- Division of Cardiology, David Geffen School of Medicine, Department of Medicine, University of California, Los Angeles, Los Angeles, CA, United States
Cardiovascular diseases are the leading cause of death around the world. Despite the larger number of genes and loci identified, the precise mechanisms by which these genes influence risk of cardiovascular disease is not well understood. Recent advances in the development and optimization of high-throughput technologies for the generation of “omics data” have provided a deeper understanding of the processes and dynamic interactions involved in human diseases. However, the integrative analysis of “omics” data is not straightforward and represents several logistic and computational challenges. In spite of these difficulties, several studies have successfully applied integrative genomics approaches for the investigation of novel mechanisms and plasma biomarkers involved in cardiovascular diseases. In this review, we summarized recent studies aimed to understand the molecular framework of these diseases using multi-omics data from mice and humans. We discuss examples of omics studies for cardiovascular diseases focused on the integration of genomics, epigenomics, transcriptomics, and proteomics. This review also describes current gaps in the study of complex diseases using systems genetics approaches as well as potential limitations and future directions of this emerging field.
Introduction
Coronary artery disease (CAD) is the most common cause of cardiovascular death (1). Studies conducted in twins (2, 3) and in the general population have estimated a heritability of CAD at ~40–50% (4). In addition, genome-wide association studies (GWAS) have identified more than 150 genetic loci associated with CAD risk (5–18). Although GWAS studies have been successful on identifying common DNA variation implicated in cardiovascular diseases, they provide little or no molecular evidence of gene causality. In this context, the premise that rare genetic variation could have stronger functional effects on disease manifestation still is arguable (19). This realization has motivated researchers to integrate genetics studies with additional high-throughput data designed to interrogate the transcriptome, epigenome, proteome, metabolome, etc. Recent studies have implemented the integration of multi-omics data to accelerate the identification of novel mechanisms for complex diseases and understand the dynamics of disease manifestation (20–23). The relevance of integrating multi-omics data and the current statistical tools available for data integration have been reviewed in detail elsewhere (24–34). In this review, we summarize the state-of-the-art of multi-omics studies conducted in mice and humans to understand the molecular mechanisms underlying cardiovascular diseases including CAD (35–47), stroke (42, 48), heart failure (13, 49, 50), cardiac hypertrophy (13, 51), aortic valve disease (52, 53), and heart regeneration (54). We also discuss the gaps of multi-omics studies including the utility of generating multi-omics data in animal models, the importance of sex stratification on gene discovery, the inclusion of diverse populations and the integration of metabolomics and metagenomics with other omics platforms. Finally, we discuss future directions of multi-omics approaches for cardiovascular diseases and their importance in the era of precision health.
Multi-Omics Studies for the Investigation of Cardiovascular Disease
The simultaneous integration of multi-omics approaches including but not limited to genomics, epigenomics, transcriptomics, proteomics, and metabolomics (Figure 1), represents a powerful approach for understanding the mechanisms connecting identified genetic variation to cardiovascular diseases with gene causality, where many sources of variability are integrated into statistical models to identify key drivers and pathways that have the largest contribution to the disease (25). Importantly, most of the risk variants associated with CAD or other cardiovascular diseases (5, 7, 14, 17, 18, 37, 55, 56) identified by GWAS are located in noncoding regions of the genome (intronic or intergenic), suggesting that these variants are likely to affect cis or trans regulatory elements that bind transcription factors, enhancers or promoters (57). Previous multi-omic studies for CAD were mainly focused on the integration of GWAS data with global transcriptomics using eQTL analysis. In recent years, high-throughput technology have further facilitated the integration of omics data for the identification of causal genes and molecular mechanisms involved in the development of cardiovascular events in mice (13, 37, 39, 41, 58) and humans (36–39, 48) (Table 1).
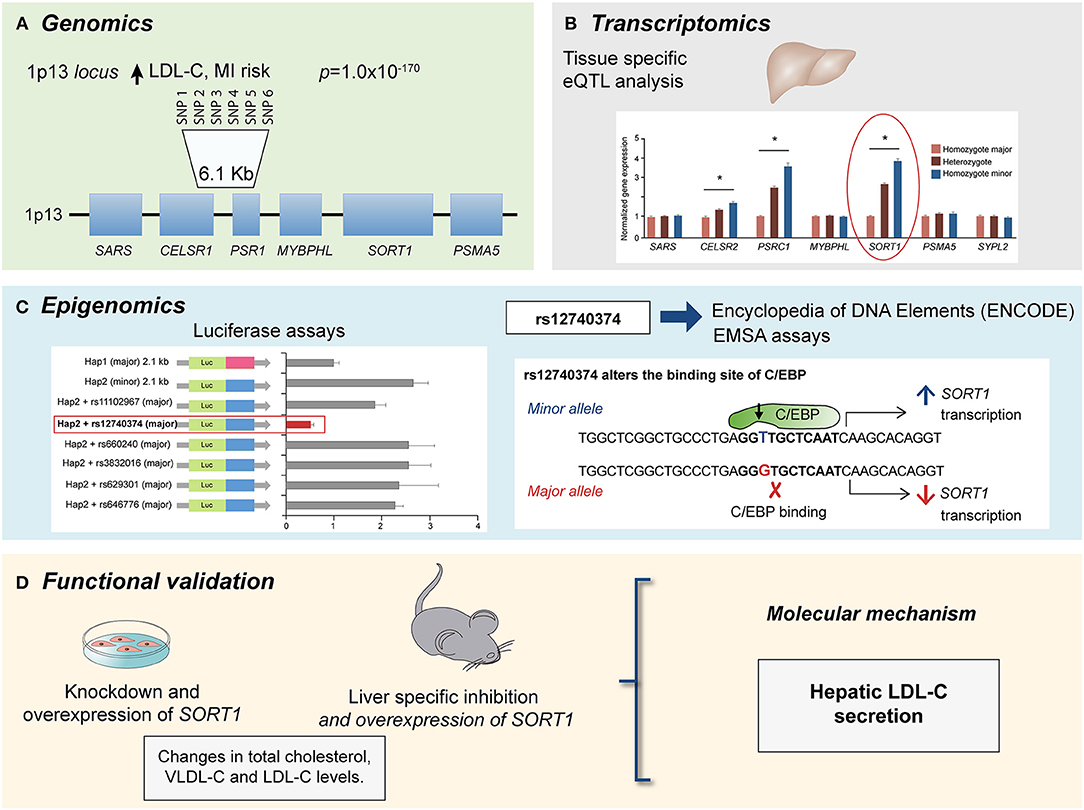
Figure 1. Multi-omics approach to identify the causal gene associated with LDL-C levels and CAD risk at the 1p13 locus. (A) GWAs meta-analysis showed several SNPs at the 1p13 locus strongly associated with LDL-C levels (p = 1.0 × 10−170) and CAD risk. The 1p13 locus contains several genes (squares). The most significantly associated haplotype for LDL-C comprise six SNPs in high linkage disequilibrium (LD) and is located between CELSR1 and PSR1 genes. (B) Liver eQTL analysis showed the minor haplotype significantly associated with higher expression of CELSR1, PSR1, and SORT1 genes with SORT1 gene showed the largest difference modified from Musunuru et al. (74). (C) By using luciferase assays and ENCODE database it was identified a common polymorphism at the 1p13 locus, rs12740374 that alters the expression of the SORT1 gene in liver with the minor allele (T) creating a C/EBP (CCAAT/enhancer binding protein) transcription factor binding site and the major allele (G) disrupting it. The C/EBP transcriptional factor regulates the expression of hepatic genes involved in metabolism. (D) Functional approaches for SORT1 using small interfering RNA (siRNA) knockdown and viral overexpression in mouse liver showed that SORT1 results in significant changes in plasma LDL-C and very low-density lipoprotein (VLDL) particle levels by modulating hepatic VLDL secretion.
