- 1Department of Cardiology, Shanghai Tenth People's Hospital, Tongji University School of Medicine, Shanghai, China
- 2Department of Cardiology, Tongji Hospital, Tongji University School of Medicine, Shanghai, China
Background: Acute myocardial infarction (AMI) is among the leading causes of death worldwide. Patients with AMI may have the risk of developing recurrent cardiovascular events leading to rehospitalization or even death. The present study aimed to investigate the prediction factors of poor prognosis (mortality and/or readmission) after AMI during a 6-month follow-up.
Methods: A total of 206 consecutive patients hospitalized for the first visit with AMI were enrolled. Data collection included demographic characteristics, medical history, clinical information, laboratory results, and oral medications within 24 h of admission. At 1, 3, and 6 months after discharge, AMI patients were followed up to assess the occurrence of composite endpoint events including in-hospital and out-of-hospital death and/or readmission due to recurrent myocardial infarction (MI) or exacerbated symptoms of heart failure following MI.
Results: After 6-month follow-up, a total of 197 AMI patients were available and divided in two groups according to good prognosis (n = 144) and poor prognosis (n = 53). Our data identified serum myoglobin ≥651 ng/mL, serum creatinine ≥96 μM, Killip classification 2–4, and female gender as independent predictors of 6-month mortality and/or readmission after AMI. Moreover, we demonstrated that Killip classification 2–4 combined with either myoglobin (AUCKillip class 2−4+myoglobin = 0.784, sensitivity = 69.8%, specificity = 79.9%) or creatinine (AUCKillip class 2−4+creatinine = 0.805, sensitivity = 75.5%, specificity = 77.1%) could further enhance the predictive capacity of poor 6-month prognosis among AMI patients.
Conclusions: Patients with AMI ranked in the higher Killip class need to be evaluated and monitored with attention. Multibiomarker approach using Killip classification 2–4 and myoglobin or creatinine may be an effective way for 6-month prognosis prediction in AMI patients.
Introduction
Acute myocardial infarction (AMI) is among the leading causes of death worldwide (1, 2). Despite that great progress has been made in the pharmacological and interventional therapy of AMI, patients with AMI may have the risk of developing recurrent cardiovascular events leading to rehospitalization or even death (3, 4). A deep understanding of the prediction factors of AMI prognosis can provide important information for disease stratification and clinical treatment of patients.
Because of the rapid advancement of laboratory techniques, a number of biomarkers have been identified for diagnosis of AMI. Among the biomarkers of cardiac necrosis injury, cardiac troponins, especially troponin I (cTnI) and troponin T, are considered as highly specific and sensitive markers of AMI diagnosis (5). Myoglobin and creatine kinase-MB (CK-MB), although with less specificity, are also valuable diagnostic biomarkers because of their rapid elevation in the early stage of AMI (6). For prognosis of AMI, cardiac troponins, brain natriuretic peptide (BNP), and N-terminal pro–brain natriuretic peptide (NT-proBNP) were proved to have prognostic values of heart failure and/or mortality in AMI patients (7, 8). In addition, increased heart-type fatty acid–binding protein and C-reactive protein (CRP) levels were reported to be possibly predictive of heart failure or mortality during the follow-up of AMI patients (9, 10). However, there is actually no gold standard prognostic biomarker for AMI (11). Multiple factors such as demographics, clinical presentations, and comorbidities are associated with AMI prognosis (12–14). Clinical studies are still highly needed to evaluate the factors predicting prognosis of AMI, especially with continuous advances in cardiovascular care (15).
In the present study, we aimed to analyze the prediction factors of poor prognosis (mortality and/or readmission) of AMI patients during a 6-month follow-up.
Patients and Methods
Patients
A total of 206 consecutive patients hospitalized for the first visit with AMI were enrolled from October 2015 to August 2017 at Department of Cardiology in Tongji Hospital affiliated to Tongji University (Shanghai, China). This cohort of patients was previously used to analyze gender-specific predictive markers of poor AMI prognosis, which was an independent analysis from the present study (16). The diagnosis of AMI was made by cardiologists according to Guidelines for the Diagnosis and Treatment of AMI in China. Those with malignant tumors, severe mental illness, and/or uncontrolled systemic diseases were excluded from the present study. The study protocol was approved by the independent ethics committee of Tongji Hospital affiliated to Tongji University (Shanghai, China). The written informed consent form was provided by all patients.
Data Collection Flow
Data collection included demographic characteristics, medical history, clinical information, laboratory results, and oral medications within 24 h of admission. At admission or on the next morning, venous blood was taken and immediately analyzed in the Core Laboratory of Tongji Hospital for examinations of blood biochemistry, markers of myocardium injury (e.g., CK, CK-MB, myoglobin, cTnI, NT-proBNP), CRP, hemoglobin A1c (HbA1c), glycated serum albumin (GSA), D-dimer, and folic acid.
Follow-Up and Primary Endpoint
At 1, 3, and 6 months after discharge, AMI patients were followed up to assess the occurrence of composite endpoint events by trained researchers. The primary endpoint events were a composite of all-cause mortality (including in-hospital and out-of-hospital death) and/or readmission due to recurrent myocardial infarction (MI) or exacerbated symptoms of heart failure following MI. During 6-month follow-up, nine patients (4.4%) were lost over time as reported before, because they provided wrong telephone number or disconnected the call (16). The primary endpoint events were eventually confirmed by patients themselves, their families, and local hospital doctors. For analysis of predictive markers, AMI patients were divided into good prognosis vs. poor prognosis groups according to the occurrence of death and/or readmission.
Statistical Analysis
Statistical analysis was performed using SPSS version 25.0 (SPSS Inc., Chicago, IL, USA) and MedCalc version 19.0.2 (MedCalc Software, Mariakerke, Belgium). Continuous variables with normal distribution were presented as mean ± standard deviation. For the cases of skewed distribution, median with interquartile range would be selected. All categorical variables and frequency of events were shown as numbers (percentage). The comparison between groups was performed with the independent-samples t-test, Mann-Whitney U test, or χ2 test as appropriate. Based on the significant (P < 0.05) variables between good prognosis and poor prognosis groups, forward stepwise COX regression analyses (entry only if P ≤ 0.10 and removal only if P > 0.10) were further applied to identify the independent predictors of 6-month prognosis. Receiver operating characteristic (ROC) curves and Kaplan–Meier curves were then constructed to determine the cutoff point and predictive value of these markers in the prediction of poor AMI prognosis. P < 0.05 was considered as statistically significant.
