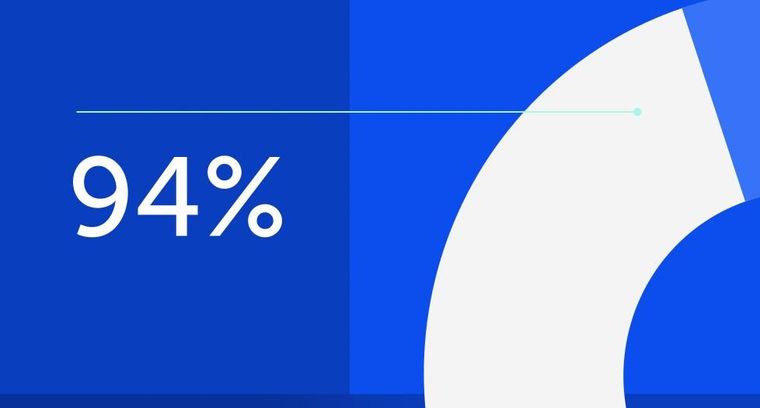
94% of researchers rate our articles as excellent or good
Learn more about the work of our research integrity team to safeguard the quality of each article we publish.
Find out more
MINI REVIEW article
Front. Cell Dev. Biol., 22 January 2020
Sec. Signaling
Volume 7 - 2019 | https://doi.org/10.3389/fcell.2019.00395
This article is part of the Research TopicPerspectives in Mammary Gland Development and Breast Cancer ResearchView all 13 articles
Breast cancer incidence is increasing worldwide with more than 600,000 deaths reported in 2018 alone. In current practice treatment options for breast cancer patients consists of surgery, chemotherapy, radiotherapy or targeting of classical markers of breast cancer subtype: estrogen receptor (ER) and HER2. However, these treatments fail to prevent recurrence and metastasis. Improved understanding of breast cancer and metastasis biology will help uncover novel biomarkers and therapeutic opportunities to improve patient stratification and treatment. We will first provide an overview of current methods and models used to study breast cancer biology, focusing on 2D and 3D cell culture, including organoids, and on in vivo models such as the MMTV mouse model and patient-derived xenografts (PDX). Next, genomic, transcriptomic, and proteomic approaches and their integration will be considered in the context of breast cancer susceptibility, breast cancer drivers, and therapeutic response and resistance to treatment. Finally, we will discuss how ‘Omics datasets in combination with traditional breast cancer models are useful for generating insights into breast cancer biology, for suggesting individual treatments in precision oncology, and for creating data repositories to undergo further meta-analysis. System biology has the potential to catalyze the next great leap forward in treatment options for breast cancer patients.
Breast cancer is the leading cause of cancer-related deaths in women worldwide (Bray et al., 2018). It is a heterogeneous disease (Nik-Zainal et al., 2016), commonly separated into Luminal A (LumA), Luminal B (LumB), epidermal growth factor receptor ERBB2/HER2-overexpressing (HER2+), basal epithelial-like (BL) based on gene expression profiles (Sørlie et al., 2001). Breast cancer is currently treated with surgery, radiotherapy, cytotoxic chemotherapy and/or targeted therapies to eradicate viable cancer cells (Fisher et al., 2002).
