- 1Center for Public Health Genomics, University of Virginia, Charlottesville, VA, United States
- 2Department of Biochemistry and Molecular Genetics, University of Virginia, Charlottesville, VA, United States
- 3School of Nursing, University of Virginia, Charlottesville, VA, United States
- 4Data Science Institute, University of Virginia, Charlottesville, VA, United States
- 5Department of Biomedical Engineering, University of Virginia, Charlottesville, VA, United States
- 6Department of Public Health Sciences, University of Virginia, Charlottesville, VA, United States
Atherosclerosis is a complex inflammatory disease of the vessel wall involving the interplay of multiple cell types including vascular smooth muscle cells, endothelial cells, and macrophages. Large-scale genome-wide association studies (GWAS) and the advancement of next generation sequencing technologies have rapidly expanded the number of long non-coding RNA (lncRNA) transcripts predicted to play critical roles in the pathogenesis of the disease. In this review, we highlight several lncRNAs whose functional role in atherosclerosis is well-documented through traditional biochemical approaches as well as those identified through RNA-sequencing and other high-throughput assays. We describe novel genomics approaches to study both evolutionarily conserved and divergent lncRNA functions and interactions with DNA, RNA, and proteins. We also highlight assays to resolve the complex spatial and temporal regulation of lncRNAs. Finally, we summarize the latest suite of computational tools designed to improve genomic and functional annotation of these transcripts in the human genome. Deep characterization of lncRNAs is fundamental to unravel coronary atherosclerosis and other cardiovascular diseases, as these regulatory molecules represent a new class of potential therapeutic targets and/or diagnostic markers to mitigate both genetic and environmental risk factors.
Introduction
Despite intensive research into the underlying pathogenesis of atherosclerosis/coronary artery disease (CAD), this disease still remains a significant public health burden. Atherosclerosis is a complex disease involving both environmental and genetic risk factors resulting in plaque formation and inflammation in the vessel wall. A number of cellular responses have been proposed to contribute to disease progression, including endothelial cell dysfunction, vascular smooth muscle cell phenotypic switching, macrophage/foam cell activation/necrosis/defective efferocytosis, and defective lipid/lipoprotein metabolism (1, 2). Much of the research to date has focused on the role of protein coding genes in atherosclerosis leading to the identification of a number of proteins described as key drivers. However, our understanding of the causal mechanisms of this disease remains limited, likely due to our incomplete functional knowledge of the non-coding genome. It is now well-established that more than 90% of disease-associated variants reside in non-coding regions, once considered “junk sequences” (3). An increasing number of studies, especially those utilizing high-throughput sequencing technologies, have shown that a number of non-coding RNAs (ncRNAs) are differentially regulated during disease, supporting potential functional roles for these molecules (4).
The formation of the GENCODE project (part of the ENCODE project) and other large-scale initiatives such as NONCODE have revealed 75% of the genome is transcribed, yet only 2% encodes for protein, suggesting alternative functional roles for ncRNA transcripts (5, 6). Since the development of RNA-seq and other high-throughput sequencing assays, ncRNAs are now appreciated as key regulators of gene expression (7). A number of the essential types of non-coding RNAs are already well-characterized such as transfer, spliceosomal, and ribosomal RNAs. Aside from these housekeeping RNA elements, the remaining types of non-coding RNAs are subdivided into two classes, small and long non-coding RNAs, based on their size. Examples of small non-coding RNAs include microRNAs (miRNA), small nucleolar RNAs (snoRNA), and PIWI-interacting RNAs (piRNA) (8). These elements can act as positive or negative regulators of gene expression and generally exert their influence through complementary base pairing to their target transcript 3′ or 5′ untranslated regions (9).
Long non-coding RNAs (lncRNAs) represent a heterogeneous class of non-coding RNAs that includes transcripts >200 nucleotides, which lack functional protein coding ability (10). Within this lncRNA class, they are also classified based on their genomic location and broadly encompass enhancer-related RNAs (eRNAs), transcribed ultraconserved RNAs, intronic RNAs, long intergenic RNAs (lincRNAs), and natural antisense transcripts (NATs) (10). Contrary to canonical linear lncRNAs, a distinct group of lncRNAs are known as circular RNAs (circRNAs) due to their circular structure, which often results from backsplicing (11). By participating in both transcriptional and post-transcriptional stages, lncRNAs modulate gene expression through multiple distinct mechanisms. Further insight into these regulatory mechanisms will facilitate a better understanding of disease biology and identify additional viable targets for therapeutic intervention or diagnostics. Here, we present an overview of various lncRNAs relevant to atherosclerosis and highlight next-generation sequencing approaches to systematically investigate lncRNA function, as well as the ongoing challenges in this exciting field.
Mechanisms of Long Non-coding RNA Function
LncRNAs are a heterogeneous class of ncRNAs (>200 nucleotides in length) that do not contain a functional open reading frame (12). LncRNAs can be encoded on either the sense or antisense DNA strand and may be located within a protein coding gene or in the intergenic regions (12). Similar to mRNAs, lncRNAs are transcribed by RNA polymerase II. Many transcripts are polyadenylated, multi-exonic, undergo RNA splicing, and contain a 5' cap. Often, their active promoters are marked with H3K4me3 and gene bodies have H3K36me3 histone modifications (13). Unlike protein coding genes, lncRNAs are not translated into protein and thereby lack functional initiation and termination codons (8). They are expressed at a much lower levels relative to their protein coding counterparts and lack robust evolutionary conservation. Despite this low level of conservation, the expression pattern of lncRNAs has been shown to be relatively cell/tissue specific (14–16).
The mechanism of action of these regulatory factors were categorized into four broad groups proposed by Wang and Chang (17). Signaling lncRNAs represent a class which exhibit a high degree of spatial and temporal specificity that serve a role in signal transduction. Once transcribed, these signaling lncRNAs have effector functions in activating appropriate downstream pathways in response to a stimulus. Additionally, their presence may indicate a particular developmental cell state, condition, or overall transcriptional activity (17). Another mechanism in which lncRNAs exert their regulatory function is by acting as decoy molecules to limit the availability of RNA binding factors to interact with their partners. By impairing the ability of chromatin remodelers, transcription factors, and miRNA from binding to their target genes, decoy lncRNAs can inhibit downstream effector functions (17). It is noteworthy that miRNAs also have the ability to target lncRNAs directly and thereby influence transcriptional regulation and vascular functions (18–20). Aided by their ability to bind protein as well as base pair with target sequences, guide lncRNAs are responsible for localizing transcriptional regulators to specific regions. Similar to the role of guide lncRNAs, scaffold lncRNAs use their protein binding ability to provide a surface to mediate protein-protein interactions (17). In these various ways, lncRNAs represent a distinct class of regulatory elements to modulate transcriptional activities.
Overview of LncRNAs Involved in Atherosclerosis
Functional Studies of LncRNAs in Atherosclerosis
Depending on the cell types involved, lncRNAs play myriad roles in diverse atherosclerotic processes in the vessel wall including cell proliferation, migration, differentiation, apoptosis, and inflammation. They also play important roles in the regulation of cholesterol and lipid metabolism. Pertinent cell types include smooth muscle cells, endothelial cells, macrophages, and hepatocytes (Figure 1). A more comprehensive overview of key lncRNAs in atherogenic processes is given in Table 1. Perhaps the most well-studied in atherosclerosis is CDKN2B-AS1 or ANRIL (Antisense Non-coding RNA in the INK4 Locus), which acts in several cell types relevant to CAD (21, 22, 81). ANRIL acts as a guide lncRNA to localize polycomb repressive complex (PRC) at target promoters through a direct interaction with its subunits, CBX7 or SUZ12. PRC then adds H3K27me3 modifications to this region to repress transcription (22). Loss of function studies have suggested ANRIL acts in cis in order to regulate transcription of the nearby tumor suppressors, CDKN2A and CDKN2B (82, 83). Consistent with this mechanism of action, ANRIL expression correlates with a more proliferative phenotype in endothelial cells and vascular smooth muscle cells (VSMC) (22, 84, 85). In addition to acting in cis, ANRIL also acts in trans (via Alu elements) to regulate other genes that participate in proatherogenic pathways (22). Since ANRIL is not well-conserved in mice, in vivo functional studies have been challenging (86). A more complete overview of the atherogenic roles of ANRIL RNA species has been recently documented in a review by Holdt and Teupser (87).
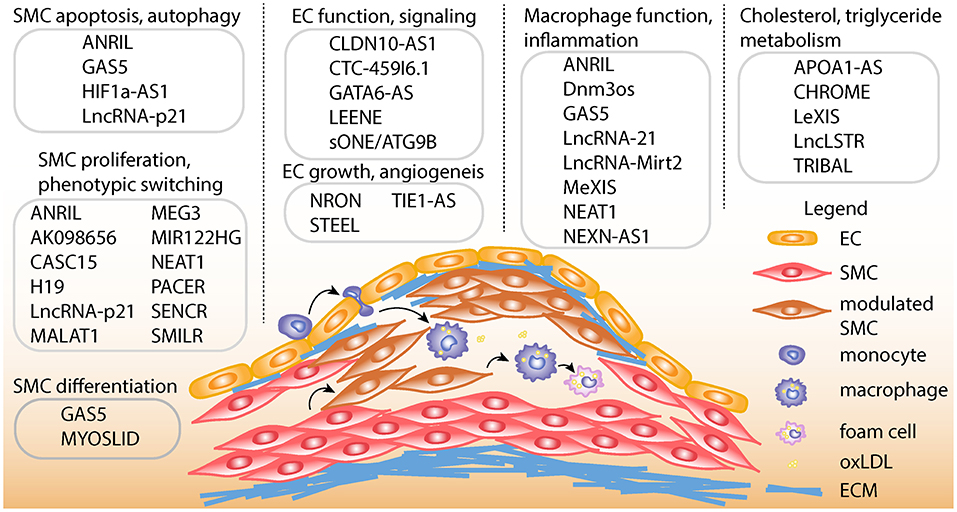
Figure 1. Schematic of atherosclerotic processes and specific lncRNA functions. Top, LncRNAs are shown with described smooth muscle cell (SMC) functions, such as proliferation, apoptosis, autophagy, phenotypic switching, and differentiation. LncRNAs are also shown with endothelial cell (EC) functions such as differentiation, regulation of endothelial nitric oxide synthase (eNOS) mediated signaling, growth and angiogenesis. LncRNAs are shown with macrophage functions, such as macrophage polarization, cholesterol efflux, and inflammation. Also, lncRNAs are listed with functions in regulating cholesterol and triglyceride metabolism in hepatocytes and/or macrophages. Bottom, schematic showing example of atherosclerotic lesion after invasion of vascular endothelium by activated monocytes, which become macrophages upon chronic inflammatory stimulation. Exposure to oxidized LDL (oxLDL) particles promote macrophage transformation to lipid-laden foam cells. Also depicted is the transformation of contractile SMCs to de-differentiated or modulated SMCs, as well as the transition of modulated SMCs to macrophage-like cells in the lesion. ECM, Extracellular matrix.
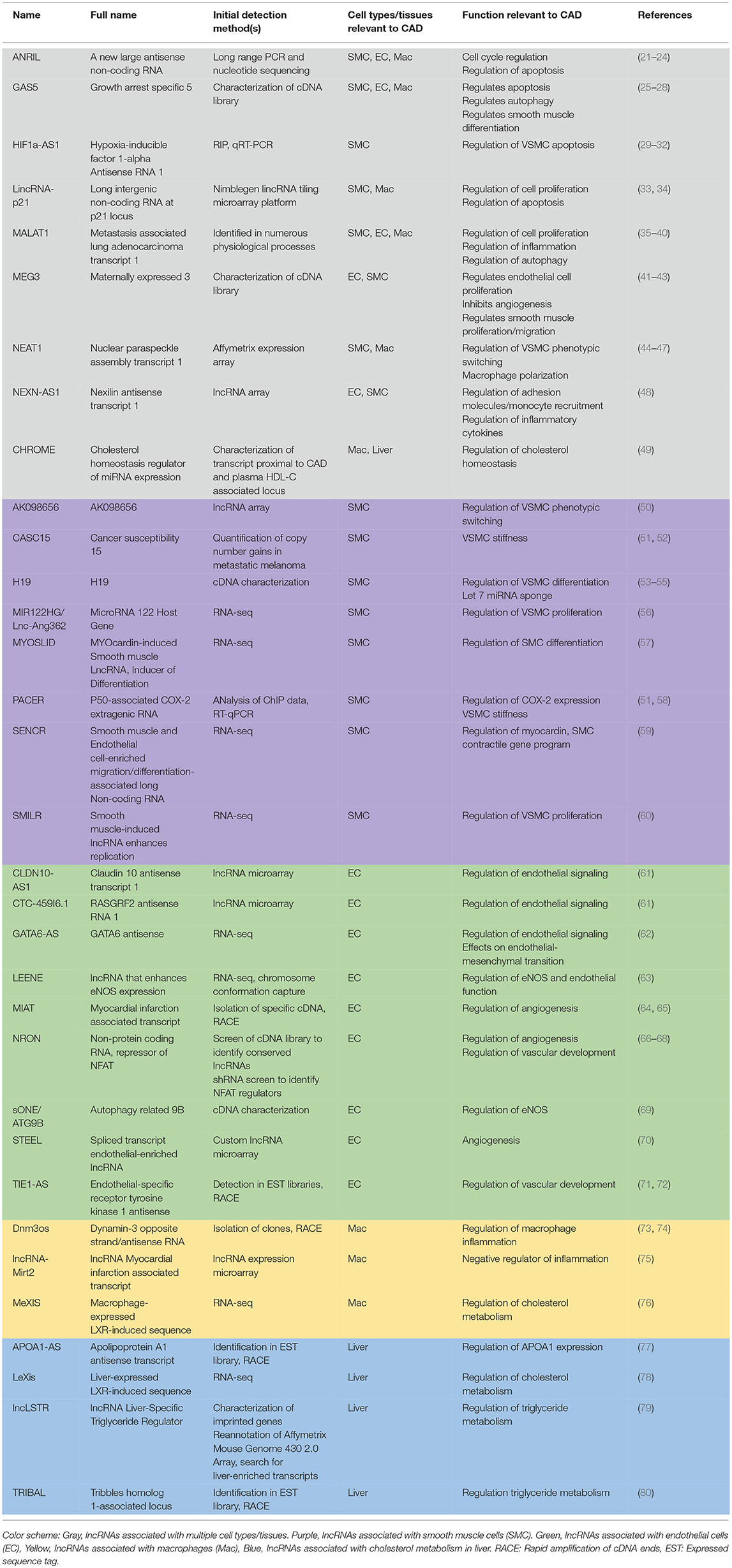
Table 1. List of long non-coding RNAs with functional relevance in coronary artery disease cell types/tissues.