Results
Clinical Characteristics of AMI Patients
After 6-month follow-up, a total of 197 AMI patients were available and divided into two groups according to prognosis. Those with death and/or readmission events (n = 53) were defined as poor prognosis, whereas the other AMI patients without death and/or readmission (n = 144) were defined as good prognosis (Table 1). In patients with poor prognosis (n = 53), 41 patients were rehospitalized (including three patients died during readmission), and 15 patients died (including both in-hospital and out-of-hospital death). Demographics showed that AMI patients with poor prognosis were older than those with good prognosis (71.3 ± 14.1 vs. 61.7 ± 13.3 years, P < 0.001). Patients with AMI with poor prognosis also had lower diastolic blood pressure (70.5 ± 13.5 mmHg vs. 75.2 ± 12.2 mmHg, P < 0.05), less current or past smoking experience (43.4 vs. 61.8%, P < 0.05), and higher prevalence of diabetes mellitus (41.5 vs. 21.5%, P < 0.01) when compared to those with good prognosis. A significantly larger proportion of AMI patients with poor prognosis had Killip classification 2–4 compared to those with good prognosis (69.8 vs. 20.1%, P < 0.001). Meanwhile, AMI prognosis was analyzed according to different genders, which showed that the proportion of AMI patients with poor prognosis (including death and/or readmission) was 45.0% in females (n = 18 among 40 females), which was significantly higher than 22.3% in males (n = 35 among 157 males) (P < 0.01).
Oral medications were recorded at admission. Among the commonly used medicine, a larger proportion of AMI patients in poor prognosis group were prescribed with loop diuretics compared to those with good prognosis (41.5 vs. 12.5%, P < 0.001). No difference was found in other oral medications, including angiotensin-converting enzyme inhibitor/angiotensin receptor blocker, β-blockers, antiplatelet drugs, anticoagulant drugs, and statins, between the good prognosis and poor prognosis groups (Table 2).
Biochemical Examinations of AMI Patients
At admission or on the next morning, biochemical examinations were performed for AMI patients and were further compared between AMI patients with good and poor prognosis (Table 3). Compared to those with good prognosis, patients with poor prognosis were present with slightly lower serum levels of albumin, hemoglobin, and sodium, but higher levels of CRP, blood urea nitrogen (BUN), creatinine, and uric acid. Most of the biochemical data mentioned above had a mean value or median value within the normal range, except for BUN, creatinine, and uric acid, which were slightly above the normal range in the group of AMI patients with poor prognosis. Meanwhile, AMI patients had obviously higher levels of CK, CK-MB, myoglobin, cTnI, and NT-proBNP. Among these biochemical data indicating myocardial necrosis and heart failure, myoglobin and NT-proBNP were significantly elevated in AMI patients with poor prognosis. In particular, AMI patients with poor prognosis had a median value of NT-proBNP more than 5-fold higher than those with good prognosis [1,517.3–12,885.3 (4,448.5) U/L vs. 388.4–1,619.8 (788.4) U/L, P < 0.001]. Moreover, AMI patients with poor prognosis had slightly higher levels of HbA1c, GSA, and D-dimer when compared to those with good prognosis.
Myoglobin, Creatinine, Killip Classification 2–4, and Gender Are Independent Predictors of Poor AMI Prognosis
We then constructed multivariate COX stepwise regression analysis to identify the independent predictors of poor AMI prognosis during 6-month follow-up in the present study. With stepwise variable selection using the covariates based on the significant (P < 0.05) variables in baseline characteristics and biochemical data between good vs. poor prognosis groups, myoglobin, creatinine, Killip classification 2–4, and gender were identified as potential independent predictors of poor AMI prognosis (Table 4).
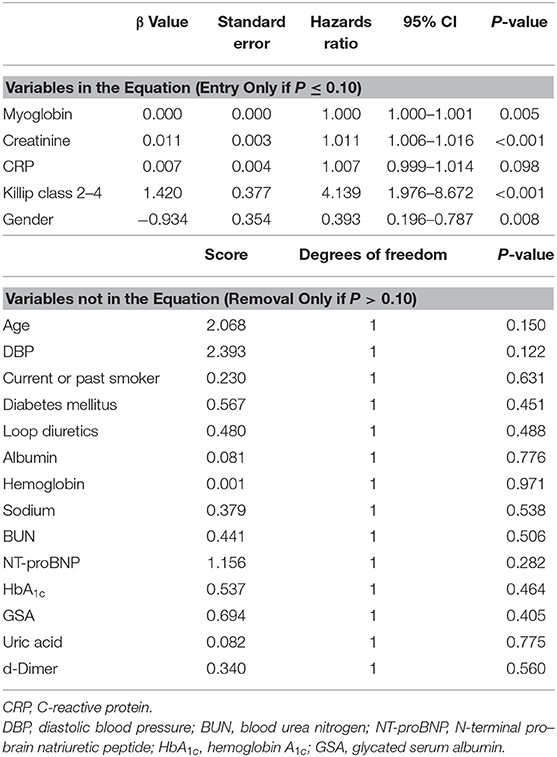
Table 4. Forward stepwise COX regression analysis (entry only if P ≤ 0.10 and removal only if P > 0.10) for poor AMI prognosis.
Receiver operating characteristic curves further demonstrated that area under the curve (AUC) was AUCmyoglobin = 0.632 (95% CI = 0.561–0.700, sensitivity = 41.5%, specificity = 82.6%), AUCcreatinine = 0.706 (95% CI = 0.637–0.768, sensitivity = 58.5%, specificity = 78.5%), AUCKillip class 2−4 = 0.748 (95% CI = 0.682–0.807, sensitivity = 69.8%, specificity = 79.9%), and AUCgender = 0.593 (95% CI = 0.521–0.663, sensitivity = 34.0%, specificity = 84.7%), respectively (Figure 1). Using the cutoff point calculated from ROC analysis, myoglobin ≥651 ng/mL, creatinine ≥96 μM, Killip classification 2–4, and female gender were found to be significant predictors of poor prognosis in AMI patients (Figure 2).