LumA and LumB breast cancers are both estrogen receptor (ER)-positive (Sørlie et al., 2001). Deregulated ER signaling is associated with cancer hallmarks (Hanahan and Weinberg, 2011). For instance, ER target genes like cyclin-dependent kinase (CDK) 1 or the kinase Src promote cell proliferation, invasion and epithelial–mesenchymal transition (EMT) (Stender et al., 2007; Saha Roy and Vadlamudi, 2012). LumB cancers have high expression of the proliferation marker Ki67, which correlates with increased risk of developing distant metastases (Colzani et al., 2014), and reduced expression of the progesterone receptor (PR) (Cho, 2016), which shifts gene expression toward more tumorigenic genes (Mohammed et al., 2015). LumA and LumB tumors are treated using ER antagonists (e.g., tamoxifen), aromatase inhibitors and selective estrogen receptor degraders (e.g., fulvestrant). However, therapeutic resistance may arise through loss of ER expression, mutations in ER or overexpression of alternative breast cancer-driving pathways such as ERBB1/EGFR (Garcia-Becerra et al., 2012; Clarke et al., 2015; Ma et al., 2015). To overcome resistance to traditional ER antagonists targeted therapies against phosphoinositide 3-kinases (PI3K), mammalian target of rapamycin (mTOR), and CDK4/6 have recently been proven beneficial in the clinical setting (Beaver and Park, 2012; Kornblum et al., 2018; Pernas et al., 2018).
HER2 + breast cancers overexpress ERBB2/HER2 (Iqbal and Iqbal, 2014) which promotes proliferation by regulating CDKs and Cyclins (Timms et al., 2002). Additionally, HER2 dimerization with EGFR induces activation of mitogen-activated protein kinase (MAPK), c-Jun N-terminal kinases (JNK), and phosphoinositide phospholipase C (PLCγ) signaling pathways resulting in increased cell proliferation, migration and apoptosis resistance (Masuda et al., 2012). HER2 + breast cancers are treated with targeted agents such as trastuzumab, pertuzumab, and neratinib. Trastuzumab is an antibody which inhibits HER2 dimerization, promotes natural killer cell recruitment to tumors and stimulates ubiquitin-dependent HER2 degradation (Vu and Claret, 2012; McCann and Hurvitz, 2018; Schmid et al., 2018; Vikas et al., 2018). Therapeutic resistance to trastuzumab occurs via HER2 dimerization with other ERBB family members or constitutive HER2 activation (Vu and Claret, 2012).
BL breast cancers do not generally express ER, PR or HER2 (Milioli et al., 2017), like triple negative breast cancers (TNBCs) (Lehmann et al., 2016). BLs are highly heterogeneous and include basal-like1-2, claudin-low, and immunomodulatory subgroups (Garrido-Castro et al., 2019). BLs have a highly proliferative and invasive phenotype with high risk of relapse in early breast cancer (Fallahpour et al., 2017). BLs are typically treated by chemotherapy and radiotherapy (Wahba and El-Hadaad, 2015) although recent advances have led to novel treatment opportunities for BL cancer patients. For instance, immunomodulatory BLs can be treated with immune checkpoint programed cell death protein 1 (PD-1) and poly (ADP-ribose) polymerase (PARP) inhibitors (McCann and Hurvitz, 2018; Schmid et al., 2018; Vikas et al., 2018).
Two major challenges in breast cancer treatment are therapeutic resistance and the formation of metastasis to secondary sites (lung, bone, lymph nodes, brain, and liver) inevitably leading to patient mortality (Minn et al., 2005). As 10 year survival for metastatic breast cancer patients remains below 5% (Kontani et al., 2014) and response to targeted therapies varies from 15 to 40% for all subtypes (Bartsch et al., 2007; Haque and Desai, 2019) the need for novel therapeutic options for breast cancer patients remains a priority.
Here, we will describe several models that have contributed to knowledge of breast cancer biology and the repertoire of currently available therapeutic targets. Thereafter, we will introduce system biology-based approaches and finally discuss how their integration with traditional models is revolutionizing breast cancer translational research.
Breast cancer has been traditionally studied using immortalized cell lines derived from patient samples (Holliday and Speirs, 2011) which are easy and inexpensive to grow. These cell lines express biomarkers of the different molecular subtypes of breast cancer (Dai et al., 2017) and recapitulate some parent tumor characteristics including drug responses (Holliday and Speirs, 2011) and transcriptomic profiles (Neve et al., 2006). Cell lines have enabled major discoveries in breast cancer research, such as the identification of oncogenes (Elenbaas et al., 2001) and drivers of metastatic tropism (Minn et al., 2005). However, breast cancer cell lines have increased gene copy number variations compared to primary tumors (Larramendy et al., 2000), lack the in vivo microenvironment (Vincent et al., 2015), and do not maintain primary tumor heterogeneity (Dai et al., 2017; Liu et al., 2019) (Figure 1A).