The ubiquitously expressed and evolutionarily conserved lncRNA, MALAT1, is decreased in atherosclerotic plaques (88, 89), and reduced MALAT1 levels in hematopoietic cells promotes atherosclerosis and inflammation in mice in vivo (89). In contrast, MALAT1 knockdown in VSMC and EC results in cell cycle arrest and reduced proliferation (35, 36). Other lncRNAs (e.g., lincRNA-p21, HIF1α-AS, circular ANRIL, and GAS5) have been implicated in cell death/apoptotic pathways through various mechanisms (23, 29, 33, 90), as described elsewhere (91, 92). In particular, lincRNA-p21 was shown to be reduced in CAD patients and mouse models of atherosclerosis, and regulates p53-dependent smooth muscle cell proliferation and apoptosis (33). Many lncRNAs have established immune and inflammatory roles. For example, heterozygous MALAT1-deficient ApoE-/- mice have increased inflammation and atherosclerosis (93). LncRNA Dnm3os (dynamin 3 opposite strand) is upregulated in diabetic induced macrophages and regulates nucleolin and epigenetic mediated inflammatory responses (73). Finally, in human macrophages treated with oxidized LDL, HOTAIR regulates oxidative stress and inflammation (94).
There are several lncRNAs with regulatory roles in lipid and cholesterol metabolism. CHROME (cholesterol homeostasis regulator of miRNA expression) is a lncRNA upregulated in carotid plaques, which regulates cholesterol homeostasis in primates in liver and macrophages by inhibiting miRNAs, such as miR-33 (49). NEAT1 promotes pro-atherogenic functions in THP-1 human macrophage cells such as increased ox-LDL lipid accumulation and inflammation by serving as a sponge of miR-342-3p target (44). Finally, differential expression of TRIBAL, APOA1-AS, and lncLSTR is linked to defects in lipid metabolic pathways, mainly in the liver. TRIBAL (TRIB1 associated locus) regulates Trib1 mRNA stability through mitogen activated kinase, consistent with Trib1 regulation (80, 95, 96). Increased TRIBAL expression stabilizes Trib1 expression and upregulates fatty acid oxidative pathways (80). Likewise, lncLSTR (liver-specific triglyceride regulator) regulates plasma triglyceride clearance by modulating apolipoprotein C2 (APOC2) levels and lipoprotein lipase activity (79). APOA1-AS regulates cholesterol levels through epigenetic modulation of APOA1, a protein involved in the cholesterol efflux pathway (77).
LncRNAs With Genetic Associations in Atherosclerosis
Genome wide association studies (GWAS) have linked genetic variation at the ANRIL locus (9p21.3) to many complex phenotypes including CAD, stroke, type 2 diabetes and multiple cancers (97). In addition to ANRIL, the 9p21.3 locus encodes three tumor suppressor proteins: CDKN2A, CDKN2B, and MTAP. Despite each being attractive candidates underlying the locus association with various diseases, several studies report CAD risk polymorphisms associated with ANRIL expression (21, 98). However, association studies of 9p21.3 genotype with ANRIL expression remain complex due to the numerous linear and circular ANRIL forms (23).
Other less studied lncRNAs have been identified from genetic studies of CAD or related traits and may play critical roles in atherosclerosis. For instance, genetic variation in the imprinted lncRNA H19, involved in embryonic development (99) and oncogenesis (100), was associated with CAD and ischemic stroke in Chinese populations (101, 102). H19 was initially shown to be re-expressed in smooth muscle cells in human and rodent atherosclerotic plaques (103), and promotes VSMC proliferation by acting as a let-7a miRNA sponge to upregulate cyclin D (104). However, a recent study revealed endothelial cell restricted expression in human atherosclerotic plaques and a role in endothelial cell aging by suppressing STAT3 signaling (105), similar to lncRNA MEG3 (106). Another endothelial cell lncRNA, MIAT (Myocardial Infarction Associated Transcript), was previously associated with myocardial infarction in a large genetic study of a Japanese population (64). MIAT is upregulated in atherosclerosis plaques (88), and regulates microvascular dysfunction by acting as a competing endogenous RNA (65). Another lncRNA associated with CAD through large-scale GWAS is known as TARID (TCF21 antisense RNA inducing promoter demethylation) (107). TARID was identified as an eQTL target gene in human coronary artery smooth muscle cells (108), and molecular studies suggest this lncRNA guides GADD45A mediated DNA demethylation and inactivation of TCF21 (109), a known tumor suppressor and vascular wall transcription factor associated with CAD (110–113). Yet, functional studies of TARID both in VSMCs and in vivo are needed to elucidate its potential role in atherosclerosis. With larger GWAS sample sizes, and complementary eQTL colocalization (114), and transcriptome-wide association studies (TWAS) (115), it is anticipated that even more lncRNAs will be identified with genetic association evidence.
Application of Transcriptomics to Identify and Study LncRNAs Relevant to CAD
General Considerations for Transcriptomics Studies of LncRNAs
While traditional methods to profile lncRNA transcriptomes have relied on microarrays or serial analysis of gene expression (SAGE), these approaches have largely been replaced with the decreasing costs and greater output achieved by RNA-seq (116). In general, RNA-seq provides greater sensitivity and specificity to detect a broad range of ncRNA transcripts, novel isoforms, and interactions between ncRNAs (117). Nonetheless there are some important considerations when designing and conducting RNA-seq based lncRNA screening experiments. For instance, given that lncRNAs are approximately 10X less abundant than mRNAs on average, the basal expression of a typical lncRNA is < 5 fragments per kilobase of transcript per million mapped reads (FPKM) (118). Thus, it is highly recommended to obtain deeper sequencing per sample (~100X read depth) than a typical RNA-seq experiment. Also, while up to 50% of lncRNAs appear to be poly-adenylated (119) and would be detected with mRNA library preparation kits, a more comprehensive landscape of lncRNAs, other ncRNAs, including eRNAs, would require total RNA [poly(A) and non-poly(A)], ribosomal RNA depletion methods of purification. Distinguishing lncRNA transcripts from mRNA transcripts from short-read sequencing data remains a challenge, however deeper, paired-end and stranded sequencing should improve identification of lncRNAs (120). Also, careful study design is needed to ensure sufficient power to detect differentially expressed and transcript-specific lncRNAs, when using standard count-based tools (120). Since many lncRNAs are tissue and cell-specific (121–123), it is also worth considering the effects of diluting weak signals from bulk populations of cells, as well as specific environmental contexts that may regulate lncRNA transcript levels. Below, we summarize recent findings of RNA-seq based lncRNA discoveries in specific cell types relevant to CAD/atherosclerosis.
Transcriptomics of Vascular Smooth Muscle Cell Function
The first VSMC lncRNA discovered via RNA-seq was Lnc-Ang362 (HG-MIR222), which is upregulated in rat aortic smooth muscle cells upon stimulation with angiotensin II (56). Lnc-Ang362 promotes VSMC proliferation and is the host-transcript for both miR-221 and miR-222. Bell et al. conducted RNA-seq in human coronary artery smooth muscle cells and identified 31 previously unidentified lncRNAs (59). Notably, one of these was Smooth muscle and Endothelial cell-enriched migration/differentiation-associated long Non-coding RNA (SENCR), which is located antisense to the FLI1 gene. SENCR functionally promotes a contractile smooth muscle phenotype and inhibits migration (59). In a follow-up study, RNA-seq was performed in human coronary artery smooth muscle cells to examine the effect of myocardin (MYOCD) overexpression (57). MYOCD is a potent co-factor that binds with serum response factor (SRF) to activate an array of smooth muscle-specific genes that maintain smooth muscle cell differentiation (124–128). Over 100 lncRNAs were differentially expressed, one of which was identified as MYOcardin-induced Smooth muscle LncRNA, Inducer of Differentiation (MYOSLID). Functional studies demonstrated that MYOSLID, a direct transcriptional target of MYOCD/SRF, promotes smooth muscle differentiation and inhibits proliferation (57).
Yu et al. used RNA-seq to compare transcriptomes of coronary and aortic smooth muscle cells subjected to both normal and pathological aortic stiffness, a subclinical risk factor for CAD and various aortic diseases (51). Only two of the top 20 ranked differentially expressed lncRNAs have been studied to date: CASC15 and PACER (RP5-973M2.2). These lncRNAs regulate expression of protein-coding genes in cis and PACER activates COX2 expression (52, 58, 129). Analysis of RNA-seq data highlighted the lncRNA MALAT1 as a key regulator of VSMC stiffness-induced proliferation and migration. Although MALAT1 was originally described as an endothelial lncRNA, MALAT1 regulates the phenotyping switching of VSMCs via activation of the autophagy pathway (36). Using RNA-seq in human smooth muscle cells Ballantyne et al. identified over 300 differentially expressed lncRNAs upon platelet-derived growth factor and interleukin-1 alpha stimulation. The novel lncRNA, Smooth Muscle-Induced LncRNA enhances Replication (SMILR) identified from this study enhances smooth muscle cell proliferation and has increased expression in unstable atherosclerotic plaques (60). The lncRNA NEAT1 (nuclear paraspeckle assembly transcript 1) has recently been implicated in promoting the phenotypic switching of VSMCs (45). RNA-seq demonstrated NEAT1 silencing increases the mRNA levels of numerous critical smooth muscle cell marker genes. Finally, to identify lncRNAs key in smooth muscle cell differentiation, Lim et al. combined and queried diverse RNA-seq datasets from Gene Expression Omnibus (GEO). Dozens of lncRNAs with no previous evidence for roles in VSMC differentiation were identified in this analysis that warrant further investigation, either as cis transcriptional regulators or suppressing miRNA function (130).
The development of custom lncRNA arrays has been applied to identify lncRNAs involved in various processes critical in atherosclerosis. One example is a microarray analysis which identified 580 lncRNAs differentially expressed upon exposure of human aortic smooth muscle cells to cyclic mechanical stretch (131). Another example is identification of AK098656, predominantly expressed in VSMCs, also upregulated in hypertensive patients and involved in promoting a synthetic smooth muscle cell phenotype (50).
Transcriptomics of Endothelial Cell Function
Although not all lncRNAs have a poly(A) tail, Michalik et al. performed deep sequencing of poly(A)-selected RNA in human umbilical vein endothelial cells (HUVECs) and found over half of total RNA composed of non-coding RNA, many of which are lncRNAs (35). This study focused on five lncRNAs with high endothelial expression and strong conservation between mice and humans: MALAT1, linc00493, maternally expressed 3 (MEG3), taurine upregulated gene 1 (TUG1), and linc00657. MALAT1 and MEG3 are strongly upregulated in response to hypoxia while linc006757 are TUG1 are moderately upregulated. In regards to angiogenesis, MALAT1 promotes angiogenesis and induces a switch of endothelial cells from a migratory cell phenotype to a proliferative cell phenotype (132). Huang et al. postulated exosomal MALAT1 from oxidized LDL (oxLDL) treated endothelial cells (HUVECs) promotes macrophage polarization toward the M2 phenotype (133). MEG3 was shown to interact with epigenetic modifiers, to inhibit angiogenesis and contribute to age-related endothelial dysfunction (106, 134, 135).
In another study Miao et al. conducted RNA-seq profiling of endothelial cells subjected to both physiological and pathological flow for various time points (63). They identified and characterized LEENE (lncRNA that enhances eNOS expression) as a lncRNA highly correlated with endothelial nitric oxide synthase (eNOS) expression levels, which is downregulated upon pathological flow (63). Several lncRNAs characterized in smooth muscle cells also have functional significance in endothelial cells. For instance, the SMC lncRNA SENCR regulates the differentiation of pluripotent cells into endothelial cells and promotes angiogenesis in HUVECs (136).
Custom lncRNA microarrays have identified endothelial cell enriched lncRNAs (70) and endothelial lncRNAs differentially expressed in response to specific treatments (e.g., oxidized LDL) (61). These arrays have revealed new candidate lncRNAs in atherosclerosis including spliced-transcript endothelial-enriched lncRNA (STEEL) (70), NEXN-AS1 (48), CLDN10-AS1, and CTC-459I6.1 (61). STEEL facilitates the transcriptional stimulation of both eNOS and Kruppel-like factor (KLF2) and in vivo promotes angiogenesis (70). STEEL expression is decreased in HUVECs exposed to “atheroprotective” flow and expression is increased in HUVECs exposed to disturbed “atheroprone” flow.
Transcriptomics of Macrophage Function and Inflammation
In macrophages, LXR activation promotes cholesterol efflux through activation of target genes such as Abca1 during the formation of HDL. To investigate the regulation of LXR-dependent transcription in macrophages, a recent study conducted large-scale transcriptional profiling of mouse peritoneal macrophages in response to the LXR agonist GW3965. LXR activation stimulated transcription of an array of lncRNAs, of which MeXis was among the strongest induced (76). MeXis is well-conserved in mice and was shown to amplify the LXR-dependent expression of Abca1 in vivo and promote cholesterol efflux in macrophages (76). Loss of MeXis in Ldlr−/− mice was shown to accelerate atherosclerosis through impaired Abca1 expression in macrophages and resulted in decreased cholesterol efflux (76). ATAC-seq in peritoneal macrophages demonstrated decreased chromatin accessibility across the Abca1 locus in response to loss of MeXis. Querying the MeXis interactome through mass spectrometry revealed protein interactions with the nuclear receptor coactivator DDX17. Either directly or indirectly through one of its interacting targets, MeXis represents a potential therapeutic target to regulate macrophage cholesterol efflux.
RNA-seq and lncRNA arrays have identified a number of other macrophage lncRNAs that could represent novel CAD targets. Zhang et al. performed deep RNA sequencing of human monocyte-derived macrophages as well as M1 activated (via interferon gamma and lipopolysaccharide stimulation) and M2 activated (via interleukin 4 stimulation) macrophages (137). This study identified 861 previously unannotated lincRNAs, most of which are not syntenic in mouse. Furthermore, the lncRNA expression profile is dramatically shifted upon M1 activation, supporting the inflammatory nature of atherosclerosis. Similarly, 109 unannotated CD14+ monocyte lincRNAs were highlighted upon exposure to inflammatory stress in vivo (138). Other recent array studies highlighted the macrophage lncRNAs Dnm3os amd Mirt2. Dnm3os is upregulated in bone marrow derived macrophages in diabetic mice compared to controls and is higher in monocytes in human type 2 diabetic patients compared to controls (73). Dnm3os alters global histone modifications in macrophages and upregulates various immune-response and inflammatory genes. LncRNA-Mirt2 is strongly induced by LPS, a toll-like receptor 4 (TLR4) ligand where it acts as a negative feedback inflammatory regulator (75).