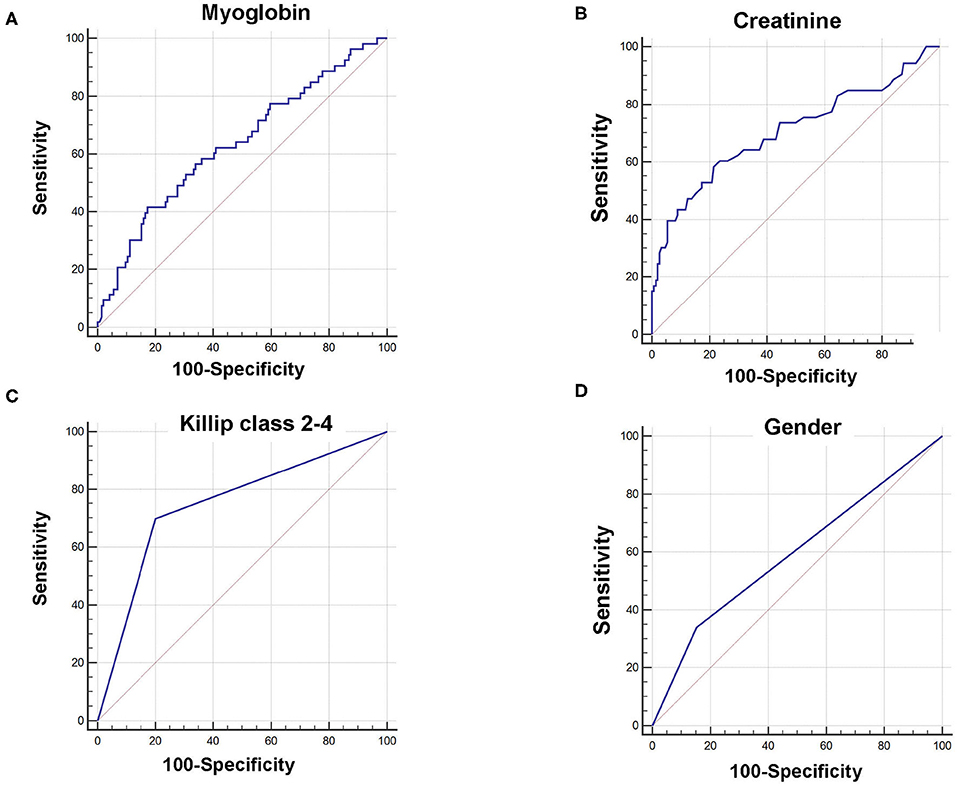
Figure 1. Receiver operating characteristic curve (ROC) of myoglobin, creatinine, Killip classification 2–4, and gender for predicting 6-month prognosis in AMI patients. (A) AUCmyoglobin = 0.632, 95% CI = 0.561–0.700, sensitivity = 41.5%, specificity = 82.6%, cutoff point: 651; (B) AUCcreatinine = 0.706, 95% CI = 0.637–0.768, sensitivity = 58.5%, specificity = 78.5%, cutoff point: 96; (C) AUCKillip class 2−4 = 0.748, 95% CI = 0.682–0.807, sensitivity = 69.8%, specificity = 79.9%. (D) AUCgender = 0.593, 95% CI = 0.521–0.663, sensitivity = 34.0%, specificity = 84.7%.
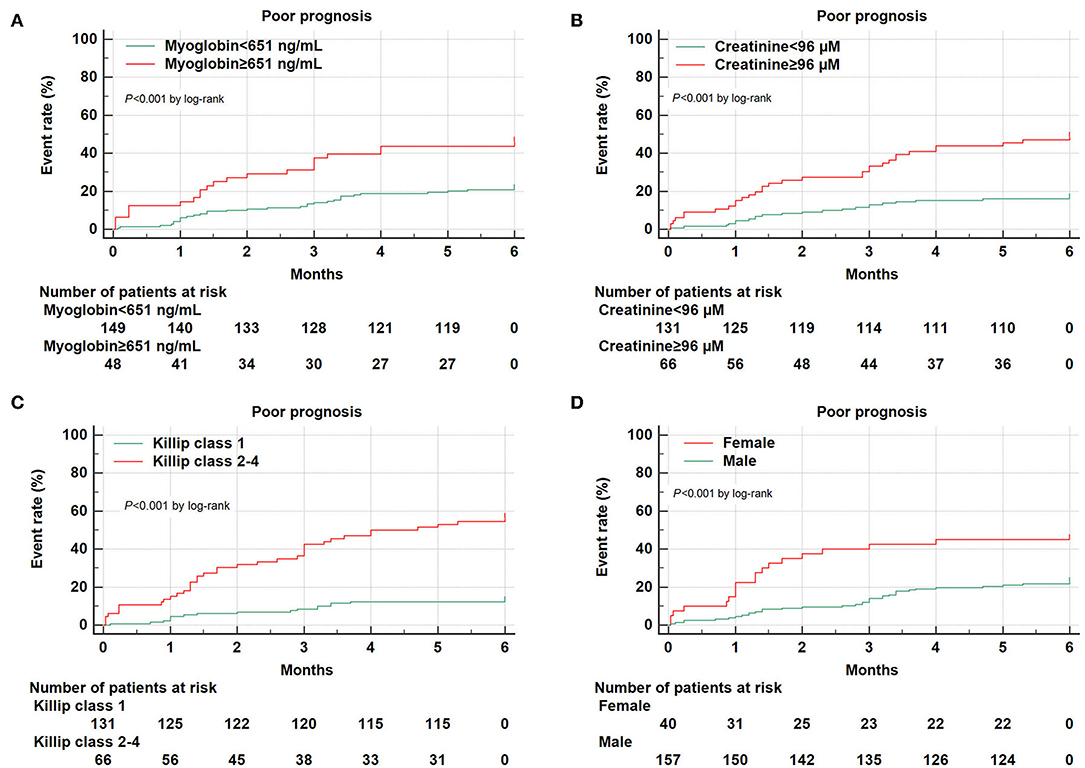
Figure 2. Kaplan–Meier survival curves for 6-month prognosis of AMI patients. Kaplan–Meier survival curves were constructed according to myoglobin (A), creatinine (B), Killip classification 2–4 (C), and gender (D).