Success Stories of Multi-Omics Studies in Cardiovascular Diseases
Although there have been few studies integrating multi-omics profiles for the investigation of mechanisms associated with cardiovascular diseases, this approach has revealed the potential function of previously identified GWAS loci and respective mechanisms involved in these common diseases. In this section, we summarize recent studies using multi-omics approaches focusing on the integration of genomics, epigenomics, transcriptomics, and proteomics.
Genomics, Transcriptomics, and Epigenomics
There is a large body of literature linking genetic variation with gene expression and/or epigenetic marks to understand the potential mechanisms of identified DNA variants in disease manifestation. One example on the integration of genomics with transcriptomics is a study conducted to investigate the role of the 9p21 locus (63), which was identified as one of the most significant loci for CAD in previous GWAs (64, 65). The association of CAD with this locus have been consistently replicated in multiple studies (56, 66), although the causal link of this locus remained unclear. This locus contains several genes including CDKN2A (encoding cyclin p14, p16), CDKN2B (encoding cyclin p15), MTAP (encoding methylthioadenosine phosphorylase), and the long non-coding RNA ANRIL. Integration of genetic and transcriptomic data led to the identification of ANRIL as the top candidate causal gene for CAD at the 9p21 region (63). Functional studies in cell lines showed possible mechanisms that could explain the role of 9p21 in CAD (67, 68). For instance, a previous study showed that alleles at the 9p21 locus were associated with different isoforms of ANRIL (linear or circular isoforms), where linear transcripts were associated with atherosclerosis and circular transcripts were protective against atherosclerosis. This process is mediated through the expression of multiple genes regulated in both, cis and trans (69, 70). Moreover, a recent study showed that ANRIL (DQ485454) is involved in endothelial cells functions important to the development of CAD including monocyte adhesion to endothelial cells, trans-endothelial monocyte migration, and endothelial cell migration (71).
Another example is the investigation of the region of the gene cluster CELSR2-PSRC1-MYBPHL-SORT at the 1p13.3 locus associated with low-density lipoprotein cholesterol (LDL-C) levels and cardiovascular risk (55, 72, 73). Incorporation of eQTL analysis also showed that SNPs associated with a lower risk of CAD in the 1p13.3 locus were associated with an increased gene expression of SORT1, PSRC1, and CELSR2, with SORT1 displaying the largest expression change in the liver (73, 74). This finding allowed the construction of new hypothesis to elucidate the molecular mechanism of the 1p13.3 locus on CAD development. Studies of SORT1 and PSRC1 overexpression in mouse models of hyperlipidemia showed that, while PSCR1 overexpression had no metabolic effects, SORT1 overexpression led to a significant reduction in plasma LDL-C and very low-density lipoprotein (VLDL) particle levels by modulating hepatic VLDL secretion, suggesting an important role of SORT1 in CAD (74). Finally, a similar omics approach was applied to identify genes associated with isoproterenol-induced hypertrophy and heart failure in the Hybrid Mouse Diversity Panel (HMDP) (13, 22, 23, 41, 75–83). The integration of genomic information and cardiac transcriptome enabled the identification of several candidate causal genes that determined the degree of cardiac hypertrophy. Specifically, Hes1 was predicted to be involved in the progression of heart damage in cardiac hypertrophy (13). This study showed that knocking down Hes1 in ventricular myocytes resulted in a reduction of up to 90% hypertrophy, confirming the role of Hes1 in cardiac hypertrophy (13). More recently, several studies have demonstrated that epigenetic modifications are associated with CAD risk (38, 42, 43, 47, 49, 59, 61, 62, 84, 85), and other CVD related risk factors (61, 62, 84). Epigenetic changes that have been investigated in the context of CVD include DNA methylation (38, 43, 49), chromatin organization (42), and microRNAs (47). In recent years, efforts have been conducted to identify interactions between functional non-coding active elements of the genome and enhancers, defined as cis-acting DNA sequences that can increase the transcription of genes (60, 61, 86). Several methods have been developed for the identification of these interactions including, chromatin immunoprecipitation followed by high-throughput sequencing (ChIP-seq), chromatin conformation capture (3C, HiC), and most recently, chromatin interaction paired-end tagging (ChIA-PET). These technologies offer the advantage to identify genome-wide protein-DNA interactions.