Transcriptomics of Cholesterol Metabolism and Hepatocyte Function
Liver X receptors (LXRs) are nuclear factor transcription factors that are important mediators of lipid and cholesterol metabolism. LXR targets include the ABC family of transporters, ApoE, LPL, and SREBP (139, 140). Liver-specific LXR alpha knockout mice develop increased cholesterol levels and atherosclerosis (141). Sallam et al. performed genome-wide transcriptional profiling of primary mouse hepatocytes upon stimulation with an LXR agonist (78). The strongest induced gene was a non-coding RNA termed LeXis (liver-expressed LXR-induced sequence) that lies adjacent to the Abca1 gene. LeXis regulates several genes with roles in cholesterol biosynthesis, subsequently altering both liver and plasma cholesterol levels. Mass spectrometry was used to characterize the LeXis interactome and revealed binding to RALY, a ribonucleoprotein that acts a transcriptional cofactor in regulation of cholesterol biosynthetic genes (78). In the context of atherosclerosis, adenoviral overexpression of LeXis in the liver reduces atherosclerosis in a familial hypercholesterolemia mouse model (142). As discussed above, CHROME is another LXR-regulated lncRNA involved in cholesterol homeostasis (49). CHROME was first identified through a combination of genetic association studies for premature CAD and HDL-C and microarray based expression profiling in human atherosclerotic plaques (49). RNA-seq of control and CHROME shRNA treated HepG2 hepatocytes revealed downstream pathways affected, including the LXR pathway, bile acid metabolism, cholesterol excretion and fatty-acid β-oxidation pathways (49).
Application of Novel Genomic Technologies to Detect and Study LncRNA Functions
Novel Sequencing Technologies to Discover and Annotate Long Non-coding RNAs
Although next-generation sequencing has resulted in the identification of thousands of lncRNAs in the genome, many of these lncRNAs remain poorly characterized and annotated. It is often unclear where transcription begins and which exons are present in a particular isoform. Since lncRNAs are often expressed at lower levels compared to protein-coding genes, current transcriptomic data is unable to provide comprehensive mapping/characterization of isoforms. However, new sequencing technologies allow for better characterization due to longer read lengths, higher sensitivity, and higher accuracy. Techniques such as Iso-Seq (Pacific Biosystems) offer long-read sequencing using single-molecule, real-time (SMRT) sequencing, in which the sequence of a full-length transcript is captured in a single read (143). Despite these benefits, these single-molecule sequencers yield higher error rates compared with short read sequencing technologies (e.g., Illumina). Nanopore technologies such as the MinION instrument (Oxford Nanopore Technologies) also allow single cDNA molecules to be sequenced without the need for amplification, providing sufficient read lengths to cover the full-length non-coding RNA, and results in less bias than other long-read approaches (144). This technique passes nucleic acids through an orifice 10−9 m in diameter, where instrumental electric current changes are utilized to decipher the identity of each nucleotide (145).
Since lncRNAs are typically less abundant than protein coding genes (usually one order of magnitude less), they remain a challenge to study in bulk transcriptomic datasets. To improve the detection and annotation of lncRNAs, a method known as RACE (Rapid Amplification of cDNA Ends)-Seq was developed (146), however this approach was limited by its low-throughput. Later a technique called RNA CaptureSeq was developed to enrich for long non-coding RNAs (147). RNA CaptureSeq employs an array of oligonucleotide probes to capture select genes of interest, which can be applied to pull-down lncRNAs of interest (148, 149). More recently the GENCODE consortium improved upon RNA CaptureSeq by developing RNA Capture Long Seq (RNA CLS) with the goal of annotating lncRNAs with much higher confidence (150). RNA CLS overcomes the short-read length hurdle of RNA CaptureSeq by first capturing lncRNAs and then integrating with long-read sequencing.
DNA-Based LncRNA Interactions
Despite their low abundance, lncRNAs are known to function through specific molecular interactions with other RNA species and RNA binding proteins. Several high-throughput methods are now available to uncover the genomic DNA sequences that lncRNAs interact with and likely regulate (Figure 2A). Chromatin Isolation by RNA Purification (ChIRP-Seq) is a well-established technique to study lncRNA-chromatin interactions through RNA/chromatin crosslinking, purification using biotinylated antisense oligonucleotides, followed by high-throughput sequencing (151, 152). Domain-specific ChIRP (dChIRP) is a variation of ChIRP that can characterize lncRNA function and architecture at the RNA domain level (153). dChIRP can not only investigate lncRNA-chromatin interactions but also pairwise lncRNA-RNA and lncRNA-protein interactions.
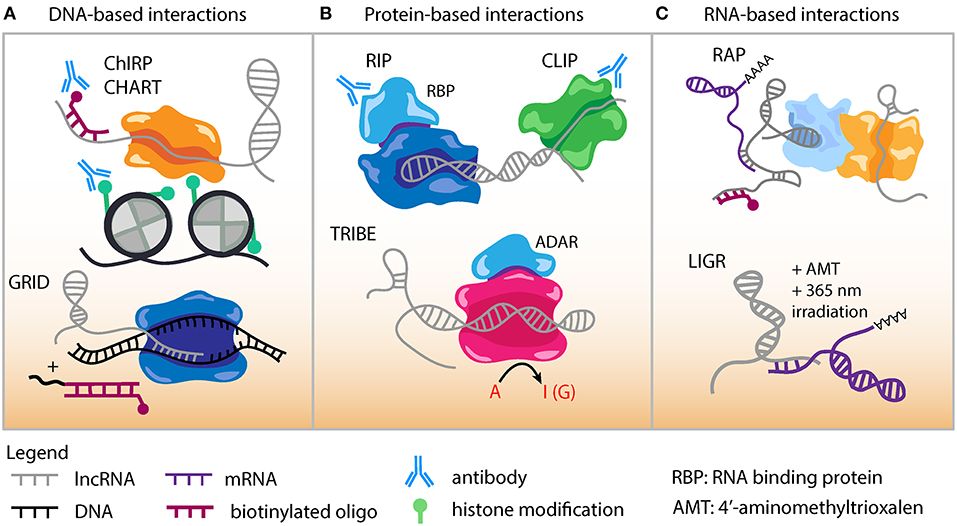
Figure 2. Genomic approaches to capture lncRNA interactions. (A) DNA-based lncRNA interactions include Chromatin Isolation by RNA Purification (ChIRP) and Capture Hybridization. Analysis of RNA Targets (CHART). An in situ based method to capture Global RNA Interactions with DNA (GRID) followed by deep sequencing uses a biotinylated bivalent linker to ligate RNA and dsDNA. (B) Protein-based lncRNA interactions include RNA Immunoprecipitation (RIP) which uses an antibody against RNA binding protein (RBP) to capture RNA-protein interactions. Cross-linking Immunoprecipitation (CLIP) combines UV cross-linking with immunoprecipitation to capture RNA-protein interactions. Targets of RNA-binding proteins Identified By Editing (TRIBE) couples an RBP to an RNA editing enzyme (ADAR). Targets of RBP are marked by adenosine to inositol RNA editing events and identified by sequencing. (C) RNA-based lncRNA interactions include RNA Antisense Purification, which uses a biotinylated probe to capture interacting RNAs that could be followed with sequencing or mass spectrometry. LIGation of interacting RNA (LIGR) followed by sequencing is a powerful approach to capture lncRNA-RNA interactions by in vivo crosslinking of RNA duplexes using the psoralen derivative 4'-aminomethyltrioxalen (AMT) and UV irradiation at 365 nm.
Capture hybridization analysis of RNA targets (CHART) (154, 155) is a similar method to experimentally determine where lncRNAs target and localize in the genome (Figure 2A). In the CHART protocol chromatin is crosslinked and lncRNAs subsequently hybridized to biotinylated C-oligos. After bead immobilization of lncRNA/DNA complexes, sequencing is conducted to identify lncRNA binding DNA regions.
GRID-seq (global RNA interactions with DNA by deep sequencing) is a new unbiased method to capture global RNA-interactions (Figure 2A) that can be applied to investigate lncRNA-DNA interactions in cell lines relevant to atherosclerosis (156). This GRID-seq technique uses a bivalent linker consisting of double-stranded DNA and single-stranded RNA to link RNAs with DNA in nuclei that have been fixed. Finally, MARGI (mapping RNA-genome interactions) is a high-throughput method that can be performed in vivo or on cells and reveal the genomic target sites of lncRNAs (157).
Protein-Based LncRNA Interactions
ChIRP-MS is an adaptation of the ChIRP protocol and used to characterize the interacting proteome for a lncRNA (158). ChIRP-MS has identified protein interactors for lncRNAs such as LeXis, MeXis, and AK098656 (50, 76, 78). lncRNA pull-down followed by mass spectrometry has been conducted for several lncRNAs with potential roles in CAD such as circANRIL (23), STEEL (70), MALAT1 (159, 160), Dnm3os (73), lncLSTR (79), and GATA6-AS (62). Numerous additional methods exist to decipher the proteins binding lncRNAs. RAP-MS uses ultraviolet light to crosslink direct RNA-protein interactions (161). UV-C crosslinking immunoprecipitation (CLIP) is another powerful technique to interrogate direct protein-RNA interactions and many variations have been adapted based on the implementation of high-throughput sequencing (Figure 2B). These include iCLIP, PAR-CLIP, HITS-CLIP, irCLIP, and eCLIP (162–166). High-throughput sequencing of RNA isolation by crosslinking immunoprecipitation (HITS-CLIP) was developed as genome-wide means to interrogate RNA-protein interactions in vivo (164).
TRIBE (targets of RNA-binding proteins identified by editing) is designed for identifying RNA molecules that bind to RNA binding proteins (RBP) (Figure 2B) (167). Advantages of TRIBE include application to in vivo samples, ability to performed on a small number of cells, and no need for antibodies in the procedure. The TRIBE protocol couples an RNA editing enzyme to the RBP and RNA targets that have been edited are identified via next-generation sequencing (TRIBE-seq). HyperTRIBE extends upon the TRIBE procedure by introducing a hyperactive mutation into the RNA editing enzyme, which improves the RNA editing efficiency and reduces the sequence bias of editing (168).
RNA-Based LncRNA Interactions
RNA-centric RNA antisense purification (RAP) is a general approach to identify and study lncRNA functions (Figure 2C). This method uses long capture probes (120 nucleotides) tiled across an entire RNA sequence to pull down lncRNAs, followed by stringent wash conditions to reduce non-specific binding (169). There are now next-generation sequencing derived methodologies that have been established to better define RNA-RNA interactions. LIGR-seq (LIGation of interacting RNA followed by high-throughput sequencing) can capture base-paired RNA-RNA interactions (Figure 2C) (170). In LIGR-seq, RNA duplexes are cross linked with the psoralen derivative 4'-aminomethyltrioxalen (AMT) along with UV irradiation at 365 nm, and RNase R is added to digest linear and structural RNAs. This step enriches for AMT-crosslinked RNA-RNA duplexes that are subsequently subjected to next-generation sequencing. Though LIGR-seq does not work well for small RNAs such as microRNA (miRNA), it should be able to uncover novel dynamic and long range interactions between lncRNAs and other RNA molecules. Various other methods have been developed to study the RNA interactome for lncRNAs with functional relevance in atherosclerosis including PARIS (Psoralen Analysis of RNA Interactions and Structures) (171), SPLASH (172), and MARIO (173). These techniques all can provide valuable information because many lncRNA sequence and structural motifs act as functional scaffolds in the assembly of RNA-protein complexes (17). However, it should be noted that many of these assays could be biased toward capturing stable interactions, while more transient and stimulation specific interactions may require some enrichment steps.
In situ Hybridization-Based Methods
A critical consideration when interrogating a given lncRNA function, is identifying its endogenous tissue and cellular localization. While many lncRNAs are expected to be cytosolic and contribute to post-transcriptional, translational or post-translational gene regulation, nuclear lncRNAs could participate in transcriptional regulation, chromatin structure or mRNA export mechanisms (17). RNA Fluorescence in situ Hybridization (FISH) has been a traditional method to identify the subcellular localization of RNA within cells, however it lacks sensitivity for lowly expressed lncRNAs. Single-molecule RNA FISH (smFISH) is a quantitative technique that provides the sensitivity to detect these lncRNAs and measures absolute transcript levels by using multiple short probes per target RNA (174). However, given that smFISH relies heavily on the optical detection of a limited number of fluorophores, it is restricted in its multiplexing capacity. Attempts to overcome this issue include implementation of combinatorial labeling by spectral barcodes and the incorporation of sequential hybridizations (seqFISH) using different colored probes in each hybridization round (175, 176). In seqFISH individual transcripts are imaged as different colored dots and quantified by counting the number of dots. Multiplex error-robust combinatorial labeling (merFISH) is an in situ targeted approach that utilizes two-step labeling and the detection of binary barcodes assigned to specific targets. This is accomplished by several rounds of hybridization, imaging, and cleavage of fluorophores from probes conjugated to readout sequences that interchange each cycle. Hybridization to readout sequences by the merFISH technique is much less time consuming than methods that utilize hybridization directly to target RNAs (177, 178). RNA SPOTS (sequential probing of targets) follows the same rationale as merFISH, except that it is used in vitro instead of in situ (179).
While still in the nascent stage, the emergence of spatial transcriptomics facilitates integration of RNA-seq expression data with spatial locations of RNA molecules in individual tissue sections (180). In this procedure, fixed tissue samples are annealed to regionally barcoded reverse transcription primers. Following reverse transcription, RNA-seq followed by computational reconstruction allows the two-dimensional localization and quantification of RNA molecules (180). This barcoded method has already been applied to spatially resolve gene expression in the human adult heart (181). While this procedure was originally developed to study mRNAs, it shows promise for the spatial resolution of lncRNAs, given the increased sensitivity and ability to identify context-specific expression profiles. One consideration for atherosclerosis FISH experiments, is that heterogeneous cell types in lesions may be impacted differently by various fixation and hybridization conditions, so careful titration of reagents is recommended.
