- 1Lab for Molecular Design & Pharmaceutical Biophysics, Department of Pharmacy and Biochemistry, Institute of Pharmaceutical Sciences, Eberhard Karls Universität Tübingen, Tübingen, Germany
- 2Center for Bioinformatics Tübingen (ZBIT), Eberhard Karls Universität Tübingen, Tübingen, Germany
Halogen bonds have recently gained attention in life sciences and drug discovery. However, it can be difficult to harness their full potential, when newly introducing them into an established hit or lead structure by molecular design. A possible solution to overcome this problem is the use of halogen-enriched fragment libraries (HEFLibs), which consist of chemical probes that provide the opportunity to identify halogen bonds as one of the main features of the binding mode. Initially, we have suggested the HEFLibs concept when constructing a focused library for finding p53 mutant stabilizers. Herein, we broaden and extent this concept aiming for a general HEFLib comprising a huge diversity of binding motifs and, thus, increasing the applicability to various targets. Using the construction principle of feature trees, we represent each halogenated fragment by treating all simple to complex substituents as modifiers of the central (hetero)arylhalide. This approach allows us to focus on the proximal binding interface around the halogen bond and, thus, its integration into a network of interactions based on the fragment's binding motif. As a first illustrative example, we generated a library of 198 fragments that unifies a two-fold strategy: Besides achieving a diversity-optimized basis of the library, we have extended this “core” by structurally similar “satellite compounds” that exhibit quite different halogen bonding interfaces. Tuning effects, i.e., increasing the magnitude of the σ-hole, can have an essential influence on the strength of the halogen bond. We were able to implement this key feature into the diversity selection, based on the rapid and efficient prediction of the highest positive electrostatic potential on the electron isodensity surface, representing the σ-hole, by VmaxPred.
Introduction
The manifold and constantly increasing application of halogen bonding (XB) in different areas of life sciences, e.g., biomolecular engineering (Carlsson et al., 2018) and drug discovery (Scholfield et al., 2013; Sirimulla et al., 2013; Zimmermann et al., 2014), emphasizes the demand for a profound understanding of the requirements and versatility of this highly directed molecular interaction. XB as a non-bonded interaction is established by the attraction of a partially positive region at the halogen in extension of the R-X axis, where X is in most cases a chlorine, bromine or iodine atom and R is an electron withdrawing group, with an electron donor moiety, i.e., a π- or n-electrons (Clark et al., 2007; Politzer et al., 2010). Note that also fluorine can undergo halogen bonding in rather rare situations which are unlikely to be observable in drug discovery projects (Metrangolo et al., 2011; Eskandari and Lesani, 2015). This region of positive electrostatic potential is called the σ-hole and can be explained by the electron configuration of the heavier halides , where the pz orbital is oriented along the R-X axis. In most cases of simple and symmetric molecules, this positive region is surrounded by a negative belt (Clark et al., 2007). The binding energy of halogen bonding varies between very weak and strong ionically assisted interactions (180 kJ/mol of ionic complexes), depending on the interaction partners (Metrangolo et al., 2005; Domagała et al., 2018). While certainly dependent on the type of the halogen atom and the Lewis base (LB), interaction hotspots can typically be expected at distances dX⋯LB of ~2.75–3.5 Å and σ-hole angles αC−X⋯LB between 155 and 180° (Wilcken et al., 2013). These short optimal distances can be explained by the non-spherically symmetric electron densities of heavy halides, which is described by the term “polar flattening” (Sedlak et al., 2015). The σ-hole can be characterized by its magnitude, size, linearity, and range (Kolár and Hobza, 2016). The magnitude is commonly expressed as the maximum electrostatic potential (ESP) value (called VS, max or Vmax) located on the halogen surface of electron density of 0.001 or 0.002 au (Murray and Politzer, 1991; Riley et al., 2011). At this electron density, 96–97% of the molecular charge is included (Bader et al., 1987; Politzer et al., 2010; Kolár and Hobza, 2016). Different (hetero)aromatic ring systems as well as their substitution patterns have a significant influence on the shape and the magnitude of the σ-hole (see Figure 1) (Lange et al., 2019). This effect is also known as “tuning” (Riley et al., 2011, 2013).
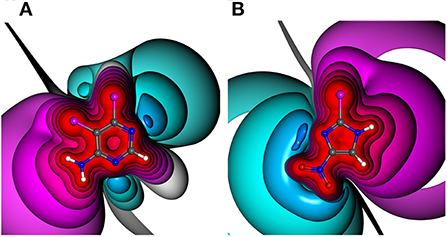
Figure 1. Electrostatic potential (ESP) plots of different halogen-bearing aromatic ring systems with net charge of null. ESP from −0.34 au (dark blue) via zero (white) to +0.34 au (red). Example molecules out of generated HEFLib: (A) 5,6-dichloro-4-pyrimidinamine and (B) 2-bromo-4-nitro-1H-imidazole.