Figure 1. Models and methods to study breast cancer. Summary of the advantages (left column) and disadvantages (middle column) of existing breast cancer models (A–D) and ‘omics technologies (E) to study breast cancer. Right column reports a brief summary of how different methods and models have contributed to major discoveries in the field of breast cancer.
Organoids are three dimensional (3D) cell cultures which mimic healthy tissues and cancer lesions (Xu et al., 2018). Organoids are usually grown in matrices such as MatrigelTM, collagen or peptide hydrogels which aim to recapitulate the breast microenvironment (Djomehri et al., 2019). The group of Mina Bissel in the ‘80s began to investigate how organoids were a better model for studying breast tissue compared to 2D cell culture (Weaver et al., 1995). More recently, primary and metastatic organoids have been developed which accurately recapitulate parent tumor characteristics including histopathology, genomic abnormalities and drug responses (Sachs et al., 2018). Organoids are easy to modify, can be propagated for up to 3 months (Fatehullah et al., 2016), and allow drug screening (Dutta et al., 2017). Recently, the issue of availability of primary patient samples for laboratories without access to biobanks has been solved by the creation of living biobanks of frozen organoids (Dutta et al., 2017). Organoids can be used as models to study different breast cancer subtypes and to identify potential novel therapeutic targets. Organoid are better models than 2D cultures to analyze drug response due to a more representative microenvironment and selection for stem-like cells, like those responsible for metastatic initiation (Velasco-Velazquez et al., 2011; Imamura et al., 2015). Despite these promising characteristics for breast cancer translational research, organoids lack components of the in vivo microenvironment and may suffer for counterselection of hyperproliferative cells (Fujii et al., 2016; Weeber et al., 2017) (Figure 1B).
In vivo modeling of breast cancers generally entails inducing oncogene expression (e.g., Erbb2) or knocking out a tumor suppressor gene (e.g., p53) in mice. Examples include the mouse mammary tumor virus (MMTV) promoter-driven or the 4T1-based SMMs (Holen et al., 2017). GEMMs include a natural (mouse) microenvironment and immune system, and partially mimic all human subtypes save luminal cancers (Pfefferle et al., 2013; Holen et al., 2017). However, GEMMs involve extensive costs and breeding time, often express supra-physiological levels of the transgene, and can be genetically different compared to their human counterpart (Pfefferle et al., 2013). Only 16 of the 30 most commonly mutated genes in human breast cancers were found to be mutated in a panel of metastatic GEMMs and SMMs (Yang et al., 2017). Although SMMs have higher mutational burden in metastases than in primaries like human breast cancers (Yang et al., 2017; Yates et al., 2017), GEMMs and SMMs rarely mimic clinical metastasis (Holen et al., 2017). In spite of these pitfalls, GEMMS have been instrumental in generating insights into breast cancer biology – e.g., determining that BRCA1 mutant tumors derive from luminal progenitor rather than basal cells (Molyneux et al., 2010) and in testing novel drugs combinations (Jaspers et al., 2013) (Figure 1C).
Patient-derived xenografts (PDXs), which involve injection of human cancer cells either orthopically in the mouse mammary fat pad or subcutaneously into immunocompromised mice, provide an in vivo alternative to GEMMs (Hidalgo et al., 2014; Holen et al., 2017). They have helped address clinically relevant questions including the contribution of heterogeneity to, and the mechanism of, drug resistance (Byrne et al., 2017). PDXs can be passaged in different mice allowing expansion of patient tissue whilst still maintaining ‘omics profiles of the patient tumor; and they spontaneously metastasize (DeRose et al., 2011; Dobrolecki et al., 2016). Drawbacks for the use of PDXs include the selection of more aggressive cells within the patient sample and the use of immunocompromised mice to prevent tumor rejection. Developing mice with humanized immune systems can help to address this problem (Hasgur et al., 2016), as recently shown for a metastasis model (Rosato et al., 2018) (Figure 1D).