Other LncRNA Functions
Finally, there are various omics methods that can define the dynamics of lncRNA transcription, stability and RNA modifications. Nascent RNA sequencing analysis, including global nuclear run-on sequencing (GRO-seq) and precision run-on sequencing (PRO-seq) assays, could enable comprehensive detection of transient RNA transcriptional events for multiple RNA species, including mRNA, lncRNA, and eRNA (182). While most transcriptomic datasets capture steady-state levels of lncRNA transcripts, they do not provide direct insights into the stability of lncRNAs. BRIC-seq (5'-bromo-uridine immunoprecipitation chase-deep sequencing analysis) is a method that pulse-labels endogenous RNAs and employs next-generation sequencing to measure RNA decay over time (183). Total RNAs (including lncRNAs) can be isolated from cells at desired time points under various cell-specific perturbations to facilitate functional analysis of lncRNA stability (e.g., lncRNA related to CAD). For example, direct measurements of lncRNA stability in response to CRISPR based loss/gain of gene function or drug treatments could be examined.
Another technique, ICE-seq (inosine chemical erasing coupled with sequencing) (184) represents a promising approach to globally identify lncRNA adenosine to inosine modifications (e.g., in the context of atherosclerosis). Adenosine to inosine (A-to-I) RNA editing is the most abundant form of RNA editing in humans and results from adenosine deaminase acting on RNA (ADAR). A-to-I editing is common to all lncRNAs and affects lncRNA function through altered stability and target recognition (185, 186). A-to-I RNA editing of mRNA has already been demonstrated to have important functional consequences in atherosclerosis. For example, A-to-I editing of cathepsin S mRNA (CTSS) is associated with cathepsin S levels in patients with atherosclerosis. Treatment of endothelial cells with inflammatory cytokines or exposure to hypoxia was shown to induce cathepsin S RNA editing and gene/protein expression (187).
Novel Computational Tools for LncRNA Annotation and Functional Prediction
Genomic annotation of lncRNA sequences requires defining the precise genomic coordinates of lncRNA exons and their respective transcription start sites. LncRNA annotation also involves functional annotation with respect to predicted biological mechanisms, subcellular localization, and affected cell types/tissues. While lncRNAs share some similarities with mRNAs such as transcript length and splicing structure (188), proper identification and characterization of specific long non-coding transcripts still remains a challenge. Unlike mRNAs, lncRNAs often exhibit lower stability, lower abundance, less splicing and greater nuclear localization (189).
With the widespread application of high-throughput sequencing technologies, both automated and manual methods have been adopted to properly define lncRNA sequences from RNA-seq data. Automated annotation generates a larger catalog of lncRNAs and harnesses a transcriptome assembly consisting of two distinct strategies. In one automated approach, reads are first aligned to the reference genome to reveal all the possible splicing events which are subsequently assembled into transcripts (190, 191). In another automated approach, transcripts are built de novo from experimental reads and later aligned to a particular reference genome. Fu et al. (192) used both short and long sequencing reads to demonstrate superior sensitivity of transcript assembly and isoform annotation accuracy with the de novo approach. Automated assembly is fast as it does not require wet-lab based characterization, and it is considerable cheaper than the manual approach (144).
Although it produces a smaller catalog of lncRNAs compared to the automated method, manual annotation produces higher quality lncRNA transcript sequences and thus improves functional characterization. The widely adopted GENCODE project annotation of lncRNAs utilizes a manual curation approach (193), and integrates different sources of data together with computational analyses to generate a transcript model. cDNA and expressed sequence tag (EST) sequences deposited in publicly available databases are typically the starting point for manually annotating lncRNA transcripts. These are integrated with Cap Analysis of Gene Expression (5′-CAGE) and poly(A) position profiling by sequencing (3P-seq) to characterize 5′ and 3′ ends, respectively. These manually annotated transcripts are then mapped to reference genomes and assigned exon and splice site locations (119). The RefSeq (Reference Sequence) project also implements manual annotation of long non-coding RNAs that are integrated with automated methods (194). Manually annotated lncRNAs can be further divided into subclasses such as intergenic lncRNAs (lincRNAs), antisense lncRNAs, and intronic lncRNAs. As cDNA annotation depends on the availability of full length transcripts, manual annotation focuses primarily on genomic annotation. As a result the manual approach produces a more comprehensive set of pseudogenes and alternatively spliced transcripts (193).
Another comprehensive database established in 2016 is NONCODE that dedicates itself to collecting lncRNAs through integration with other databases (e.g., RefSeq and Ensembl) and exhaustive annotation. Compared to these other databases, NONCODE has collected more lncRNA transcripts (excluding tRNAs and rRNAs) and provides unique annotations of lncRNAs (e.g., RNA secondary structure, expression in exosomes, associations between lncRNA and disease) (6, 195). NONCODE also provides lncRNAs for over 15 species including mouse, zebrafish, and C-elegans. The latest version of NONCODE (v5), which also captures lncRNAs from the literature, consists of nearly 550,000 annotated lncRNAs (195).
There are now an array of computational tools to annotate the sequences and functions of the expanding catalog of lncRNAs, as described in Table 2. Existing computational methods for lncRNA identification include those that require a reference genome and those that are reference-free. Examples of methods requiring a reference genome include UClncR, lncScore (205), COME (206), and lncRScan-SVM (207). Reference-free methods to identify lncRNAs from RNA-seq data include LncADeep (198), lncRNAnet (197, 208), FEElnc (197), longdist (204), lncRNA-MFDL (209), and CPC2 (210). Many of these tools employ artificial intelligence algorithms (e.g., machine learning, deep learning) in order to distinguish lncRNAs from their protein-coding transcript counterparts.
Unlike protein functions that can be inferred from protein-coding sequences, it is more difficult to infer lncRNA function from RNA sequences. Zhou et al. developed a tool, lncFunTK that calculates a Functional Information Score (FIS) to quantitatively measure the functional importance of a lncRNA (199), based on the top Gene Ontology and inferred regulatory networks for lncRNAs and their neighboring genes. Another tool, FEELnc, annotates lncRNA function by evaluating neighboring genes to predict both lncRNA function and mRNA partners (197). Given that lncRNA function often depends on subcellular localization, the lncLocator tool predicts five lncRNA categories: nucleus, cytoplasm, cytosol, exosome, and ribosome (200). LncADeep provides enriched pathways and functional modules for lncRNA functional annotation by integrating KEGG and Reactome Pathway databases in a deep learning framework (211). A novel method for lncRNA classification is SEEKR, which counts lncRNA k-mer frequencies from nucleotide sequences, which may be correlated with lncRNA localization or protein binding (202).
Like other ncRNAs, lncRNA functional annotation also depends on accurate secondary structure prediction. There are several computational tools to predict lncRNA structures such as pysster (212), RNAfold (213), RNAstructure (214), and UNAfold (215). Other tools are available to study the evolutionary conservation of lncRNAs, including EvoFold (216), Evolinc (217), RNAz (218), and SISSIz (219). Since lncRNAs are generally poorly conserved, there are strategies to examine remnants of protein coding sequences in these lncRNAs (220). Identifying evolutionarily conserved lncRNA structures and binding domains may provide clues to predict lncRNA function for follow-up experimentation.
Conclusion
The emergence of RNA-seq and other omics technologies in the past decade have catalyzed the identification of a plethora of novel lncRNAs. To date, more than 30 lncRNAs with functional relevance to CAD have been characterized (Table 1), yet numerous lncRNAs remain to be studied in greater detail that are linked to endothelial, smooth muscle, macrophage, and lipid traits. With the growing number of CAD GWAS candidate loci harboring lncRNAs, and improved fine-mapping and annotation approaches, there is an opportunity to functionally dissect these regions to develop novel strategies to target non-coding genomic risk factors. As outlined in this review, a multi-faceted approach is likely required to successfully prioritize and study these lncRNAs, which may include implementation of long-read and high-depth sequencing, improved computational tools, coupled with orthogonal high-throughput experimental validation assays. Careful consideration of the lower abundance, context-specific expression of lncRNAs, and thoughtful study designs may improve chances of success in these multi-omics assays. However, it should also be noted that in many cases, more traditional and lower throughput approaches would be equally appropriate to characterize a given lncRNA, thus reducing the overall costs and required expertise.
For conserved lncRNAs with predicted roles in altering CAD pathogenesis, loss of function studies can be performed in animal models, such as the mouse (ApoE−/− or LDLR−/− backgrounds) or zebrafish. However, with the majority of human lncRNAs being poorly conserved across species, they may be better suited to studies in primary human cells or induced pluripotent stem cell (iPSC) derived vascular cells. In the context of CAD and other cardiometabolic disorders, genetic manipulation of lncRNAs via antisense oligonucleotides (221) or CRISPR/Cas9 to either delete (23, 222, 223) or activate/repress lncRNA expression, may lead to the identification of specific lncRNA binding partners, subcellular localization and functional insights relevant to CAD. lncRNA discovery/annotation can be further improved by integrating these genetic perturbations with high-dimensional transcriptomic and epigenomic assays (e.g., RNA-seq, ATAC-seq and ChIP-seq) to mark lncRNA promoters, decipher RNA polymerase and transcription factor binding, and reveal the dynamics of lncRNA regulatory activities. Single-cell based assays may also shed light on cell-specific markers and dynamics of lncRNAs across lineages (224, 225). Unraveling the complexity of lncRNA function in the setting of atherosclerosis may hold the key to delineate causal disease-associated pathways. In this regard it will also be important to determine whether lncRNAs operate synergistically, serve redundant and/or compensatory roles with other dysregulated lncRNAs and/or mRNAs associated with CAD.
Author Contributions
AT and CM conceived of the manuscript. AT, DW, MK, CD, MP, and CM wrote the manuscript.
Funding
This work was supported by National Institutes of Health (NIH) grants R00 HL125912 (CM) and F31 NR017821 (CD) and a Leducq Foundation Transatlantic Network of Excellence award (CM). The funders had no role in study design, data collection and analysis, decision to publish, or preparation of the manuscript.
Conflict of Interest Statement
The authors declare that the research was conducted in the absence of any commercial or financial relationships that could be construed as a potential conflict of interest.
References
1. Weber C, Noels H. Atherosclerosis: current pathogenesis and therapeutic options. Nat Med. (2011) 17:1410–22. doi: 10.1038/nm.2538
2. Tabas I, García-Cardeña G, Owens GK. Recent insights into the cellular biology of atherosclerosis. J Cell Biol. (2015) 209:13–22. doi: 10.1083/jcb.201412052
3. St. Laurent G, Vyatkin Y, Kapranov P. Dark matter RNA illuminates the puzzle of genome-wide association studies. BMC Med. (2014) 12:97. doi: 10.1186/1741-7015-12-97
4. Liu Y, Zheng L, Wang Q, Hu Y-W. Emerging roles and mechanisms of long noncoding RNAs in atherosclerosis. Int J Cardiol. (2017) 228:570–82. doi: 10.1016/j.ijcard.2016.11.182
5. Djebali S, Davis CA, Merkel A, Dobin A, Lassmann T, Mortazavi A, et al. Landscape of transcription in human cells. Nature (2012) 489:101–8. doi: 10.1038/nature11233
6. Zhao Y, Li H, Fang S, Kang Y, Wu W, Hao Y, et al. NONCODE 2016: an informative and valuable data source of long non-coding RNAs. Nucleic Acids Res. (2016) 44:D203–8. doi: 10.1093/nar/gkv1252
7. Palazzo AF, Lee ES. Non-coding RNA: what is functional and what is junk? Front Genet. (2015) 6:2. doi: 10.3389/fgene.2015.00002
8. Bhartiya D, Scaria V. Genomic variations in non-coding RNAs: Structure, function and regulation. Genomics (2016) 107:59–68. doi: 10.1016/j.ygeno.2016.01.005
9. Costa MC, Leitão AL, Enguita FJ. Biogenesis and mechanism of action of small non-coding RNAs: insights from the point of view of structural biology. Int J Mol Sci. (2012) 13:10268–95. doi: 10.3390/ijms130810268
10. Bhat SA, Ahmad SM, Mumtaz PT, Malik AA, Dar MA, Urwat U, et al. Long non-coding RNAs: mechanism of action and functional utility. Noncoding RNA Res. (2016) 1:43–50. doi: 10.1016/j.ncrna.2016.11.002
11. Bartsch D, Zirkel A, Kurian L. Characterization of Circular RNAs (circRNA) Associated with the Translation Machinery. Methods Mol Biol. (2018). 1724:159–66. doi: 10.1007/978-1-4939-7562-4_13
12. Shi X, Sun M, Liu H, Yao Y, Song Y. Long non-coding RNAs: a new frontier in the study of human diseases. Cancer Lett. (2013) 339:159–66. doi: 10.1016/j.canlet.2013.06.013
13. Guttman M, Amit I, Garber M, French C, Lin MF, Feldser D, et al. Chromatin signature reveals over a thousand highly conserved large non-coding RNAs in mammals. Nature (2009) 458:223–7. doi: 10.1038/nature07672
14. Pang KC, Frith MC, Mattick JS. Rapid evolution of noncoding RNAs: lack of conservation does not mean lack of function. Trends Genet. (2006) 22:1–5. doi: 10.1016/j.tig.2005.10.003
15. Qu Z, Adelson DL. Evolutionary conservation and functional roles of ncRNA. Front Genet. (2012) 3:205. doi: 10.3389/fgene.2012.00205
16. Diederichs S. The four dimensions of noncoding RNA conservation. Trends Genet. (2014) 30:121–3. doi: 10.1016/j.tig.2014.01.004
17. Wang KC, Chang HY. Molecular mechanisms of long noncoding RNAs. Mol Cell. (2011) 43:904–14. doi: 10.1016/j.molcel.2011.08.018
18. Natarelli L, Geißler C, Csaba G, Wei Y, Zhu M, di Francesco A, et al. miR-103 promotes endothelial maladaptation by targeting lncWDR59. Nat Commun. (2018) 9:2645. doi: 10.1038/s41467-018-05065-z
19. Santovito D, Egea V, Weber C. Small but smart: MicroRNAs orchestrate atherosclerosis development and progression. Biochim Biophys Acta (2016) 1861(12 Pt B):2075–86. doi: 10.1016/j.bbalip.2015.12.013
20. Feinberg MW, Moore KJ. Microrna regulation of atherosclerosis. Circ Res. (2016) 118:703–20. doi: 10.1161/CIRCRESAHA.115.306300
21. Jarinova O, Stewart AFR, Roberts R, Wells G, Lau P, Naing T, et al. Functional analysis of the chromosome 9p21.3 coronary artery disease risk locus. Arterioscler Thromb Vasc Biol. (2009) 29:1671–7. doi: 10.1161/ATVBAHA.109.189522
22. Holdt LM, Hoffmann S, Sass K, Langenberger D, Scholz M, Krohn K, et al. Alu elements in ANRIL non-coding RNA at chromosome 9p21 modulate atherogenic cell functions through trans-regulation of gene networks. PLoS Genet. (2013) 9:e1003588. doi: 10.1371/journal.pgen.1003588
23. Holdt LM, Stahringer A, Sass K, Pichler G, Kulak NA, Wilfert W, et al. Circular non-coding RNA ANRIL modulates ribosomal RNA maturation and atherosclerosis in humans. Nat Commun. (2016) 7:12429. doi: 10.1038/ncomms12429
24. Pasmant E, Laurendeau I, Héron D, Vidaud M, Vidaud D, Bièche I. Characterization of a germ-line deletion, including the entire INK4/ARF locus, in a melanoma-neural system tumor family: identification of ANRIL, an antisense noncoding RNA whose expression coclusters with ARF. Cancer Res. (2007) 67:3963–9. doi: 10.1158/0008-5472.CAN-06-2004
25. Schneider C, King RM, Philipson L. Genes specifically expressed at growth arrest of mammalian cells. Cell (1988) 54:787–93. doi: 10.1016/S0092-8674(88)91065-3
26. Coccia EM, Cicala C, Charlesworth A, Ciccarelli C, Rossi GB, Philipson L, et al. Regulation and expression of a growth arrest-specific gene (gas5) during growth, differentiation, and development. Mol Cell Biol. (1992) 12:3514–21. doi: 10.1128/MCB.12.8.3514
27. Chen L, Yang W, Guo Y, Chen W, Zheng P, Zeng J, et al. Exosomal lncRNA GAS5 regulates the apoptosis of macrophages and vascular endothelial cells in atherosclerosis. PLoS ONE (2017) 12:e0185406. doi: 10.1371/journal.pone.0185406
28. Tang R, Zhang G, Wang Y-C, Mei X, Chen S-Y. The long non-coding RNA GAS5 regulates transforming growth factor β (TGF-β)-induced smooth muscle cell differentiation via RNA Smad-binding elements. J Biol Chem. (2017) 292:14270–8. doi: 10.1074/jbc.M117.790030
29. Wang S, Zhang X, Yuan Y, Tan M, Zhang L, Xue X, et al. BRG1 expression is increased in thoracic aortic aneurysms and regulates proliferation and apoptosis of vascular smooth muscle cells through the long non-coding RNA HIF1A-AS1 in vitro. Eur J Cardiothorac Surg. (2015) 47:439–46. doi: 10.1093/ejcts/ezu215
30. Baranello L, Bertozzi D, Fogli MV, Pommier Y, Capranico G. DNA topoisomerase I inhibition by camptothecin induces escape of RNA polymerase II from promoter-proximal pause site, antisense transcription and histone acetylation at the human HIF-1alpha gene locus. Nucleic Acids Res. (2010) 38:159–71. doi: 10.1093/nar/gkp817
31. Bertozzi D, Iurlaro R, Sordet O, Marinello J, Zaffaroni N, Capranico G. Characterization of novel antisense HIF-1α transcripts in human cancers. Cell Cycle (2011) 10:3189–97. doi: 10.4161/cc.10.18.17183
32. Zhao Y, Feng G, Wang Y, Yue Y, Zhao W. Regulation of apoptosis by long non-coding RNA HIF1A-AS1 in VSMCs: implications for TAA pathogenesis. Int J Clin Exp Pathol. (2014) 7:7643–52.