The rather narrow geometric demands for good to optimal halogen bonds can be challenging barriers in the process of molecular design, especially when trying to introduce them into an already established and more complex framework of interactions between a protein and a ligand. To overcome these difficulties and to identify halogen bonding “hot spots” of a protein, while including the advantages of fragment-based drug discovery (FBDD), halogen-enriched fragment libraries (HEFLibs) can be used as a suitable toolkit. This concept was already successfully applied in order to identify p53 mutant stabilizers (Wilcken et al., 2012). The unconventional binding motifs of these fragments resulted in a series of ligands with remarkable affinities. FBDD is driven by the idea to identify molecular hits for a drug target by using less and significantly smaller compounds than these of conventional lead-like or drug-like libraries (Ray et al., 2017). In comparison to different estimates of drug discovery-relevant molecules in chemical space, from 16.6·1010 for up to 17 non-hydrogen atoms to 1060 for up to 30 non-hydrogen atoms, the size of the chemical space from which typical fragment libraries are selected is much smaller (Bohacek et al., 1996; Ruddigkeit et al., 2012). By applying simple combinatorics it is obvious that the higher the atom count of a molecular graph, the higher the number of molecules that can be generated. In the reverse case, the smaller the number of atoms, the lower the number of possible enumerations. Thus, medium-sized fragment libraries are considered better representatives of their respective chemical space, than large-scale HTS libraries. The binding of a small molecule to a drug target is induced by a number of directed and non-directed interactions. In case of fragments, the number of possible interactions per molecule is smaller than those of drug-like molecules. In line with the concept of “molecular complexity and obesity” (Hann et al., 2001; Hann, 2011), small parts of a binding site can accommodate a fragment with higher ligand efficiency (Keserü and Makara, 2009; Schultes et al., 2010) at the cost of lower absolute affinities. As a consequence, more targets can be addressed resulting in a higher hit rate (Chessari and Woodhead, 2009) of weak binders. To compensate for these low affinities, screening experiments are performed at relatively high concentrations, requiring a high solubility of the fragments (Boyd et al., 2012). By growing or linking small-sized hits to lead structures, new drug candidates with higher affinities can be created (Erlanson, 2011; Trapero et al., 2018). Whereas, drug-like libraries are commonly built with respect to Lipinski's rule of five (Lipinski et al., 2001), for fragment libraries the rule of three (Congreve et al., 2003) is applied, which requires an octanol-water partition coefficient logP ≤ 3, MW ≤ 300 Da, not more than 3 hydrogen bond donors or acceptors and not more than 3 rotatable bonds. Such restriction enforce a small number of interactions per hit (Joseph-McCarthy et al., 2014). Strategic enrichment of a desired interaction type can significantly increase the probability of observing this particular interaction as a key binding motif. Fragment libraries can either be unfocused or they can be designed for addressing serine, cysteine (Backus et al., 2016; Craven et al., 2018) and lysine residues by establishing covalent bonds (Kathman and Statsyuk, 2016) with distinct functional groups like boronic acids, epoxides, acrylamides, or N-succinimidyl ester.
In addition, they can be designed to address difficultly shaped binding pockets by a considerable fraction of sp3-hybridized moieties. Another possible strategy is to design a fragment library focused on a specific target by including a knowledge-based set of suitable interaction motif into each fragment, e.g., well-known kinase hinge binder (Xing et al., 2015).
Herein, we present a generalized design strategy for halogen-enriched fragment libraries (HEFLibs) as chemical probes for identifying halogen bonds as key binding elements. Tuning effects of halogen atoms connected to (hetero)aromatic ring systems are included as a selection criterion into the feature tree-like (Rarey and Dixon, 1998) diversity assessment aiming for a maximal interface diversity proximal to the halogen bond. By treating each aromatic ring system with a connected heavier halogen, i.e., chlorine, bromine, or iodine, as the central element for molecular similarity assessment and all of its substituents as modifiers, we created a similarity measure that is tailor-made for emphasizing the influence of the local environment of the respective halogen on the possible interaction pattern of this “binding motif.” At the same time, the influence of more halogen-distant molecular features is decreased. This construction principle of a rooted tree, based on the abstraction of the molecular graph, was successfully implemented to predict Vmax (Heidrich et al., 2018) and is herein utilized for the diversity assessment of halogenated fragments. As a first showcase application, we compiled a library of 198 fragments that consists of a diversity-optimized “core” which is extended by structurally similar, but with regard to their halogen bonding interface quite different, “satellite compounds.” When applying this diversity-optimized HEFLib to a variety of different target proteins, we propose that the experimental results can inspire a better understanding of geometric requirements and tuning effects of halogen bonds. Thus, it can be a useful tool for studying halogen bonding with biochemical, biophysical and structural methods. In addition, the use of our diversity-optimized HEFLib can yield hits with unprecedented binding modes and unique features for patenting.
Methods
Diversity Assessment
In contrast to the original implementation of feature trees by Rarey and Dixon (1998) our approach uses a rooted tree, where the aromatic ring with a halogen defines the key property for diversity assessment.
All simple and complex substituents (e.g., single hydrogen atoms or annulated ring systems) connected to this central aromatic ring are treated as residues resulting in a halogen interface-focused diversity measure (see Figure 2). Similarity assessment of two halogenated fragments is done by the pair-wise comparison of their aromatic moieties and their connected residues with regard to the topological constitution. By taking topologically shifted and unmatched residues into consideration, an optimal matching is determined. Each halogen attached to an aromatic ring is considered as a single “configuration” that is pair-wise compared to another molecule's configuration by preserving the nodal matching between the two halogen atoms (for an example see Figure 2). Besides the basic nodal properties of the central aromatic moiety and its attached halogen (see yellow and blue circle in Figure 2), information about charges, i.e., positively and negatively charged atoms, hydrogen bond donors/acceptors, rings, and linkers are included. Thereby defined nodes contain property values of atom count (Todeschini and Consonni, 2009), lone-pair electrostatic interaction (Cheng and Yuan, 2006; Todeschini and Consonni, 2009), information index on proton-neutron composition (Bonchev et al., 1976; Todeschini and Consonni, 2009), kappa shape index-related flexibility index (Kier, 1989; Hall and Kier, 2007; Todeschini and Consonni, 2009), edge connectivity index (Cash, 1995; Estrada, 1995; Todeschini and Consonni, 2009), and group electronegativity (Zhou et al., 2007; Todeschini and Consonni, 2009). Moreover, each node that encodes an aromatic halogen atom contains the information of the predicted Vmax value at the electron isodensity level of 0.020 au (Heidrich et al., 2018), which was derived by a machine-learned SVM model. Furthermore, atom types and topologies of the central aromatic ring are taken into consideration. Different weighting parameters for feature tree-like comparison were empirically determined by generating small test cases and comparing the output to the desired relative similarity.
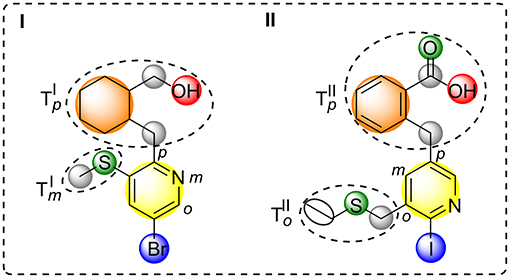
Figure 2. Schematic depiction of feature tree-like comparison of two molecules (I,II). Comparison node-based on aromatic ring (yellow) that carries halogen (blue) and defines the central molecular property, hydrogen bond acceptors and donors (green and red), ring structures (orange), and linkers (gray). Rooted comparison of ortho, meta, and para sub tree of molecule I and II ().