In conclusion, choosing the correct model to study breast cancer depends on several factors including the biomedical question, sample availability, costs, etc. (Figure 1). We envision that future interdisciplinary research will be based on a combination of different models to identify and validate new therapeutic targets for breast cancer treatment with the advent of next generation sequencing and more robust instrumentation, ‘omics approaches, like genomics and proteomics, are becoming more accessible and are increasing the information that can be obtained from breast cancer models. Thus, ‘omics approaches applied to the combination of different models will provide molecular information on a global scale and will identify novel targets.
System biology based on ‘omics approaches and network science are becoming popular in cancer research (Manem et al., 2018), despite high costs in terms of sample handling, instrumentation, and time for data analysis. Integrating ‘omics approaches allows the unbiased analysis of the whole genome, transcriptome, proteome, or metabolome starting from different types of samples (Figure 1E and Table 1).
Table 1. A selection of single- and multi-‘omics-based breast cancer studies that have contributed to major discoveries in the field of breast cancer research where method strengths and weakness are reported.
Next generation sequencing (NGS) allows rapid and relatively inexpensive DNA sequencing covering the whole genome (Park and Kim, 2016). Genomic approaches helped redefine breast cancer subtypes (Cancer Genome Atlas Network, 2012), identify mutational landscapes (Stephens et al., 2012) or single nucleotide polymorphisms (SNPs) as a biomarker of breast cancer susceptibility (Michailidou et al., 2017) or therapeutic response (Kus et al., 2016). NGS has also facilitated the discovery of breast cancer driver mutations (Nik-Zainal et al., 2016), tumor heterogeneity (Yates et al., 2015) and novel therapeutic targets in metastatic disease (Bertucci et al., 2019). Finally, single-cell analysis allowed the study of breast cancer stem cells (Lawson et al., 2015). However, accurate genomic analysis requires large numbers of sequence reads which increases both time and cost.
These discoveries demonstrate the potential for genomics to transform breast cancer treatment (Hamdan et al., 2019). For instance, genomics helped identify patients for clinical trials (Curtis et al., 2012) or high risk individuals through mutation screening in breast cancer susceptibility (BRCA) 1–2 genes (Evans et al., 2008) and contributed to therapeutic decision making (Tsoutsou et al., 2017; Bergom et al., 2019). As an invaluable resource for researchers, the Catalogue of Somatic Mutations in Cancer (COSMIC) has compiled genomic data from breast cancer patient samples and correlated them to cellular functions and drug resistance (Forbes et al., 2017). Finally, genomic analysis for the early identification of tailored therapy for cancer patients has been made possible with the development of the Cancer Genome Atlas (TCGA)1. We envision that TCGA and COSMIC databases will revolutionize cancer patient diagnosis and treatment (Ashton-Prolla et al., 2015). This is already being realized in the MOSCATO trial where druggable genomic aberrations were identified and targeted in patients (Massard et al., 2017).
In addition, cell-free/circulating tumor DNA (cf/ctDNA) can be useful in monitoring clonal evolution and residual tumor presence following treatment (Buono et al., 2019). However, as ctDNA usually comprises 180–200 bp fragments from apoptotic cells, there are varying degrees of success in identifying useful biomarkers with high sensitivity (Sefrioui et al., 2015). Despite this, serial screening for mutations in ctDNA has allowed metastatic detection 8 months before clinical presentation (Garcia-Murillas et al., 2015).
Together with genomics, epigenomics (the study of DNA modifications and their impact) is also providing novel markers for breast cancer prognosis (Davalos et al., 2017) and for detection of metastasis (Legendre et al., 2015). Epigenomics has begun to illuminate the link between menopause and lifestyle factors with breast cancer risk and so may provide prognostic utility in future (Crujeiras et al., 2017).