Combined Analysis of Independent Predictors of Poor AMI Prognosis
We further performed combined analysis of these independent markers to explore whether this could further enhance their predictive capacity for poor AMI prognosis (Figure 3). We found that a combination of Killip classification 2–4 with myoglobin was sufficient to enhance AUC (AUCKillip class 2−4+myoglobin = 0.784, 95% CI = 0.720–0.839, sensitivity = 69.8%, specificity = 79.9%) when compared to AUCKillip class 2−4 or AUCMyoglobin alone (P < 0.05 and P < 0.001, respectively). Similarly, a combination of Killip classification 2–4 with creatinine was also able to enhance AUC (AUCKillip class 2−4+creatinine = 0.805, 95% CI = 0.743–0.858, sensitivity = 75.5%, specificity = 77.1%) when compared to AUCKillip class 2−4 or AUCcreatinine alone (P < 0.05 and P < 0.01, respectively).
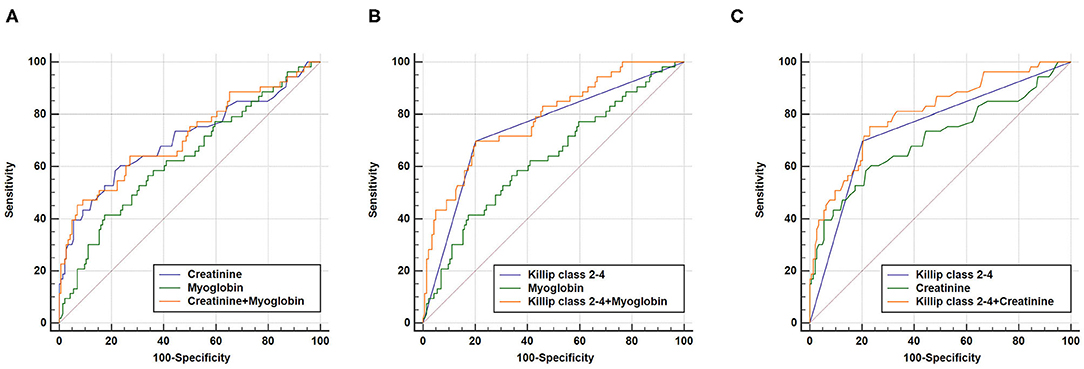
Figure 3. Combination of two independent predictors in construction of Receiver operating characteristic (ROC) curve. (A) AUCcreatinine+myoglobin = 0.713, 95% CI = 0.645–0.775, sensitivity = 45.3%, specificity = 93.1%, AUCcreatinine+myoglobin vs. AUCcreatinine (P = 0.5736), AUCcreatinine+myoglobin vs. AUCmyoglobin (P = 0.1094); (B) AUCKillip class 2−4+myoglobin = 0.784, 95% CI = 0.720–0.839, sensitivity = 69.8%, specificity = 79.9%, AUCKillip class 2−4+myoglobin vs. AUCKillip class 2−4 (P = 0.0369), AUCKillip class 2−4+myoglobin vs. AUCmyoglobin (P = 0.0003); (C) AUCKillip class 2−4+creatinine = 0.805, 95% CI = 0.743–0.858, sensitivity = 75.5%, specificity = 77.1%, AUCKillip class 2−4+creatinine vs. AUCKillip class 2−4 (P = 0.0108), AUCKillip class 2−4+creatinine vs. AUCcreatinine (P = 0.0038).
As expected, a combination of Killip class 2–4, myoglobin, and creatinine could also increase AUC (AUCKillip class 2−4+myoglobin+creatinine = 0.800, 95% CI = 0.737–0.853, sensitivity = 73.6%, specificity = 78.5%) compared to either predictive marker alone (Figure 4). Noteworthy, although AUCKillip class 2−4+myoglobin+creatinine was significantly larger than AUCcreatinine+myoglobin, no significant difference was found for AUCKillip class 2−4+myoglobin+creatinine when compared to AUCKillip class 2−4+myoglobin or AUCKillip class 2−4+creatinine. Taken together, these data suggest that a combination of Killip classification 2–4 either with myoglobin (AUCKillip class 2−4+myoglobin) or creatinine (AUCKillip class 2−4+creatinine) was sufficient to enhance the predictive capacity for AMI poor prognosis.
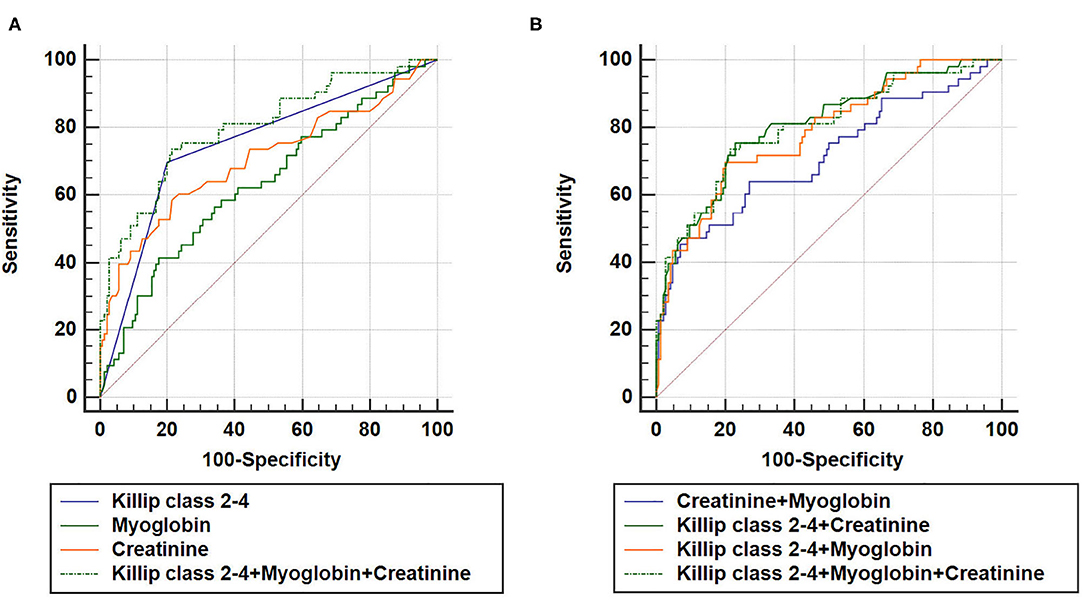
Figure 4. Combination of three independent predictors in construction of Receiver operating characteristic (ROC) curve. AUCKillip class 2−4+creatinine+myoglobin = 0.800, 95% CI = 0.737–0.853, sensitivity = 73.6%, specificity = 78.5%. (A) AUCKillip class 2−4+myoglobin+creatinine vs. AUCKillip class 2−4 (P = 0.0224), AUCKillip class 2−4+myoglobin+creatinine vs. AUCmyoglobin (P = 0.0007), AUCKillip class 2−4+myoglobin+creatinine vs. AUCcreatinine (P = 0.0088). (B) AUCKillip class 2−4+myoglobin+creatinine vs. AUCcreatinine+myoglobin (P = 0.0056), AUCKillip class 2−4+myoglobin+creatinine vs. AUCKillip class 2−4+creatinine (P = 0.3557), AUCKillip class 2−4+myoglobin+creatinine vs. AUCKillip class 2−4+myoglobin (P = 0.4398).