Adding Another Layer: Proteomics
The incorporation of protein expression profiles into the multi-omics studies for CAD has been less explored compared with multi-omics studies incorporating mRNA expression (43–45, 47, 51–54). This may be due to the costs and the highly specialized expertise required for instrument operation, data acquisition, and analysis of quantitative proteomics (87). Recently, Emilsson et al. showed that co-expression protein modules associated with complex diseases are highly regulated by cis and trans acting genetic variants (88). Therefore, the integration of proteomic data can add valuable information about the molecular processes involved in the development of CAD. One of the more interesting studies incorporating proteomic data in mice was conducted by Lau et al. which in addition to genomic and proteomic data, integrated protein dynamics (51). This study showed modules involved in cell adhesion, glycolytic process, actin filament organization, translation, and sodium ion transport associated with heart hypertrophy (51). In another multi-omics study conducted by Schlotter et al. for the identification of mechanisms involved in calcified aortic valve disease (CAVD) (52), the authors performed global transcriptomics and proteomics of human stenotic valves to identified novel regulatory networks in CAVD. Novel potential molecular drivers of CAVD development and progression were identified including alkaline phosphatase, apolipoprotein B, matrix metalloproteinase activation, and mitogen-activated protein kinase. Moreover, this approach also identified inflammation pathways as a significant contributor to CVD (52). This study emphasizes the relevance of extensive phenotypic characterization for multi-omics approaches to define markers associated with disease subgroups and to design more specific therapeutic strategies. In summary, these studies showed that the knowledge generated from the integration of genomics, epigenomics, transcriptomics, and proteomics could provide initial insights into the identification of mechanisms for cardiovascular diseases.
Metabolomics and Metagenomic Studies for the Study of CAD
Metabolomics and metagenomics represent additional layers of complexity because they integrate the influences of the intake, utilization and flux of nutrients. Moreover, these omics data have proven to be useful tools for the identification of biomarkers with potential clinical applicability (89). However, studies integrating metabolomics, lipidomics, or metagenomics data in the context of CAD are limited (Table 1). In a GWAS study for metabolite levels conducted by Suhre et al. (35), the authors found several loci including ABO, NAT2, CPS1, NAT8, ALPL, KLKB1 genes associated with both metabolites and a high risk of CAD (35). Interestingly, KLKB1 was associated with bradykinin concentrations and with a higher CAD risk. It is known that bradykinin is a potent endothelium-dependent vasodilator that contributes to vasodilation and hypotension (90). These findings suggest that the integration of metabolomic data with other omic data can help to identify novel biomarkers for CVD diagnosis. Regarding studies integrating metagenomic data, there are only two studies for CVD so far that integrate metabolomics and metagenomics data (40, 50) (Table 1). These studies have shown species of bacteria associated with risk of CAD and plasma metabolites. For example, the bacteria Veillonella was associated with chronic heart failure and was also inversely correlated with known cardiovascular protective metabolites such as niacin, cinnamic acid and orotic acid (50). Nevertheless, it should be noted that these studies are only based on correlations and do not make an integrative analysis of the data, which reflects the complexity and the opportunity to develop novel statistical approaches.
Integration of Multi-Omics, Multi-Ethnic, and Multi-Species Models of Disease
It has been suggested that comparison of “omics” data between human and animal models can provide an important contribution to the understanding of the molecular mechanism implicated in CAD (24). While studies in humans have greater translational potential, studies using animal models can help validate their biological relevance and to recapitulate the findings in humans under different environmental stimulus (22, 24, 78). This has been demonstrated in recent studies integrating multi-omics approaches for the study of CAD in both humans and animal models (39, 41). An example of a large-scale integrative multi-omic approach is the study conducted by Shu and colleagues that involved CAD and T2D GWAS data of five multi-ethnic studies (41). In this study, genetic and transcriptomic data of 16 relevant tissues for CAD were included to construct co-regulation networks for CVD and T2D (41). This network modeling allowed the identification of pathways involved in lipid metabolism, glucose, and branched-chain amino acids, along with process involved in oxidation, extracellular matrix, immune response, and neuronal system in CAD and T2D (41). Moreover, this strategy helped to dissect the molecular mechanism of HMGCR, identified as a top key driver for both CAD and T2D. Interestingly, the authors showed that HMGCR was associated with CVD and T2D in opposite directions, while genetic variants in HMGCR decrease CVD risk, they increase T2D risk. These findings could have important implications in the pharmacological treatment of both diseases. The integration of existing omics-data from mice and humans deposited in the cardiovascular disease database (C/VDdb), including, microRNA, genomics, proteomics and metabolomics, has recently been analyzed to identified novel drivers for CVD. In an exercise to demonstrate the utility of the C/VD database, integrative analysis of this “omics” studies showed enrichment of lipid metabolism, extracellular matrix remodeling, inflammation, and cardiac hypertrophy pathways. In addition, regulatory mechanisms mediated through miRNAs associated with the development of CAD were reported (47). Altogether, these studies illustrate that high-level integration approaches are powerful tools to extract robust biological signals across molecular layers, phenotypes, tissue types, and even species and to prioritize new therapeutic avenues for cardiometabolic diseases. Of note, there is a limited overlap in the metabolic regulators, co-expression modules and key driver gene identified across different multi-omics studies for CVD, except for markers involved in lipid metabolism which seem to be consistent among different studies. This highlights the importance of lipid metabolism in the development of cardiovascular disorders (91–93). Discrepancies of these findings could be explained by differences in the statistical tools, phenotypic characterization, ethnic origin, sex, and pathophysiological conditions (13, 23–25, 79, 94).