33. Wu G, Cai J, Han Y, Chen J, Huang Z-P, Chen C, et al. LincRNA-p21 regulates neointima formation, vascular smooth muscle cell proliferation, apoptosis, and atherosclerosis by enhancing p53 activity. Circulation (2014) 130:1452–65. doi: 10.1161/CIRCULATIONAHA.114.011675
34. Huarte M, Guttman M, Feldser D, Garber M, Koziol MJ, Kenzelmann-Broz D, et al. A large intergenic noncoding RNA induced by p53 mediates global gene repression in the p53 response. Cell (2010) 142:409–19. doi: 10.1016/j.cell.2010.06.040
35. Michalik KM, You X, Manavski Y, Doddaballapur A, Zörnig M, Braun T, et al. Long noncoding RNA MALAT1 regulates endothelial cell function and vessel growth. Circ Res. (2014) 114:1389–97. doi: 10.1161/CIRCRESAHA.114.303265
36. Song T-F, Huang L-W, Yuan Y, Wang H-Q, He H-P, Ma W-J, et al. LncRNA MALAT1 regulates smooth muscle cell phenotype switch via activation of autophagy. Oncotarget. (2018) 9:4411–26. doi: 10.18632/oncotarget.23230
37. Luo J-H, Ren B, Keryanov S, Tseng GC, Rao UNM, Monga SP, et al. Transcriptomic and genomic analysis of human hepatocellular carcinomas and hepatoblastomas. Hepatology (2006) 44:1012–24. doi: 10.1002/hep.21328
38. Guo F, Li Y, Liu Y, Wang J, Li Y, Li G. Inhibition of metastasis-associated lung adenocarcinoma transcript 1 in CaSki human cervical cancer cells suppresses cell proliferation and invasion. Acta Biochim Biophys Sin (Shanghai) (2010) 42:224–9. doi: 10.1093/abbs/gmq008
39. Koshimizu T, Fujiwara Y, Sakai N, Shibata K, Tsuchiya H. Oxytocin stimulates expression of a noncoding RNA tumor marker in a human neuroblastoma cell line. Life Sci. (2010) 86(11–12):455–60. doi: 10.1016/j.lfs.2010.02.001
40. Guffanti A, Iacono M, Pelucchi P, Kim N, Soldà G, Croft LJ, et al. A transcriptional sketch of a primary human breast cancer by 454 deep sequencing. BMC Genomics (2009) 10:163. doi: 10.1186/1471-2164-10-163
41. Miyoshi N, Wagatsuma H, Wakana S, Shiroishi T, Nomura M, Aisaka K, et al. Identification of an imprinted gene, Meg3/Gtl2 and its human homologue MEG3, first mapped on mouse distal chromosome 12 and human chromosome 14q. Genes Cells (2000) 5:211–20. doi: 10.1046/j.1365-2443.2000.00320.x
42. Wu Z, He Y, Li D, Fang X, Shang T, Zhang H, et al. Long noncoding RNA MEG3 suppressed endothelial cell proliferation and migration through regulating miR-21. Am J Transl Res. (2017) 9:3326–35.
43. Zhu B, Gong Y, Yan G, Wang D, Qiao Y, Wang Q, et al. Down-regulation of lncRNA MEG3 promotes hypoxia-induced human pulmonary artery smooth muscle cell proliferation and migration via repressing PTEN by sponging miR-21. Biochem Biophys Res Commun. (2018) 495:2125–32. doi: 10.1016/j.bbrc.2017.11.185
44. Wang L, Xia J-W, Ke Z-P, Zhang B-H. Blockade of NEAT1 represses inflammation response and lipid uptake via modulating miR-342–3 p in human macrophages THP-1 cells. J Cell Physiol. (2019) 234:5319–26. doi: 10.1002/jcp.27340
45. Ahmed ASI, Dong K, Liu J, Wen T, Yu L, Xu F, et al. Long noncoding RNA NEAT1 (nuclear paraspeckle assembly transcript 1) is critical for phenotypic switching of vascular smooth muscle cells. Proc Natl Acad Sci USA. (2018) 115:E8660–7. doi: 10.1073/pnas.1803725115
46. Hutchinson JN, Ensminger AW, Clemson CM, Lynch CR, Lawrence JB, Chess A. A screen for nuclear transcripts identifies two linked noncoding RNAs associated with SC35 splicing domains. BMC Genomics (2007) 8:39. doi: 10.1186/1471-2164-8-39
47. Imamura K, Imamachi N, Akizuki G, Kumakura M, Kawaguchi A, Nagata K, et al. Long noncoding RNA NEAT1-dependent SFPQ relocation from promoter region to paraspeckle mediates IL8 expression upon immune stimuli. Mol Cell (2014) 53:393–406. doi: 10.1016/j.molcel.2014.06.013
48. Hu Y-W, Guo F-X, Xu Y-J, Li P, Lu Z-F, McVey DG, et al. Long non-coding RNA NEXN-AS1 mitigates atherosclerosis by regulating the actin-binding protein NEXN. J Clin Invest. (2018). doi: 10.1172/JCI98230 [Epub ahead of print].
49. Hennessy EJ, van Solingen C, Scacalossi KR, Ouimet M, Afonso MS, Prins J, et al. The long noncoding RNA CHROME regulates cholesterol homeostasis in primates. Nat Metab. (2018). 1:98–110. doi: 10.1038/s42255-018-0004-9
50. Jin L, Lin X, Yang L, Fan X, Wang W, Li S, et al. AK098656, a novel vascular smooth muscle cell-dominant long noncoding RNA, promotes hypertension. Hypertension (2018) 71:262–72. doi: 10.1161/HYPERTENSIONAHA.117.09651
51. Yu CK, Xu T, Assoian RK, Rader DJ. Mining the stiffness-sensitive transcriptome in human vascular smooth muscle cells identifies long noncoding RNA stiffness regulators. Arterioscler Thromb Vasc Biol. (2018) 38:164–73. doi: 10.1161/ATVBAHA.117.310237
52. Lessard L, Liu M, Marzese DM, Wang H, Chong K, Kawas N, et al. The CASC15 long intergenic noncoding RNA locus is involved in melanoma progression and phenotype switching. J Invest Dermatol. (2015) 135:2464–74. doi: 10.1038/jid.2015.200
53. Feil R, Walter J, Allen ND, Reik W. Developmental control of allelic methylation in the imprinted mouse Igf2 and H19 genes. Development (1994) 120:2933–43.
54. Feinberg AP, Kalikin LM, Johnson LA, Thompson JS. Loss of imprinting in human cancer. Cold Spring Harb Symp Quant Biol. (1994) 59:357–64. doi: 10.1101/SQB.1994.059.01.040
55. Kallen AN, Zhou X-B, Xu J, Qiao C, Ma J, Yan L, et al. The imprinted H19 lncRNA antagonizes let-7 microRNAs. Mol Cell (2013) 52:101–12. doi: 10.1016/j.molcel.2013.08.027
56. Leung A, Trac C, Jin W, Lanting L, Akbany A, Sætrom P, et al. Novel long noncoding RNAs are regulated by angiotensin II in vascular smooth muscle cells. Circ Res. (2013) 113:266–78. doi: 10.1161/CIRCRESAHA.112.300849
57. Zhao J, Zhang W, Lin M, Wu W, Jiang P, Tou E, et al. MYOSLID is a novel serum response factor-dependent long noncoding rna that amplifies the vascular smooth muscle differentiation program. Arterioscler Thromb Vasc Biol. (2016) 36:2088–99. doi: 10.1161/ATVBAHA.116.307879
58. Krawczyk M, Emerson BM. p50-associated COX-2 extragenic RNA (PACER) activates COX-2 gene expression by occluding repressive NF-κB complexes. Elife (2014) 3:e01776. doi: 10.7554/eLife.01776
59. Bell RD, Long X, Lin M, Bergmann JH, Nanda V, Cowan SL, et al. Identification and initial functional characterization of a human vascular cell-enriched long noncoding RNA. Arterioscler Thromb Vasc Biol. (2014) 34:1249–59. doi: 10.1161/ATVBAHA.114.303240
60. Ballantyne MD, Pinel K, Dakin R, Vesey AT, Diver L, Mackenzie R, et al. Smooth muscle enriched long noncoding RNA (SMILR) regulates cell proliferation. Circulation (2016) 133:2050–65. doi: 10.1161/CIRCULATIONAHA.115.021019
61. Singh KK, Matkar PN, Pan Y, Quan A, Gupta V, Teoh H, et al. Endothelial long non-coding RNAs regulated by oxidized LDL. Mol Cell Biochem. (2017) 431:139–49. doi: 10.1007/s11010-017-2984-2
62. Neumann P, Jaé N, Knau A, Glaser SF, Fouani Y, Rossbach O, et al. The lncRNA GATA6-AS epigenetically regulates endothelial gene expression via interaction with LOXL2. Nat Commun. (2018) 9:237. doi: 10.1038/s41467-017-02431-1
63. Miao Y, Ajami NE, Huang T-S, Lin F-M, Lou C-H, Wang Y-T, et al. Enhancer-associated long non-coding RNA LEENE regulates endothelial nitric oxide synthase and endothelial function. Nat Commun. (2018) 9:292. doi: 10.1038/s41467-017-02113-y
64. Ishii N, Ozaki K, Sato H, Mizuno H, Saito S, Takahashi A, et al. Identification of a novel non-coding RNA, MIAT, that confers risk of myocardial infarction. J Hum Genet. (2006) 51:1087–99. doi: 10.1007/s10038-006-0070-9
65. Yan B, Yao J, Liu J-Y, Li X-M, Wang X-Q, Li Y-J, et al. lncRNA-MIAT regulates microvascular dysfunction by functioning as a competing endogenous RNA. Circ Res. (2015) 116:1143–56. doi: 10.1161/CIRCRESAHA.116.305510
66. Willingham AT, Orth AP, Batalov S, Peters EC, Wen BG, Aza-Blanc P, et al. A strategy for probing the function of noncoding RNAs finds a repressor of NFAT. Science (2005) 309:1570–3. doi: 10.1126/science.1115901
67. Tang Y, Wo L, Chai H. [Effects of noncoding RNA NRON gene regulation on human umbilical vein endothelial cells functions]. Zhonghua Xin Xue Guan Bing Za Zhi. (2013) 41:245–50. doi: 10.3760/cma.j.issn.0253-3758.2013.03.015
68. Tsao H-W, Tai T-S, Tseng W, Chang H-H, Grenningloh R, Miaw S-C, et al. Ets-1 facilitates nuclear entry of NFAT proteins and their recruitment to the IL-2 promoter. Proc Natl Acad Sci USA. (2013) 110:15776–81. doi: 10.1073/pnas.1304343110
69. Robb GB, Carson AR, Tai SC, Fish JE, Singh S, Yamada T, et al. Post-transcriptional regulation of endothelial nitric-oxide synthase by an overlapping antisense mRNA transcript. J Biol Chem. (2004) 279:37982–96. doi: 10.1074/jbc.M400271200
70. Man HSJ, Sukumar AN, Lam GC, Turgeon PJ, Yan MS, Ku KH, et al. Angiogenic patterning by STEEL, an endothelial-enriched long noncoding RNA. Proc Natl Acad Sci USA. (2018) 115:2401–6. doi: 10.1073/pnas.1715182115
71. Li K, Blum Y, Verma A, Liu Z, Pramanik K, Leigh NR, et al. A noncoding antisense RNA in tie-1 locus regulates tie-1 function in vivo. Blood (2010) 115:133–9. doi: 10.1182/blood-2009-09-242180
72. Chowdhury TA, Koceja C, Eisa-Beygi S, Kleinstiver BP, Kumar SN, Lin C-W, et al. Temporal and Spatial Post-Transcriptional Regulation of Zebrafish tie1 mRNA by long noncoding RNA during brain vascular assembly. Arterioscler Thromb Vasc Biol. (2018) 38:1562–75. doi: 10.1161/ATVBAHA.118.310848
73. Das S, Reddy MA, Senapati P, Stapleton K, Lanting L, Wang M, et al. Diabetes mellitus-induced long noncoding RNA Dnm3os regulates macrophage functions and inflammation via nuclear mechanisms. Arterioscler Thromb Vasc Biol. (2018) 38:1806–20. doi: 10.1161/ATVBAHA.117.310663
74. Loebel DAF, Tsoi B, Wong N, Tam PPL. A conserved noncoding intronic transcript at the mouse Dnm3 locus. Genomics (2005) 85:782–9. doi: 10.1016/j.ygeno.2005.02.001
75. Du M, Yuan L, Tan X, Huang D, Wang X, Zheng Z, et al. The LPS-inducible lncRNA Mirt2 is a negative regulator of inflammation. Nat Commun. (2017) 8:2049. doi: 10.1038/s41467-017-02229-1
76. Sallam T, Jones M, Thomas BJ, Wu X, Gilliland T, Qian K, et al. Transcriptional regulation of macrophage cholesterol efflux and atherogenesis by a long noncoding RNA. Nat Med. (2018) 24:304–12. doi: 10.1038/nm.4479
77. Halley P, Kadakkuzha BM, Faghihi MA, Magistri M, Zeier Z, Khorkova O, et al. Regulation of the apolipoprotein gene cluster by a long noncoding RNA. Cell Rep. (2014) 6:222–30. doi: 10.1016/j.celrep.2013.12.015
78. Sallam T, Jones MC, Gilliland T, Zhang L, Wu X, Eskin A, et al. Feedback modulation of cholesterol metabolism by the lipid-responsive non-coding RNA LeXis. Nature (2016) 534:124–8. doi: 10.1038/nature17674
79. Li P, Ruan X, Yang L, Kiesewetter K, Zhao Y, Luo H, et al. A liver-enriched long non-coding RNA, lncLSTR, regulates systemic lipid metabolism in mice. Cell Metab. (2015) 21:455–67. doi: 10.1016/j.cmet.2015.02.004
