy Lifestyle. Toward Robotic Socially Believable Behaving Systems - Volume II: Modeling Social Signals. Cham: Springer International Publishing (2016). p. 121–49. doi: 10.1007/978-3-319-31053-4_8
24. Gabrielli S, Dianti M, Maimone R, Betta M, Filippi L, Ghezzi M, et al. Design of a mobile app for nutrition education (trec-lifestyle) and formative evaluation with families of overweight children. JMIR Mhealth Uhealth. (2017) 5:e48. doi: 10.2196/mhealth.7080
25. Fadhil A, Wang Y, and Reiterer H. Assistive conversational agent for health coaching: a validation study. Methods Inf Med. (2019) 58:9–23. doi: 10.1055/s-0039-1688757
26. Brandenburgh AW, van Breda W, van der Ham, Klein M, and Moeskops L. Increasing Physical and Social Activity through Virtual Coaching in an Ambient Environment. Cham: Springer International Publishing (2014). doi: 10.1007/978-3-319-09912-5_41
27. Báez M, Ibarra F, Far IK, Ferron M, and Casati F. Online group-exercises for older adults of different physical abilities. In: 2016 International Conference on Collaboration Technologies and Systems (CTS), Orlando, FL (2016). doi: 10.1109/CTS.2016.0098
28. Espie CA, Kyle SD, Williams C, Ong JC, Douglas NJ, Hames P, et al. A randomized, placebo-controlled trial of online cognitive behavioral therapy for chronic insomnia disorder delivered via an automated media-rich web application. Sleep. (2012) 35:769–81. doi: 10.5665/sleep.1872
29. Beun RJ, Fitrianie S, Griffioen-Both F, Spruit S, Horsch C, Lancee J, Brinkman W, et al. Tools: the best of both worlds in mobile user interfaces for E-coaching. Personal Ubiquitous Comp. (2017) 21:661–74. doi: 10.1007/s00779-017-1021-5
30. Lorenz N, Heim E, Roetger A, Birrer E, and Maercker A. Randomized controlled trial to test the efficacy of an unguided online intervention with automated feedback for the treatment of insomnia. Behav Cogn Psychother. (2019) 47:287–302. doi: 10.1017/S1352465818000486
31. An LC, Demers MR, Kirch MA, Considine-Dunn S, Nair V, Dasgupta K, et al. A randomized trial of an avatar-hosted multiple behavior change intervention for young adult smokers. J Natl Cancer Inst Monogr. (2013) 2013:209–15. doi: 10.1093/jncimonographs/lgt021
32. Abdullah AS, Gaehde S, and Bickmore T. A tablet based embodied conversational agent to promote smoking cessation among veterans: a feasibility study. J Epidemiol Glob Health. (2018) 8:225–30. doi: 10.2991/j.jegh.2018.08.104
33. Connelly J, Kirk A, Masthoff J, and MacRury S. A website to promote physical activity in people with type 2 diabetes living in remote or rural locations: feasibility pilot randomized controlled trial. JMIR Diabetes. (2017) 2:e26. doi: 10.2196/diabetes.6669
34. Block G, Azar KM, Romanelli RJ, Block TJ, Hopkins D, Carpenter HA, et al. Diabetes prevention and weight loss with a fully automated behavioral intervention by email, web, and mobile phone: a randomized controlled trial among persons with prediabetes. J Med Internet Res. (2015) 17:e240. doi: 10.2196/jmir.4897
35. Ellis T, Latham NK, DeAngelis TR, Thomas CA, Saint-Hilaire M, and Bickmore TW. Feasibility of a virtual exercise coach to promote walking in community-dwelling persons with Parkinson disease. Am J Phys Med Rehabil. (2013) 92:472–481; quiz 482–475. doi: 10.1097/PHM.0b013e31828cd466
36. van der Kolk N, de Vries MNM, Kessels R, Joosten PCH, Zwinderman AH, Post B, and Bloem BR. Effectiveness of home-based and remotely supervised aerobic exercise in Parkinson's disease: a double-blind, randomised controlled trial. Lancet Neurol. (2019) 18:998–1008. doi: 10.1016/S1474-4422(19)30285-6
37. Andrade AD, Anam R, Karanam C, Downey P, and Ruiz JG. An overactive bladder online self-management program with embedded avatars: a randomized controlled trial of efficacy. Urology. (2015) 85:561–7. doi: 10.1016/j.urology.2014.11.017