Solubility Prediction
Fragment solubility is one of the key properties for a successful in vitro screening, since fragments are known to bind only with a millimolar to high micromolar affinity, screening experiments are conducted under relatively high ligand concentrations. We applied a consensus prediction scheme using six known solubility predictors [AlogPS 2.1 (Tetko et al., 2001; Tetko and Tanchuk, 2002), WSKOWIN 1.24 (U.S. Environmental Protection Agency, 2017), ESOL (Delaney, 2004), MOE (Chemical Computing Group ULC, 2018), QPlogS and CIQPlogS of Schrödinger Suite] of which three need to predict a logS better than −2 in case of a heavy atom count (HAC) small than 16, or better than −1 for fragments with up to 22 heavy atoms. For predicted solubility values see Figure S1.
Experimental Solubility Assessment
Turbidimetric solubility assessment of fragments available in larger quantities was done in 50 mM HEPES pH 7.0 and 100 mM NaCl at room temperature. Ninety-six-well plate containing ligand stocks in DMSO of 100 mM was diluted by factor 5/6 in DMSO. Diluted compounds were added to buffer resulting in 5% DMSO in 200 μL using VIAFLO 96 automated multi-channel pipette (INTEGRA Biosciences Deutschland GmbH). Measurement at 600–800 nm was done in five kinetic cycles of each 115 s with double orbital shaking at 300 rpm for 60 s before readout using CLARIOstar (BMG Labtech) resulting in a total observation time of approximately 15 min. Since compounds showed no significant absorption at higher dilution levels in this wavelength spectrum, any increase in absorption is assumed to be based on insoluble particles/precipitation of the ligand.
Filtering
Pricing information was gathered from Aldrich Market Select website and used as hard limit ($5/mg). Compounds without aromatic halogen and larger than 20 heavy atoms were removed from vendor library. Additionally, unwanted and reactive substructures were removed by applying SMARTS filter. All structure filtering steps were done using KNIME (Berthold et al., 2007) and the RDKit (Landrum, 2006).
Fragment Selection
A set of 150 diverse “core” compounds was selected applying a MaxMin (Ashton et al., 2002) picking scheme with different initially selected compounds from the pair-wise calculated distance matrix of 2,685 compounds of Aldrich Market Select that fulfill the minimum requirements defined above. Additionally, the five most similar “satellite” compounds were denoted and a sub selection was done with expert opinion, based on affordability and predicted solubility, resulting in 200 compounds. By including affordable and highly soluble “satellite” compounds into our library, we aimed to increase the probability of reaching high ligand concentrations in in vitro experiments for each of the selected halogen bonding interfaces of the “core” compounds. Due to delivery problems, 2 compounds could not be ordered resulting in the final HEFLib with 198 fragments. The library provided in SMILES codes and respective properties of each fragment can be downloaded as Supplementary Material (Data Sheet 1).
Results
The Necessity for an Advanced XB Interface Description
Halogen bonding is typically rationalized by considering the electrostatic features of the ligand and target. Still, it should not be forgotten that to some extend induction, dispersion (Politzer et al., 2010; Riley et al., 2013) and charge transfer (Rezáč and de la Lande, 2016) will also play a role in halogen bonding. The electrostatic potential (ESP) (Murry and Politzer, 2011), as a physical observable, was used to define and visualize the XB interface of the fragments in our library. The high directionality of halogen bonding can be explained by the electron anisotropy of heavy halides (chlorine, bromine, and iodine) and their belt of negative electrostatic potential surrounding the σ-hole in case of simple aryl halides. Nevertheless, this symmetric shape can be significantly altered by neighboring groups and heteroaromatic scaffolds, having a strong impact on optimal interaction angles and distances. Our initial, unfocused library design aims at highly diverse XB interfaces, where the halogen bond is embedded in a multitude of chemical environments, leading to variations of the electrostatic features and resulting pharmacophoric interactions with a putative binding site (see Figure 3).
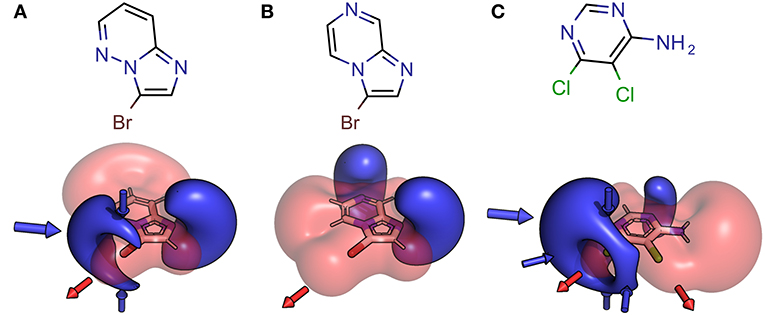
Figure 3. Structural formula and 3D depictions of electrostatic potentials, illustrating similarities and diversities with respect to chemotype and XB interface (binding motif). Three examples from the herein presented showcase HEFLib are shown. Pharmacophoric arrows indicate typical vectors of electrophilic attack toward the ligand (blue) or toward the target by σ-hole interactions (red). Upon shifting the pyridine-type nitrogen atom from position 5 in 3-bromoimidazo[1,2-b]pyridazine (A) to position 7 in 3-bromoimidazo[1,2-a]pyrazine (B), electron density is withdrawn from the negative belt of the halogen toward the opposite direction of the σ-hole. Consequently, a much larger positive electrostatic potential representing a significantly tuned σ-hole with reduced directionality is characteristic for the halogen bonding interface of (B). In case of 5,6-dichloropyrimidin-4-amine (C) the same molecule offers one classical XB interface with addressable electron density around the halogen atom and one significantly tuned halogen bonding interface. Despite significant differences in the chemotype, the XB interface of (A) and the classical XB interface of (C) share some obvious similarities.
Characterization of the Herein Selected Showcase HEFLib
To avoid a selection bias due to molecular weight restrictions, no hard limit of molecular weight was included in the process of fragment selection from vendor libraries. Weight and size deviate strongly for the heavy halogens, bromine (equivalent weight to 6.7 carbon atoms), and iodine (equivalent weight to 10.6 carbon atoms). Thus, halogen-enriched fragments are expected to have a larger molecular weight than standard fragments. Nevertheless, only two fragments violated the rule of three in terms of molecular weight. Thirty one fragments contain more than three hydrogen bond acceptors. Two compounds have more than three rotatable bonds and 56 have a larger polar surface area (PSA) than 60 Å2 (see Figure 4). Both parameters, rotatable bonds and polar surface area, can give a hint for oral availability. High values of these parameters might go hand in hand with high solubility, which we aimed for. Interestingly, linear models were found that show a negative correlation of solubility with topological polar surface area, i.e., with decreasing TPSA aqueous solubility increases (Ali et al., 2012). Iteratively refined values for optimal polar surface area of fragments are described by Ray et al. (2017). Applying their limitation of up to 90 Å2 for the PSA, only 9 fragments of the generated HEFLib violate this guideline. Furthermore, they increased the number of favorable hydrogen bond acceptors to 6, which includes all fragments of the generated HEFLib.