Data Integration Using Freely Available Public Databases
The access to big biologic public databases allows the integration of genomic data with other “omics” including transcriptomics, proteomics and metabolomics datasets through freely available public databases such as GTEx (95) Encode (Encode project c, Roadmap (Roadmap Epigenomics Consortium, 2015), Snyderome (96) and bioRxiv, to mention a few. One of the main advantages of these databases is that allow simultaneous analysis of regulatory mechanism in different tissues, which are usually difficult to obtain in genetic studies conducted in humans. In this regard, the Genotype-Tissue Expression (GTEx) project is one of the most complete gene expression datasets currently available. This database was generated as a repository for identifying genetic variants associated with changes in gene expression (expression quantitative trait loci, eQTLs) and contains a broad tissue collection obtained from deceased donors. The last release v7, provides 11,688 transcriptomes from 714 individuals and 53 tissues. In addition GTEx also includes pathology and histology data as well as other characteristics as ethnicity, age, and sex (95). Moreover, in order to increase information about potential molecular mechanisms, the Enhancing GTEx (eGTEx) project extends the GTEx project to combine gene expression with DNase I hypersensitivity, ChIP–seq, DNA and RNA methylation, ASE, protein expression, somatic mutation, and telomere length assays (97). The Encyclopedia of DNA Elements (ENCODE) project has identified and annotated a significant amount of functional elements in the human and mice genome through diverse approaches as DNA hypersensitivity, DNA methylation, and immunoprecipitation (IP) assays of proteins that interact with DNA and RNA. The last version includes over 35 high-throughput experimental methods in > 250 different cell and tissue types, resulting in over 4,000 experiments. As GTEx database, ENCODE also includes relevant information about ethnicity, sex and age (98). Additional databases such as Roadmap (99), which has an extensive collection of DNA methylation, histone modifications, chromatin accessibility, and small RNA transcripts. The utility of these databases has been demonstrated in several studies for CAD, where their integration with genetic data facilitated the identification of regulatory mechanisms, potential targets and allows the functional validation. One example, is the prediction of the disruption of C/EBP binding site by the G allele of rs12740374 SNP using ENCODE data, functional studies showed that this variant results in a lower transcription of the SORT1 gene in liver and a higher VLDL-secretion, explaining the association of the variant with LDL-C levels in genetic studies (Figure 1) (74). Therefore, the integration of various data frameworks could be highly successfully to understand the mechanisms implicated in disease manifestation.
Future Directions
The identification of causal genes is a critical step toward the translation of genetic loci into biologic processes. The integration of “omic” strategies will accelerate the identification, in a more precise way, of novel molecular mechanisms implicated in CVD. This may eventually result in the characterization of novel pathways and drug targets. Although multi-omics approaches have been successfully applied for the investigation of cardiovascular diseases, the number of studies using this approach is still limited. These studies have been primarily focused on the integration of genomics, transcriptomics, epigenomics, and proteomics. Given the potential of metabolomics, metatranscriptomics, and metagenomics as tools for the identification of biomarkers with potential clinical applicability, the integration of such data will increase the understanding of cardiovascular diseases and accelerate the identification of new diagnostics or therapeutic targets (100). Finally, research efforts should be directed to the application of multi-omics and the generation of big data in more diverse populations and into the investigation of sex-specific mechanisms.
Author Contributions
PL-M, JW, and AH-V drafted and edited the manuscript.
Funding
AH-V is funded by the NIH U54 DK120342 grant and NIH/CTSI UL1 TR00188. JW is funded by the NIH K08 HL133491 and NIH R01 HL129639.
Conflict of Interest Statement
The authors declare that the research was conducted in the absence of any commercial or financial relationships that could be construed as a potential conflict of interest.
References
1. Writing Group M, Mozaffarian D, Benjamin EJ, Go AS, Arnett DK, Blaha MJ, et al. Heart disease and stroke statistics-2016 update: a report from the american heart association. Circulation. (2016) 133:e38–360. doi: 10.1161/CIR.0000000000000350
2. Zdravkovic S, Wienke A, Pedersen NL, Marenberg ME, Yashin AI, De Faire U. Heritability of death from coronary heart disease: a 36-year follow-up of 20 966 Swedish twins. J Intern Med. (2002) 252:247–54. doi: 10.1046/j.1365-2796.2002.01029.x
3. Marenberg ME, Risch N, Berkman LF, Floderus B, de Faire U. Genetic susceptibility to death from coronary heart disease in a study of twins. N Engl J Med. (1994) 330:1041–6. doi: 10.1056/NEJM199404143301503
4. Won HH, Natarajan P, Dobbyn A, Jordan DM, Roussos P, Lage K, et al. Disproportionate contributions of select genomic compartments and cell types to genetic risk for coronary artery disease. PLoS Genet. (2015) 11:e1005622. doi: 10.1371/journal.pgen.1005622