Discussion
A great number of AMI patients are at risk of recurrent cardiovascular events, which leads to readmission or even dearth. Biomarkers are useful in the prediction of AMI prognosis, which may differ from endpoint events and follow-up durations. Based on a cohort of 197 AMI patients followed up for 6 months, our study shows that serum myoglobin ≥651 ng/mL, serum creatinine ≥96 μM, Killip classification 2–4, and female gender are independent predictors of 6-month mortality and/or readmission. Our data also demonstrate that the combination of Killip classification 2–4 either with creatinine or myoglobin could further enhance the predictive capacity for AMI poor prognosis.
Demographic characteristics and oral medications at admission were first compared in AMI patients with good prognosis (n = 144) and poor prognosis (n = 53). Compared to those with good prognosis, patients with poor prognosis were about 10 years older and more likely to have previous history of diabetes mellitus. Aging and diabetes are both well-known risk factors for worse outcomes after MI that have strong associations with death or recurrent cardiovascular events (17, 18). The increased risk of adverse outcomes in patients with older age or diabetes is likely multifactorial, which may be explained by more complicating diseases, higher prevalence of multivessel disease, and less implementation of evidence-based therapies (19–21).
Gender-related difference exists in the assessment, treatment, and outcomes of coronary artery diseases (22, 23). Based on the same cohort of AMI patients followed up for 6 months, we previously analyzed and reported gender-specific predictive markers of poor AMI prognosis in male and female patients; in that study, although there was no significant difference in 6-month mortality between males and females, the readmission rate was significantly higher in females than in males (16). Here, when we analyzed death and readmission events together in AMI patients, we observed that 45.0% of females had death and/or readmission events during 6-month follow-up, which was significantly higher than 22.3% in males. COX regression analyses and Kaplan–Meier curves further demonstrated that female gender was an independent predictor of 6-month mortality and/or death after AMI. The predictive value of female gender in AMI prognosis may differ by age as well as by endpoint events analyzed in different studies. A great number of studies support that younger female patients with AMI were at higher risk of both short- and long-term mortality than male patients; however, this difference was diminished in the old population (24–26). Additionally, most studies used mortality as endpoint event (27–29), whereas our study defined poor prognosis including both death and hospital readmission. Indeed, a deeper understanding of the impact of gender on the outcomes of AMI may help guide better therapeutic strategies for male and female patients.
Biochemical examination data were then analyzed between AMI patients who had good vs. poor prognosis. Among the biochemical parameters different between good and poor prognosis groups, serum myoglobin and serum creatinine were found to be independent indicators for death and/or readmission among AMI patients. Myoglobin is a widely used biomarker for early diagnosis of MI that rises earlier than troponins. However, the diagnostic value of myoglobin is limited because of its less specificity to cardiomyocyte death. Indeed, a combined analysis of myoglobin, CK-MB, and cTnI is the most often used biochemical examination for MI diagnosis among suspected patients (30). In the present study, our data showed that serum myoglobin, CK-MB, and cTnI were all markedly elevated in AMI patients. However, only myoglobin was proved to be an independent predictor for poor AMI prognosis. It was previously reported that elevated myoglobin was a predictive biomarker better than cTnI for 5-year mortality in patients evaluated in the emergency department for possible acute coronary syndromes (ACS) (31). In comparison to the long-term prognosis of patients with undifferentiated chest pain, our study analyzed the short-term prognosis of AMI patients and observed that myoglobin ≥651 ng/mL was predictive for 6-month poor prognosis after AMI. Our findings were consistent with previous studies that reported the prognostic value of myoglobin to predict mortality in patients with ACS (32) and MI (33). Indeed, despite the absence of cardiac specificity, the prognostic value of myoglobin and its cutoff point for clinical use of predicting mortality and/or readmission after AMI deserve further investigation.
Additionally, we observed that serum creatinine level was significantly higher in AMI patients with poor prognosis [83.0–140.0 (101.0) μM] than those with good prognosis [74.0–95.0 (85.5) μM]. Furthermore, serum creatinine ≥96 μM was able to independently predict 6-month death and/or readmission among AMI patients. Serum creatinine is a commonly used biomarker for kidney function. In addition to its ability to reflect kidney dysfunction, serum creatinine has been demonstrated to be an independent predictor for in-hospital and out-of-hospital mortality among patients with ACS or AMI (34, 35). In addition, subclinical serum creatinine elevation was reported to have prognostic value of adverse in-hospital outcomes among MI patients, which was independent of baseline renal function (36). The predictive value of high creatinine level for poor AMI prognosis is usually closely related to the kidney damage or dysfunction developed in those patients with impaired cardiac function (37). In our study, the median value of serum creatinine was slightly above the normal range in the group of AMI patients with poor prognosis compared to those with good prognosis. A cutoff point ≥96 μM was found to independently predict 6-month death and/or readmission among AMI patients. These data suggest that the serum creatinine elevation (even moderate elevation) also needs to be taken seriously in clinical evaluation, which may provide important information for poor prognosis among AMI patients.
