- 1Faculty of Health Sciences, Simon Fraser University, Burnaby, BC, Canada
- 2British Columbia Centre for Disease Control, Vancouver, BC, Canada
- 3Centre for Gender and Sexual Health Equity, Vancouver, BC, Canada
- 4School of Public Health and Health Systems, University of Waterloo, Waterloo, ON, Canada
- 5George and Fay Yee Centre for Healthcare Innovation, University of Manitoba, Winnipeg, MB, Canada
- 6School of Population and Public Health, University of British Columbia, Vancouver, BC, Canada
- 7Department of Medicine, University of British Columbia, Vancouver, BC, Canada
- 8British Columbia Centre for Disease Control (BCCDC) Public Health Laboratory, Vancouver, BC, Canada
- 9Department of Pathology and Laboratory Medicine, University of British Columbia, Vancouver, BC, Canada
Background: Most public health datasets do not include sexual orientation measures, thereby limiting the availability of data to monitor health disparities, and evaluate tailored interventions. We therefore developed, validated, and applied a novel computable phenotype model to classify men who have sex with men (MSM) using multiple health datasets from British Columbia, Canada, 1990–2015.
Methods: Three case surveillance databases, a public health laboratory database, and five administrative health databases were linked and deidentified (BC Hepatitis Testers Cohort), resulting in a retrospective cohort of 727,091 adult men. Known MSM status from the three disease case surveillance databases was used to develop a multivariable model for classifying MSM in the full cohort. Models were selected using “elastic-net” (GLMNet package) in R, and a final model optimized area under the receiver operating characteristics curve. We compared characteristics of known MSM, classified MSM, and classified heterosexual men.
Findings: History of gonorrhea and syphilis diagnoses, HIV tests in the past year, history of visit to an identified gay and bisexual men's clinic, and residence in MSM-dense neighborhoods were all positively associated with being MSM. The selected model had sensitivity of 72%, specificity of 94%. Excluding those with known MSM status, a total of 85,521 men (12% of cohort) were classified as MSM.
Interpretation: Computable phenotyping is a promising approach for classification of sexual minorities and investigation of health outcomes in the absence of routinely available self-report data.
Introduction
Men who have sex with men (MSM) are disproportionately represented in multiple epidemics of public health interest, including HIV, hepatitis C (HCV), hepatitis B (HBV), syphilis, and gonorrhea (1–4). MSM additionally experience numerous mental health and substance use-related inequities, including fourfold greater rates of suicide attempts and twofold greater rates of depression, anxiety, and substance use disorders, as compared with heterosexual men (5–7). These inequities are at least partially attributable to a social stigma attached to minority sexualities, which induces a minority stress response and adaptive behaviors including substance use and sexual risk-taking, in some MSM (8).
In this context, population health databases could be powerful tools for producing new insights into health status and effective disease prevention opportunities for MSM and other sexual and gender minority populations (e.g., sexual minority women, transgender people); however, measurement of sexual and gender minority status is limited in these databases for several reasons. First, sexual orientation and transgender-inclusive measures are not typically recorded in most administrative or laboratory records (3) Second, where collected, MSM status (and analogous minority sexual identities, i.e., gay/lesbian/bisexual) is known to be underreported due to social stigma and related reporting desirability biases. In a recent review, the sensitivity of self-report measures of sexual minority orientation was estimated to be 0.70 (95% credible interval 0.69, 0.71) (specificity: 0.99, 95% credible interval 0.97, 0.99) (6). Third, databases that include MSM or sexual minority self-report status tend to result in small sample sizes which limit the ability to conduct within-group analyses of MSM (9). For these reasons, sexual and gender minority health researchers recommend the use of multiple and novel sampling and measurement strategies for research with MSM and other sexual and gender minorities (9).
“Computable phenotypes” are increasingly being used within electronic health records to identify constructs where direct report or “gold standard” measures are not available, including for social and behavioral health constructs and prediction of HIV-related risk (10–12). While some studies have begun to apply sexual orientation re-classification methods to survey data, models for classifying sexual and gender minority populations within healthcare service databases are under-developed (6). Given the unique healthcare utilization and outcome patterns of MSM—e.g., frequent HIV testing and use of novel biomedical HIV prevention strategies like pre-exposure prophylaxis—linked public health testing and administrative health databases could be used to construct indicators for the identification of MSM (13). Development of MSM computable phenotype models for application within electronic health datasets would enable epidemiologic monitoring of the population-level health status of MSM, evaluation of MSM-focused interventions, and MSM population size estimates.
The British Columbia Hepatitis Testers Cohort (BC-HTC) integrates testing data on HIV and HCV with multiple administrative healthcare databases. With over one million unique individuals, the BC-HTC offers a powerful environment in which to evaluate and implement an MSM computable phenotype. In this report, we present the development, validation, and application of a novel model to classify MSM status using multiple laboratory, administrative healthcare, and public health surveillance datasets from 1990 to 2015, linked and aggregated for the purposes of public health research and monitoring.
Materials and Methods
Summary of Methods
This study included three steps, corresponding to three subsets of data from the BC-HTC, restricted to men aged 16 years and older (Figure 1). First, we used a subset of data with known MSM status (“develoment dataset”) to train (step 1) and validate (step 2) a computable phenotype model for MSM. The development dataset was randomly divided: 2/3 for model training, 1/3 for validation. We then used our model to classify the remaining records with unknown MSM status within the BC-HTC (“application dataset”) as MSM or heterosexual men (step 3).