5. Consortium CAD, Deloukas P, Kanoni S, Willenborg C, Farrall M, Assimes TL, et al. Large-scale association analysis identifies new risk loci for coronary artery disease. Nat Genet. (2013) 45:25–33. doi: 10.1038/ng.2480
6. Coram MA, Duan Q, Hoffmann TJ, Thornton T, Knowles JW, Johnson NA, et al. Genome-wide characterization of shared and distinct genetic components that influence blood lipid levels in ethnically diverse human populations. Am J Hum Genet. (2013) 92:904–16. doi: 10.1016/j.ajhg.2013.04.025
7. Coronary Artery Disease Genetics C. A genome-wide association study in Europeans and South Asians identifies five new loci for coronary artery disease. Nat Genet. (2011) 43:339–44. doi: 10.1038/ng.782
8. Klarin D, Damrauer SM, Cho K, Sun YV, Teslovich TM, Honerlaw J, et al. Genetics of blood lipids among ~300,000 multi-ethnic participants of the Million Veteran Program. Nat Genet. (2018) 50:1514–23. doi: 10.1038/s41588-018-0222-9
9. Myocardial Infarction G, Investigators CAEC, Stitziel NO, Stirrups KE, Masca NG, Erdmann J, et al. Coding variation in ANGPTL4, LPL, and SVEP1 and the risk of coronary disease. N Engl J Med. (2016) 374:1134–44. doi: 10.1056/NEJMoa1507652
10. Myocardial Infarction Genetics C, Kathiresan S, Voight BF, Purcell S, Musunuru K, Ardissino D, et al. Genome-wide association of early-onset myocardial infarction with single nucleotide polymorphisms and copy number variants. Nat Genet. (2009) 41:334–41. doi: 10.1038/ng.327
11. Nelson CP, Goel A, Butterworth AS, Kanoni S, Webb TR, Marouli E, et al. Association analyses based on false discovery rate implicate new loci for coronary artery disease. Nat Genet. (2017) 49:1385–91. doi: 10.1038/ng.3913
12. Nikpay M, Goel A, Won HH, Hall LM, Willenborg C, Kanoni S, et al. A comprehensive 1,000 Genomes-based genome-wide association meta-analysis of coronary artery disease. Nat Genet. (2015) 47:1121–30. doi: 10.1038/ng.3396
13. Santolini M, Romay MC, Yukhtman CL, Rau CD, Ren S, Saucerman JJ, et al. A personalized, multiomics approach identifies genes involved in cardiac hypertrophy and heart failure. NPJ Syst Biol Appl. (2018) 4:12. doi: 10.1038/s41540-018-0046-3
14. Schunkert H, Konig IR, Kathiresan S, Reilly MP, Assimes TL, Holm H, et al. Large-scale association analysis identifies 13 new susceptibility loci for coronary artery disease. Nat Genet. (2011) 43:333–8. doi: 10.1038/ng.784
15. Tabb KL, Hellwege JN, Palmer ND, Dimitrov L, Sajuthi S, Taylor KD, et al. Analysis of whole exome sequencing with cardiometabolic traits using family-based linkage and association in the IRAS family study. Ann Hum Genet. (2017) 81:49–58. doi: 10.1111/ahg.12184
16. Teslovich TM, Musunuru K, Smith AV, Edmondson AC, Stylianou IM, Koseki M, et al. Biological, clinical and population relevance of 95 loci for blood lipids. Nature. (2010) 466:707–13. doi: 10.1038/nature09270
17. van der Harst, Verweij NP. Identification of 64 novel genetic loci provides an expanded view on the genetic architecture of coronary artery disease. Circ Res. (2018) 122:433–43. doi: 10.1161/CIRCRESAHA.117.312086
18. Verweij N, Eppinga RN, Hagemeijer Y, van der Harst P. Identification of 15 novel risk loci for coronary artery disease and genetic risk of recurrent events, atrial fibrillation, and heart failure. Sci Rep. (2017) 7:2761. doi: 10.1038/s41598-017-03062-8
19. Wray NR, Purcell SM, Visscher PM. Synthetic associations created by rare variants do not explain most GWAS results. PLoS Biol. (2011) 9:e1000579. doi: 10.1371/journal.pbio.1000579
20. Ramazzotti D, Lal A, Wang B, Batzoglou S, Sidow A. Multi-omic tumor data reveal diversity of molecular mechanisms that correlate with survival. Nat Commun. (2018) 9:4453. doi: 10.1038/s41467-018-06921-8
21. Xiao H, Bartoszek K, Lio P. Multi-omic analysis of signalling factors in inflammatory comorbidities. BMC Bioinformat. (2018) 19(Suppl. 15):439. doi: 10.1186/s12859-018-2413-x
22. Chella Krishnan K, Kurt Z, Barrere-Cain R, Sabir S, Das A, Floyd R, et al. Integration of multi-omics data from mouse diversity panel highlights mitochondrial dysfunction in non-alcoholic fatty liver disease. Cell Syst. (2018) 6:103–15 e7. doi: 10.1016/j.cels.2017.12.006
23. Kurt Z, Barrere-Cain R, LaGuardia J, Mehrabian M, Pan C, Hui ST, et al. Tissue-specific pathways and networks underlying sexual dimorphism in non-alcoholic fatty liver disease. Biol Sex Differ. (2018) 9:46. doi: 10.1186/s13293-018-0205-7
24. Hasin Y, Seldin M, Lusis A. Multi-omics approaches to disease. Genome Biol. (2017) 18:83. doi: 10.1186/s13059-017-1215-1
25. Arneson D, Shu L, Tsai B, Barrere-Cain R, Sun C, Yang X. Multidimensional integrative genomics approaches to dissecting cardiovascular disease. Front Cardiovasc Med. (2017) 4:8. doi: 10.3389/fcvm.2017.00008
26. Vilne B, Schunkert H. Integrating genes affecting coronary artery disease in functional networks by multi-OMICs approach. Front Cardiovasc Med. (2018) 5:89. doi: 10.3389/fcvm.2018.00089
27. Arnett DK. Genetics of CVD in 2015: using genomic approaches to identify CVD-causing variants. Nat Rev Cardiol. (2016) 13:72–4. doi: 10.1038/nrcardio.2015.202
28. Raghow R. An 'omics' perspective on cardiomyopathies and heart failure. Trends Mol Med. (2016) 22:813–27. doi: 10.1016/j.molmed.2016.07.007
29. Misra BB, Langefeld CD, Olivier M, Cox LA. Integrated omics: tools, advances, and future approaches. J Mol Endocrinol. (2018) 62:R21–45. doi: 10.1530/JME-18-0055