38. Hartanto D, Brinkman WP, Kampmann IL, Morina N, and Emmelkamp P. Home-Based Virtual Reality Exposure Therapy with Virtual Health Agent Support. Cham: Springer International Publishing. (2016). doi: 10.1007/978-3-319-32270-4_9
39. Wu YK, Liu Y, Kelleher A, Pearlman J, and Cooper RA. Evaluating the usability of a smartphone virtual seating coach application for powered wheelchair users. Med Engin Phys. (2016) 38:569–75. doi: 10.1016/j.medengphy.2016.03.001
40. Pirovano M, Mainetti R G, Baud-Bovy, Lanzi PL, and Borghese NA. Intelligent Game Engine for Rehabilitation (IGER). IEEE Trans Comp Intel Games. (2016) 8:43–55. doi: 10.1109/TCIAIG.2014.2368392
41. Michel P, Richard P, Yamaguchi T, Verhulst A, Verhulst E, and Dinomais M. Virtual reality system for rehabilitation of children with cerebral palsy: a preliminary study. In: Proceedings of the 11th Joint Conference on Computer Vision, Imaging and Computer Graphics Theory and Applications, Vol. 1. Rome: SciTePress (2016), 306–313. doi: 10.5220/0005720503040311
42. Lupu RG, Irimia DC, Ungureanu F, Poboroniuc MS, and Moldoveanu BC. FES based therapy for stroke rehabilitation using VR facilities. Wireless Commun Mobile Comput. (2018) 2018:4798359. doi: 10.1155/2018/4798359
43. Pham TN, Wong JN, Terken T, Gibran NS, Carrougher GJ, and Bunnell A. Feasibility of a Kinect(®)-based rehabilitation strategy after burn injury. Burns. (2018) 44:2080–6. doi: 10.1016/j.burns.2018.08.032
44. Bell MD, and Weinstein A. Simulated job interview skill training for people with psychiatric disability: feasibility and tolerability of virtual reality training. Schizophr Bull. (2011) 37 Suppl 2(Suppl 2):S91–97. doi: 10.1093/schbul/sbr061
45. Cameron G, Cameron D, Megaw G, Bond R, and Mulvenna MS. Assessing the Usability of a Chatbot for Mental Health Care. Cham: Springer International Publishing. (2019). doi: 10.1007/978-3-030-17705-8_11
46. Fok SC, Tan F, and Lau M. Supervising Cognitive Tele-Rehabilitation with a Virtual Therapist. Appl Mech Mater. (2013) 344:213–8. doi: 10.4028/www.scientific.net/AMM.344.213
47. Wirzberger M, Schmidt R, Georgi M, Hardt W, Brunnett G, and Rey GD. Effects of system response delays on elderly humans' cognitive performance in a virtual training scenario. Sci Rep. (2019) 9:8291. doi: 10.1038/s41598-019-44718-x
48. Freeman D, Lister R, Waite F, Yu LM, Slater M, Dunn G, et al. Automated psychological therapy using virtual reality (VR) for patients with persecutory delusions: study protocol for a single-blind parallel-group randomised controlled trial (THRIVE). Trials. (2019) 20:87. doi: 10.1186/s13063-019-3198-6
49. Liu R, Salisbury JP, Vahabzadeh A, and Sahin NT. Feasibility of an autism-focused augmented reality smartglasses system for social communication and behavioral coaching. Front Pediatr. (2017) 5:145. doi: 10.3389/fped.2017.00145
50. Vahabzadeh A, Keshav NU, Salisbury JP, and Sahin NT. Improvement of attention-deficit/hyperactivity disorder symptoms in school-aged children, adolescents, and young adults with autism via a digital smartglasses-based socioemotional coaching aid: short-term, uncontrolled pilot study. JMIR Ment Health. (2018) 5:e25. doi: 10.2196/mental.9631
51. Rosenfield N, Lamkin K, Re J, Day K, Boyd L, and Linstead E. A virtual reality system for practicing conversation skills for children with autism. Multimod Technol Interact. (2019) 3:28. doi: 10.3390/mti3020028
52. Miloff A, Lindner P P, Dafgård, Deak S, Garke M, et al. Automated virtual reality exposure therapy for spider phobia vs. in-vivo one-session treatment: A randomized non-inferiority trial. Behav Res Ther. (2019) 118:130–40. doi: 10.1016/j.brat.2019.04.004
53. Kang Y, Tan AH, and Miao C. An adaptive computational model for personalized persuasion. In: Proceedings of the 24th International Conference on Artificial Intelligence. Buenos Aires: AAAI Press (2015). p. 61-67.