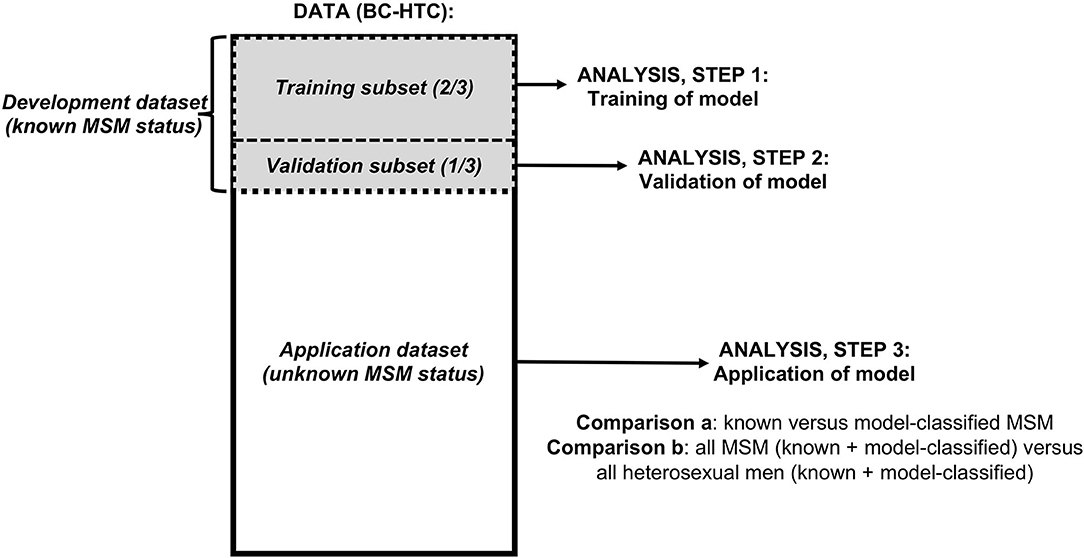
Figure 1. Flowchart of data and analyses used in study of a computable phenotype for men who have sex with men (MSM) within the British Columbia Hepatitis Testers Cohort (BC-HTC).
Data
Development Dataset
The development dataset comprised three public health case databases: the HIV/AIDS Information System (HAISYS), the Enhanced Hepatitis Strain & Surveillance System (EHSSS), and the Sexually Transmitted Infection Information System (STIIS). A flow diagram documenting the processes for data collection/acquisition and linkages has been published elsewhere (https://ndownloader.figshare.com/files/4824457) (14) HAISYS records surveillance data for new diagnoses of HIV and AIDS in BC, from 1980 onward. At the time of HIV/AIDS diagnosis, detailed demographic and risk factor information—including MSM status—is documented by a provider based on information self-reported by the person diagnosed (risk factor status complete for 74% of cases). EHSSS (2000 onward) and STIIS (1988 onward) also collect risk factors—including MSM status—for new diagnoses of HBV and HCV in EHSSS and syphilis in STIIS, though data completion in these databases is lower (35% in EHSSS, 67% in STIIS). For analysis steps 1 and 2 (development of the model), any man who reported other men as sex partners, including those who reported both men and women as sex partners, was classified as MSM. The recall time-frame for MSM status is not explicitly defined though is generally interpreted by public health nurses as representing partners since last clinic visit, or ever if first clinic visit.
Application Dataset
The application dataset included all men in the BC-HTC with unknown MSM status. The BC-HTC includes all individuals (~1.7 million) tested for HCV or HIV at the BC Center for Disease Control Public Health Laboratory (BCCDC-PHL), or reported to public health as a confirmed case of HCV, HBV, or HIV/AIDS, since 1990 (refer to additional publications for more details) (3, 14). The cohort is linked with population-based health databases including those that capture medical visits, hospitalizations, prescription drugs, cancers, and deaths (Supplementary Table 1). More than 95% of HCV and HIV serology, all HIV confirmatory testing, and all HCV RNA testing in BC are performed at the BCCDC-PHL, and thus captured in the BC-HTC.
Variable Selection
Variables for model building were selected based on theoretical and empirical knowledge about social and health characteristics of MSM (4, 15) and on expert knowledge of the BC-HTC datasets. These variables included: HIV and sexually transmitted infection (STI) testing frequency, previous STI diagnoses, substance use, visit to a clinic providing services to gay and bisexual men (16), prescription for HIV pre-exposure prophylaxis, and residence in area with higher percentage of MSM (Supplementary Table 2). At the time of this study, pre-exposure prophylaxis was recommended by Health Canada for HIV-negative MSM deemed at high risk of acquiring HIV but not publicly funded (17).
Analysis
Step 1: Training the Model
We used the training data (Figure 1) to develop the computable phenotype model. All of the variables described above were included in all models; i.e., the models differed only based on penalization weights, as follows. Many of the explanatory variables were correlated. To select an optimal model accounting for our correlated predictors, we used penalized maximum likelihood—specifically, we used the elastic-net penalty—to fit a logistic regression model. The elastic-net is a weighted combination of the penalties from lasso regression and from ridge regession. The lasso penalty leads to estimated regression coefficients of zero for less important predictors, effectively removing these predictors form the model. The ridge regression penalty encourages sharing of information between correlated predictors. The elastic-net thus leads to parsimonious estimated models with reduced risk of overfitting (18).
In elastic-net there are two tuning parameters, α and λ, that together determine the penalty term: α is a weighting factor from 0 to 1 that determines the balance between lasso regression (α = 1) and ridge regression (α = 0); λ is the parameter controlling the overall strength of the combined penalty. The glmnet package can estimate λ to optimize the area under the receiver operator characteristic curve (AUC) but does not provide any support in α tuning. Therefore, we developed models for 11 different values of α (0, 0.1, 0.2, 0.3, 0.4, 0.5, 0.6, 0.7, 0.8, 0.9, 1) so as to maximize AUC, using the validation dataset. We used the glmnet package (version 2.0–16) to fit the elastic-net model and also the caret package to explore the joint optimization of both α and λ caret (version 6.0–84). Both packages were used with the R statistical software (version 3.5.3).
Step 2: Validation of Model Perfomance
The performance of the model was assessed by estimating AUC in both the training and the validation subsets of the development data. The difference in AUC between the development and validation subsets, AUCdiff, was estimated as a measure of optimism due to overfitting. This process was repeated 1,000 times, and we computed the average of 1,000 AUCdiff (Supplementary Figure 1).