30. Civelek M, Lusis AJ. Systems genetics approaches to understand complex traits. Nat Rev Genet. (2014) 15:34–48. doi: 10.1038/nrg3575
31. MacLellan WR, Wang Y, Lusis AJ. Systems-based approaches to cardiovascular disease. Nat Rev Cardiol. (2012) 9:172–84. doi: 10.1038/nrcardio.2011.208
32. Wu S, Lusis AJ, Drake TA. A systems-based framework for understanding complex metabolic and cardiovascular disorders. J Lipid Res. (2009) 50 Suppl:S358–63. doi: 10.1194/jlr.R800067-JLR200
33. Lusis AJ, Weiss JN. Cardiovascular networks: systems-based approaches to cardiovascular disease. Circulation. (2010) 121:157–70. doi: 10.1161/CIRCULATIONAHA.108.847699
34. Lusis AJ. A thematic review series: systems biology approaches to metabolic and cardiovascular disorders. J Lipid Res. (2006) 47:1887–90. doi: 10.1194/jlr.E600004-JLR200
35. Suhre K, Shin SY, Petersen AK, Mohney RP, Meredith D, Wagele B, et al. Human metabolic individuality in biomedical and pharmaceutical research. Nature. (2011) 477:54–60. doi: 10.1038/nature10354
36. Foroughi Asl H, Talukdar HA, Kindt AS, Jain RK, Ermel R, Ruusalepp A, et al. Expression quantitative trait Loci acting across multiple tissues are enriched in inherited risk for coronary artery disease. Circ Cardiovasc Genet. (2015) 8:305–15. doi: 10.1161/CIRCGENETICS.114.000640
37. Braenne I, Civelek M, Vilne B, Di Narzo A, Johnson AD, Zhao Y, et al. Prediction of causal candidate genes in coronary artery disease loci. Arterioscler Thromb Vasc Biol. (2015) 35:2207–17. doi: 10.1161/ATVBAHA.115.306108
38. Franzen O, Ermel R, Cohain A, Akers NK, Di Narzo A, Talukdar HA, et al. Cardiometabolic risk loci share downstream cis- and trans-gene regulation across tissues and diseases. Science. (2016) 353:827–30. doi: 10.1126/science.aad6970
39. Talukdar HA, Foroughi Asl H, Jain RK, Ermel R, Ruusalepp A, Franzen O, et al. Cross-tissue regulatory gene networks in coronary artery disease. Cell Syst. (2016) 2:196–208. doi: 10.1016/j.cels.2016.02.002
40. Feng Q, Liu Z, Zhong S, Li R, Xia H, Jie Z, et al. Integrated metabolomics and metagenomics analysis of plasma and urine identified microbial metabolites associated with coronary heart disease. Sci Rep. (2016) 6:22525. doi: 10.1038/srep22525
41. Shu L, Chan KHK, Zhang G, Huan T, Kurt Z, Zhao Y, et al. Shared genetic regulatory networks for cardiovascular disease and type 2 diabetes in multiple populations of diverse ethnicities in the United States. PLoS Genet. (2017) 13:e1007040. doi: 10.1371/journal.pgen.1007040
42. Haitjema S, Meddens CA, van der Laan SW, Kofink D, Harakalova M, Tragante V, et al. Additional candidate genes for human atherosclerotic disease identified through annotation based on chromatin organization. Circ Cardiovasc Genet. (2017) 10:e001664. doi: 10.1161/CIRCGENETICS.116.001664
43. Howson JMM, Zhao W, Barnes DR, Ho WK, Young R, Paul DS, et al. Fifteen new risk loci for coronary artery disease highlight arterial-wall-specific mechanisms. Nat Genet. (2017) 49:1113–9. doi: 10.1038/ng.3874
44. Yao C, Chen G, Song C, Keefe J, Mendelson M, Huan T, et al. Genome-wide mapping of plasma protein QTLs identifies putatively causal genes and pathways for cardiovascular disease. Nat Commun. (2018) 9:3268. doi: 10.1038/s41467-018-06231-z
45. Chen G, Yao C, Hwang SJ, Liu C, Song C, Huan T, et al. Integrated proteomic analysis of cardiovascular disease reveals novel protein quantitative trait loci. Circulation. (2016) 134:A18806.
46. Lempiainen H, Braenne I, Michoel T, Tragante V, Vilne B, Webb TR, et al. Network analysis of coronary artery disease risk genes elucidates disease mechanisms and druggable targets. Sci Rep. (2018) 8:3434. doi: 10.1038/s41598-018-20721-6
47. Fernandes M, Patel A, Husi H. C/VDdb: a multi-omics expression profiling database for a knowledge-driven approach in cardiovascular disease. (CVD). PLoS ONE. (2018) 13:e0207371. doi: 10.1371/journal.pone.0207371
48. Zhao YQ, Kurt Z, Yang X. Multi-omics modeling of carotid atherosclerotic plaques reveals molecular networks and regulators of stroke. Circulation. (2017) 136:A20541.
49. Meder B, Haas J, Sedaghat-Hamedani F, Kayvanpour E, Frese K, Lai A, et al. Epigenome-wide association study identifies cardiac gene patterning and a novel class of biomarkers for heart failure. Circulation. (2017) 136:1528–44. doi: 10.1161/CIRCULATIONAHA.117.027355
50. Cui X, Ye L, Li J, Jin L, Wang W, Li S, et al. Metagenomic and metabolomic analyses unveil dysbiosis of gut microbiota in chronic heart failure patients. Sci Rep. (2018) 8:635. doi: 10.1038/s41598-017-18756-2
51. Lau E, Cao Q, Lam MPY, Wang J, Ng DCM, Bleakley BJ, et al. Integrated omics dissection of proteome dynamics during cardiac remodeling. Nat Commun. (2018) 9:120. doi: 10.1038/s41467-017-02467-3
52. Schlotter F, Halu A, Goto S, Blaser MC, Body SC, Lee LH, et al. Spatiotemporal multi-omics mapping generates a molecular atlas of the aortic valve and reveals networks driving disease. Circulation. (2018) 138:377–93. doi: 10.1161/CIRCULATIONAHA.117.032291
53. Matic LP, Jesus Iglesias M, Vesterlund M, Lengquist M, Hong MG, Saieed S, et al. Novel multiomics profiling of human carotid atherosclerotic plaques and plasma reveals biliverdin reductase B as a marker of intraplaque hemorrhage. JACC Basic Transl Sci. (2018) 3:464–80. doi: 10.1016/j.jacbts.2018.04.001
54. Lalowski MM, Bjork S, Finckenberg P, Soliymani R, Tarkia M, Calza G, et al. Characterizing the key metabolic pathways of the neonatal mouse heart using a quantitative combinatorial omics approach. Front Physiol. (2018) 9:365. doi: 10.3389/fphys.2018.00365
