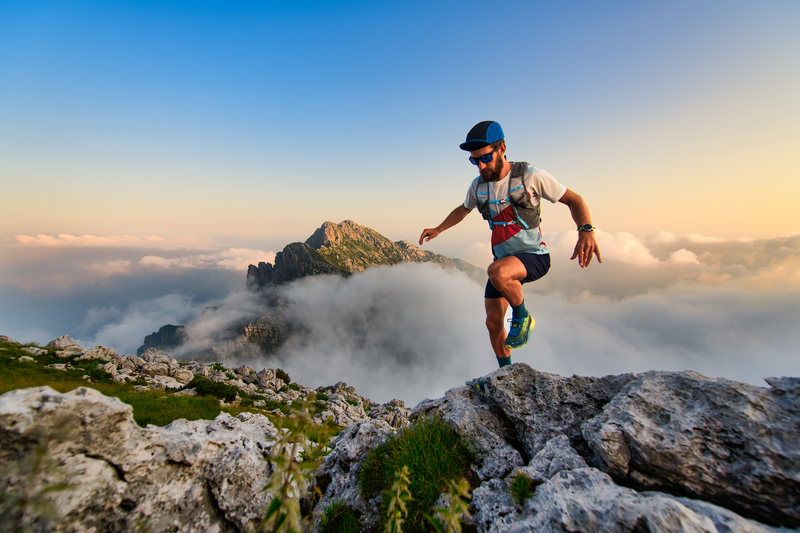
95% of researchers rate our articles as excellent or good
Learn more about the work of our research integrity team to safeguard the quality of each article we publish.
Find out more
ORIGINAL RESEARCH article
Front. Cell. Infect. Microbiol. , 21 February 2020
Sec. Microbiome in Health and Disease
Volume 10 - 2020 | https://doi.org/10.3389/fcimb.2020.00024
This article is part of the Research Topic Host-Microbiome Interactions and Influence on Performance During Acute Environmental, Nutritional, Physical, and Cognitive Stress View all 7 articles
Chronic urticaria (CU) is defined as the continuous or intermittent presence of urticaria for a period exceeding 6 weeks and sometimes occurring with angioedema. Between 66 and 93% of patients with CU have chronic spontaneous urticaria (CSU), the precise pathogenesis of which is largely unknown. The aim of this study was to determine the relationship between gut microbiota and serum metabolites and the possible pathogenesis underlying CSU. We collected feces and blood samples from CSU patients and healthy controls and the relationship between gut microbiota and serum metabolites was assessed using 16S rRNA gene sequencing and untargeted metabolomic analyses. The CSU group exhibited decreased alpha diversity of the microbial population compared to the control group. The abundance of unidentified Enterobacteriaceae was increased, while the abundance of Bacteroides, Faecalibacterium, Bifidobacterium, and unidentified Ruminococcaceae was significantly reduced in CSU patients. The serum metabolome analysis revealed altered levels of docosahexaenoic acid, arachidonic acid, glutamate, and succinic acid, suggesting changes in unsaturated fatty acids and the butanoate metabolism pathway. The combined serum metabolomics and gut microbiome datasets were correlated; specifically, docosahexaenoic acid, and arachidonic acid were positively correlated with Bacteroides. We speculate that alterations in gut microbes and metabolites may contribute to exacerbated inflammatory responses and dysregulated immune function with or without regulatory T cell dependence in the pathogenesis of CSU.
Chronic urticaria (CU) is defined by the continuous or intermittent presence of urticaria for a period exceeding 6 weeks and sometimes occurring with angioedema. CU can be classified into chronic inducible urticaria (CINDU) and chronic spontaneous urticaria (CSU) based on the triggering factor (Bernstein et al., 2014; Zuberbier et al., 2014b). Approximately 20% of individuals experience acute urticaria at least once during their lifetime, and 0.1% will develop chronic symptoms (Greaves, 2000; Zuberbier et al., 2014a). Between 66 and 93% of patients with CU have CSU. The main treatment option for CSU is to control symptoms, which significantly affect the quality of life patients (Fine and Bernstein, 2016).