Step 3: Application
The selected model was applied to adult men in the entire BC-HTC to estimate the number of MSM in the sample. Two within-sample comparisons were made, quantifying relative differences using prevalence rate ratios with 95% confidence intervals (CI). First, we compared the characteristics of known MSM to model-classified MSM in the application dataset (excluding those with known MSM status). Second, we compared the characteristics of all MSM (known and model-classified) with men who have sex exclusively with women (hereafter, heterosexual men) in the total BC-HTC. Explanatory variables included age, substance use, mental health, STI and blood-borne infection (STBBI) diagnoses, tuberculosis diagnoses, and measures of area-level material and social deprivation (19). Given our large sample size, we have based our intrpetations of the relevance of any differences by their magnitude rather than their p-values.
Two sensitivity analyses were conducted to further evaluate the validity of the prediction model. First, we stratified the BC-HTC dataset by those with at least one STI or HIV diagnosis and those with no STI or HIV diagnosis. Second, we stratified the BC-HTC dataset by those with and without an HCV diagnosis. These analyses were performed in order to investigate the potential effects of differential information bias (misclassification) introduced by using set of predictors that relate to particular STI, HIV, and HCV risk factors.
Results
Table 1 provides the information regarding the sexual behavior of the individuals in the development dataset (i.e., HAISYS, EHSSS, and STIIS databases). The individuals whose status was unknown were excluded from the further analysis. Overall sexual behavior data was available from 25,898 individuals in the development dataset, constituting 66% of all records in these three surveillance databases. 6,280 (24.2%) were identified as MSM, and 19,618 (75.8%) were identified as heterosexual. By database, 20% of EHSSS cases, 63% of HAISYS cases, and 20% of STIIS cases (gonorrhea, chlamydia, and syphilis) were MSM. The charactertics of known MSM and non-MSM in the development dataset are provided in Table 2.
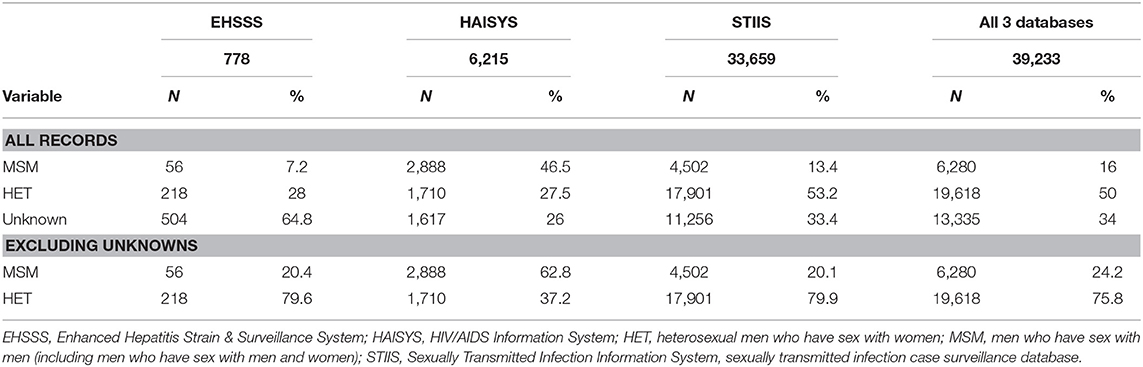
Table 1. Proportion of known MSM from different data sources included in development dataset for the development of a computable phenotype model for men who have sex with men within the British Columbia Hepatitis Testers Cohort.
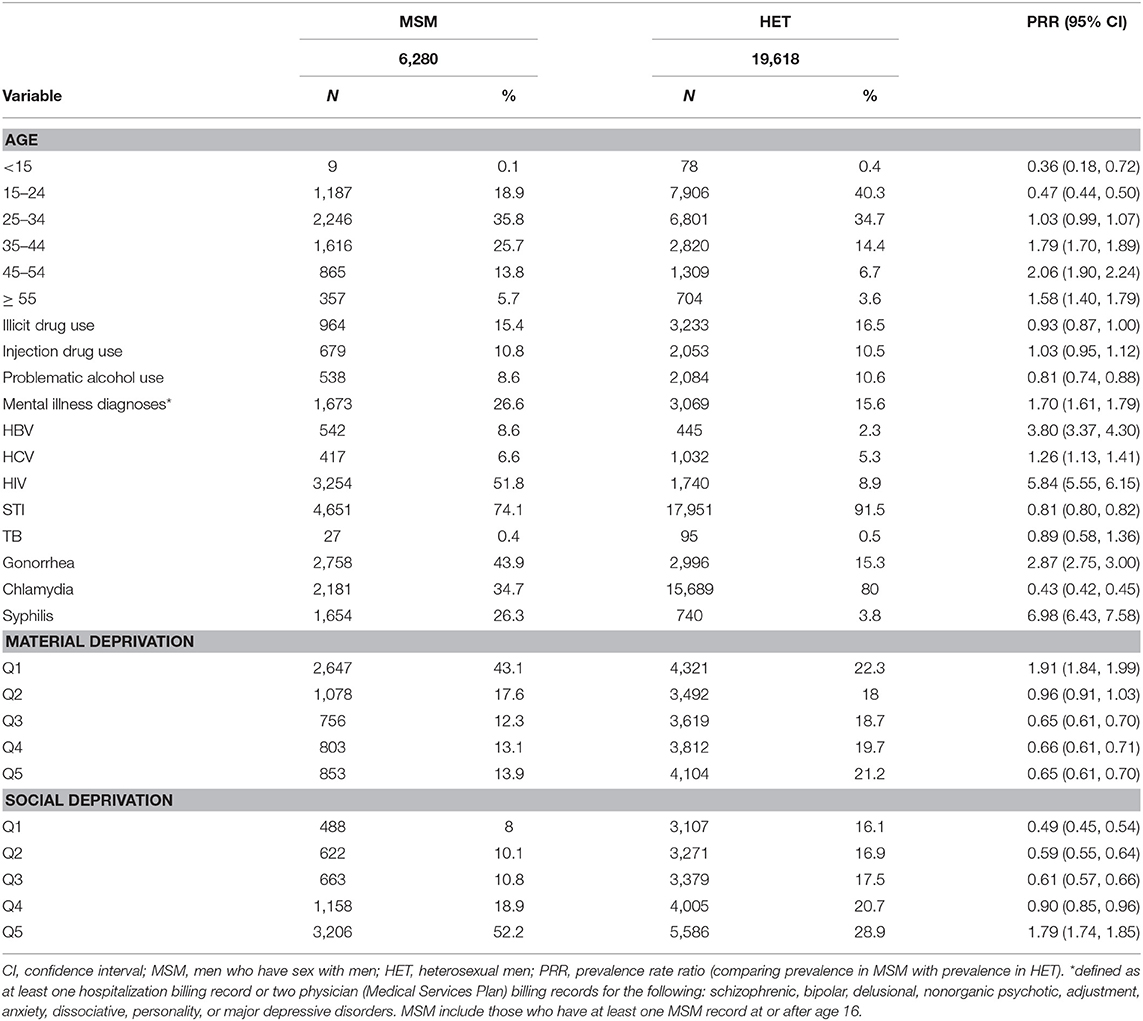
Table 2. Characteristics of self-reported men who have sex with men and heterosexual men, HIV, hepatitis, and sexually transmitted infection surveillance case reports, British Columbia, 1980–2015.