55. Samani NJ, Braund PS, Erdmann J, Gotz A, Tomaszewski M, Linsel-Nitschke P, et al. The novel genetic variant predisposing to coronary artery disease in the region of the PSRC1 and CELSR2 genes on chromosome 1 associates with serum cholesterol. J Mol Med. (2008) 86:1233–41. doi: 10.1007/s00109-008-0387-2
56. Samani NJ, Erdmann J, Hall AS, Hengstenberg C, Mangino M, Mayer B, et al. Genomewide association analysis of coronary artery disease. N Engl J Med. (2007) 357:443–53. doi: 10.1056/NEJMoa072366
57. Emilsson V, Thorleifsson G, Zhang B, Leonardson AS, Zink F, Zhu J, et al. Genetics of gene expression and its effect on disease. Nature. (2008) 452:423–8. doi: 10.1038/nature06758
58. Lau E, Wu JC. Omics, big data, and precision medicine in cardiovascular sciences. Circ Res. (2018) 122:1165–8. doi: 10.1161/CIRCRESAHA.118.313161
59. Liu B, Pjanic M, Wang T, Nguyen T, Gloudemans M, Rao A, et al. Genetic regulatory mechanisms of smooth muscle cells map to coronary artery disease risk loci. Am J Hum Genet. (2018) 103:377–88. doi: 10.1016/j.ajhg.2018.08.001
60. Lee D, Kapoor A, Safi A, Song L, Halushka MK, Crawford GE, et al. Human cardiac cis-regulatory elements, their cognate transcription factors, and regulatory DNA sequence variants. Genome Res. (2018) 28:1577–88. doi: 10.1101/gr.234633.118
61. Rask-Andersen M, Martinsson D, Ahsan M, Enroth S, Ek WE, Gyllensten U, et al. Epigenome-wide association study reveals differential DNA methylation in individuals with a history of myocardial infarction. Hum Mol Genet. (2016) 25:4739–48. doi: 10.1093/hmg/ddw302
62. Dekkers KF, van Iterson M, Slieker RC, Moed MH, Bonder MJ, van Galen M, et al. Blood lipids influence DNA methylation in circulating cells. Genome Biol. (2016) 17:138. doi: 10.1186/s13059-016-1000-6
63. Jarinova O, Stewart AF, Roberts R, Wells G, Lau P, Naing T, et al. Functional analysis of the chromosome 9p21.3 coronary artery disease risk locus. Arterioscler Thromb Vasc Biol. (2009) 29:1671–7. doi: 10.1161/ATVBAHA.109.189522
64. McPherson R, Pertsemlidis A, Kavaslar N, Stewart A, Roberts R, Cox DR, et al. A common allele on chromosome 9 associated with coronary heart disease. Science. (2007) 316:1488–91. doi: 10.1126/science.1142447
65. Wang F, Xu CQ, He Q, Cai JP, Li XC, Wang D, et al. Genome-wide association identifies a susceptibility locus for coronary artery disease in the Chinese Han population. Nat Genet. (2011) 43:345–9. doi: 10.1038/ng.783
66. Helgadottir A, Thorleifsson G, Manolescu A, Gretarsdottir S, Blondal T, Jonasdottir A, et al. A common variant on chromosome 9p21 affects the risk of myocardial infarction. Science. (2007) 316:1491–3. doi: 10.1126/science.1142842
67. Holdt LM, Teupser D. Long non-coding RNA ANRIL: Lnc-ing genetic variation at the chromosome 9p21 locus to molecular mechanisms of atherosclerosis. Front Cardiovasc Med. (2018) 5:145. doi: 10.3389/fcvm.2018.00145
68. Musunuru K, Post WS, Herzog W, Shen H, O'Connell JR, McArdle PF, et al. Association of single nucleotide polymorphisms on chromosome 9p21.3 with platelet reactivity: a potential mechanism for increased vascular disease. Circ Cardiovasc Genet. (2010) 3:445–53. doi: 10.1161/CIRCGENETICS.109.923508
69. Burd CE, Jeck WR, Liu Y, Sanoff HK, Wang Z, Sharpless NE. Expression of linear and novel circular forms of an INK4/ARF-associated non-coding RNA correlates with atherosclerosis risk. PLoS Genet. (2010) 6:e1001233. doi: 10.1371/journal.pgen.1001233
70. Holdt LM, Stahringer A, Sass K, Pichler G, Kulak NA, Wilfert W, et al. Circular non-coding RNA ANRIL modulates ribosomal RNA maturation and atherosclerosis in humans. Nat Commun. (2016) 7:12429. doi: 10.1038/ncomms12429
71. Cho H, Shen GQ, Wang X, Wang F, Archacki S, Li Y, et al. Long non-coding RNA ANRIL regulates endothelial cell activities associated with coronary artery disease by up-regulating CLIP1, EZR, and LYVE1 genes. J Biol Chem. (2019) 294:3881–98. doi: 10.1074/jbc.RA118.005050
72. Willer CJ, Sanna S, Jackson AU, Scuteri A, Bonnycastle LL, Clarke R, et al. Newly identified loci that influence lipid concentrations and risk of coronary artery disease. Nat Genet. (2008) 40:161–9. doi: 10.1038/ng.76
73. Kathiresan S, Melander O, Guiducci C, Surti A, Burtt NP, Rieder MJ, et al. Six new loci associated with blood low-density lipoprotein cholesterol, high-density lipoprotein cholesterol or triglycerides in humans. Nat Genet. (2008) 40:189–97. doi: 10.1038/ng.75
74. Musunuru K, Strong A, Frank-Kamenetsky M, Lee NE, Ahfeldt T, Sachs KV, et al. From noncoding variant to phenotype via SORT1 at the 1p13 cholesterol locus. Nature. (2010) 466:714–9. doi: 10.1038/nature09266
75. Lusis AJ, Seldin MM, Allayee H, Bennett BJ, Civelek M, Davis RC, et al. The hybrid mouse diversity panel: a resource for systems genetics analyses of metabolic and cardiovascular traits. J Lipid Res. (2016) 57:925–42. doi: 10.1194/jlr.R066944
76. Lin LY, Chun Chang S, O'Hearn J, Hui ST, Seldin M, Gupta P, et al. Systems genetics approach to biomarker discovery: GPNMB and heart failure in mice and humans. G3. (2018) 8:3499–506. doi: 10.1534/g3.118.200655
77. Bennett BJ, Davis RC, Civelek M, Orozco L, Wu J, Qi H, et al. Genetic architecture of atherosclerosis in mice: a systems genetics analysis of common inbred strains. PLoS Genet. (2015) 11:e1005711. doi: 10.1371/journal.pgen.1005711
78. Hui ST, Kurt Z, Tuominen I, Norheim F, C Davis R, Pan C, et al. The genetic architecture of diet-induced hepatic fibrosis in mice. Hepatology. (2018) 68:2182–96. doi: 10.1002/hep.30113