Killip classification is usually evaluated for AMI patients, which is classified as Killip 1 (without heart failure), Killip 2 (with mild heart failure), Killip 3 (with pulmonary edema), and Killip 4 (with cardiogenic shock). Increasing evidence has indicated that higher Killip classification is associated with poor prognosis in patients with ACS (38, 39). Killip class ≥2 at presentation was previously found to be an independent predictor of in-hospital and long-term mortality of AMI patients (40, 41). Additionally, AMI patients were reported to have higher glucose level at presentation (42). In the present study, Killip classification 2–4 was identified as a strong independent predictor of 6-month death and/or readmission among AMI patients (AUCKillip class 2−4 = 0.748, 95% CI = 0.682–0.807, sensitivity = 69.8%, specificity = 79.9%). Moreover, our data showed that Killip classification 2–4 combined with either myoglobin (AUCKillip class 2−4+myoglobin = 0.784, 95% CI = 0.720–0.839, sensitivity = 69.8%, specificity = 79.9%) or creatinine (AUCKillip class 2−4+creatinine = 0.805, 95% CI = 0.743–0.858, sensitivity = 75.5%, specificity = 77.1%) further enhanced the predictive capacity for AMI poor prognosis. Our data, together with previous reports, highly suggest that it is important to better evaluate AMI patients ranked in the higher Killip classes. Multibiomarker approach could provide more information for the risk stratification of AMI. Moreover, AMI patients with Killip classification 2–4 need to be monitored and managed differently to improve the prognosis after AMI (43).
There are some limitations that need to be noted. First, the cohort of patients is not large enough. A relatively small number of female AMI patients were enrolled in the present study. Second, despite that the medication information was not available for each patient after 6-month follow-up, whether the medication was changed or not is important information that may also influence AMI prognosis (44). Third, the prognostic value of the four identified predictors and its cutoff point for clinical use of poor AMI prognosis deserve further investigation.
In conclusion, our study identifies serum myoglobin ≥651 ng/mL, serum creatinine ≥96 μM, Killip classification 2–4, and female gender as independent predictors of 6-month mortality and/or readmission after AMI. Noteworthy, Killip classification 2–4 combined with either myoglobin or creatinine further enhances the predictive capacity of poor AMI prognosis. Multibiomarker approach using Killip classification 2–4 and myoglobin or creatinine may be an effective way for 6-month prognosis prediction in AMI patients.
Data Availability Statement
All datasets presented in this study are included in the article/supplementary material.
Ethics Statement
The studies involving human participants were reviewed and approved by Ethics Committee of Tongji Hospital affiliated to Tongji University (Shanghai, China). The patients/participants provided their written informed consent to participate in this study.
Author Contributions
JY collected clinical and biochemical data, took charge of follow-up, and analyzed the data. YX obtained informed consent from patients and participated in the follow-up of patients. YL and YT collected patient serum and ensured the quality of serum collection. JX designed and supervised the study and wrote the paper. All authors contributed to the article and approved the submitted version.
Funding
This work was supported by the Fundamental Research Funds for the Central Universities (22120180384 to JY) and the grant from National Natural Science Foundation of China (81670362 to JX).
Conflict of Interest
The authors declare that the research was conducted in the absence of any commercial or financial relationships that could be construed as a potential conflict of interest.
References
1. Levine GN, Bates ER, Bittl JA, Brindis RG, Fihn SD, Fleisher LA, et al. 2016 ACC/AHA Guideline Focused Update on Duration of Dual Antiplatelet Therapy in Patients With Coronary Artery Disease: A Report of the American College of Cardiology/American Heart Association Task Force on Clinical Practice Guidelines: An Update of the 2011 ACCF/AHA/SCAI Guideline for Percutaneous Coronary Intervention, 2011 ACCF/AHA Guideline for Coronary Artery Bypass Graft Surgery, 2012 ACC/AHA/ACP/AATS/PCNA/SCAI/STS Guideline for the Diagnosis and Management of Patients With Stable Ischemic Heart Disease, 2013 ACCF/AHA Guideline for the Management of ST-Elevation Myocardial Infarction, 2014 AHA/ACC Guideline for the Management of Patients With Non-ST-Elevation Acute Coronary Syndromes, and 2014 ACC/AHA Guideline on Perioperative Cardiovascular Evaluation and Management of Patients Undergoing Noncardiac Surgery. Circulation. (2016) 134:e123–55. doi: 10.1161/CIR.0000000000000404
2. Benjamin EJ, Blaha MJ, Chiuve SE, Cushman M, Das SR, Deo R, et al. Heart disease and stroke statistics-2017 update: a report from the American Heart Association. Circulation. (2017) 135:e146–e603. doi: 10.1161/CIR.0000000000000485
3. Roe MT, Messenger JC, Weintraub WS, Cannon CP, Fonarow GC, Dai D, et al. Treatments, trends, and outcomes of acute myocardial infarction and percutaneous coronary intervention. J Am Coll Cardiol. (2010) 56:254–63. doi: 10.1016/j.jacc.2010.05.008
4. Krumholz HM, Normand SL, Wang Y. Trends in hospitalizations and outcomes for acute cardiovascular disease and stroke, 1999-2011. Circulation. (2014) 130:966–75. doi: 10.1161/CIRCULATIONAHA.113.007787