54. Shamekhi A, Trinh H, Bickmore TW, DeAngelis TR, Ellis T, Houlihan BV, et al. A virtual self-care coach for individuals with spinal cord injury. In: Proceedings of the 18th International ACM SIGACCESS Conference on Computers Accessibility. Reno, NV: Association for Computing Machinery (2016). p. 327-328. doi: 10.1145/2982142.2982199
55. Fan J, Bian D, Zheng Z, Beuscher L, Newhouse PA, Mion LC, et al. A Robotic Coach Architecture for Elder Care (ROCARE) Based on Multi-User Engagement Models. IEEE Tran. (2017) 25:1153–63. doi: 10.1109/TNSRE.2016.2608791
56. Chiang VC, Lo KH, and Choi KS. Rehabilitation of activities of daily living in virtual environments with intuitive user interface and force feedback. Disabil Rehabil Assist Technol. (2017) 12:672–80. doi: 10.1080/17483107.2016.1218554
57. Grimm F, Naros G, and Gharabaghi A. Closed-loop task difficulty adaptation during virtual reality reach-to-grasp training assisted with an exoskeleton for stroke rehabilitation. Front Neurosci. (2016) 10:518. doi: 10.3389/fnins.2016.00518
58. Klamroth-Marganska V, Blanco J, Campen K, Curt A, Dietz V, Ettlin T, et al. Three-dimensional, task-specific robot therapy of the arm after stroke: a multicentre, parallel-group randomised trial. Lancet Neurol. (2014) 13:159–66. doi: 10.1016/S1474-4422(13)70305-3
59. De Luca R, Lo Buono V, Leo A, Russo M, Aragona B, Leonardi S, et al. Use of virtual reality in improving poststroke neglect: Promising neuropsychological and neurophysiological findings from a case study. Appl Neuropsychol. (2019) 26:96–100. doi: 10.1080/23279095.2017.1363040
60. Tsiouris KM, Gatsios D, Tsakanikas V, Pardalis AA, Kouris I, Androutsou T, et al. Designing interoperable telehealth platforms: bridging IoT devices with cloud infrastructures. Enter Inform Syst. (2020) 2020:1-25. doi: 10.1080/17517575.2020.1759146
61. Hurmuz MZM, Jansen-Kosterink SM, den Akker HO, and Hermens HJ. User experience and potential health effects of a conversational agent-based electronic health intervention: Protocol for an observational cohort study. JMIR Res Prot. (2020) 9:4. doi: 10.2196/16641
62. Manea V, and Wac K. mQoL: mobile quality of life lab: from behavior change to QoL. In: Proceedings of the 2018 ACM International Joint Conference and 2018 International Symposium on Pervasive and Ubiquitous Computing and Wearable Computers. Singapore: Association for Computing Machinery (2018).p. 642–7. doi: 10.1145/3267305.3267549
63. Petsani D, Mantziari D, Zilidou V, Konstantinidis EI, Billis A, Timoleon M, et al. Co-design the future CAPTAIN system with older adults: focusing on the e-coaching dimensions. In: Proceedings of the 12th ACM International Conference on PErvasive Technologies Related to Assistive Environments. Rhodes: Association for Computing Machinery (2019). p. 639-644. doi: 10.1145/3316782.3322765
64. Philipp P, Merkle N, Gand K, and Gißke C. Continuous support for rehabilitation using machine learning. Inform Tech. (2019) 61:273. doi: 10.1515/itit-2019-0022
65. Dimitrov Y, Gospodinova Z, Wheeler R, Žnidaršič M, Ženko B, Veleva V, and Miteva N. Social activity modelling and multimodal coaching for active aging. In: Proceedings of the 12th ACM International Conference on PErvasive Technologies Related to Assistive Environments. Rhodes: Association for Computing Machinery (2019).p. 608–15. doi: 10.1145/3316782.3322761
66. Justo R, Ben Letaifa L, Palmero C, Gonzalez-Fraile E, Torp Johansen A, Vázquez A, et al. Analysis of the interaction between elderly people and a simulated virtual coach. J Ambient Intell Human Comput. (2020) doi: 10.1007/s12652-020-01983-3
Keywords: virtual coaching, review, coaching systems, automated feedback, closed-loop systems, physical activity monitoring, rehabilitation coaching, behavioral change
Citation: Tsiouris KM, Tsakanikas VD, Gatsios D and Fotiadis DI (2020) A Review of Virtual Coaching Systems in Healthcare: Closing the Loop With Real-Time Feedback. Front. Digit. Health 2:567502. doi: 10.3389/fdgth.2020.567502
Received: 29 May 2020; Accepted: 28 August 2020;
Published: 30 September 2020.
Edited by:
Constantinos S. Pattichis, University of Cyprus, CyprusReviewed by:
Alexander James Casson, The University of Manchester, United KingdomMaurice Mars, University of KwaZulu-Natal, South Africa
Copyright © 2020 Tsiouris, Tsakanikas, Gatsios and Fotiadis. This is an open-access article distributed under the terms of the Creative Commons Attribution License (CC BY). The use, distribution or reproduction in other forums is permitted, provided the original author(s) and the copyright owner(s) are credited and that the original publication in this journal is cited, in accordance with accepted academic practice. No use, distribution or reproduction is permitted which does not comply with these terms.
*Correspondence: Dimitrios I. Fotiadis, Zm90aWFkaXNAY2MudW9pLmdy