Model Selection
ROC curves from all models overlapped (Supplementary Figure 2), and AUC, sensitivity, and specificity were relatively constant for all values of α (Supplementary Tables 3, 4). Application of these models to the validation dataset showed similar results. We therefore selected the model with α = 0.4 because this model takes advantage of both ridge and lasso models (nearly equal weight) and had higher sensitivity without compromising AUC and specificity. Application of caret selected similar values of α and λ and yielded a comparable AUC (0.922) (Supplementary Table 5).
Relationship of Explanatory Variables With MSM Status
History (yes/no) of gonorrhea and syphilis diagnoses, number of HIV tests in the past year, history of visit to an identified gay and bisexual men's clinic, and residence in neighborhoods with higher MSM density were all positively associated with being MSM, with odds ratios > 1.20 (Figure 2). History (yes/no) of chlamydia diagnosis, diagnosis of drug misuse, and diagnosis of alcohol misuse were all inversely associated with being MSM, with odds ratios < 0.83. All other variables had more moderate associations with MSM status (0.83 < OR < 1.20). ORs for variables at other values of α are shown in Supplementary Table 6.
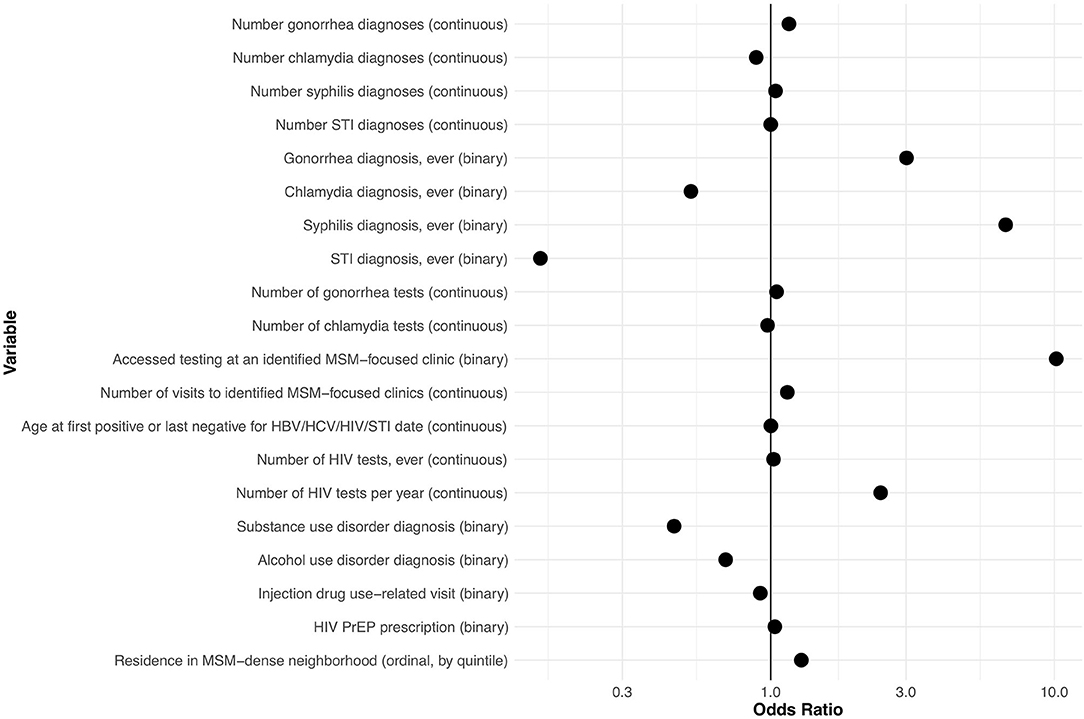
Figure 2. Odds ratios for explanatory variables in a computable phenotype model for men who have sex with men within the British Columbia Hepatitis Testers Cohort. HBV, hepatitis B virus; HCV, hepatitis C virus; MSM, men who have sex with men; PrEP, pre-exposure prophylaxis; STI, sexually transmitted infection (see Supplementary Table 2 for detailed definitions of variables).
Characteristics of Classified MSM and Heterosexual Men in BC-HTC
Table 3 provides information regarding the characterisitics of MSM and heterosexual men model-classified within the BC-HTC. A total of 727,091 records were available from adult men in the BC-HTC, 1990–2015, including the 25,898 individuals from the development dataset. Excluding the development dataset, a total of 85,521 MSM (of 701,193 adult men, without sexual behavioral data; 12%) were classified as MSM within the BC-HTC. Model-classified MSM (N = 85,521) were older than known MSM (N = 6,280). Fewer model-classified MSM had a history of substance use, mental health diagnoses, or history of STBBI, as compared with known MSM. Proportionate distribution of residence by quintiles of material and social deprivation were comparable between model-classified and known MSM.