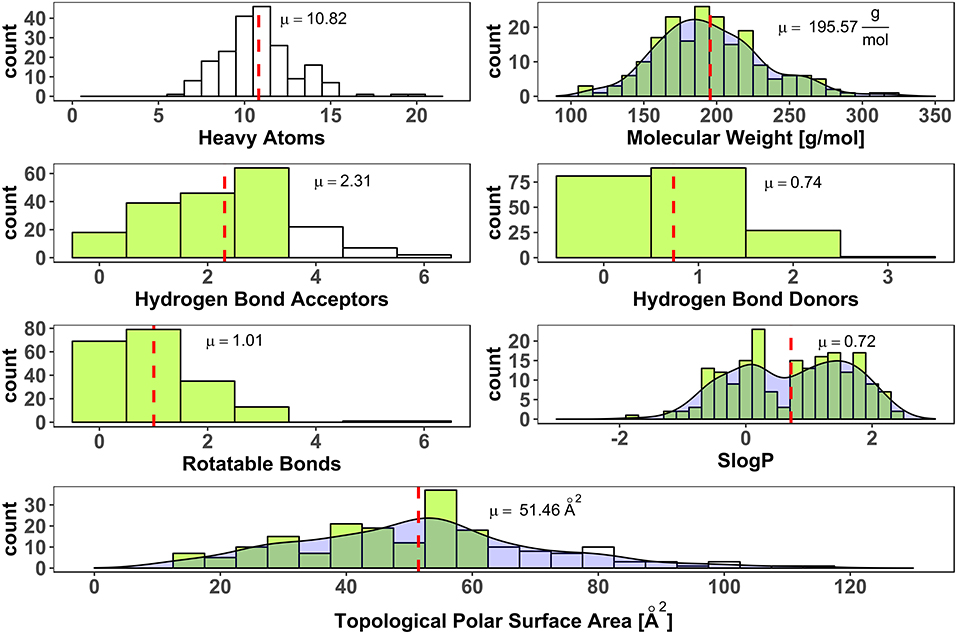
Figure 4. Distribution of number of heavy atoms (RDKit), molecular weight (Canvas Molecular Descriptors, Schrödinger), number of hydrogen bond acceptors (CDK), number of hydrogen bond donors (CDK), number of rotatable bonds (CDK, non-terminal), SlogP as cLogP (RDKit) and Topological Polar Surface Area (Ertl et al., 2000) (RDKit). Dashed red lines indicate mean values (μ). Green bars indicate bins that fulfill rule of three (Congreve et al., 2003). Density shown for continuous data.
Turbidimetric Solubility Assessment
Depending on the criterion of absorption/extinction threshold for the detection of insoluble compounds, six to nine compounds out of 96 tested fragments showed turbidity in the assay at the highest concentration of 5 mM (Figure S2). Nevertheless, all tested compounds were soluble at the lowest tested concentration of 1.67 mM, except for one compound that was not completely soluble at 100 mM in DMSO. In this case the actual concentration of the dilution series in DMSO remains unclear. It needs to be mentioned, that our assay format is much more sensitive to intrinsic absorption of the compounds than the classical nephelometric turbidity assay. For all measured compounds an absorption spectrum was recorded at a concentration of 1.25 mM. At this concentration, all compounds were soluble. Within the range of 600–800 nm, no significant absorption maximum was observed.
Three-Dimensionality
Another important factor for the fragment library design is defined by three-dimensionality (Hung et al., 2011; Bower et al., 2016). Especially when looking at successful leads, derived from fragments, a clear trend toward larger deviation from planarity can be observed (Johnson et al., 2018). Different descriptors exist, that describe the non-flatness of a molecule, like fraction of sp3 hybridized atoms (Yang et al., 2012), deviation of plane of best fit (Hall et al., 2014) and principle moments of inertia (Sauer and Schwarz, 2003; Aldeghi et al., 2013). Due to the enrichment of aromatic, sp2 hybridized, ring systems with halogens, HEFLibs are expected to be relatively flat or rod-like. As expected, the generated library contains a large fraction of molecules that is planar to rod-like with a mean of Fsp3 of 0.06 (see Figure 5).
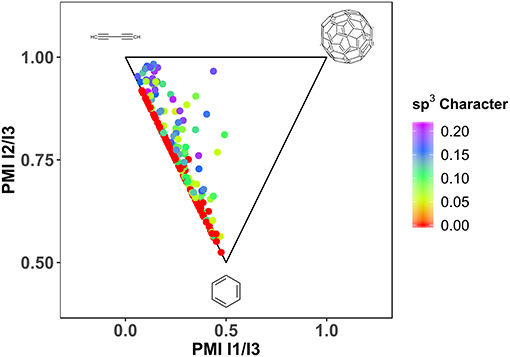
Figure 5. Shape distribution of HEFLib with n = 198. Geometry optimization by LigPrep (Schrödinger Release., 2018) (Schrödinger) and OPLS2005. sp3 character calculated with CDK (Steinbeck et al., 2003, 2006; Willighagen et al., 2017). PMI (Sauer and Schwarz, 2003) calculation with Vernalis Nodes for KNIME.
Similarity of Electrostatic Potential
Especially the influence of minor changes in structural topology to the halogen bonding interface, defined as the proximal environment of the σ-hole donor, is commonly underestimated. The explorative character of the library with respect to the binding motif (XB interface) is exemplified in Figure 6, where two constitutional isomers that are part of the novel showcase HEFLib are depicted. They only differ in the position of nitrogen atom 5 or 7. In case of the imidazo[1,2-b]pyridazine scaffold (Figure 6B) the lone pair of this nitrogen atom is pointing toward the same direction as the bromine atom and, thus, reduces the extremely tuned magnitude of the σ-hole (Vmax) of the bromine atom in comparison to 3-bromoimidazo[1,2-a]pyrazine (Figure 6A).