The precise pathogenesis underlying CSU is largely unknown; however, CSU is considered to an immune-mediated inflammatory disease (Varghese et al., 2016). Gut microbiota has an important role in maintaining health through evolution and regulation of the immune system (Knight et al., 2017). Alterations in the gut microbiota has been shown to be associated with allergic diseases, including asthma (Arnold et al., 2011), food allergies (Ling et al., 2014), and atopic dermatitis (Hulshof et al., 2017). We previously conducted an epidemiologic investigation and found relevant evidence linking gut microbiota and CSU. Several studies have suggested that the changes in gut microbiota composition are linked to CU, including Akkermansia muciniphila, Faecalibacterium prausnitzii, the Clostridium leptum group, the Enterobacteriaceae family (Nabizadeh et al., 2017), and the Lactobacillus, Bifidobacterium, and Bacteroides genera (Rezazadeh et al., 2018); however, there is no specific mechanism by which to explore the dysbiosis of gut microbiota in the pathogenesis of CSU.
Combining the microbiome and metabolome may provide reliable and comprehensive information to elucidate the microbiologic-related pathogenesis underlying CSU and the metabolome can disclose the relevant metabolic pathways. Therefore, the aim of this study was to determine the relationship between gut microbiota and serum metabolites and the possible pathogenesis underlying CSU.
A total of 100 CSU patients and 100 healthy individuals (18–75 years of age) were enrolled in this study between June 2018 and June 2019. The diagnosis of CSU was established according to the American Academy of Allergy, Asthma, and Immunology criteria (Bernstein et al., 2014). The exclusion criteria were as follows: antibiotic use in the past month; abnormal levels of vitamin D and coagulation parameters; or any known disease (allergic rhinitis, asthma, eczema, obesity, and diabetes). A questionnaire was completed for each participant. We randomly selected 10 patients with CSU and 10 healthy individuals after matching for age and gender [mean [SD] age, 45.1 [4.94] years in each group; 5 [50%] males in each group] for further gut microbiome and serum metabolome analyses. All of the participants signed the informed consent. This study was approved by the Ethics Committee at the Shanxi Medical University. The principles of the 1964 Helsinki declaration and its later amendments were followed.
Approximately 2 g of fresh stool samples were collected from patients and healthy controls and frozen at −80°C until further studies. Bacterial DNA was extracted from stool samples using the CTAB/SDS method. Distinct region genes (16S rRNA/18S rRNA/ITS) were amplified specific primers (e.g., 16S-V4+V5, 515F: 5′-GTGCCAGCMGCCGCGGTAA-3′, 907R: 5′-CCGTCAATTCCTTTGAGTTT-3′) with barcodes before polymerase chain reaction (PCR) product mixing and purification were used. Sequencing libraries were generated and the library was sequenced on an Ion Plus Fragment Library Kit 48 rxns (Thermo Scientific, Philadelphia, Massachusetts, USA) after assessing quality on a Qubit@ 2.0 fluorometer (Thermo Scientific, Philadelphia, Massachusetts, USA). The last 400 bp/600 bp single-end reads were generated (Supplementary File 1).
Sequence analysis was performed and a representative sequence for each operational taxonomic unit (OTUs) was screened for further annotation using Uparse software (Uparse v7.0.1001) (Edgar, 2013). Sequences with >97% similarity were assigned to the same OTU. Diversity analysis was performed, and the Silva Database (https://www.arb-silva.de/) was used based on the Mothur algorithm to annotate taxonomic information. To study the phylogenetic relationship of different OTUs, and the differences in the dominant species between samples (groups), multiple sequence alignment was performed using MUSCLE software (Version 3.8.31) (Edgar, 2004). Analysis of alpha and beta diversity was performed based on output normalized data. Tax4Fun functional prediction was achieved by the nearest neighbor method based on the minimum 16S rRNA sequence similarity (Supplementary File 1).
Approximately 200 ul of serum samples were obtained from patients and healthy controls and stored at −80°C for further analysis. The filtrate was injected into the liquid chromatography-tandem mass spectrometry (LC-MS/MS) system after a series of treatments. LC-MS/MS analyses were performed using a Vanquish ultra-high-pressure liquid chromatograph (UHPLC) system (Thermo Fisher, Philadelphia, Massachusetts, USA) coupled with an Orbitrap Q Exactive HF-X mass spectrometer (Thermo Fisher, Philadelphia, Massachusetts, USA). The raw data files generated by UHPLC-MS/MS were processed using Compound Discoverer 3.0 (CD 3.0, Thermo Fisher, Philadelphia, Massachusetts, USA) to perform peak alignment, peak picking, and quantification for each metabolite. Then, peak intensities were normalized to the total spectral intensity. The normalized data were used to predict the molecular formula based on additive ions, molecular ion peaks, and fragment ions. The peaks were matched with the mzCloud (https://www.mzcloud.org/) and ChemSpider (http://www.chemspider.com/) databases to obtain the accurate qualitative and relative quantitative results. Finally, the functional and taxonomic of identified metabolites were annotated through KEGG (http://www.kegg.jp/) (Supplementary File 2).