80. Douvris A, Soubeyrand S, Naing T, Martinuk A, Nikpay M, Williams A, et al. Functional analysis of the TRIB1 associated locus linked to plasma triglycerides and coronary artery disease. J Am Heart Assoc. (2014) 3:e000884. doi: 10.1161/JAHA.114.000884
81. Congrains A, Kamide K, Ohishi M, Rakugi H. ANRIL: molecular mechanisms and implications in human health. Int J Mol Sci. (2013) 14:1278–92. doi: 10.3390/ijms14011278
82. Kotake Y, Nakagawa T, Kitagawa K, Suzuki S, Liu N, Kitagawa M, et al. Long non-coding RNA ANRIL is required for the PRC2 recruitment to and silencing of p15(INK4B) tumor suppressor gene. Oncogene (2011) 30:1956–62. doi: 10.1038/onc.2010.568
83. Yap KL, Li S, Muñoz-Cabello AM, Raguz S, Zeng L, Mujtaba S, et al. Molecular interplay of the noncoding RNA ANRIL and methylated histone H3 lysine 27 by polycomb CBX7 in transcriptional silencing of INK4a. Mol Cell. (2010) 38:662–74. doi: 10.1016/j.molcel.2010.03.021
84. Motterle A, Pu X, Wood H, Xiao Q, Gor S, Ng FL, et al. Functional analyses of coronary artery disease associated variation on chromosome 9p21 in vascular smooth muscle cells. Hum Mol Genet. (2012) 21:4021–9. doi: 10.1093/hmg/dds224
85. Zhou X, Han X, Wittfeldt A, Sun J, Liu C, Wang X, et al. Long non-coding RNA ANRIL regulates inflammatory responses as a novel component of NF-κB pathway. RNA Biol. (2016) 13:98–108. doi: 10.1080/15476286.2015.1122164
86. Visel A, Zhu Y, May D, Afzal V, Gong E, Attanasio C, et al. Targeted deletion of the 9p21 non-coding coronary artery disease risk interval in mice. Nature (2010) 464:409–12. doi: 10.1038/nature08801
87. Holdt LM, Teupser D. Long Noncoding RNA ANRIL: Lnc-ing genetic variation at the chromosome 9p21 locus to molecular mechanisms of atherosclerosis. Front Cardiovasc Med. (2018) 5:145. doi: 10.3389/fcvm.2018.00145
88. Arslan S, Berkan Ö, Lalem T, Özbilüm N, Göksel S, Korkmaz Ö, et al. Long non-coding RNAs in the atherosclerotic plaque. Atherosclerosis (2017) 266:176–81. doi: 10.1016/j.atherosclerosis.2017.10.012
89. Cremer S, Michalik KM, Fischer A, Pfisterer L, Jaé N, Winter C, et al. Hematopoietic deficiency of the long non-coding RNA MALAT1 promotes atherosclerosis and plaque inflammation. Circulation (2018). doi: 10.1161/CIRCULATIONAHA.117.029015 [Epub ahead of print].
90. Koldemir O, Özgür E, Gezer U. Accumulation of GAS5 in exosomes is a marker of apoptosis induction. Biomed Rep. (2017) 6:358–62. doi: 10.3892/br.2017.848
91. Aryal B, Rotllan N, Fernández-Hernando C. Noncoding RNAs and atherosclerosis. Curr Atheroscler Rep. (2014) 16:407. doi: 10.1007/s11883-014-0407-3
92. Zhou T, Ding J, Wang X, Zheng X. Long noncoding RNAs and atherosclerosis. Atherosclerosis (2016) 248:51–61. doi: 10.1016/j.atherosclerosis.2016.02.025
93. Gast M, Rauch BH, Nakagawa S, Haghikia A, Jasina A, Haas J, et al. Immune system-mediated atherosclerosis caused by deficiency of long noncoding RNA MALAT1 in ApoE-/- mice. Cardiovasc Res. (2018) 115:302–14. doi: 10.1093/cvr/cvy202
94. Liu J, Huang G-Q, Ke Z-P. Silence of long intergenic noncoding RNA HOTAIR ameliorates oxidative stress and inflammation response in ox-LDL-treated human macrophages by upregulating miR-330–5 p. J Cell Physiol. (2019) 234:5134–42. doi: 10.1002/jcp.27317
95. Soubeyrand S, Naing T, Martinuk A, McPherson R. ERK1/2 regulates hepatocyte Trib1 in response to mitochondrial dysfunction. Biochim Biophys Acta. (2013) 1833:3405–14. doi: 10.1016/j.bbamcr.2013.10.001
96. Kiss-Toth E, Bagstaff SM, Sung HY, Jozsa V, Dempsey C, Caunt JC, et al. Human tribbles, a protein family controlling mitogen-activated protein kinase cascades. J Biol Chem. (2004) 279:42703–8. doi: 10.1074/jbc.M407732200
97. Hannou SA, Wouters K, Paumelle R, Staels B. Functional genomics of the CDKN2A/B locus in cardiovascular and metabolic disease: what have we learned from GWASs? Trends Endocrinol Metab. (2015) 26:176–84. doi: 10.1016/j.tem.2015.01.008
98. Holdt LM, Beutner F, Scholz M, Gielen S, Gäbel G, Bergert H, et al. ANRIL expression is associated with atherosclerosis risk at chromosome 9p21. Arterioscler Thromb Vasc Biol. (2010) 30:620–7. doi: 10.1161/ATVBAHA.109.196832
99. Zhou J, Xu J, Zhang L, Liu S, Ma Y, Wen X, et al. Combined single-cell profiling of lncRNAs and functional screening reveals that H19 is pivotal for embryonic hematopoietic stem cell development. Cell Stem Cell. (2019) 24:285–98.e5. doi: 10.1016/j.stem.2018.11.023
100. Yoshimura H, Matsuda Y, Yamamoto M, Kamiya S, Ishiwata T. Expression and role of long non-coding RNA H19 in carcinogenesis. Front Biosci. (2018) 23:614–25. doi: 10.2741/4608
101. Gao W, Zhu M, Wang H, Zhao S, Zhao D, Yang Y, et al. Association of polymorphisms in long non-coding RNA H19 with coronary artery disease risk in a Chinese population. Mutat Res. (2015) 772:15–22. doi: 10.1016/j.mrfmmm.2014.12.009
102. Zhu R, Liu X, He Z. Long non-coding RNA H19 and MALAT1 gene variants in patients with ischemic stroke in a northern Chinese Han population. Mol Brain (2018) 11:58. doi: 10.1186/s13041-018-0402-7
103. Kim DK, Zhang L, Dzau VJ, Pratt RE. H19, a developmentally regulated gene, is reexpressed in rat vascular smooth muscle cells after injury. J Clin Invest. (1994) 93:355–60. doi: 10.1172/JCI116967
104. Sun W, Lv J, Duan L, Lin R, Li Y, Li S, et al. Long noncoding RNA H19 promotes vascular remodeling by sponging let-7a to upregulate the expression of cyclin D1. Biochem Biophys Res Commun. (2019) 508:1038–42. doi: 10.1016/j.bbrc.2018.11.185
105. Hofmann P, Sommer J, Theodorou K, Kirchhof L, Fischer A, Li Y, et al. Long non-coding RNA H19 regulates endothelial cell aging via inhibition of STAT3 signalling. Cardiovasc Res. (2019) 115:230–42. doi: 10.1093/cvr/cvy206
106. Boon RA, Hofmann P, Michalik KM, Lozano-Vidal N, Berghäuser D, Fischer A, et al. Long noncoding RNA meg3 controls endothelial cell aging and function: implications for regenerative angiogenesis. J Am Coll Cardiol. (2016) 68:2589–91. doi: 10.1016/j.jacc.2016.09.949
107. van der Harst P, Verweij N. Identification of 64 novel genetic loci provides an expanded view on the genetic architecture of coronary artery disease. Circ Res. (2018) 122:433–43. doi: 10.1161/CIRCRESAHA.117.312086
108. Liu B, Pjanic M, Wang T, Nguyen T, Gloudemans M, Rao A, et al. Genetic regulatory mechanisms of smooth muscle cells map to coronary artery disease risk loci. Am J Hum Genet. (2018) 103:377–88. doi: 10.1016/j.ajhg.2018.08.001
109. Arab K, Park YJ, Lindroth AM, Schäfer A, Oakes C, Weichenhan D, et al. Long noncoding RNA TARID directs demethylation and activation of the tumor suppressor TCF21 via GADD45A. Mol Cell. (2014) 55:604–14. doi: 10.1016/j.molcel.2014.06.031
110. Acharya A, Baek ST, Huang G, Eskiocak B, Goetsch S, Sung CY, et al. The bHLH transcription factor Tcf21 is required for lineage-specific EMT of cardiac fibroblast progenitors. Development (2012) 139:2139–49. doi: 10.1242/dev.079970
111. Miller CL, Anderson DR, Kundu RK, Raiesdana A, Nürnberg ST, Diaz R, et al. Disease-related growth factor and embryonic signaling pathways modulate an enhancer of TCF21 expression at the 6q23.2 coronary heart disease locus. PLoS Genet. (2013) 9:e1003652. doi: 10.1371/journal.pgen.1003652
112. Miller CL, Haas U, Diaz R, Leeper NJ, Kundu RK, Patlolla B, et al. Coronary heart disease-associated variation in TCF21 disrupts a miR-224 binding site and miRNA-mediated regulation. PLoS Genet. (2014) 10:e1004263. doi: 10.1371/journal.pgen.1004263
113. Nurnberg ST, Cheng K, Raiesdana A, Kundu R, Miller CL, Kim JB, et al. Coronary artery disease associated transcription factor TCF21 regulates smooth muscle precursor cells that contribute to the fibrous cap. PLoS Genet. (2015) 11:e1005155. doi: 10.1371/journal.pgen.1005155
114. Hormozdiari F, van de Bunt M, Segrè AV, Li X, Joo JWJ, Bilow M, et al. Colocalization of GWAS and eQTL signals detects target genes. Am J Hum Genet. (2016) 99:1245–60. doi: 10.1016/j.ajhg.2016.10.003
115. Gusev A, Ko A, Shi H, Bhatia G, Chung W, Penninx BWJH, et al. Integrative approaches for large-scale transcriptome-wide association studies. Nat Genet. (2016) 48:245–52. doi: 10.1038/ng.3506
116. Kashi K, Henderson L, Bonetti A, Carninci P. Discovery and functional analysis of lncRNAs: Methodologies to investigate an uncharacterized transcriptome. Biochim Biophys Acta. (2016) 1859:3–15. doi: 10.1016/j.bbagrm.2015.10.010
117. Kukurba KR, Montgomery SB. RNA sequencing and analysis. Cold Spring Harb Protoc. (2015) 2015:951–69. doi: 10.1101/pdb.top084970
118. Freedman JE, Miano JM, National Heart, Lung, and Blood Institute Workshop Participants*. Challenges and opportunities in linking long noncoding rnas to cardiovascular, lung, and blood diseases. Arterioscler Thromb Vasc Biol. (2017) 37:21–5. doi: 10.1161/ATVBAHA.116.308513
119. Derrien T, Johnson R, Bussotti G, Tanzer A, Djebali S, Tilgner H, et al. The GENCODE v7 catalog of human long noncoding RNAs: analysis of their gene structure, evolution, and expression. Genome Res. (2012) 22:1775–89. doi: 10.1101/gr.132159.111
120. Conesa A, Madrigal P, Tarazona S, Gomez-Cabrero D, Cervera A, McPherson A, et al. A survey of best practices for RNA-seq data analysis. Genome Biol. (2016) 17:13. doi: 10.1186/s13059-016-0881-8
121. Cabili MN, Trapnell C, Goff L, Koziol M, Tazon-Vega B, Regev A, et al. Integrative annotation of human large intergenic noncoding RNAs reveals global properties and specific subclasses. Genes Dev. (2011) 25:1915–27. doi: 10.1101/gad.17446611
122. Guil S, Soler M, Portela A, Carrère J, Fonalleras E, Gómez A, et al. Intronic RNAs mediate EZH2 regulation of epigenetic targets. Nat Struct Mol Biol. (2012) 19:664–70. doi: 10.1038/nsmb.2315
123. Tsoi LC, Iyer MK, Stuart PE, Swindell WR, Gudjonsson JE, Tejasvi T, et al. Analysis of long non-coding RNAs highlights tissue-specific expression patterns and epigenetic profiles in normal and psoriatic skin. Genome Biol. (2015) 16:24. doi: 10.1186/s13059-014-0570-4
124. Ackers-Johnson M, Talasila A, Sage AP, Long X, Bot I, Morrell NW, et al. Myocardin regulates vascular smooth muscle cell inflammatory activation and disease. Arterioscler Thromb Vasc Biol. (2015) 35:817–28. doi: 10.1161/ATVBAHA.114.305218
125. Chen J, Kitchen CM, Streb JW, Miano JM. Myocardin: a component of a molecular switch for smooth muscle differentiation. J Mol Cell Cardiol. (2002) 34:1345–56. doi: 10.1006/jmcc.2002.2086
126. Wang D-Z, Li S, Hockemeyer D, Sutherland L, Wang Z, Schratt G, et al. Potentiation of serum response factor activity by a family of myocardin-related transcription factors. Proc Natl Acad Sci USA. (2002) 99:14855–60. doi: 10.1073/pnas.222561499
127. Du KL, Ip HS, Li J, Chen M, Dandre F, Yu W, et al. Myocardin is a critical serum response factor cofactor in the transcriptional program regulating smooth muscle cell differentiation. Mol Cell Biol. (2003) 23:2425–37. doi: 10.1128/MCB.23.7.2425-2437.2003