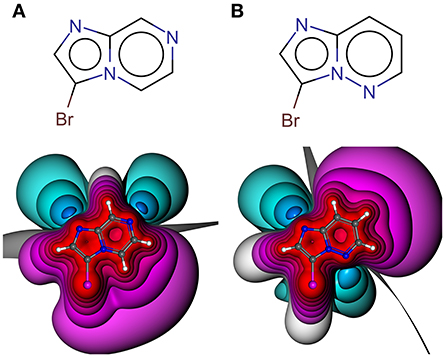
Figure 6. Depicted structures with high structural similarity, but significantly different XB interface: (A) 3-bromoimidazo[1,2-a]pyrazine and (B) 3-bromoimidazo[1,2-b]pyridazine. Electrostatic potential plot colored from −0.34 au (blue) via zero (white) to +0.34 au (red). Magnitude: Vmax, A = 0.040 au, Vmax, B = 0.028 au. Deviation of point of Vmax from C-X bond vector linearity: Φ(Vmax, A) = 2.0°, Φ(Vmax, B) = 4.6°.
The strong correlation of Vmax and the potential adduct formation energy (Politzer et al., 2013; Heidrich et al., 2018; Lange et al., 2019) and its demonstrated usefulness as descriptor for the magnitude of the σ-hole, is the basis for our choice of Vmax as an important parameter for our diversity assessment, which is centered on the XB interface. As an indicator for the diversity of our showcase HEFLib, we use the distribution of calculated Vmax values at the classical definition of the electron isodensity level of 0.001 au while including all possible tautomeric states with a net charge of zero. It needs to be mentioned that several of these states, generated by LigPrep/EPIK (Schrödinger Suite), might contribute with a very low probability to the overall distribution of states. In comparison to Vmax values of chlorobenzene (0.008 au), bromobenzene (0.016 au), and iodobenzene (0.025 au) all median values and also the corresponding first quartile of each halogen type are above the reference (see Figure 7). Remarkably, the range of Vmax values for the chlorine fragments is larger than that of iodine fragments. Given the higher tunability of iodine in comparison to bromine or chlorine, it should be noted that the spectrum of σ-hole magnitude for iodine compounds in the library was limited by the restrictive cost filter of $5/mg compound.
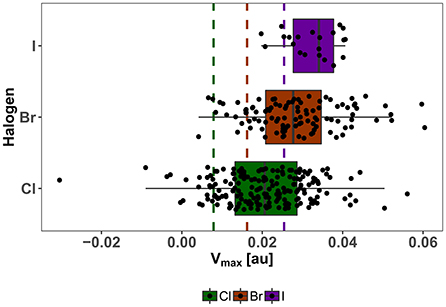
Figure 7. Distribution of Vmax of neutral isomers in the showcase HEFLib. Color encodes the type of aromatic halogen: Green for chlorine, brown for bromine, and purple for iodine. Same color code is used for reference Vmax values (dashed lines) of chloro-, bromo-, and iodobenzene. Extreme values are found for zwitterionic fragments.
By evaluating our herein demonstrated showcase library with different target proteins, first hits featuring halogen bonding were successfully identified.
Conclusion
We aimed for a design strategy with particular focus on the XB interface diversity of halogen-enriched fragments as well as an initial showcase HEFLib with a broad spectrum of targetable features in close proximity to the halogen that can form specific interactions with various elements of the binding site. Parallel to the concept of “privileged structures” (Duarte et al., 2007) that contain binding motifs for targeting specific binding sites, e.g., GPCRs or kinases, our concept brings into focus the molecular interactions of halogen atoms and their proximal environment. By introducing structural diversity into halogenated fragments, exerting significant influences on the predicted Vmax, we obtain a variety of XB interfaces that are able to address a broad spectrum of targets with halogen bonding as key interaction motif.
We have demonstrated that in our generated HEFLib, a diverse halogen bonding interface is also reflected by diverse σ-hole magnitudes of the fragments. We admit that chemical space coverage of our initial showcase HEFLib, especially with regard to three-dimensionality and iodine content, was significantly lowered by limited financial resources in an academic environment. Our library is available for other working groups and can be seen as starting point for further expansion, which is a well-known method of the iterative process of library design and screening (Brewer et al., 2008). Next evolutionary steps of the library are focused on three-dimensionality and even higher tuning values of Vmax. Since approximation of Vmax values can be efficiently performed using VmaxPred (Heidrich et al., 2018), we plan to enrich fragments with greater σ-hole magnitudes by custom synthesis using carefully selected building blocks. Based on an in silico synthesis approach, vast numbers of potential halogenated fragments can be efficiently proposed and used as a starting point for a selection process as outlined herein. This strategy will significantly increase the probability of establishing stronger halogen bonds as key interactions in early hit finding phases of academic and industrial drug discovery.
The implemented feature tree-like diversity measure can be used for the diversity assessment of halogenated fragments in other projects and is available for other working groups.
Author Contributions
FB initiated the research. JH implemented similarity measure and performed library filtering. LS and JH performed and evaluated turbidimetric solubility assay. FB and JH prepared the manuscript.
Conflict of Interest Statement
The authors declare that the research was conducted in the absence of any commercial or financial relationships that could be construed as a potential conflict of interest.
Acknowledgments
We acknowledge support by the state of Baden-Württemberg through bwHPC and support by Deutsche Forschungsgemeinschaft and Open Access Publishing Fund of University of Tübingen.
Supplementary Material
The Supplementary Material for this article can be found online at: https://www.frontiersin.org/articles/10.3389/fchem.2019.00009/full#supplementary-material
References
Aldeghi, M., Malhotra, S., Selwood, D. L., and Chan, A. W. (2013). Two- and three-dimensional rings in drugs. Chem. Biol. Drug Des. 83, 450–461. doi: 10.1111/cbdd.12260
Ali, J., Camilleri, P., Brown, M. B., Hutt, A. J., and Kirton, S. B. (2012). Revisiting the general solubility equation: in silico prediction of aqueous solubility incorporating the effect of topographical polar surface area. J. Chem. Inform. Model. 52, 420–428. doi: 10.1021/ci200387c
Ashton, M., Barnard, J., Casset, F., Charlton, M., Downs, G., Gorse, D., et al. (2002). Identification of diverse database subsets using property-based and fragment-based molecular descriptions. Quan. Struct. Activity Relationships 21, 598–604. doi: 10.1002/qsar.200290002
Backus, K. M., Correia, B. E., Lum, K. M., Forli, S., Horning, B. D., González-Páez, G. E., et al. (2016). Proteome-wide covalent ligand discovery in native biological systems. Nature 534, 570–574. doi: 10.1038/nature18002
Bader, R. F. W., Carroll, M. T., Cheeseman, J. R., and Chang, C. (1987). Properties of atoms in molecules: atomic volumes. J. Am. Chem. Soc. 109, 7968–7979. doi: 10.1021/ja00260a006
Berthold, M. R., Cebron, N., Dill, F., Gabriel, T. R., Kötter, T., Meinl, T., et al. (2007). “KNIME: the konstanz information miner,” in Studies in Classification, Data Analysis, and Knowledge Organization (GfKL 2007), eds C. Preisach, H. Burkhardt, L. Schmidt-Thieme, and R. Decker (Heidelberg: Springer), 319–326.