Transcriptomics uses microarrays, which quantify a set of predetermined sequences, and RNA sequencing (RNA-Seq), which uses high-throughput sequencing to capture all sequences to determine the quantity of a transcript (Lowe et al., 2017). These approaches have been used to classify breast cancer molecular subtypes in cell lines (Neve et al., 2006) and patient-derived samples (Wu et al., 2018; Jiang et al., 2019), to compare primary breast cancers and their metastases (Varešlija et al., 2018), and to visualize phenotypic features of breast cancer cells in 3D culture (Tirier et al., 2019). In addition, transcriptomics is allowing immune cell characterization in normal breast and tumor tissue (Chung et al., 2017; Azizi et al., 2018), potentially providing a mechanism to inform immunotherapeutic decisions.
As transcriptomics does not provide information on the expression, post-translational modifications (PTMs), or activation status of proteins it is less informative than proteomics for novel therapeutic target discovery. Recent advancements in single cell analysis may open a new era in breast cancer research to identify drivers, biomarkers, and novel therapeutic targets (Hong et al., 2019).
In the clinic, analysis of mRNA expression of gene subsets, involved in ER signaling, HER2 signaling, proliferation and invasion, is already used to predict relapse and determine whether patients would benefit from neoadjuvant chemotherapy (Vieira and Schmitt, 2018). Furthermore, as patients with elevated expression of a migratory mRNA signature had worse overall survival than those with a proliferative mRNA signature and so responded significantly better to chemotherapeutics that targeted the cytoskeleton (Nair et al., 2019) transcriptomics has the potential to inform chemotherapeutic decisions in future.
As patient tumor biopsies are typically formalin fixed and paraffin-embedded (FFPE), a preservation procedure that reduces RNA integrity (von Ahlfen et al., 2007), fresh frozen tissue collection should become the standard procedure for mRNA expression to inform clinical decisions.
Proteomics studies the expressed proteome and its PTMs by mass spectrometry (MS), protein microarrays, and, more recently, mass cytometry. Advances in samples handling, instrumentation, and data analysis now provide unprecedented insights into the abundance and function of the (modified) proteome (Doll et al., 2019). Proteomics can assess tissue or blood samples, thus lending itself to clinical applications (Mardamshina and Geiger, 2017). For instance, specific serum biomarkers have been discovered by proteomic studies (Li et al., 2002; Raso et al., 2012), potentially providing an early diagnosis signature (Saadatmand et al., 2015). Correlation between RNA or gene copy number with protein expression is rather low (Mertins et al., 2016; Johansson et al., 2019) thus analyzing the patient proteome holds promise for identifying novel preventative or therapeutic targets not previously identified at the genomic or transcriptomic level. This idea is supported by the fact that currently used anti-breast cancer drugs predominantly act against proteins.
MS-based proteomics has been used to characterize cell lines (Huang F. K. et al., 2017), to reveal novel layers of breast cancer classification (Tyanova et al., 2016; Yanovich et al., 2018), and to identify proteins involved in drug resistance (Liu et al., 2006). Furthermore, phosphoproteomics that identify phosphorylated proteins (von Stechow et al., 2015) has been used to connect somatic mutations to signaling (proteogenomics) (Mertins et al., 2016), to identify kinases signatures in TNBC (Zagorac et al., 2018), and to map drug targets for personalized treatments (Pierobon et al., 2018). These discoveries have diagnostic and prognostic potential which is worth further exploring and implementing in the clinic when phosphoproteomics methods will become common practice.