5. Katus HA, Remppis A, Looser S, Hallermeier K, Scheffold T, Kubler W. Enzyme linked immuno assay of cardiac troponin T for the detection of acute myocardial infarction in patients. J Mol Cell Cardiol. (1989) 21:1349–53. doi: 10.1016/0022-2828(89)90680-9
6. Alpert JS, Thygesen K, Antman E, Bassand JP. Myocardial infarction redefined–a consensus document of The Joint European Society of Cardiology/American College of Cardiology Committee for the redefinition of myocardial infarction. J Am Coll Cardiol. (2000) 36:959–69. doi: 10.1016/S0735-1097(00)00804-4
7. Newby LK, Storrow AB, Gibler WB, Garvey JL, Tucker JF, Kaplan AL, et al. Bedside multimarker testing for risk stratification in chest pain units: The chest pain evaluation by creatine kinase-MB, myoglobin, and troponin I. (CHECKMATE) study. Circulation. (2001) 103:1832–7. doi: 10.1161/01.CIR.103.14.1832
8. McCord J, Nowak RM, Hudson MP, McCullough PA, Tomlanovich MC, Jacobsen G, et al. The prognostic significance of serial myoglobin, troponin I, and creatine kinase-MB measurements in patients evaluated in the emergency department for acute coronary syndrome. Ann Emerg Med. (2003) 42:343–50. doi: 10.1016/S0196-0644(03)00411-6
9. Yousuf O, Mohanty BD, Martin SS, Joshi PH, Blaha MJ, Nasir K, et al. High-sensitivity C-reactive protein and cardiovascular disease: a resolute belief or an elusive link? J Am Coll Cardiol. (2013) 62:397–408. doi: 10.1016/j.jacc.2013.05.016
10. Jones JD, Chew PG, Dobson R, Wootton A, Ashrafi R, Khand A. The prognostic value of heart type fatty acid binding protein in patients with suspected acute coronary syndrome: a systematic review. Curr Cardiol Rev. (2017) 13:189–98. doi: 10.2174/1573403X13666170116121451
11. Chen Y, Tao Y, Zhang L, Xu W, Zhou X. Diagnostic and prognostic value of biomarkers in acute myocardial infarction. Postgrad Med J. (2019) 95:210–6. doi: 10.1136/postgradmedj-2019-136409
12. Batacan RB Jr, Duncan MJ, Dalbo VJ, Buitrago GL, Fenning AS. Effect of different intensities of physical activity on cardiometabolic markers and vascular and cardiac function in adult rats fed with a high-fat high-carbohydrate diet. J Sport Health Sci. (2018) 7:109–19. doi: 10.1016/j.jshs.2016.08.001
13. Castro-Dominguez Y, Dharmarajan K, McNamara RL. Predicting death after acute myocardial infarction. Trends Cardiovasc Med. (2018) 28:102–9. doi: 10.1016/j.tcm.2017.07.011
14. Wang L, Lv Y, Li G, Xiao J. MicroRNAs in heart and circulation during physical exercise. J Sport Health Sci. (2018) 7:433–41. doi: 10.1016/j.jshs.2018.09.008
15. Drzymalski K, Schulman-Marcus J. Editorial commentary: death after acute myocardial infarction, possible to predict? Trends Cardiovasc Med. (2018) 28:110–1. doi: 10.1016/j.tcm.2017.08.005
16. Wang P, Yao J, Xie Y, Luo M. Gender-specific predictive markers of poor prognosis for patients with acute myocardial infarction during a 6-month follow-up. J Cardiovasc Transl Res. (2020) 13:27–38. doi: 10.1007/s12265-019-09946-6
17. Nauta ST, Deckers JW, Akkerhuis KM, van Domburg RT. Short- and long-term mortality after myocardial infarction in patients with and without diabetes: changes from 1985 to 2008. Diabetes Care. (2012) 35:2043–7. doi: 10.2337/dc11-2462
18. Rapsomaniki E, Thuresson M, Yang E, Blin P, Hunt P, Chung SC, et al. Using big data from health records from four countries to evaluate chronic disease outcomes: a study in 114 364 survivors of myocardial infarction. Eur Heart J Qual Care Clin Outcomes. (2016) 2:172–83. doi: 10.1093/ehjqcco/qcw004
19. Avezum A, Makdisse M, Spencer F, Gore JM, Fox KA, Montalescot G, et al. Impact of age on management and outcome of acute coronary syndrome: observations from the Global Registry of Acute Coronary Events (GRACE). Am Heart J. (2005) 149:67–73. doi: 10.1016/j.ahj.2004.06.003
20. Bagnall AJ, Goodman SG, Fox KA, Yan RT, Gore JM, Cheema AN, et al. Influence of age on use of cardiac catheterization and associated outcomes in patients with non-ST-elevation acute coronary syndromes. Am J Cardiol. (2009) 103:1530–6. doi: 10.1016/j.amjcard.2009.01.369
21. Alabas OA, Allan V, McLenachan JM, Feltbower R, Gale CP. Age-dependent improvements in survival after hospitalisation with acute myocardial infarction: an analysis of the Myocardial Ischemia National Audit Project (MINAP). Age Ageing. (2014) 43:779–85. doi: 10.1093/ageing/aft201
22. Dey S, Flather MD, Devlin G, Brieger D, Gurfinkel EP, Steg PG, et al. Sex-related differences in the presentation, treatment and outcomes among patients with acute coronary syndromes: the Global Registry of Acute Coronary Events. Heart. (2009) 95:20–6. doi: 10.1136/hrt.2007.138537
23. Yahagi K, Davis HR, Arbustini E, Virmani R. Sex differences in coronary artery disease: pathological observations. Atherosclerosis. (2015) 239:260–7. doi: 10.1016/j.atherosclerosis.2015.01.017
24. Vaccarino V, Parsons L, Every NR, Barron HV, Krumholz HM. Sex-based differences in early mortality after myocardial infarction. National Registry of Myocardial Infarction 2 Participants. N Engl J Med. (1999) 341:217–25. doi: 10.1056/NEJM199907223410401
25. Vaccarino V, Krumholz HM, Yarzebski J, Gore JM, Goldberg RJ. Sex differences in 2-year mortality after hospital discharge for myocardial infarction. Ann Intern Med. (2001) 134:173–81. doi: 10.7326/0003-4819-134-3-200102060-00007
26. Dreyer RP, Ranasinghe I, Wang Y, Dharmarajan K, Murugiah K, Nuti SV, et al. Sex differences in the rate, timing, and principal diagnoses of 30-day readmissions in younger patients with acute myocardial infarction. Circulation. (2015) 132:158–66. doi: 10.1161/CIRCULATIONAHA.114.014776