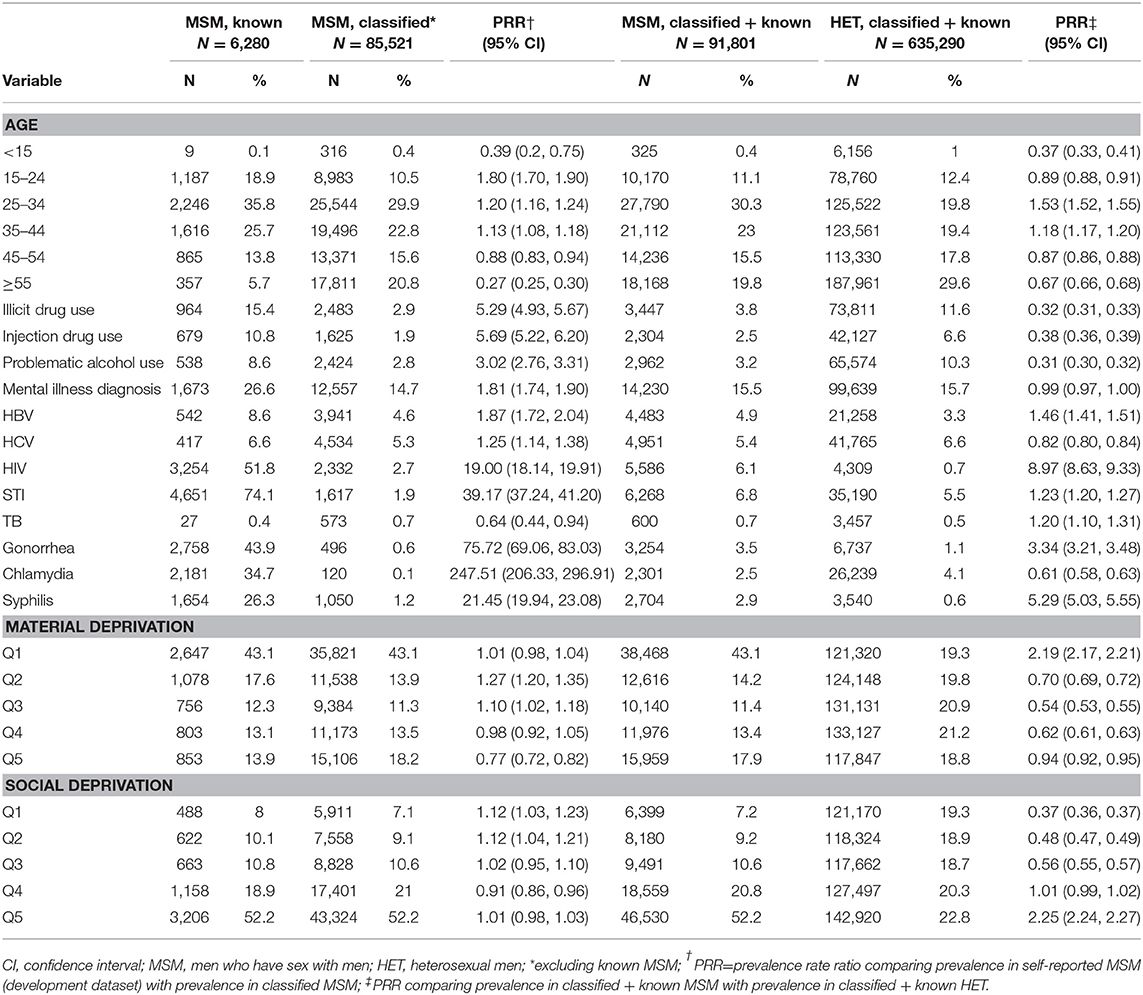
Table 3. Characteristics of known and classified men who have sex with men and heterosexual men in the British Columbia hepatitis testers cohort.
From Table 3, several differences were discernable while comparing the MSM (both model-classified and known) with heterosexual men (model-classified and known). Proportionately more MSM (42%) than heterosexual men (33%) were <35 years of age. Substance use (including illicit drug use, injection drug use, and alcohol use) was more common among heterosexual men than among MSM. HIV, HBV, gonorrhea, and syphilis, diagnoses were more common among MSM, while HCV diagnoses were more common among heterosexual men. As in the development dataset, more MSM lived in neighborhoods in the lowest quintile of material deprivation (least materially deprived) and those in the highest quintile of social deprivation (most socially deprived), as compared with heterosexual men.
Although the absolute value of the percentages of model-classified MSM and heterosexual men with these characteristics changed with stratification by STBBI history, the relative comparisons between MSM and heterosexual men all remained unchanged (Supplementary Tables 7, 8).
Discussion
In this study, we applied a computable phenotyping approach to develop a model to classify MSM within a large population-based administrative cohort with over 1 million individuals accessing HIV and HCV testing. This model includes history of STBBI and STBBI testing, use of MSM-tailored clinical services, lack of drug or alcohol misuse diagnoses, and residence in MSM-dense neighborhoods. The selected model had a sensitivity of 72% and specificity of 94% and ultimately classified 12% (N = 85,521) of the HIV/HCV testing cohort as MSM.
Interpretation of Findings
We interpret and evaluate our model with respect to its performance, sub-group comparisons within the BC-HTC, and external validity, in relation to the larger MSM literature. In within-sample comparisons of our model, we found that MSM and heterosexual men were similar between the known-MSM-status datasets and the larger BC-HTC, with a few exceptions. On average, known MSM were older than known heterosexual men, while model-classified MSM were younger than model-classified heterosexual men. This may be partially attributable to the fact that most known MSM came from HIV, gonorrhea, or syphilis case reports—STI diagnoses that tend to occur at older ages (median age among men in BC: 36 years for HIV, 31 years for gonorrhea, 42 years for syphilis)—while most known heterosexual men came from chlamydia case reports, which tend to occur at younger ages (median age among men in BC: 26 years) (20).