An alternative to MS-based proteomics is provided by mass cytometry where single cells are probed with metal ion-labeled antibodies and then samples are analyzed by time-of-flight mass spectrometry (Leelatian et al., 2017). In breast cancer research this technology has been recently used to identify cell types and immune infiltrates within a tumor (Wagner et al., 2019). However, this method remains limited by antibody availability. Similarly to transcriptomics, phosphoproteomics is also limited by the availability of fresh frozen tissue as the phosphoproteome is substantially altered by FFPE preservation (Wakabayashi et al., 2014).
In conclusion, analyzing the proteome and phosphoproteome of patients at different breast cancer stages will help identify signatures for personalized treatments, ideally starting from liquid biopsies. In future proteomics may be used to follow the response to treatment by analyzing changes in patient proteome so to adapt the therapeutic plan.
Metabolomics is the system-wide identification of endogenous metabolites from bodily fluids in a targeted or unbiased manner (Silva et al., 2019). Metabolomics has been used to correlate changes in metabolism with proliferation rate in breast cancer cells (Jerby et al., 2012), to cluster tumor subtypes (Haukaas et al., 2016), to analyze the lipids content in breast cancer cells (Lisa et al., 2017), and to correlate nutrients with breast cancer risk (Playdon et al., 2017). More recently, this approach has begun paving the way for the identification of metabolic-state specific biomarkers for breast cancer diagnosis (Jasbi et al., 2019). Therefore, metabolomics will allow further insights into correlation between metabolism, epigenomic and proteomic alterations and breast cancer progression or treatment.
The contribution of each aforementioned ‘omics technology to the understanding of breast cancer biology and to the discovery of novel targets or biomarkers has been substantial. Integrating these approaches is predicted to be even more powerful (Chakraborty et al., 2018; Manem et al., 2018) (Table 1) For instance, a genomic/transcriptomic/proteomic combined approach has confirmed the existence of the known molecular subtypes (LumA, LumB, HER2+, and BL) of breast cancer (Cancer Genome Atlas Network, 2012) as well as allowing identification of novel therapeutic targets in PDX models (Huang K. L. et al., 2017). Recently, a comprehensive analysis of clinical, genomics, and transcriptomics data has uncovered the TNBC landscape (Jiang et al., 2019). Proteogenomics has challenged the way in which somatic mutations contribute to signaling changes (Mertins et al., 2016), highlighting the need of both these analyses to confirm the therapeutic importance of a genetic alteration. For instance, patients lacking HER2 amplification were found to have enriched HER2 signaling (Pierobon et al., 2018), underlining the importance of analyzing changes in signaling to plan the correct therapeutic approach. With the development of single cell analysis in genomics, transcriptomics and proteomics (Linnarsson and Teichmann, 2016; Hong et al., 2019; Marx, 2019; Wagner et al., 2019) there are opportunities to better understand breast cancer heterogeneity and the role of the microenvironment. Finally, it would be fascinating to integrate ‘omics approaches with radiomics (quantitative information from digital images) (Pinker et al., 2018) and with imaging-based mass spectrometry that is rapidly changing the field of spatial proteomics (Keren et al., 2018) to guide patient-specific therapy or patient stratification.
Integrating ‘omics approaches with traditional methods has already helped underline the validity of some of the models, for example, highlighting that omics profiles are maintained in PDX models through multiple passages (Zhang et al., 2013). Multiomics technologies have also facilitated novel discoveries in existing models (Chakraborty et al., 2018). A combination of genomics, transcriptomics and proteomics has elucidated drivers of mesenchymal-to-epithelial transition in 2D culture (Bhatia et al., 2019). Transcriptomics in GEMM and SMM-derived cell lines allowed identification of differentially regulated genes and their contribution to metastases (Yang et al., 2017) Transcriptomics and proteogenomics in PDXs have finally helped to profile gene/proteins expression to identify novel targets (Huang K. L. et al., 2017).