128. Yoshida T, Sinha S, Dandré F, Wamhoff BR, Hoofnagle MH, Kremer BE, et al. Myocardin is a key regulator of CArG-dependent transcription of multiple smooth muscle marker genes. Circ Res. (2003) 92:856–64. doi: 10.1161/01.RES.0000068405.49081.09
129. Russell MR, Penikis A, Oldridge DA, Alvarez-Dominguez JR, McDaniel L, Diamond M, et al. CASC15-S is a tumor suppressor lncRNA at the 6p22 neuroblastoma susceptibility locus. Cancer Res. (2015) 75:3155–66. doi: 10.1158/0008-5472.CAN-14-3613
130. Lim Y-H, Kwon D-H, Kim J, Park WJ, Kook H, Kim Y-K. Identification of long noncoding RNAs involved in muscle differentiation. PLoS ONE (2018) 13:e0193898. doi: 10.1371/journal.pone.0193898
131. Mantella L-E, Singh KK, Sandhu P, Kantores C, Ramadan A, Khyzha N, et al. Fingerprint of long non-coding RNA regulated by cyclic mechanical stretch in human aortic smooth muscle cells: implications for hypertension. Mol Cell Biochem. (2017) 435(1–2):163–73. doi: 10.1007/s11010-017-3065-2
132. Zhang X, Tang X, Hamblin MH, Yin K-J. Long Non-Coding RNA Malat1 regulates angiogenesis in Hindlimb Ischemia. Int J Mol Sci. (2018) 19:E1723. doi: 10.3390/ijms19061723
133. Huang C, Han J, Wu Y, Li S, Wang Q, Lin W, et al. Exosomal MALAT1 derived from oxidized low-density lipoprotein-treated endothelial cells promotes M2 macrophage polarization. Mol Med Report. (2018) 18:509–15. doi: 10.3892/mmr.2018.8982
134. He C, Yang W, Yang J, Ding J, Li S, Wu H, et al. Long noncoding RNA MEG3 negatively regulates proliferation and angiogenesis in vascular endothelial cells. DNA Cell Biol. (2017) 36:475–81. doi: 10.1089/dna.2017.3682
135. Liu J, Li Q, Zhang K-S, Hu B, Niu X, Zhou S-M, et al. Downregulation of the Long Non-Coding RNA Meg3 Promotes Angiogenesis After Ischemic Brain Injury by Activating Notch Signaling. Mol Neurobiol. (2017) 54:8179–90. doi: 10.1007/s12035-016-0270-z
136. Boulberdaa M, Scott E, Ballantyne M, Garcia R, Descamps B, Angelini GD, et al. A role for the long noncoding RNA SENCR in commitment and function of endothelial cells. Mol Ther. (2016) 24:978–90. doi: 10.1038/mt.2016.41
137. Zhang H, Xue C, Wang Y, Shi J, Zhang X, Li W, et al. Deep RNA sequencing uncovers a repertoire of human macrophage long intergenic noncoding rnas modulated by macrophage activation and associated with cardiometabolic diseases. J Am Heart Assoc. (2017) 6:e007431. doi: 10.1161/JAHA.117.007431
138. Xue C, Zhang X, Zhang H, Ferguson JF, Wang Y, Hinkle CC, et al. De novo RNA sequence assembly during in vivo inflammatory stress reveals hundreds of unannotated lincRNAs in human blood CD14+ monocytes and in adipose tissue. Physiol Genomics (2017) 49:287–305. doi: 10.1152/physiolgenomics.00001.2017
139. Hong C, Tontonoz P. Liver X receptors in lipid metabolism: opportunities for drug discovery. Nat Rev Drug Discov. (2014) 13:433–44. doi: 10.1038/nrd4280
140. Parikh M, Patel K, Soni S, Gandhi T. Liver X receptor: a cardinal target for atherosclerosis and beyond. J Atheroscler Thromb. (2014) 21:519–31. doi: 10.5551/jat.19778
141. Zhang Y, Breevoort SR, Angdisen J, Fu M, Schmidt DR, Holmstrom SR, et al. Liver LXRα expression is crucial for whole body cholesterol homeostasis and reverse cholesterol transport in mice. J Clin Invest. (2012) 122:1688–99. doi: 10.1172/JCI59817
142. Tontonoz P, Wu X, Jones M, Zhang Z, Salisbury D, Sallam T. Long noncoding RNA facilitated gene therapy reduces atherosclerosis in a murine model of familial hypercholesterolemia. Circulation (2017) 136:776–8. doi: 10.1161/CIRCULATIONAHA.117.029002
143. Tilgner H, Jahanbani F, Blauwkamp T, Moshrefi A, Jaeger E, Chen F, et al. Comprehensive transcriptome analysis using synthetic long-read sequencing reveals molecular co-association of distant splicing events. Nat Biotechnol. (2015) 33:736–42. doi: 10.1038/nbt.3242
144. Uszczynska-Ratajczak B, Lagarde J, Frankish A, Guigó R, Johnson R. Towards a complete map of the human long non-coding RNA transcriptome. Nat Rev Genet. (2018) 19:535–48. doi: 10.1038/s41576-018-0017-y
145. Jain M, Olsen HE, Paten B, Akeson M. The Oxford Nanopore MinION: delivery of nanopore sequencing to the genomics community. Genome Biol. (2016) 17:239. doi: 10.1186/s13059-016-1103-0
146. Lagarde J, Uszczynska-Ratajczak B, Santoyo-Lopez J, Gonzalez JM, Tapanari E, Mudge JM, et al. Extension of human lncRNA transcripts by RACE coupled with long-read high-throughput sequencing (RACE-Seq). Nat Commun. (2016) 7:12339. doi: 10.1038/ncomms12339
147. Mercer TR, Clark MB, Crawford J, Brunck ME, Gerhardt DJ, Taft RJ, et al. Targeted sequencing for gene discovery and quantification using RNA CaptureSeq. Nat Protoc. (2014) 9:989–1009. doi: 10.1038/nprot.2014.058
148. Clark MB, Mercer TR, Bussotti G, Leonardi T, Haynes KR, Crawford J, et al. Quantitative gene profiling of long noncoding RNAs with targeted RNA sequencing. Nat Methods (2015) 12:339–42. doi: 10.1038/nmeth.3321
149. Bussotti G, Leonardi T, Clark MB, Mercer TR, Crawford J, Malquori L, et al. Improved definition of the mouse transcriptome via targeted RNA sequencing. Genome Res. (2016) 26:705–16. doi: 10.1101/gr.199760.115
150. Lagarde J, Uszczynska-Ratajczak B, Carbonell S, Pérez-Lluch S, Abad A, Davis C, et al. High-throughput annotation of full-length long noncoding RNAs with capture long-read sequencing. Nat Genet. (2017) 49:1731–40. doi: 10.1038/ng.3988
151. Chu C, Quinn J, Chang HY. Chromatin isolation by RNA purification (ChIRP). J Vis Exp. (2012) e3912. doi: 10.3791/3912
152. Chu C, Qu K, Zhong FL, Artandi SE, Chang HY. Genomic maps of long noncoding RNA occupancy reveal principles of RNA-chromatin interactions. Mol Cell (2011) 44:667–78. doi: 10.1016/j.molcel.2011.08.027
153. Quinn JJ, Ilik IA, Qu K, Georgiev P, Chu C, Akhtar A, et al. Revealing long noncoding RNA architecture and functions using domain-specific chromatin isolation by RNA purification. Nat Biotechnol. (2014) 32:933–40. doi: 10.1038/nbt.2943
154. Simon MD, Wang CI, Kharchenko PV, West JA, Chapman BA, Alekseyenko AA, et al. The genomic binding sites of a noncoding RNA. Proc Natl Acad Sci USA. (2011) 108:20497–502. doi: 10.1073/pnas.1113536108
155. Simon MD. Capture hybridization analysis of RNA targets (CHART). Curr Protoc Mol Biol. (2013) Chapter 21:Unit 21.25. doi: 10.1002/0471142727.mb2125s101
156. Li X, Zhou B, Chen L, Gou L-T, Li H, Fu X-D. GRID-seq reveals the global RNA-chromatin interactome. Nat Biotechnol. (2017) 35:940–50. doi: 10.1038/nbt.3968
157. Sridhar B, Rivas-Astroza M, Nguyen TC, Chen W, Yan Z, Cao X, et al. Systematic Mapping of RNA-chromatin interactions in vivo. Curr Biol. (2017) 27:602–9. doi: 10.1016/j.cub.2017.01.011
158. Chu C, Zhang QC, da Rocha ST, Flynn RA, Bharadwaj M, Calabrese JM, et al. Systematic discovery of Xist RNA binding proteins. Cell (2015) 161:404–16. doi: 10.1016/j.cell.2015.03.025
159. Yang L, Lin C, Liu W, Zhang J, Ohgi KA, Grinstein JD, et al. ncRNA- and Pc2 methylation-dependent gene relocation between nuclear structures mediates gene activation programs. Cell (2011) 147:773–88. doi: 10.1016/j.cell.2011.08.054
160. Chen R, Liu Y, Zhuang H, Yang B, Hei K, Xiao M, et al. Quantitative proteomics reveals that long non-coding RNA MALAT1 interacts with DBC1 to regulate p53 acetylation. Nucleic Acids Res. (2017) 45:9947–59. doi: 10.1093/nar/gkx600
161. McHugh CA, Guttman M. RAP-MS: a method to identify proteins that interact directly with a specific RNA molecule in cells. Methods Mol Biol. (2018) 1649:473–88. doi: 10.1007/978-1-4939-7213-5_31
162. Huppertz I, Attig J, D'Ambrogio A, Easton LE, Sibley CR, Sugimoto Y, et al. iCLIP: protein-RNA interactions at nucleotide resolution. Methods (2014) 65:274–87. doi: 10.1016/j.ymeth.2013.10.011
163. Hafner M, Landthaler M, Burger L, Khorshid M, Hausser J, Berninger P, et al. Transcriptome-wide identification of RNA-binding protein and microRNA target sites by PAR-CLIP. Cell (2010) 141:129–41. doi: 10.1016/j.cell.2010.03.009
164. Licatalosi DD, Mele A, Fak JJ, Ule J, Kayikci M, Chi SW, et al. HITS-CLIP yields genome-wide insights into brain alternative RNA processing. Nature (2008) 456:464–9. doi: 10.1038/nature07488
165. Zarnegar BJ, Flynn RA, Shen Y, Do BT, Chang HY, Khavari PA. irCLIP platform for efficient characterization of protein-RNA interactions. Nat Methods (2016) 13:489–92. doi: 10.1038/nmeth.3840
166. Van Nostrand EL, Pratt GA, Shishkin AA, Gelboin-Burkhart C, Fang MY, Sundararaman B, et al. Robust transcriptome-wide discovery of RNA-binding protein binding sites with enhanced CLIP (eCLIP). Nat Methods (2016) 13:508–14. doi: 10.1038/nmeth.3810
167. McMahon AC, Rahman R, Jin H, Shen JL, Fieldsend A, Luo W, et al. TRIBE: Hijacking an RNA-editing enzyme to identify cell-specific targets of RNA-binding proteins. Cell (2016) 165:742–53. doi: 10.1016/j.cell.2016.03.007
168. Rahman R, Xu W, Jin H, Rosbash M. Identification of RNA-binding protein targets with HyperTRIBE. Nat Protoc. (2018) 13:1829–49. doi: 10.1038/s41596-018-0020-y
169. Engreitz J, Lander ES, Guttman M. RNA antisense purification (RAP) for mapping RNA interactions with chromatin. Methods Mol Biol. (2015) 1262:183–97. doi: 10.1007/978-1-4939-2253-6_11
170. Sharma E, Sterne-Weiler T, O'Hanlon D, Blencowe BJ. Global mapping of human RNA-RNA Interactions. Mol Cell. (2016) 62:618–26. doi: 10.1016/j.molcel.2016.04.030
171. Lu Z, Zhang QC, Lee B, Flynn RA, Smith MA, Robinson JT, et al. RNA duplex map in living cells reveals higher-order transcriptome structure. Cell (2016) 165:1267–79. doi: 10.1016/j.cell.2016.04.028
172. Aw JGA, Shen Y, Wilm A, Sun M, Lim XN, Boon K-L, et al. In vivo mapping of Eukaryotic RNA interactomes reveals principles of higher-order organization and regulation. Mol Cell. (2016) 62:603–17. doi: 10.1016/j.molcel.2016.04.028
173. Nguyen TC, Cao X, Yu P, Xiao S, Lu J, Biase FH, et al. Mapping RNA-RNA interactome and RNA structure in vivo by MARIO. Nat Commun. (2016) 7:12023. doi: 10.1038/ncomms12023
174. Femino AM, Fay FS, Fogarty K, Singer RH. Visualization of single RNA transcripts in situ. Science (1998) 280:585–90. doi: 10.1126/science.280.5363.585
175. Shah S, Lubeck E, Zhou W, Cai L. seqFISH accurately detects transcripts in single cells and reveals robust spatial organization in the hippocampus. Neuron (2017) 94:752–758.e1. doi: 10.1016/j.neuron.2017.05.008
176. Lubeck E, Coskun AF, Zhiyentayev T, Ahmad M, Cai L. Single-cell in situ RNA profiling by sequential hybridization. Nat Methods (2014) 11:360–1. doi: 10.1038/nmeth.2892
177. Moffitt JR, Zhuang X. RNA Imaging with Multiplexed Error-Robust Fluorescence in situ Hybridization (MERFISH). Meth Enzymol. (2016) 572:1–49. doi: 10.1016/bs.mie.2016.03.020
178. Moffitt JR, Hao J, Bambah-Mukku D, Lu T, Dulac C, Zhuang X. High-performance multiplexed fluorescence in situ hybridization in culture and tissue with matrix imprinting and clearing. Proc Natl Acad Sci USA. (2016) 113:14456–61. doi: 10.1073/pnas.1617699113