Data were derived from the questionnaire using EpiData database double entry and the established database was analyzed by single factor analysis (χ2-test). The variables were analyzed by multi-factor logistic regression (P < 0.05) with SPSS23.0 statistical software. Alpha and beta diversity were calculated with QIIME (Version1.9.1) and displayed with R software (Version 2.15.3). Differential abundances of genera and metabolites were analyzed by parametric and non-parametric tests, including t-tests and Wilcoxon rank sum tests. Principal component analysis (PCA), fold change analysis, and partial least squares discriminant analysis (PLS-DA) were performed by MetaX software (Version 2.68). The metabolites with significant differences between 16S rRNA analysis and metabolomics analysis were analyzed based on the Pearson correlation coefficient. To further verify the authenticity of the correlation between bacteria and metabolites, a scatter plot was used. An adjusted p-value < 0.05 was considered statistically significant unless stated otherwise.
Two hundred participants completed the survey. There were 51 males and 49 females in the patient group, with a median age of 43.22 + 12.5 years. There were 48 males and 52 females in the healthy control group, with an average age of 39.04 + 10.6 years. No significant differences in age or gender existed between the two groups. Compared with the healthy controls, univariate analysis showed that place of residence, whether or not antibiotics were used during pregnancy, mode of production, feeding mode, dietary habits, whether, or not antibiotics were used in the past year, whether or not surgery was performed in the last 6 months, mental trauma and other stress factors, and whether or not alcohol consumption were significantly related to the onset of CSU (P < 0.05) (Supplementary Table 1). Multivariate logistic regression analysis showed that antibiotic use during pregnancy, cesarean section, a high-fat, low-carbohydrate diet, antibiotic use in the past year, and alcohol consumption were independent risk factors for CSU (Table 1).
After quality control, an average of 76,183 valid data were obtained, 1,983 OTUs were identified, then the OTU sequence and Silva Database were annotated. A total of 1,010 (50.93%) OTUs were annotated to the genera level. Participants were divided into the two groups in the Principal Co-ordinates Analysis (PCoA) (Figure 1A). Alpha diversity was applied to determine the ecologic diversity, including observed species, ACE, and Shannon. There were significant differences (P = 0.01) in the observed species measured between CSU patients and healthy controls (Figure 1B). Both the ACE and Shannon indices reflect the reduced community richness, evenness, and species diversity in CSU patients, which showed a statistically significant difference (Figure 1B).
Figure 1. Microbiome multivariate analysis. (A) Principal Component Analysis (PCoA) based on unweighted UniFrac distances between gut bacterial communities of CSU patients and healthy controls. (B) Alpha-diversity indexes for each sample group, showing the adjusted p-value computed using Wilcoxon rank sum test. (C) Bacterial composition and abundance in phylum level. Each bar represents the top ten bacterial species ranked by the relative abundance in CSU patients and healthy controls. (D) The relative abundance of significantly altered bacterial taxa including phylum, genus levels in the CSU group compared to that in the control group (*P < 0.05). (E) Taxonomic distributions of bacteria at the genus level (top 30) between CSU patients and healthy controls. (F) Lefse analysis was performed on the bacterial taxa relative abundance values between the two groups. Bacterial with LDA score >2.0 and P < 0.05 were considered to be significantly discriminant. (G) The effect of the altered gut microbiota on predicted functional metabolic pathways.
As shown in Figure 1C, the two groups were mainly composed of Firmicutes, Bacteroidetes, Proteobacteria, and Actinobacteria. Firmicutes is the most prominent gut bacterial community, accounting for 63 and 59% of CSU patients and healthy controls, respectively. At the phylum level, the relative abundance of Firmicutes in CSU patients was markedly decreased compared to healthy controls (Figure 1D). At the genus level, members of the Bacteroides, Faecalibacterium, Bifidobacterium, Lactobacillus, and unidentified Ruminococcaceae were relatively lower and unidentified Enterobacteriaceae were relatively increased compared to healthy controls (Figure 1E). And unidentified Enterobacteriaceae were increased, while Bacteroides, Faecalibacterium, Bifidobacterium, and unidentified Ruminococcaceae were decreased in CSU patients (Figure 1D).