Demographic comparisons between model-classified MSM and heterosexual men showed that MSM were younger than heterosexual men, less likely to live in a neighborhood with material deprivation, and more likely to live in a neighborhood with social deprivation. Whether MSM are indeed younger than heterosexual men is difficult to evaluate; theoretical plausibility for this trend comes from generational effects in reduction in the stigma attached to same-gender sexual relations (21), i.e., younger men are more likely to engage in same-gender sexual relationships because they have come of age in a more supportive social environment. However, empirical data comparing the age distributions of sexual minority and heterosexual men have yielded mixed results—likely owing to differences in samples and variables used to identify sexual minorities (i.e., behavioral definitions vs. identity-based definitions of sexual orientation) (15). We may have differentially misclassified older MSM (i.e., with lower sensitivity) because of the predictive variables included in our model. For example, one of the predictors with the largest coefficients was having visited MSM-targeted STBBI testing clinics, some of which are disproportionately accessed by younger men.
The observed patterns of residence in low-material-deprivation and high-social-deprivation neighborhoods can be explained by more carefully examining the composite variables in each of these deprivation indices. The material deprivation index reflects local aggregate measures of education, employment, and income (19). Although most data regarding the socio-economic status of sexual minority men suggests that they experience levels of education, employment, and income that are comparable to or lower than those of heterosexual men (22), the use of certain explanatory variables such as access to MSM-focused clinics, may have biased our MSM predictions to more affluent neighborhoods. The social deprivation index reflects local aggregate measures of marital status and family structure (19). The positive association between predicted MSM status and residence in high-social-deprivation neighborhoods is consistent with other literature that demonstrates that sexual minority men are substantially less likely than heterosexual men to be partnered or married, or to have children (23). Both of these measures, however, rely upon heteronormative assumptions about family and household composition; as noted in other analyses applying area-level measures of social and economic structural factors to sexual minority health, there is a need for the development of sexual minority-specific social and material deprivation measures (24, 25).
Comparative estimates of the burden of STBBI between MSM and heterosexual men, using our computable phenotype model, were consistent with those described elsewhere in the literature on MSM health. In particular, the elevated cumulative prevalence of HIV, gonorrhea, and syphilis in MSM, relative to heterosexual men, are in the same direction as, though smaller in magnitude than, comparative estimates from other studies in North America (2, 26). By contrast, our finding that MSM were no more likely than heterosexual men to experience mental health or substance use disorders is at odds with the multiple systematic reviews that demonstrate a robust 2–4-fold disparity for these outcomes (5–7). The lack of a difference in our dataset between MSM and heterosexual men may be explained by the nature of the population. Our cohort by definition includes individuals at elevated risk of STBBI. Given numerous studies that show associations between STBBI and “syndemic” factors like mental health and substance use disorders (25, 27), it is perhaps not surprising that we failed to detect differences by sexual orientation within this cohort. While most of the above-highlighted findings are consistent with the larger MSM literature, the particular and novel contribution of our study is the development and application of a computable phenotype—combining all of these predictive characteristics—in a way that can be used for in-depth research and public health monitoring within MSM sub-cohorts. We therefore offer these findings to encourage application and exploration of this model, and similar approaches, in comparable public health data-sets in settings beyond BC.
Limitations
The degree to which this cohort of MSM is representative of MSM in the general population (i.e., including those not accessing HIV/HCV testing) remains to be determined. Future research should employ cross-sample comparisons to better understand which MSM are captured in each of the respective study designs currently employed in MSM and other sexual and gender minority health research. These typically include non-probability venue-based samples, probabilitistic general population samples using self-identification questions, and network-based samples, such as those that employ respondent-driven sampling (28). We note that the number of MSM identified in the BC-HTC (85,521) far exceeds provincial estimates of MSM derived using other methods, e.g., 50,900 in one recent analysis (29). This difference is likely explained by the 94% specificity of our model; i.e., a 6% “false positive” misclassification rate applied to a large population of N = 635,290 heterosexual men produces as many as 38,117 heterosexual men who are misclassified as MSM. Future work is needed to improve the specificity of this model, likely through addition of new explanatory variables that may be added as the BC-HTC expands.
We suggest that, as with all observational epidemiologic research, the MSM phenotypic sub-cohort of the BC-HTC will offer some methodologic strengths and some limitations in relation to the other sampling designs. Major strengths of our approach include the large sample size, the ability to integrate multiple data sources, and direct applicability to questions of public health relevance, due to the definitional basis of the cohort including those accessing HIV and hepatitis tests. To optimize classification characteristics, we used elastic-net, implemented with the GLMNet package in R. The elastic-net approach can be particularly beneficial when there are many possibly correlated explanatory variables and there is a concern about the potential for overfitting the regression models.
Drawbacks of our computational phenotype model include misclassification of MSM, a selection bias to disproportionately represent MSM at highest risk of STBBI, and the lack of detailed, self-reported health measures that are typically captured in surveys. Future studies may address these limitations by modeling the effects of misclassification—including those stemming from underreporting of MSM status during STBBI case surveillance (6). Relatedly, MSM status was missing for 34% of all case surveillance records, which may have limited the validity of our model. We further acknowledge that there are other MSM-related explanatory variables that should be explored in future analyses but were unfortunately not available in our dataset. These may include household characteristics (e.g., genders of other household members) and other health characteristics (e.g., receipt of vaccines recommended to sexually active MSM), among others. Future studies should similarly explore more parsimonious models that reduce the number of explanatory variables, including models that may sacrifice sensitivity to achieve high specificity.
Finally, we acknowledge the critical importance of ensuring that patient privacy is protected in all stages of computable phenotyping—particularly for a topic that remains stigmatized in our society (i.e., sexual minority status). As others have asserted (10, 30), technological advances associated with big data that enable new analyses for public good need not (and must not) compromise principles of confidentiality. The BC-HTC uses several measures to ensure protection of sensitive data, including: (a) de-identification of linked data used for analysis; (b) storing all data within a robust security system (14); and (c) ensuring there are no ways for newly derived variables (e.g., model-classified MSM status) to return to patient charts or other electronic databases where individual characteristics could be read or misused. We further suggest that there is a need for ongoing research and monitoring to understand sexual minority community perspectives on the use of computable phenotype tools—both to understand how these methods can be applied to urgent questions of community interest and to address any real or perceived threats to individual privacy, community representations, and other ethical concerns yet to be established.