‘Omics technologies have not only improved the power of traditional models in breast cancer research, but also revolutionized the analysis of patient samples, making them an indispensble tool in translational studies. Integration of ‘omics approaches requires powerful computational and statistical methods to analyze and interpret the vast quantity of available data, for instance combining linear mathematical models with machine learning and network science principles (Manem et al., 2018). This requires collaboration between cancer scientists, computational biologists and medical statisticians to create robust methods to gain insights into cancer biology and to inform clinical trials and personalized therapeutic regimes.
With ‘omics technologies applied to patient samples becoming robust, our understanding of the mechanisms driving breast cancer and the discovery of novel biomarkers and therapeutic targets have improved significantly over the last few years (Chakraborty et al., 2018; Manem et al., 2018). For instance, the use of molecular assays, including OncotypeDx and MammaPrint in the clinic is based on advancements in genomic technologies (Gupta et al., 2015; Vieira and Schmitt, 2018). Transparent sharing of ‘omics data in databases like COSMIC (Forbes et al., 2008), PRIDE (Jones et al., 2006) and others (Table 2) will allow unbiased analysis of available data by different groups to find previously unnoticed potential genes or proteins of interest as biomarkers or therapeutic targets.
Table 2. A selection of ‘omics data repositories built for data sharing and to support research questions (Bamford et al., 2004; Fontaine et al., 2011; Omenn, 2014; Speake et al., 2015; Tomczak et al., 2015; Clough and Barrett, 2016; Rudnick et al., 2016; Chou et al., 2019; Tate et al., 2019).
The implementation of ‘omics approaches in clinical practice will allow analysis of changes in patients at a global level by improving diagnosis and choice of therapeutic plan so far based on a few markers. We predict that ‘omics technologies-guided biomarker identification will allow early tumor detection so that treatments can start earlier and that the identification of novel targets will decrease reliance on non-targeted therapies, thus improving the quality of life for breast cancer patients.
All authors listed have made a substantial, direct and intellectual contribution to the work, and approved it for publication.
Research in the lab of CF was supported by Wellcome Trust (Sir Henry Dale Fellowship grant number 107636/Z/15/Z) and by the Biotechnology and Biological Sciences Research Council (BBSRC responsive mode project grant number BB/R015864/1). JP was supported by R-UK Non-Clinical Training Award – 2018 grant number A27445.
The authors declare that the research was conducted in the absence of any commercial or financial relationships that could be construed as a potential conflict of interest.
We thank all members of the Francavilla lab and Dr. Bruno Simoes, Prof. Robert Clarke (The University of Manchester) and Dr. Ciara O’Brien (The Christie Hospital NHS Foundation Trust and The University of Manchester) for helpful discussion and for reading the manuscript. We also thank members of the Bio-MS facility, The University of Manchester. We apologize to authors whose work could not be cited due to space limitations.
Ashton-Prolla, P., Goldim, J. R., Vairo, F. P., da Silveira Matte, U., and Sequeiros, J. (2015). Genomic analysis in the clinic: benefits and challenges for health care professionals and patients in Brazil. J. Community Genet. 6, 275–283. doi: 10.1007/s12687-015-0238-0
Azizi, E., Carr, A. J., Plitas, G., Cornish, A. E., Konopacki, C., Prabhakaran, S., et al. (2018). Single-cell map of diverse immune phenotypes in the breast tumor microenvironment. Cell 174, 1293.e36–1308.e36. doi: 10.1016/j.cell.2018.05.060
Bamford, S., Dawson, E., Forbes, S., Clements, J., Pettett, R., Dogan, A., et al. (2004). The COSMIC (catalogue of somatic mutations in cancer) database and website. Br. J. Cancer 91, 355–358. doi: 10.1038/sj.bjc.6601894
Bartsch, R., Wenzel, C., Zielinski, C. C., and Steger, G. G. (2007). HER-2-positive breast cancer: hope beyond trastuzumab. Biodrugs 21, 69–77. doi: 10.2165/00063030-200721020-00001
Beaver, J. A., and Park, B. H. (2012). The BOLERO-2 trial: the addition of everolimus to exemestane in the treatment of postmenopausal hormone receptor-positive advanced breast cancer. Future Oncol. 8, 651–657. doi: 10.2217/fon.12.49
Bergom, C., West, C. M., Higginson, D. S., Abazeed, M. E., Arun, B., Bentzen, S. M., et al. (2019). The implications of genetic testing on radiotherapy decisions: a guide for radiation oncologists. Int. J. Radiat. Oncol. Biol. Phys. 105, 698–712.