179. Eng C-HL, Shah S, Thomassie J, Cai L. Profiling the transcriptome with RNA SPOTs. Nat Methods (2017) 14:1153–5. doi: 10.1038/nmeth.4500
180. Ståhl PL, Salmén F, Vickovic S, Lundmark A, Navarro JF, Magnusson J, et al. Visualization and analysis of gene expression in tissue sections by spatial transcriptomics. Science (2016) 353:78–82. doi: 10.1126/science.aaf2403
181. Asp M, Salmén F, Ståhl PL, Vickovic S, Felldin U, Löfling M, et al. Spatial detection of fetal marker genes expressed at low level in adult human heart tissue. Sci Rep. (2017) 7:12941. doi: 10.1038/s41598-017-13462-5
182. Wang J, Zhao Y, Zhou X, Hiebert SW, Liu Q, Shyr Y. Nascent RNA sequencing analysis provides insights into enhancer-mediated gene regulation. BMC Genomics (2018) 19:633. doi: 10.1186/s12864-018-5016-z
183. Tani H, Mizutani R, Salam KA, Tano K, Ijiri K, Wakamatsu A, et al. Genome-wide determination of RNA stability reveals hundreds of short-lived noncoding transcripts in mammals. Genome Res. (2012) 22:947–56. doi: 10.1101/gr.130559.111
184. Sakurai M, Ueda H, Yano T, Okada S, Terajima H, Mitsuyama T, et al. A biochemical landscape of A-to-I RNA editing in the human brain transcriptome. Genome Res. (2014) 24:522–34. doi: 10.1101/gr.162537.113
185. Nishikura K. A-to-I editing of coding and non-coding RNAs by ADARs. Nat Rev Mol Cell Biol. (2016) 17:83–96. doi: 10.1038/nrm.2015.4
186. Nigita G, Veneziano D, Ferro A. A-to-I RNA editing: current knowledge sources and computational approaches with special emphasis on non-coding RNA molecules. Front Bioeng Biotechnol. (2015) 3:37. doi: 10.3389/fbioe.2015.00037
187. Stellos K, Gatsiou A, Stamatelopoulos K, Perisic Matic L, John D, Lunella FF, et al. Adenosine-to-inosine RNA editing controls cathepsin S expression in atherosclerosis by enabling HuR-mediated post-transcriptional regulation. Nat Med. (2016) 22:1140–50. doi: 10.1038/nm.4172
188. Guttman M, Rinn JL. Modular regulatory principles of large non-coding RNAs. Nature (2012) 482:339–46. doi: 10.1038/nature10887
189. Schlackow M, Nojima T, Gomes T, Dhir A, Carmo-Fonseca M, Proudfoot NJ. Distinctive patterns of transcription and RNA processing for human lincRNAs. Mol Cell (2017) 65:25–38. doi: 10.1016/j.molcel.2016.11.029
190. Steijger T, Abril JF, Engström PG, Kokocinski F, RGASP Consortium, Hubbard TJ, et al. Assessment of transcript reconstruction methods for RNA-seq. Nat Methods (2013) 10:1177–84. doi: 10.1038/nmeth.2714
191. You B-H, Yoon S-H, Nam J-W. High-confidence coding and noncoding transcriptome maps. Genome Res. (2017) 27:1050–62. doi: 10.1101/gr.214288.116
192. Fu S, Ma Y, Yao H, Xu Z, Chen S, Song J, et al. IDP-denovo: de novo transcriptome assembly and isoform annotation by hybrid sequencing. Bioinformatics (2018) 34:2168–76. doi: 10.1093/bioinformatics/bty098
193. Harrow J, Frankish A, Gonzalez JM, Tapanari E, Diekhans M, Kokocinski F, et al. GENCODE: the reference human genome annotation for The ENCODE Project. Genome Res. (2012) 22:1760–74. doi: 10.1101/gr.135350.111
194. O'Leary NA, Wright MW, Brister JR, Ciufo S, Haddad D, McVeigh R, et al. Reference sequence (RefSeq) database at NCBI: current status, taxonomic expansion, and functional annotation. Nucleic Acids Res. (2016) 44(D1):D733–45. doi: 10.1093/nar/gkv1189
195. Fang S, Zhang L, Guo J, Niu Y, Wu Y, Li H, et al. NONCODEV5: a comprehensive annotation database for long non-coding RNAs. Nucleic Acids Res. (2018) 46(D1):D308–14. doi: 10.1093/nar/gkx1107
196. Hou M, Tang X, Tian F, Shi F, Liu F, Gao G. AnnoLnc: a web server for systematically annotating novel human lncRNAs. BMC Genomics (2016) 17:931. doi: 10.1186/s12864-016-3287-9
197. Wucher V, Legeai F, Hédan B, Rizk G, Lagoutte L, Leeb T, et al. FEELnc: a tool for long non-coding RNA annotation and its application to the dog transcriptome. Nucleic Acids Res. (2017) 45:e57. doi: 10.1093/nar/gkw1306
198. Yang C, Yang L, Zhou M, Xie H, Zhang C, Wang MD, et al. LncADeep: an ab initio lncRNA identification and functional annotation tool based on deep learning. Bioinformatics (2018) 34:3825–34. doi: 10.1093/bioinformatics/bty428
199. Zhou J, Huang Y, Ding Y, Yuan J, Wang H, Sun H. lncFunTK: a toolkit for functional annotation of long noncoding RNAs. Bioinformatics (2018) 34:3415–6. doi: 10.1093/bioinformatics/bty339
200. Cao Z, Pan X, Yang Y, Huang Y, Shen H-B. The lncLocator: a subcellular localization predictor for long non-coding RNAs based on a stacked ensemble classifier. Bioinformatics (2018) 34:2185–94. doi: 10.1093/bioinformatics/bty085
201. Hu Y, Lin J, Hu J, Hu G, Wang K, Zhang H, et al. PennDiff: detecting differential alternative splicing and transcription by RNA sequencing. Bioinformatics (2018) 34:2384–91. doi: 10.1093/bioinformatics/bty097
202. Kirk JM, Kim SO, Inoue K, Smola MJ, Lee DM, Schertzer MD, et al. Functional classification of long non-coding RNAs by k-mer content. Nat Genet. (2018) 50:1474–82. doi: 10.1038/s41588-018-0207-8
203. Sun Z, Nair A, Chen X, Prodduturi N, Wang J, Kocher J-P. UClncR: Ultrafast and comprehensive long non-coding RNA detection from RNA-seq. Sci Rep. (2017) 7:14196. doi: 10.1038/s41598-017-14595-3
204. Schneider HW, Raiol T, Brigido MM, Walter MEMT, Stadler PF. A support vector machine based method to distinguish long non-coding RNAs from protein coding transcripts. BMC Genomics (2017) 18:804. doi: 10.1186/s12864-017-4178-4
205. Zhao J, Song X, Wang K. lncScore: alignment-free identification of long noncoding RNA from assembled novel transcripts. Sci Rep. (2016) 6:34838. doi: 10.1038/srep34838
206. Hu L, Xu Z, Hu B, Lu ZJ. COME: a robust coding potential calculation tool for lncRNA identification and characterization based on multiple features. Nucleic Acids Res. (2017) 45:e2. doi: 10.1093/nar/gkw798
207. Sun L, Liu H, Zhang L, Meng J. lncRScan-SVM: a tool for predicting long non-coding RNAs using support vector machine. PLoS ONE (2015) 10:e0139654. doi: 10.1371/journal.pone.0139654
208. Baek J, Lee B, Kwon S, Yoon S. lncRNAnet: long Non-coding RNA identification using deep learning. Bioinformatics (2018) 34:3889–97. doi: 10.1093/bioinformatics/bty418
209. Fan X-N, Zhang S-W. lncRNA-MFDL: identification of human long non-coding RNAs by fusing multiple features and using deep learning. Mol Biosyst. (2015) 11:892–7. doi: 10.1039/C4MB00650J
210. Kang Y-J, Yang D-C, Kong L, Hou M, Meng Y-Q, Wei L, et al. CPC2: a fast and accurate coding potential calculator based on sequence intrinsic features. Nucleic Acids Res. (2017) 45(W1):W12–6. doi: 10.1093/nar/gkx428
211. Kanehisa M, Goto S, Furumichi M, Tanabe M, Hirakawa M. KEGG for representation and analysis of molecular networks involving diseases and drugs. Nucleic Acids Res. (2010) 38(Database issue):D355–60. doi: 10.1093/nar/gkp896
212. Budach S, Marsico A. pysster: classification of biological sequences by learning sequence and structure motifs with convolutional neural networks. Bioinformatics (2018) 34:3035–7. doi: 10.1093/bioinformatics/bty222
213. Lorenz R, Bernhart SH, Höner Zu Siederdissen C, Tafer H, Flamm C, Stadler PF, et al. ViennaRNA Package 2.0. Algorithms Mol Biol. (2011) 6:26. doi: 10.1186/1748-7188-6-26
214. Reuter JS, Mathews DH. RNAstructure: software for RNA secondary structure prediction and analysis. BMC Bioinformatics (2010) 11:129. doi: 10.1186/1471-2105-11-129
215. Markham NR, Zuker M. UNAFold: software for nucleic acid folding and hybridization. Methods Mol Biol. (2008) 453:3–31. doi: 10.1007/978-1-60327-429-6_1
216. Pedersen JS, Bejerano G, Siepel A, Rosenbloom K, Lindblad-Toh K, Lander ES, et al. Identification and classification of conserved RNA secondary structures in the human genome. PLoS Comput Biol. (2006) 2:e33. doi: 10.1371/journal.pcbi.0020033
217. Nelson ADL, Devisetty UK, Palos K, Haug-Baltzell AK, Lyons E, Beilstein MA. Evolinc: a tool for the identification and evolutionary comparison of long intergenic non-coding RNAs. Front Genet. (2017) 8:52. doi: 10.3389/fgene.2017.00052
218. Gruber AR, Neuböck R, Hofacker IL, Washietl S. The RNAz web server: prediction of thermodynamically stable and evolutionarily conserved RNA structures. Nucleic Acids Res. (2007) 35(Web Server issue):W335–8. doi: 10.1093/nar/gkm222
219. Gesell T, Washietl S. Dinucleotide controlled null models for comparative RNA gene prediction. BMC Bioinformatics (2008) 9:248. doi: 10.1186/1471-2105-9-248
220. Talyan S, Andrade-Navarro MA, Muro EM. Identification of transcribed protein coding sequence remnants within lincRNAs. Nucleic Acids Res. (2018) 46:8720–9. doi: 10.1093/nar/gky608
221. Zhang X, Xue C, Lin J, Ferguson JF, Weiner A, Liu W, et al. Interrogation of nonconserved human adipose lincRNAs identifies a regulatory role of linc-ADAL in adipocyte metabolism. Sci Transl Med. (2018) 10:aar5987. doi: 10.1126/scitranslmed.aar5987
222. Aparicio-Prat E, Arnan C, Sala I, Bosch N, Guigo R, Johnson R. DECKO: Single-oligo, dual-CRISPR deletion of genomic elements including long non-coding RNAs. BMC Genomics (2015) 16:846. doi: 10.1186/s12864-015-2086-z
223. Covarrubias S, Robinson EK, Shapleigh B, Vollmers A, Katzman S, Hanley N, et al. CRISPR/Cas-based screening of long non-coding RNAs (lncRNAs) in macrophages with an NF-KB reporter. J Biol Chem. (2017) 292:20911–20. doi: 10.1074/jbc.M117.799155
224. Kim DH, Marinov GK, Pepke S, Singer ZS, He P, Williams B, et al. Single-cell transcriptome analysis reveals dynamic changes in lncRNA expression during reprogramming. Cell Stem Cell. (2015) 16:88–101. doi: 10.1016/j.stem.2014.11.005
Keywords: long noncoding (lnc) RNAs, genomics, cardiovascular disease, atherosclerosis, gene regulation
Citation: Turner AW, Wong D, Khan MD, Dreisbach CN, Palmore M and Miller CL (2019) Multi-Omics Approaches to Study Long Non-coding RNA Function in Atherosclerosis. Front. Cardiovasc. Med. 6:9. doi: 10.3389/fcvm.2019.00009
Received: 03 November 2018; Accepted: 30 January 2019;
Published: 19 February 2019.
Edited by:
Daniel Duerschmied, University of Freiburg, GermanyReviewed by:
Donato Santovito, Klinikum der Universität München, GermanyVille-Petteri Makinen, South Australian Health and Medical Research Institute (SAHMRI), Australia
Copyright © 2019 Turner, Wong, Khan, Dreisbach, Palmore and Miller. This is an open-access article distributed under the terms of the Creative Commons Attribution License (CC BY). The use, distribution or reproduction in other forums is permitted, provided the original author(s) and the copyright owner(s) are credited and that the original publication in this journal is cited, in accordance with accepted academic practice. No use, distribution or reproduction is permitted which does not comply with these terms.
*Correspondence: Clint L. Miller, clintm@virginia.edu