79. Norheim F, Hasin-Brumshtein Y, Vergnes L, Chella Krishnan K, Pan C, Seldin MM, et al. Gene-by-sex interactions in mitochondrial functions and cardio-metabolic traits. Cell Metab. (2019) 29:932–49.e4. doi: 10.1016/j.cmet.2018.12.013
80. Park S, Ranjbarvaziri S, Lay FD, Zhao P, Miller MJ, Dhaliwal JS, et al. Genetic regulation of fibroblast activation and proliferation in cardiac fibrosis. Circulation. (2018) 138:1224–35. doi: 10.1161/CIRCULATIONAHA.118.035420
81. Buscher K, Ehinger E, Gupta P, Pramod AB, Wolf D, Tweet G, et al. Natural variation of macrophage activation as disease-relevant phenotype predictive of inflammation and cancer survival. Nat Commun. (2017) 8:16041. doi: 10.1038/ncomms16041
82. Rau CD, Civelek M, Pan C, Lusis AJ. A suite of tools for biologists that improve accessibility and visualization of large systems genetics datasets: applications to the hybrid mouse diversity panel. Methods Mol Biol. (2017) 1488:153–88. doi: 10.1007/978-1-4939-6427-7_7
83. Rau CD, Romay MC, Tuteryan M, Wang JJ, Santolini M, Ren S, et al. Systems genetics approach identifies gene pathways and Adamts2 as drivers of isoproterenol-induced cardiac hypertrophy and cardiomyopathy in mice. Cell Syst. (2017) 4:121–8 e4. doi: 10.1016/j.cels.2016.10.016
84. Breitling LP, Yang RX, Korn B, Burwinkel B, Brenner H. Tobacco-smoking-related differential DNA methylation: 27K discovery and replication. Am J Hum Genet. (2011) 88:450–7. doi: 10.1016/j.ajhg.2011.03.003
85. Fernandez-Sanles A, Sayols-Baixeras S, Curcio S, Subirana I, Marrugat J, Elosua R. DNA methylation and age-independent cardiovascular risk, an epigenome-wide approach: the REGICOR study (REgistre GIroni del COR). Arterioscler Thromb Vasc Biol. (2018) 38:645–52. doi: 10.1161/ATVBAHA.117.310340
86. Wang X, He L, Goggin SM, Saadat A, Wang L, Sinnott-Armstrong N, et al. High-resolution genome-wide functional dissection of transcriptional regulatory regions and nucleotides in human. Nat Commun. (2018) 9:5380. doi: 10.1038/s41467-018-07746-1
87. Schubert OT, Rost HL, Collins BC, Rosenberger G, Aebersold R. Quantitative proteomics: challenges and opportunities in basic and applied research. Nat Protoc. (2017) 12:1289–94. doi: 10.1038/nprot.2017.040
88. Emilsson V, Ilkov M, Lamb JR, Finkel N, Gudmundsson EF, Pitts R, et al. Co-regulatory networks of human serum proteins link genetics to disease. Science. (2018) 361:769–73. doi: 10.1126/science.aaq1327
89. Wang N, Zhu F, Chen L, Chen K. Proteomics, metabolomics, and metagenomics for type 2 diabetes and its complications. Life Sci. (2018) 212:194–202. doi: 10.1016/j.lfs.2018.09.035
90. Cruden NL, Witherow FN, Webb DJ, Fox KA, Newby DE. Bradykinin contributes to the systemic hemodynamic effects of chronic angiotensin-converting enzyme inhibition in patients with heart failure. Arterioscler Thromb Vasc Biol. (2004) 24:1043–8. doi: 10.1161/01.ATV.0000129331.21092.1d
91. Ridker PM. LDL cholesterol: controversies and future therapeutic directions. Lancet. (2014) 384:607–17. doi: 10.1016/S0140-6736(14)61009-6
92. Rader DJ, Hovingh GK. HDL and cardiovascular disease. Lancet. (2014) 384:618–25. doi: 10.1016/S0140-6736(14)61217-4
93. Nordestgaard BG, Varbo A. Triglycerides and cardiovascular disease. Lancet. (2014) 384:626–35. doi: 10.1016/S0140-6736(14)61177-6
94. Johnson KW, Shameer K, Glicksberg BS, Readhead B, Sengupta PP, Bjorkegren JLM, et al. Enabling precision cardiology through multiscale biology and systems medicine. JACC Basic Transl Sci. (2017) 2:311–27. doi: 10.1016/j.jacbts.2016.11.010
95. Consortium GT. The genotype-tissue expression (GTEx) project. Nat Genet. (2013) 45:580–5. doi: 10.1038/ng.2653
96. Chen R, Mias GI, Li-Pook-Than J, Jiang L, Lam HY, Chen R, et al. Personal omics profiling reveals dynamic molecular and medical phenotypes. Cell. (2012) 148:1293–307. doi: 10.1016/j.cell.2012.02.009
97. eGTEx Project. Enhancing GTEx by bridging the gaps between genotype, gene expression, and disease. Nat Genet. (2017) 49:1664–70. doi: 10.1038/ng.3969
98. Consortium EP. An integrated encyclopedia of DNA elements in the human genome. Nature. (2012) 489:57–74. doi: 10.1038/nature11247
99. Roadmap Epigenomics C, Kundaje A, Meuleman W, Ernst J, Bilenky M, Yen A, et al. Integrative analysis of 111 reference human epigenomes. Nature. (2015) 518:317–30. doi: 10.1038/nature14248
Keywords: multi-omics, cardiovascular disease, heart disease, systems biology, data integration
Citation: Leon-Mimila P, Wang J and Huertas-Vazquez A (2019) Relevance of Multi-Omics Studies in Cardiovascular Diseases. Front. Cardiovasc. Med. 6:91. doi: 10.3389/fcvm.2019.00091
Received: 02 March 2019; Accepted: 19 June 2019;
Published: 17 July 2019.
Edited by:
Clint L. Miller, University of Virginia, United StatesReviewed by:
Christopher G. Bell, Queen Mary University of London, United KingdomHauke Busch, Universität zu Lübeck, Germany
Copyright © 2019 Leon-Mimila, Wang and Huertas-Vazquez. This is an open-access article distributed under the terms of the Creative Commons Attribution License (CC BY). The use, distribution or reproduction in other forums is permitted, provided the original author(s) and the copyright owner(s) are credited and that the original publication in this journal is cited, in accordance with accepted academic practice. No use, distribution or reproduction is permitted which does not comply with these terms.
*Correspondence: Adriana Huertas-Vazquez, ahuertasvazquez@mednet.ucla.edu