Bohacek, R. S., McMartin, C., and Guida, W. C. (1996). The art and practice of structure-based drug design: a molecular modeling perspective. Med. Res. Rev. 16, 3–50. doi: 10.1002/(SICI)1098-1128(199601)16:1<3::AID-MED1>3.0.CO;2-6
Bonchev, D., Peev, T., and Russeva, B. (1976). Information Study of Atomic Nuclei. Information for Proton-Neutron Composition. MATCH Communications in Mathematical and in Computer Chemistry, No. 2, 123–137.
Bower, J., Pugliese, A., and Drysdale, M. (2016). “Strategies for fragment library design,” in Fragment-Based Drug Discovery Lessons and Outlook, eds D. A. Erlanson and W. Jahnke (Weinheim: Wiley-VCH Verlag GmbH & Co. KGaA), 99–118.
Boyd, S. M., Turnbull, A. P., and Walse, B. (2012). Fragment library design considerations. Wiley Interdisciplin. Rev. Comput. Mol. Sci. 2, 868–885. doi: 10.1002/wcms.1098
Brewer, M., Ichihara, O., Kirchhoff, C., Schade, M., and Whittaker, M. (2008). “Assembling a fragment library,” in Fragment-Based Drug Discovery: A Practical Approach, eds E. R. Zartler and M. J. Shapiro (Chichester; West Sussex: Wiley-Blackwell), 39–62.
Carlsson, A. C., Scholfield, M. R., Rowe, R. K., Ford, M. C., Alexander, A. T., Mehl, R. A., et al. (2018). Increasing enzyme stability and activity through hydrogen bond-enhanced halogen bonds. Biochemistry 57, 4135–4147. doi: 10.1021/acs.biochem.8b00603
Cash, G. G. (1995). Correlation of physicochemical properties of alkylphenols with their graph-theoretical ϵ parameter. Chemosphere 31, 4307–4315. doi: 10.1016/0045-6535(95)00295-J
Chemical Computing Group ULC (2018). Molecular Operating Environment (MOE) (Version 2013.08). Montreal, QC: Chemical Computing Group ULC. Available online at: https://www.chemcomp.com
Cheng, Y. Y., and Yuan, H. (2006). Quantitative study of electrostatic and steric effects on physicochemical property and biological activity. J. Mol. Graph. Model. 24, 219–226. doi: 10.1016/j.jmgm.2005.08.005
Chessari, G., and Woodhead, A. J. (2009). From fragment to clinical candidate—a historical perspective. Drug Discov. Today 14, 668–675. doi: 10.1016/j.drudis.2009.04.007
Clark, T., Hennemann, M., Murray, J. S., and Politzer, P. (2007). Halogen bonding: the σ-hole. J. Mol. Model. 13, 291–296. doi: 10.1007/s00894-006-0130-2
Congreve, M., Carr, R., Murray, C., and Jhoti, H. (2003). A ‘rule of three’ for fragment-based lead discovery? Drug Discov. Today 8, 876–877. doi: 10.1016/S1359-6446(03)02831-9
Craven, G. B., Affron, D. P., Allen, C. E., Matthies, S., Greener, J. G., Morgan, R. M. L., et al. (2018). High-throughput kinetic analysis for target-directed covalent ligand discovery. Angew. Chem. Int. Ed. 57, 5257–5261. doi: 10.1002/anie.201711825
Delaney, J. S. (2004). ESOL: estimating aqueous solubility directly from molecular structure. J. Chem. Inform. Comput. Sci. 44, 1000–1005. doi: 10.1021/ci034243x
Domagała, M., Lutynska, A., and Palusiak, M. (2018). Extremely strong halogen bond. The case of a double-charge-assisted halogen bridge. J. Phys. Chem. A 122, 5484–5492. doi: 10.1021/acs.jpca.8b03735
Duarte, C. D., Barreiro, E. J., and Fraga, C. A. (2007). Privileged structures: a useful concept for the rational design of new lead drug candidates. Mini Rev. Med. Chem. 7, 1108–1119. doi: 10.2174/138955707782331722
Erlanson, D. A. (2011). “Introduction to fragment-based drug discovery,” in Fragment-Based Drug Discovery and X-Ray Crystallography. Topics in Current Chemistry, Vol. 317, eds T. Davies and M. Hyvönen (Berlin; Heidelberg: Springer).