27. Gottlieb S, Goldbourt U, Boyko V, Harpaz D, Mandelzweig L, Khoury Z, et al. Mortality trends in men and women with acute myocardial infarction in coronary care units in Israel. A comparison between 1981-1983 and 1992-1994. For the SPRINT and the Israeli Thrombolytic Survey Groups. Eur Heart J. (2000) 21:284–95. doi: 10.1053/euhj.1999.1868
28. Singh JA, Lu X, Ibrahim S, Cram P. Trends in and disparities for acute myocardial infarction: an analysis of Medicare claims data from 1992 to 2010. BMC Med. (2014) 12:190. doi: 10.1186/s12916-014-0190-6
29. Alzuhairi KS, Sogaard P, Ravkilde J, Gislason G, Kober L, Torp-Pedersen C. Incidence and outcome of first myocardial infarction according to gender and age in Denmark over a 35-year period (1978-2012). Eur Heart J Qual Care Clin Outcomes. (2015) 1:72–8. doi: 10.1093/ehjqcco/qcv016
30. Eggers KM, Oldgren J, Nordenskjold A, Lindahl B. Diagnostic value of serial measurement of cardiac markers in patients with chest pain: limited value of adding myoglobin to troponin I for exclusion of myocardial infarction. Am Heart J. (2004) 148:574–81. doi: 10.1016/j.ahj.2004.04.030
31. Jaffery Z, Nowak R, Khoury N, Tokarski G, Lanfear DE, Jacobsen G, et al. Myoglobin and troponin I elevation predict 5-year mortality in patients with undifferentiated chest pain in the emergency department. Am Heart J. (2008) 156:939–45. doi: 10.1016/j.ahj.2008.06.020
32. de Lemos JA, Morrow DA, Gibson CM, Murphy SA, Sabatine MS, Rifai N, et al. The prognostic value of serum myoglobin in patients with non-ST-segment elevation acute coronary syndromes. Results from the TIMI 11B and TACTICS-TIMI 18 studies. J Am Coll Cardiol. (2002) 40:238–44. doi: 10.1016/S0735-1097(02)01948-4
33. Kontos MC, Garg R, Anderson FP, Roberts CS, Ornato JP, Tatum JL, et al. Ability of myoglobin to predict mortality in patients admitted for exclusion of myocardial infarction. Am J Emerg Med. (2007) 25:873–9. doi: 10.1016/j.ajem.2007.01.002
34. Granger CB, Goldberg RJ, Dabbous O, Pieper KS, Eagle KA, Cannon CP, et al. Predictors of hospital mortality in the global registry of acute coronary events. Arch Intern Med. (2003) 163:2345–53. doi: 10.1001/archinte.163.19.2345
35. McNamara RL, Wang Y, Partovian C, Montague J, Mody P, Eddy E, et al. Development of a hospital outcome measure intended for use with electronic health records: 30-day risk-standardized mortality after acute myocardial infarction. Med Care. (2015) 53:818–26. doi: 10.1097/MLR.0000000000000402
36. Margolis G, Gal-Oz A, Khoury S, Keren G, Shacham Y. Relation of subclinical serum creatinine elevation to adverse in-hospital outcomes among myocardial infarction patients. Eur Heart J Acute Cardiovasc Care. (2018) 7:732–8. doi: 10.1177/2048872617716389
37. Chin CT, Chen AY, Wang TY, Alexander KP, Mathews R, Rumsfeld JS, et al. Risk adjustment for in-hospital mortality of contemporary patients with acute myocardial infarction: the acute coronary treatment and intervention outcomes network (ACTION) registry-get with the guidelines (GWTG) acute myocardial infarction mortality model and risk score. Am Heart J. (2011) 161:113–22 e112. doi: 10.1016/j.ahj.2010.10.004
38. de Gevigney G, Ecochard R, Rabilloud M, Gaillard S, Cheneau E, Ducreux C, et al. Worsening of heart failure during hospital course of an unselected cohort of 2507 patients with myocardial infarction is a factor of poor prognosis: the PRIMA study. Prise en charge de l'Infarctus du Myocarde Aigu. Eur J Heart Fail. (2001) 3:233–41. doi: 10.1016/S1388-9842(00)00154-9
39. de Carvalho LP, Gao F, Chen Q, Sim LL, Koh TH, Foo D, et al. Long-term prognosis and risk heterogeneity of heart failure complicating acute myocardial infarction. Am J Cardiol. (2015) 115:872–8. doi: 10.1016/j.amjcard.2015.01.010
40. Yano K, Grove JS, Reed DM, Chun HM. Determinants of the prognosis after a first myocardial infarction in a migrant Japanese population. The Honolulu Heart Program. Circulation. (1993) 88:2582–95. doi: 10.1161/01.CIR.88.6.2582
41. Valero-Masa MJ, Velasquez-Rodriguez J, Diez-Delhoyo F, Devesa C, Juarez M, Sousa-Casasnovas I, et al. Sex differences in acute myocardial infarction: Is it only the age? Int J Cardiol. (2017) 231:36–41. doi: 10.1016/j.ijcard.2016.11.010
42. Cheng HH, Yen PC. Killip classification and glucose level in patients with acute myocardial infarction. Am J Emerg Med. (2010) 28:853–6. doi: 10.1016/j.ajem.2009.04.029
43. Miller WL, Wright RS, Grill JP, Kopecky SL. Improved survival after acute myocardial infarction in patients with advanced Killip class. Clin Cardiol. (2000) 23:751–8. doi: 10.1002/clc.4960231012
Keywords: acute myocardial infarction, prognosis, death, readmission, biomarker
Citation: Yao J, Xie Y, Liu Y, Tang Y and Xu J (2020) Prediction Factors of 6-Month Poor Prognosis in Acute Myocardial Infarction Patients. Front. Cardiovasc. Med. 7:130. doi: 10.3389/fcvm.2020.00130
Received: 10 May 2020; Accepted: 25 June 2020;
Published: 13 August 2020.
Edited by:
Junjie Xiao, Shanghai University, ChinaReviewed by:
Guoping Li, Massachusetts General Hospital and Harvard Medical School, United StatesChen Liu, First Affiliated Hospital of Sun Yat-sen University, China
Copyright © 2020 Yao, Xie, Liu, Tang and Xu. This is an open-access article distributed under the terms of the Creative Commons Attribution License (CC BY). The use, distribution or reproduction in other forums is permitted, provided the original author(s) and the copyright owner(s) are credited and that the original publication in this journal is cited, in accordance with accepted academic practice. No use, distribution or reproduction is permitted which does not comply with these terms.
*Correspondence: Jiahong Xu, xujiahong@tongji.edu.cn