LEfSe analysis showed that the CSU patients were characterized by a higher abundance of unidentified Enterobacteriaceae, whereas the healthy controls primarily showed higher enrichment with Firmicutes, Bacteroides and Bifidobacteriales (LDA score >2.0 with P < 0.05) (Figure 1F). Tax4Fun functional prediction mainly indicated that modifications of the gut microbiota may lead to changes in related metabolic pathways, such as nucleotide metabolism (Figure 1G).
LC-MS/MS analyses separated the identified metabolite profiles into positive and negative ion modes (Want et al., 2010; Dunn et al., 2011). The number of differential metabolites in the two groups was 154 in the positive ion mode, of which 60 were significantly up-regulated, and 56 were in the negative ion mode, of which 26 were significantly up-regulated (Figure 2A). These metabolites included amino acids (glutamic acid and succinic acid), fatty acids (docosahexaenoic acid and arachidonic acid), carbohydrates (sucrose), and purines (Supplementary File 3). The PLS-DA scores showed an obvious separation trend in serum metabolic profiles between the CSU and control groups (Figure 2B). The heatmap analysis revealed a remarkable difference between the composition of CSU serum metabolites and the healthy controls (Figure 2C).
Figure 2. Metabolic profile multivariate analysis. (A) Volcano plot of the identified metabolites in positive ion mode (left) and in negative ion mode (right). (B) Plot of OPLS-DA scores of the control (red) and CSU (blue) groups. (C) The heatmap showed the abundance of metabolites in different clusters from each sample.
Spearman correlation analysis showed that docosahexaenoic acid and arachidonic acid had a positive correlation with Bacteroides. L-glutamic acid had a negative correlation with unidentified Enterobacteriaceae and a positive correlation with Bifidobacterium. Succinic acid showed a positive correlation with Faecalibacterium (Figure 3A). According to the KEGG-enriched bubble chart, we demonstrated biosynthesis of unsaturated fatty acids and butanoate metabolism in the positive and negative ion modes, respectively. The former has significant difference between CSU patients and healthy controls (Figure 3B). Interestingly, the KEGG pathway map results indicated that docosahexaenoic acid and arachidonic acid belongs to the biosynthesis of unsaturated fatty acid metabolism, while L-glutamic acid and succinic acid were products of butanoate metabolism (Figure 3C).
Figure 3. Correlation analysis of differential gut microbiota and metabolites. (A) Heatmap of correlation between microbial genus abundances and serum metabolites in positive ion mode (left) and in negative ion mode (right). Only significant correlations (p ≤ 0.05) are colored. Positive correlations are indicated in blue and negative correlations in red. (B) Metabolites were annotated into different metabolic pathways between the positive ion mode (left) and in negative ion mode (right) by KEGG enriched bubble chart based on KEGG. (C) Metabolic pathway map in CSU patients. Green solid circles are marked as annotated metabolites, and blue circles are marked as down-regulated differential metabolites.
We demonstrated that antibiotic use during pregnancy, cesarean section, high-fat, low-carbohydrate diets, antibiotic use in the past year, and alcohol consumption are independent risk factors for CSU. Recent evidence revealed that a high-fat diet in mice altered the intestinal microbiota composition; specifically, the number of Bifidobacterium were significantly reduced (Zhou et al., 2019). Several studies have shown that the administration of antibiotics can lead to disorders of intestinal flora and decreased microbial diversity (Angelucci et al., 2019; Sun et al., 2019). Therefore, through epidemiologic investigation, we found an indirect relationship between intestinal microbial and the incidence of CSU.