Ertl, P., Rohde, B., and Selzer, P. (2000). Fast calculation of molecular polar surface area as a sum of fragment-based contributions and its application to the prediction of drug transport properties. J. Med. Chem. 43, 3714–3717. doi: 10.1021/jm000942e
Eskandari, K., and Lesani, M. (2015). Does fluorine participate in halogen bonding? Chem. Eur. J. 21, 4739–4746. doi: 10.1002/chem.201405054
Estrada, E. (1995). Edge adjacency relationships and a novel topological index related to molecular volume. J. Chem. Inform. Comput. Sci. 35, 31–33. doi: 10.1021/ci00023a004
Hall, L. H., and Kier, L. B. (2007). “The molecular connectivity chi indexes and kappa shape indexes in structure-property modeling,” in Reviews in Computational Chemistry, eds K. B. Lipkowitz and D. B. Boyd (New York, NY: Wiley). doi: 10.1002/9780470125793.ch9
Hall, R. J., Mortenson, P. N., and Murray, C. W. (2014). Efficient exploration of chemical space by fragment-based screening. Prog. Biophys. Mol. Biol. 116, 82–91. doi: 10.1016/j.pbiomolbio.2014.09.007
Hann, M. M. (2011). Molecular obesity, potency and other addictions in drug discovery. MedChemComm 2, 349–355. doi: 10.1039/C1MD00017A
Hann, M. M., Leach, A. R., and Harper, G. (2001). Molecular complexity and its impact on the probability of finding leads for drug discovery. J. Chem. Inform. Comput. Sci. 41, 856–864. doi: 10.1021/ci000403i
Heidrich, J., Exner, T., and Boeckler, F. M. (2018). Predicting the magnitude of σ-holes using VmaxPred, a fast and efficient tool supporting the application of halogen bonds in drug discovery. J. Chem. Inform. Model. doi: 10.1021/acs.jcim.8b00622
Hung, A. W., Ramek, A., Wang, Y., Kaya, T., Wilson, J. A., Clemons, P. A., et al. (2011). Route to three-dimensional fragments using diversity-oriented synthesis. Proc. Nat. Acad. Sci. U.S.A. 108, 6799–6804. doi: 10.1073/pnas.1015271108
Johnson, C. N., Erlanson, D. A., Jahnke, W., Mortenson, P. N., and Rees, D. C. (2018). Fragment-to-lead medicinal chemistry publications in 2016. J. Med. Chem. 61, 1774–1784. doi: 10.1021/acs.jmedchem.7b01298
Joseph-McCarthy, D., Campbell, A. J., Kern, G., and Moustakas, D. (2014). Fragment-based lead discovery and design. J. Chem. Inform. Model. 54, 693–704. doi: 10.1021/ci400731w
Kathman, S. G., and Statsyuk, A. V. (2016). Covalent tethering of fragments for covalent probe discovery. MedChemComm 7, 576–585. doi: 10.1039/c5md00518c
Keserü, G. M., and Makara, G. M. (2009). The influence of lead discovery strategies on the properties of drug candidates. Nat. Rev. Drug Discov. 8, 203–212. doi: 10.1038/nrd2796
Kier, L. B. (1989). An index of molecular flexibility from kappa shape attributes. Quan. Struct. Activity Relationships 8, 221–224. doi: 10.1002/qsar.19890080307
Kolár, M. H., and Hobza, P. (2016). Computer modeling of halogen bonds and other σ-hole interactions. Chem. Rev. 116, 5155–5187. doi: 10.1021/acs.chemrev.5b00560
Landrum, G. (2006). RDKit: Open-Source Cheminformatics. RDKit: Open-Source Cheminformatics Software. Available online at: http://www.rdkit.org/
Lange, A., Heidrich, J., Zimmermann, M. O., Exner, T., and Boeckler, F. M. (2019). Scaffold effects on halogen bonding strength. J. Chem. Inform. Model. doi: 10.1021/acs.jcim.8b00621. [Epub ahead of print].
Lipinski, C. A., Lombardo, F., Dominy, B. W, and Feeney, P. J. (2001). Experimental and computational approaches to estimate solubility and permeability in drug discovery and development settings. Adv. Drug Deliv. Rev. 46, 3–26. doi: 10.1016/S0169-409X(00)00129-0
Metrangolo, P., Murray, J. S., Pilati, T., Politzer, P., Resnati, G., and Terraneo, G. (2011). Fluorine-centered halogen bonding: a factor in recognition phenomena and reactivity. Cryst. Growth Des. 11, 4238–4246. doi: 10.1021/cg200888n
Metrangolo, P., Neukirch, H., Pilati, T., and Resnati, G. (2005). Halogen bonding based recognition processes: a world parallel to hydrogen bonding. Accounts Chem. Res. 38, 386–395. doi: 10.1021/ar0400995
Murray, J. S., and Politzer, P. (1991). Correlations between the solvent hydrogen-bond-donating parameter ɑ and the calculated molecular surface electrostatic potential. J. Organ. Chem. 56, 6715–6717. doi: 10.1021/jo00023a045
Murry, J. S., and Politzer, P. (2011). The electrostatic potential: an overview. Wiley Interdisciplin. Rev. Comput. Mol. Sci. 1, 153–163. doi: 10.1002/wcms.19
Politzer, P., Murray, J. S., and Clark, T. (2010). Halogen bonding: an electrostatically-driven highly directional noncovalent interaction. Phys. Chem. Chem. Phys. 12, 7748–7757. doi: 10.1039/C004189K
Politzer, P., Murray, J. S., and Clark, T. (2013). Halogen bonding and other σ-hole interactions: a perspective. Phys. Chem. Chem. Phys. 15, 11178–11189. doi: 10.1039/C3CP00054K
Rarey, M., and Dixon, J. S. (1998). Feature trees: a new molecular similarity measure based on tree matching. J. Comput. Aided Mol. Des. 12, 471–490.