Conclusions
Computable phenotyping is a promising approach for the identification of sexual and gender minorities, in order to strengthen efforts in population health monitoring, particularly in the absence of routinely available self-report data. Our computable phenotype model had classification characteristics similar to interviewer-elicited survey measures. This method may ultimately allow for larger samples and triangulation between other sexual minority samples, with their own particular limitations (9). In this context, we recommend greater application and exploration of computable phenotyping in sexual minority health research.
Data Availability Statement
The data analyzed in this study is subject to the following licenses/restrictions: Data are sensitive in nature and stewarded by appropriate public health authorities in British Columbia. Requests to access these datasets should be directed to bmF2ZWVkLmphbmp1YUBiY2NkYy5jYQ==.
Ethics Statement
This study was reviewed and approved by the University of British Columbia Research Ethics Board (H14-01649). Written informed consent for participation was not required for this study in accordance with the national legislation and the institutional requirements.
Author Contributions
ZB, RB, and NJ: study design. ZB, SW, JW, AY, MA, CR, MG, MK, and NJ: collection and construction of data cohort. TS, SW, and YA: analysis. TS and ZB: preparation of manuscript. TS, ZB, SW, YA, RB, and NJ: tables and figures. AR, AA, JW, TG, MG, and NJ: contributions to literature review, interpretation, and discussion of model results. All authors contributing to drafting this manuscript and have approved it for submission.
Funding
This work was supported by British Columbia Centre for Disease Control; Canadian Institutes of Health Research [NHC-142832, PJT-156117, PJT-156066, PHE-141773].
Disclaimer
All inferences, opinions, and conclusions drawn in this manuscript are those of the authors, and do not reflect the opinions or policies of the Data Steward(s).
Conflict of Interest
TG was an investigator on research grants funded by Gilead and Merck. MK has received grant funding via his institution from Roche Molecular Systems, Boehringer Ingelheim, Merck, Siemens Healthcare Diagnostics, and Hologic Inc.
The remaining authors declare that the research was conducted in the absence of any commercial or financial relationships that could be construed as a potential conflict of interest.
Acknowledgments
We acknowledge the assistance of BCCDC, PHSA Performance Measurement and Reporting, Information Analysts, Ministry of Health Data Access, Research and Stewardship, MSP, DAD, Medical Beneficiary and Pharmaceutical Services program areas, BC Ministry of Health, BC Cancer and their staff involved in data access, procurement and data management. We gratefully acknowledge the residents of British Columbia whose data are integrated in the BC-HTC and for whom this work is intended to benefit.
Supplementary Material
The Supplementary Material for this article can be found online at: https://www.frontiersin.org/articles/10.3389/fdgth.2020.547324/full#supplementary-material
References
1. Mohammed H, Mitchell H, Sile B, Duffell S, Nardone A, and Hughes G. Increase in sexually transmitted infections among men who have sex with men, England, 2014. Emerg Infect Dis. (2016) 22:88–91. doi: 10.3201/eid2201.151331
2. Stenger MR, Pathela P, Anschuetz G, Bauer H, Simon J, Kohn R, et al. Increases in the rate of neisseria gonorrhoeae among gay, bisexual and other men who have sex with men-findings from the sexually transmitted disease surveillance network 2010-2015. Sex Transm Dis. (2017) 44:393–7. doi: 10.1097/OLQ.0000000000000623
3. McKee G, Butt ZA, Wong S, Salway T, Gilbert M, Wong J, et al. Syndemic characterization of HCV, HBV, and HIV co-infections in a large population based cohort study. EClinicalMedicine. (2018) 4-5:99–108. doi: 10.1016/j.eclinm.2018.10.006
4. Beyrer C, Baral SD, van Griensven F, Goodreau SM, Chariyalertsak S, Wirtz AL, et al. Global epidemiology of HIV infection in men who have sex with men. Lancet. (2012) 380:367–77. doi: 10.1016/S0140-6736(12)60821-6
5. Plöderl M, and Tremblay P. Mental health of sexual minorities. a systematic review. Int Rev Psychiatry. (2015) 27:367–85. doi: 10.3109/09540261.2015.1083949
6. Salway T, Plöderl M, Liu J, and Gustafson P. Effects of multiple forms of information bias on estimated prevalence of suicide attempts by sexual orientation: an application of a Bayesian misclassification correction method to data from a systematic review. Am J Epidemiol. (2019) 188:239–49. doi: 10.1093/aje/kwy200
7. King M, Semlyen J, Tai SS, Killaspy H, Osborn D, Popelyuk D, et al. A systematic review of mental disorder, suicide, and deliberate self harm in lesbian, gay and bisexual people. BMC Psychiatry. (2008) 8:70. doi: 10.1186/1471-244X-8-70
8. Kamen C, Burns M, and Beach SR. Minority stress in same-sex male relationships: when does it impact relationship satisfaction? J Homosex. (2011) 58:1372–90. doi: 10.1080/00918369.2011.614904
9. Meyer IH, and Wilson PA. Sampling lesbian, gay, and bisexual populations. J Couns Psychol. (2009) 56:23–31. doi: 10.1037/a0014587
10. Hripcsak G, and Albers DJ. Next-generation phenotyping of electronic health records. J Am Med Inform Assoc. (2013) 20:117–21. doi: 10.1136/amiajnl-2012-001145
11. Krakower DS, Gruber S, Hsu K, Menchaca JT, Maro JC, Kruskal BA, et al. Development and validation of an automated HIV prediction algorithm to identify candidates for pre-exposure prophylaxis: a modelling study. Lancet HIV. (2019) 6:e696–e704. doi: 10.1016/S2352-3018(19)30139-0
12. Marcus JL, Hurley LB, Krakower DS, Alexeeff S, Silverberg MJ, and Volk JE. Use of electronic health record data and machine learning to identify candidates for HIV pre-exposure prophylaxis: a modelling study. Lancet HIV. (2019) 6:e688–95. doi: 10.1016/S2352-3018(19)30137-7
13. Beyrer C. Strategies to manage the HIV epidemic in gay, bisexual, and other men who have sex with men. Curr Opin Infect Dis. (2014) 27:1–8. doi: 10.1097/QCO.0000000000000037
14. Janjua NZ, Kuo M, Chong M, Yu A, Alvarez M, Cook D, et al. Assessing hepatitis C burden and treatment effectiveness through the british columbia hepatitis Testers Cohort (BC-HTC): design and characteristics of linked and unlinked participants. PLoS ONE. (2016) 11:e0150176. doi: 10.1371/journal.pone.0150176
15. Institute of Medicine. The Health of Lesbian, Gay, Bisexual, and Transgender People: Building a Foundation for Better Understanding. Washington, DC: National Academies Press (2011).