We further analyzed the composition and diversity of microbes by Illumina-based 16S rRNA gene sequencing. Both the ACE and Shannon indices reflected a reduction in the bacterial diversity and richness in CSU patients, which is highly consistent with other allergic diseases (Bisgaard et al., 2011). At the phylum level, when compared with healthy controls, there was a relative reduced abundance of Bacteroidetes and Firmicutes in CSU patients, which is partly in agreement with the airway allergies in young children (Chiu et al., 2019). At the genus level, members of the Bacteroides, Faecalibacterium, Bifidobacterium, Lactobacillus, and unidentified Ruminococcaceae were relatively lower and members of unidentified Enterobacteriaceae were relatively increased compared to healthy controls, which is partly consistent with previous studies about CU or allergic diseases (Sjogren et al., 2009; Johansson et al., 2011; Candela et al., 2012; Nabizadeh et al., 2017; Rezazadeh et al., 2018; Chiu et al., 2019). For example, Nabizadeh et al. (2017) and Rezazadeh et al. (2018) reported a depletion in the relative amounts of Faecalibacterium prausnitzii, Clostridia, Akkermansia muciniphila, Bifidobacterium, and Lactobacillus in CU patients. The mean of the relative amounts of Enterobacteriaceae was increased. In the current study, we did not detect Akkermansia muciniphila in the top 30 bacterial species at the genus level.
The genus Bacteroides is defined as a gram-negative, rod-shaped, obligate anaerobic that plays an important role in maintaining immune homeostasis and regulating immunity and has an association with unsaturated fatty acids (Lee et al., 2003). Recent research has examined that reduced maternal n-3 polyunsaturated fatty acid exposure resulted in a significant decrease of Epsilonproteobacteria, Bacteroides, and Akkermansia and increased relative abundance of Clostridia (Robertson et al., 2018). Similar results have been reported that unsaturated fatty acids have a stimulating effect on the growth of Bacteroides (Mirjafari Tafti et al., 2019). Our findings showed a significant reduction in Bacteroides in patients with CSU and a positive correlation between docosahexaenoic acid and arachidonic acid. Furthermore, these two metabolites were down-regulated in the unsaturated fatty acid biosynthesis pathway. Unsaturated fatty acids have anti-inflammatory properties and suppresses inflammatory responses (Calder and Grimble, 2002; Morrison and Preston, 2016). Therefore, we concluded that the reduction in unsaturated fatty acids exacerbated the inflammatory responses to promote the development of CSU.
The metabolome also confirmed that there were differences in butanoate metabolism between CSU patients and healthy controls. Butyrate is involved in the maintenance of intestinal epithelial cells and plays a significant role in regulating intestinal immune tolerance to antigens (Chang et al., 2014). Recent evidence revealed that the abundance of butyrate was decreased in children with asthma and was negatively correlated with serum IgE levels. This suggests that the dysregulation of the intestinal epithelial barrier due to reduced butyrate secretion may be related to allergen uptake and immunological responses in allergic asthma (Chiu et al., 2019). We detected a decrease in the serum levels of glutamate and succinic acid in CSU patients which both belong to butanoate metabolism. More importantly, a reduction in Bifidobacterium and Faecalibacterium, which had a positive correlation with glutamate and succinic acid, respectively. The increased in unidentified Enterobacteriaceae had a negative correlation with glutamate. The results indicated that lower butyrate levels due to dysregulation of the gut microbiota may play an important role in the pathogenesis of CSU.
Ruminococcus is a genus of strictly anaerobic, Gram-positive, non-motile cocci, and is one of the most predominant organisms in the colon from the phylum Firmicutes (Hold et al., 2002; La Reau and Suen, 2018). Arshi et al. reported that a reduction in the population of Ruminococcus in the gut induces inflammatory responses by Toll-like receptors and subsequently participates in the development of eczema in infancy (Arshi et al., 2014). In our study, the abundance of unidentified Ruminococcaceae was significantly lower in CSU patients, which is consistent with previous studies of allergic diseases (West et al., 2015; Simonyte Sjödin et al., 2016). In addition, several previous studies demonstrated that the number and function of regulatory T (Treg) cells was reduced in CU patients (Chen et al., 2008; Arshi et al., 2014). In summary, we speculate that depletion of certain bacteria, such as Ruminococcus, may modulate the immune function of Treg cells by inducing Toll-like receptors contributing to CSU.
Our study revealed profound changes in the composition of gut microbes-metabolites and the corresponding relationship, including Bacteroides associated with docosahexaenoic acid and arachidonic acid, Bifidobacterium, Faecalibacterium, and unidentified Enterobacteriaceae associated with glutamate and succinic acid, and the decreased abundance of Ruminococcus. Taken together, we speculate that the changes may contribute to exacerbated inflammatory responses and dysregulated immune function with or without Treg cell dependence in the pathogenesis of CSU. The major limitation of this study was the relatively small population of participants. More research is needed to understand the mechanism underlying these associations.