Ray, P. C., Kiczun, M., Huggett, M., Lim, A., Prati, F., Gilbert, I. H., et al. (2017). Fragment library design, synthesis and expansion: nurturing a synthesis and training platform. Drug Discov. Today 22, 43–56. doi: 10.1016/j.drudis.2016.10.005
Rezáč, J., and de la Lande, A. (2016). On the role of charge transfer in halogen bonding. Phys. Chem. Chem. Phys. 19, 791–803. doi: 10.1039/C6CP07475Hs
Riley, K. E., Murray, J. S., Fanfrlík, J., Rezáč, J., Solá, R. J., Concha, M. C., et al. (2011). Halogen bond tunability I: the effects of aromatic fluorine substitution on the strengths of halogen-bonding interactions involving chlorine, bromine, and iodine. J. Mol. Model. 17, 3309–3318. doi: 10.1007/s00894-011-1015-6
Riley, K. E., Murray, J. S., Fanfrlík, J., Rezáč, J., Solá, R. J., Concha, M. C., et al. (2013). Halogen bond tunability II: the varying roles of electrostatic and dispersion contributions to attraction in halogen bonds. J. Mol. Model. 19, 4651–4659. doi: 10.1007/s00894-012-1428-x
Ruddigkeit, L., van Deursen, R., Blum, L. C., and Reymond, J. L. (2012). Enumeration of 166 billion organic small molecules in the chemical universe database GDB-17. J. Chem. Inform. Model. 52, 2864–2875. doi: 10.1021/ci300415d
Sauer, W. H., and Schwarz, M. K. (2003). Molecular shape diversity of combinatorial libraries: a prerequisite for broad bioactivity. J. Chem. Inform. Comput. Sci. 43, 987–1003. doi: 10.1021/ci025599w
Scholfield, M. R., Zanden, C. M., Carter, M., and Ho, P. S. (2013). Halogen bonding (X-bonding): a biological perspective. Protein Sci. Publ. Protein Soc. 22, 139–152. doi: 10.1002/pro.2201
Schrödinger Release. (2018). Schrödinger Release 2018-1: LigPrep. New York, NY: LLC. Available online at: https://www.schrodinger.com
Schultes, S., de Graaf, C., Haaksma, E. E. J., de Esch, I. J. P., Leurs, R., and Krämer, O. (2010). Ligand efficiency as a guide in fragment hit selection and optimization. Drug Discov. Today Technol. 7, e157–e162. doi: 10.1016/j.ddtec.2010.11.003
Sedlak, R., Kolár, M. H., and Hobza, P. (2015). Polar flattening and the strength of halogen bonding. J. Chem. Theory Comput. 11, 4727–4732. doi: 10.1021/acs.jctc.5b00687
Sirimulla, S., Bailey, J. B., Vegesna, R., and Narayan, M. (2013). Halogen interactions in protein-ligand complexes: implications of halogen bonding for rational drug design. J. Chem. Inform. Model. 53, 2781–2791. doi: 10.1021/ci400257k
Steinbeck, C., Han, Y., Kuhn, S., Horlacher, O., Luttmann, E., and Willighagen, E. (2003). The Chemistry Development Kit (CDK): an open-source java library for chemo- and bioinformatics. J. Chem. Inform. Comput. Sci. 43, 493–500. doi: 10.1021/ci025584y
Steinbeck, C., Hoppe, C., Kuhn, S., Floris, M., Guha, R., and Willighagen, E. L. (2006). Recent developments of the Chemistry Development Kit (CDK)—an open-source java library for chemo- and bioinformatics. Curr. Pharmaceut. Des. 12, 2111–2120. doi: 10.2174/138161206777585274
Tetko, I. V., and Tanchuk, V. Y. (2002). Application of associative neural networks for prediction of lipophilicity in ALOGPS 2.1 program. J. Chem. Inform. Comput. Sci. 42, 1136–1145. doi: 10.1021/ci025515j
Tetko, I. V., Tanchuk, V. Y., Kasheva, T. N., and Villa, A. E. (2001). Estimation of aqueous solubility of chemical compounds using E-state indices. J. Chem. Inform. Comput. Sci. 41, 1488–1493. doi: 10.1021/ci000392t
Todeschini, R., and Consonni, V. (2009). Molecular Descriptors for Chemoinformatics: Volume I: Alphabetical Listing/Volume II: Appendices, References. Methods and Principles in Medicinal Chemistry. Weinheim: Wiley-VCH Verlag GmbH & Co. KGaA.
Trapero, A., Pacitto, A., Singh, V., Sabbah, M., Coyne, A. G., Mizrahi, V., et al. (2018). Fragment-based approach to targeting Inosine-5′-monophosphate dehydrogenase (IMPDH) from Mycobacterium tuberculosis. J. Med. Chem. 61, 2806–2822. doi: 10.1021/acs.jmedchem.7b01622
U.S. Environmental Protection Agency (2017). WSKOWIN (Version 4.11). EPI Suite. U.S. Environmental Protection Agency.
Wilcken, R., Liu, X., Zimmermann, M. O., Rutherford, T. J., Fersht, A. R., Joerger, A. C., et al. (2012). Halogen-enriched fragment libraries as leads for drug rescue of mutant P53. J. Am. Chem. Soc. 134, 6810–6818. doi: 10.1021/ja301056a
Wilcken, R., Zimmermann, M. O., Lange, A., Joerger, A. C., and Boeckler, F. M. (2013). Principles and applications of halogen bonding in medicinal chemistry and chemical biology. J. Med. Chem. 56, 1363–1388. doi: 10.1021/jm3012068
Willighagen, E. L., Mayfield, J. W., Alvarsson, J., Berg, A., Carlsson, L., Jeliazkova, N., et al. (2017). The Chemistry Development Kit (CDK) v2.0: atom typing, depiction, molecular formulas, and substructure searching. J. Cheminformatics 9:33. doi: 10.1186/s13321-017-0220-4
Xing, L., Klug-Mcleod, J., Rai, B., and Lunney, E. A. (2015). Kinase hinge binding scaffolds and their hydrogen bond patterns. Bioorgan. Med. Chem. 23, 6520–6527. doi: 10.1016/j.bmc.2015.08.006
Yang, Y., Engkvist, O., Llinàs, A., and Chen, H. (2012). Beyond size, ionization state, and lipophilicity: influence of molecular topology on absorption, distribution, metabolism, excretion, and toxicity for druglike compounds. J. Med. Chem. 55, 3667–3677. doi: 10.1021/jm201548z
Zhou, C., Nie, C., Li, S., and Li, Z. (2007). A novel semi-empirical topological descriptor Nt and the application to study on QSPR/QSAR. J. Comput. Chem. 28, 2413–2423. doi: 10.1002/jcc.20540
Keywords: fragment, library, HEFLib, design, diversity, Vmax
Citation: Heidrich J, Sperl LE and Boeckler FM (2019) Embracing the Diversity of Halogen Bonding Motifs in Fragment-Based Drug Discovery—Construction of a Diversity-Optimized Halogen-Enriched Fragment Library. Front. Chem. 7:9. doi: 10.3389/fchem.2019.00009
Received: 27 September 2018; Accepted: 07 January 2019;
Published: 18 February 2019.
Edited by:
Jamie Platts, Cardiff University, United KingdomReviewed by:
Gabriella Cavallo, Politecnico di Milano, ItalyAndrzej Jacek Bojarski, Polish Academy of Sciences, Poland
Copyright © 2019 Heidrich, Sperl and Boeckler. This is an open-access article distributed under the terms of the Creative Commons Attribution License (CC BY). The use, distribution or reproduction in other forums is permitted, provided the original author(s) and the copyright owner(s) are credited and that the original publication in this journal is cited, in accordance with accepted academic practice. No use, distribution or reproduction is permitted which does not comply with these terms.
*Correspondence: Frank M. Boeckler, frank.boeckler@uni-tuebingen.de
†Present Address: Laura E. Sperl, Structural Membrane Biochemistry, Bavarian NMR Center at the Department of Chemistry and Institute for Advanced Study Technical University of Munich, Garching, Germany