16. Gilbert M, Cook D, Steinberg M, Kwag M, Robert W, Doupe G, et al. Targeting screening and social marketing to increase detection of acute HIV infection in men who have sex with men in Vancouver, British Columbia. AIDS. (2013) 27:2649–54. doi: 10.1097/QAD.0000000000000001
17. Tan DHS, Hull MW, Yoong D, Tremblay C, O'Byrne P, Thomas R, et al. Canadian guideline on HIV pre-exposure prophylaxis and nonoccupational postexposure prophylaxis. Can Med Assoc J. (2017) 189:E1448–58. doi: 10.1503/cmaj.170494
18. Friedman J, Hastie T, and Tibshirani R. Regularization paths for generalized linear models via coordinate descent. J Stat Softw. (2010) 33:1–22. doi: 10.18637/jss.v033.i01
19. Pampalon R, Hamel D, Gamache P, Philibert MD, Raymond G, and Simpson A. An area-based material and social deprivation index for public health in Québec and Canada. Can J Public Health. (2012) 103(8 Suppl. 2):S17–22. doi: 10.1007/BF03403824
20. Karamouzian M, Mirzazadeh A, Shokoohi M, Khajehkazemi R, Sedaghat A, Haghdoost AA, et al. British Columbia Centre for Disease Control. STI in British Columbia: Annual Surveillance Report 2016. Vancouver, BC (2018). Available online at: http://www.bccdc.ca/health-professionals/data-reports/communicable-diseases/sexually-transmitted-infections
21. Hammack PL, Frost DM, Meyer IH, and Pletta DR. Gay men's health and identity: social change and the life course. Arch Sex Behav. (2017) 47:59–74. doi: 10.1007/s10508-017-0990-9
22. Brennan DJ, Ross LE, Dobinson C, Veldhuizen S, Steele LS, Brennan DJ, Ross LE, Dobinson C, et al. Men's sexual orientation and health in Canada. Can J Public Heal. (2010) 101:255–8. doi: 10.1007/BF03404385
23. Operario D, Gamarel KE, Grin BM, Lee JH, Kahler CW, Marshall BD, et al. Sexual minority health disparities in adult men and women in the united states: national health and nutrition examination survey, 2001-2010. Am J Public Health. (2015) 105:e27–34. doi: 10.2105/AJPH.2015.302762
24. Salway T, Gesink D, Lukac C, Roth D, Ryan V, Mak S, et al. The spatial-temporal epidemiology of the syphilis epidemic in British Columbia, 2005-2016. Sex Transm Dis. (2019) 46:571–78. doi: 10.1097/OLQ.0000000000001034
25. Butt ZA, Shrestha N, Wong S, Kuo M, Gesink D, Gilbert M, et al. A syndemic approach to assess the effect of substance use and social disparities on the evolution of HIV/HCV infections in British Columbia. PLoS ONE. (2017) 12:e0183609. doi: 10.1371/journal.pone.0183609
26. Purcell DW, Johnson CH, Lansky A, Prejean J, Stein R, Denning P, et al. Estimating the population size of men who have sex with men in the United States to obtain HIV and syphilis rates. Open AIDS J. (2012) 6:98–107. doi: 10.2174/1874613601206010098
27. Tsai AC, Mendenhall E, Trostle JA, and Kawachi I. Co-occurring epidemics, syndemics, and population health. Lancet. (2017) 389:978–82. doi: 10.1016/S0140-6736(17)30403-8
28. Brennan DJ, Bauer GR, Bradley K, and Tran OV. Methods used and topics addressed in quantitative health research on gay, bisexual and other men who have sex with men: a systematic review of the literature. J Homosex. (2017) 64:1519–38. doi: 10.1080/00918369.2016.1247537
29. The Center for Global Health (University of Manitoba). Estimates of key population size of people who use injection drugs (PWID), men who have sex with men (MSM) and sex workers (SW) who are at risk of acquiring HIV and hepatitis C in the five health regions of the province of British Columbia. (2016). Available online at: http://www.bccdc.ca/resource-gallery/Documents/Statistics%20and%20Research/Statistics%20and%20Reports/STI/PSE%20Project%20Final%20Report.pdf
Keywords: sexual and gender minorities, computable phenotypes, big data, HIV, administrative data
Citation: Salway T, Butt ZA, Wong S, Abdia Y, Balshaw R, Rich AJ, Ablona A, Wong J, Grennan T, Yu A, Alvarez M, Rossi C, Gilbert M, Krajden M and Janjua NZ (2020) A Computable Phenotype Model for Classification of Men Who Have Sex With Men Within a Large Linked Database of Laboratory, Surveillance, and Administrative Healthcare Records. Front. Digit. Health 2:547324. doi: 10.3389/fdgth.2020.547324
Received: 03 June 2020; Accepted: 02 September 2020;
Published: 06 October 2020.
Edited by:
Harry Hochheiser, University of Pittsburgh, United StatesCopyright © 2020 Salway, Butt, Wong, Abdia, Balshaw, Rich, Ablona, Wong, Grennan, Yu, Alvarez, Rossi, Gilbert, Krajden and Janjua. This is an open-access article distributed under the terms of the Creative Commons Attribution License (CC BY). The use, distribution or reproduction in other forums is permitted, provided the original author(s) and the copyright owner(s) are credited and that the original publication in this journal is cited, in accordance with accepted academic practice. No use, distribution or reproduction is permitted which does not comply with these terms.
*Correspondence: Travis Salway, dHJhdmlzX3NhbHdheUBzZnUuY2E=; Naveed Z. Janjua, bmF2ZWVkLmphbmp1YUBiY2NkYy5jYQ==