All datasets generated for this study are included in the article/Supplementary Material.
The studies involving human participants were reviewed and approved by Ethics Committee at the Shanxi Medical University. The patients/participants provided their written informed consent to participate in this study.
HH and LG collected samples and made clinical records. HC, DW, and LG performed the experiments. HC conceived the study and will oversee all aspects of the project. DW and SG wrote the first draft of this manuscript. All authors read, approved the final manuscript, and made an intellectual contribution to this study.
This study was supported by the National Natural Science Foundation (81502714), Doctoral Fund of the First Hospital of Shanxi Medical University (YB161703), Second-class Subsidies for Postdoctoral Science Foundation Project in China (2017M611197), Scientific and Technological Innovation Projects of Colleges and Universities in Shanxi Province (20171102).
The authors declare that the research was conducted in the absence of any commercial or financial relationships that could be construed as a potential conflict of interest.
The Supplementary Material for this article can be found online at: https://www.frontiersin.org/articles/10.3389/fcimb.2020.00024/full#supplementary-material
Angelucci, F., Cechova, K., Amlerova, J., and Hort, J. (2019). Antibiotics, gut microbiota, and Alzheimer's disease. J. Neuroinflammation 16:108. doi: 10.1186/s12974-019-1494-4
Arnold, I. C., Dehzad, N., Reuter, S., Martin, H., Becher, B., Taube, C., et al. (2011). Helicobacter pylori infection prevents allergic asthma in mouse models through the induction of regulatory T cells. J. Clin. Invest. 121, 3088–3093. doi: 10.1172/JCI45041
Arshi, S., Babaie, D., Nabavi, M., Tebianian, M., Ghalehbaghi, B., Jalali, F., et al. (2014). Circulating level of CD4+ CD25+ FOXP3+ T cells in patients with chronic urticaria. Int. J. Dermatol. 53, e561–e566. doi: 10.1111/ijd.12630
Bernstein, J. A., Lang, D. M., Khan, D. A., Craig, T., Dreyfus, D., Hsieh, F., et al. (2014). The diagnosis and management of acute and chronic urticaria: 2014 update. J. Allergy Clin. Immunol. 133, 1270–1277. doi: 10.1016/j.jaci.2014.02.036
Bisgaard, H., Li, N., Bonnelykke, K., Chawes, B. L., Skov, T., Paludan-Müller, G., et al. (2011). Reduced diversity of the intestinal microbiota during infancy is associated with increased risk of allergic disease at school age. J. Allergy Clin. Immunol. 128, 646–652. e1–5. doi: 10.1016/j.jaci.2011.04.060
Calder, P. C., and Grimble, R. F. (2002). Polyunsaturated fatty acids, inflammation and immunity. Eur. J. Clin. Nutr. 56, S14–S19. doi: 10.1038/sj.ejcn.1601478
Candela, M., Rampelli, S., Turroni, S., Severgnini, M., Consolandi, C., De Bellis, G., et al. (2012). Unbalance of intestinal microbiota in atopic children. BMC Microbiol. 12:95. doi: 10.1186/1471-2180-12-95
Chang, P. V., Hao, L., Offermanns, S., and Medzhitov, R. (2014). The microbial metabolite butyrate regulates intestinal macrophage function via histone deacetylase inhibition. Proc. Natl. Acad. Sci. U.S.A. 111, 2247–2252. doi: 10.1073/pnas.1322269111
Chen, W. C., Chiang, B. L., Liu, H. E., Leu, S. J., and Lee, Y. L. (2008). Defective functions of circulating CD4+CD25+ and CD4+CD25- T cells in patients with chronic ordinary urticaria. J. Dermatol. Sci. 51, 121–130. doi: 10.1016/j.jdermsci.2008.02.012
Chiu, C. Y., Chan, Y. L., Tsai, M. H., Wang, C. J., Chiang, M. H., and Chiu, C. C. (2019). Gut microbial dysbiosis is associated with allergen-specific IgE responses in young children with airway allergies. World Allergy Organ. J. 12:100021. doi: 10.1016/j.waojou.2019.100021
Dunn, W. B., Broadhurst, D., Begley, P., Zelena, E., Francis-McIntyre, S., Anderson, N., et al. (2011). Procedures for large-scale metabolic profiling of serum and plasma using gas chromatography and liquid chromatography coupled to mass spectrometry. Nat. Protoc. 6, 1060–1083. doi: 10.1038/nprot.2011.335
Edgar, R. C. (2004). MUSCLE: multiple sequence alignment with high accuracy and high throughput. Nucleic Acids Res. 32, 1792–1797. doi: 10.1093/nar/gkh340
Edgar, R. C. (2013). UPARSE: highly accurate OTU sequences from microbial amplicon reads. Nat. Methods 10, 996–998. doi: 10.1038/nmeth.2604
Fine, L. M., and Bernstein, J. A. (2016). Guideline of chronic urticaria beyond. Allergy Asthma Immunol. Res. 8, 396–403. doi: 10.4168/aair.2016.8.5.396
Greaves, M. (2000). Chronic urticaria. J. Allergy Clin. Immunol. 105, 664–672. doi: 10.1067/mai.2000.105706
Hold, G. L., Pryde, S. E., Russell, V. J., Furrie, E., and Flint, H. J. (2002). Assessment of microbial diversity in human colonic samples by 16S rDNA sequence analysis. FEMS Microbiol. Ecol. 39, 33–39. doi: 10.1111/j.1574-6941.2002.tb00904.x
Hulshof, L., Van't Land, B., Sprikkelman, A. B., and Garssen, J. (2017). Role of microbial modulation in management of atopic dermatitis in children. Nutrients 9:E854. doi: 10.3390/nu9080854
Johansson, M. A., Sjögren, Y. M., Persson, J. O., Nilsson, C., and Sverremark-Ekström, E. (2011). Early colonization with a group of Lactobacilli decreases the risk for allergy at five years of age despite allergic heredity. PLoS ONE 6:e23031. doi: 10.1371/journal.pone.0023031
Knight, R., Callewaert, C., Marotz, C., Hyde, E. R., Debelius, J. W., McDonald, D., et al. (2017). The microbiome and human biology. Annu. Rev. Genomics Hum. Genet. 183, 65–86. doi: 10.1146/annurev-genom-083115-022438
La Reau, A. J., and Suen, G. (2018). The Ruminococci: key symbionts of the gut ecosystem. J. Microbiol. 56, 199–208. doi: 10.1007/s12275-018-8024-4
Lee, J. Y., Plakidas, A., Lee, W. H., Heikkinen, A., Chanmugam, P., Bray, G., et al. (2003). Differential modulation of Toll-like receptors by fatty acids preferential inhibition by n-3 polyunsaturated fatty acids. J. Lipid Res. 44, 479–486. doi: 10.1194/jlr.M200361-JLR200
Ling, Z., Li, Z., Liu, X., Cheng, Y., Luo, Y., Tong, X., et al. (2014). Altered fecal microbiota composition associated with food allergy in infants. Appl. Environ. Microbiol. 80, 2546–2554. doi: 10.1128/AEM.00003-14
Mirjafari Tafti, Z. S., Moshiri, A., Ettehad Marvasti, F., Tarashi, S., Sadati Khalili, S. F., Motahhary, A., et al. (2019). The effect of saturated and unsaturated fatty acids on the production of outer membrane vesicles from Bacteroides fragilis and Bacteroides thetaiotaomicron. Gastroenterol. Hepatol. Bed Bench 12, 155–162. doi: 10.22037/ghfbb.v12i2.1523
Morrison, D. J., and Preston, T. (2016). Formation of short chain fatty acids by the gut microbiota and their impact on human metabolism. Gut Microbes 7, 189–200. doi: 10.1080/19490976.2015.1134082
Nabizadeh, E., Jazani, N. H., Bagheri, M., and Shahabi, S. (2017). Association of altered gut microbiota composition with chronic urticaria. Ann. Allergy Asthma Immunol. 119, 48–53. doi: 10.1016/j.anai.2017.05.006
Rezazadeh, A., Shahabi, S., Bagheri, M., Nabizadeh, E., and Jazani, N. H. (2018). The protective effect of Lactobacillus and Bifidobacterium as the gut microbiota members against chronic urticaria. Int. Immunopharmacol. 59, 168–173. doi: 10.1016/j.intimp.2018.04.007
Robertson, R. C., Kaliannan, K., Strain, C. R., Ross, R. P., Stanton, C., et al. (2018). Maternal omega-3 fatty acids regulate offspring obesity through persistent modulation of gut microbiota. Microbiome 6:95. doi: 10.1186/s40168-018-0476-6
Simonyte Sjödin, K., Vidman, L., Rydén, P., and West, C. E. (2016). Emerging evidence of the role of gut microbiota in the development of allergic diseases. Curr. Opin. Allergy Clin. Immunol. 16, 390–395. doi: 10.1097/ACI.0000000000000277
Sjogren, Y. M., Jenmalm, M. C., Bottcher, M. F., Bjorksten, B., and Sverremark-Ekstrom, E. (2009). Altered early infant gut microbiota in children developing allergy up to 5 years of age. Clin. Exp. Allergy 39, 518–526. doi: 10.1111/j.1365-2222.2008.03156.x
Sun, Y., Liu, T., Si, Y., Cao, B., Zhang, Y., Zheng, X., et al. (2019). Integrated metabolomics and 16S rRNA sequencing to investigate the regulation of Chinese yam on antibiotic-induced intestinal dysbiosis in rats. Artif Cells Nanomed. Biotechnol. 47, 3382–3390. doi: 10.1080/21691401.2019.1649271
Varghese, R., Rajappa, M., Chandrashekar, L., Kattimani, S., Archana, M., Munisamy, M., et al. (2016). Association among stress, hypocortisolism, systemic inflammation, and disease severity in chronic urticaria. Ann. Allergy Asthma Immunol. 116, 344–348.e1. doi: 10.1016/j.anai.2016.01.016
Want, E. J., Wilson, I. D., Gika, H., Theodoridis, G., Plumb, R. S., Shockcor, J., et al. (2010). Global metabolic profiling procedures for urine using UPLC–MS. Nat Protoc. 5, 1005–1018. doi: 10.1038/nprot.2010.50
West, C. E., Rydén, P., Lundin, D., Engstrand, L., Tulic, M. K., and Prescott, S. L. (2015). Gut microbiome and innate immune response patterns in IgE-associated eczema. Clin. Exp. Allergy 45, 1419–1429. doi: 10.1111/cea.12566
Zhou, Y., Zhao, S., Jiang, Y., Wei, Y., and Zhou, X. (2019). Regulatory function of buckwheat-resistant starch supplementation on lipid profile and gut microbiota in mice fed with a high-fat diet. J. Food Sci. 84, 2674–2681. doi: 10.1111/1750-3841.14747
Zuberbier, T., Aberer, W., Asero, R., Bindslev-Jensen, C., Brzoza, Z., Canonica, G. W., et al. (2014b). Methods report on the development of the 2013 revision and update of the EAACI/GA2 LEN/EDF/WAO guideline for the definition, classification, diagnosis, and management of urticaria. Allergy 69, e1–29. doi: 10.1111/all.12370
Keywords: chronic spontaneous urticaria (CSU), pathogenesis, gut microbiota, serum metabolites, correlation analysis
Citation: Wang D, Guo S, He H, Gong L and Cui H (2020) Gut Microbiome and Serum Metabolome Analyses Identify Unsaturated Fatty Acids and Butanoate Metabolism Induced by Gut Microbiota in Patients With Chronic Spontaneous Urticaria. Front. Cell. Infect. Microbiol. 10:24. doi: 10.3389/fcimb.2020.00024
Received: 11 October 2019; Accepted: 15 January 2020;
Published: 21 February 2020.
Edited by:
Nicholas Zachos, Johns Hopkins University, United StatesReviewed by:
Yuchen Liu, Shenzhen University, ChinaCopyright © 2020 Wang, Guo, He, Gong and Cui. This is an open-access article distributed under the terms of the Creative Commons Attribution License (CC BY). The use, distribution or reproduction in other forums is permitted, provided the original author(s) and the copyright owner(s) are credited and that the original publication in this journal is cited, in accordance with accepted academic practice. No use, distribution or reproduction is permitted which does not comply with these terms.
*Correspondence: Hongzhou Cui, bWVuanVuY3VpQDE2My5jb20=
†These authors have contributed equally to this work
Disclaimer: All claims expressed in this article are solely those of the authors and do not necessarily represent those of their affiliated organizations, or those of the publisher, the editors and the reviewers. Any product that may be evaluated in this article or claim that may be made by its manufacturer is not guaranteed or endorsed by the publisher.
Research integrity at Frontiers
Learn more about the work of our research integrity team to safeguard the quality of each article we publish.