Bertucci, F., Ng, C. K. Y., Patsouris, A., Droin, N., Piscuoglio, S., Carbuccia, N., et al. (2019). Genomic characterization of metastatic breast cancers. Nature 569, 560–564. doi: 10.1038/s41586-019-1056-z
Bhatia, S., Monkman, J., Blick, T., Duijf, P. H., Nagaraj, S. H., and Thompson, E. W. (2019). Multi-omics characterization of the spontaneous mesenchymal-epithelial transition in the PMC42 breast cancer cell lines. J. Clin. Med. 8:E1253. doi: 10.3390/jcm8081253
Bray, F., Ferlay, J., Soerjomataram, I., Siegel, R. L., Torre, L. A., and Jemal, A. (2018). Global cancer statistics 2018: GLOBOCAN estimates of incidence and mortality worldwide for 36 cancers in 185 countries. Cancer J. Clin. 68, 394–424. doi: 10.3322/caac.21492
Buono, G., Gerratana, L., Bulfoni, M., Provinciali, N., Basile, D., Giuliano, M., et al. (2019). Circulating tumor DNA analysis in breast cancer: Is it ready for prime-time? Cancer Treatm. Rev. 73, 73–83. doi: 10.1016/j.ctrv.2019.01.004
Byrne, A. T., Alferez, D. G., Amant, F., Annibali, D., Arribas, J., Biankin, A. V., et al. (2017). Interrogating open issues in cancer precision medicine with patient-derived xenografts. Nat. Rev. Cancer 17, 254–268. doi: 10.1038/nrc.2016.140
Cancer Genome Atlas Network. (2012). Comprehensive molecular portraits of human breast tumours. Nature 490, 61–70. doi: 10.1038/nature11412
Chakraborty, S., Hosen, M. I., Ahmed, M., and Shekhar, H. U. (2018). Onco-Multi-OMICS approach: a new frontier in cancer research. Biomed. Res. Int. 2018:9836256. doi: 10.1155/2018/9836256
Cho, N. (2016). Molecular subtypes and imaging phenotypes of breast cancer. Ultrasonography 35, 281–288. doi: 10.14366/usg.16030
Chou, P. H., Liao, W. C., Tsai, K. W., Chen, K. C., Yu, J. S., and Chen, T. W. (2019). TACCO, a database connecting transcriptome alterations, pathway alterations and clinical outcomes in cancers. Sci. Rep. 9:3877. doi: 10.1038/s41598-019-40629-z
Chung, W., Eum, H. H., Lee, H. O., Lee, K. M., Lee, H. B., Kim, K. T., et al. (2017). Single-cell RNA-seq enables comprehensive tumour and immune cell profiling in primary breast cancer. Nat. Commun. 8:15081. doi: 10.1038/ncomms15081
Clarke, R., Tyson, J. J., and Dixon, J. M. (2015). Endocrine resistance in breast cancer – An overview and update. Mol. Cell. Endocrinol. 418, 220–234. doi: 10.1016/j.mce.2015.09.035
Clough, E., and Barrett, T. (2016). The gene expression omnibus database. Methods Mol. Biol. 1418, 93–110. doi: 10.1007/978-1-4939-3578-9_5
Colzani, E., Johansson, A. L., Liljegren, A., Foukakis, T., Clements, M., Adolfsson, J., et al. (2014). Time-dependent risk of developing distant metastasis in breast cancer patients according to treatment, age and tumour characteristics. Br. J. Cancer 110, 1378–1384. doi: 10.1038/bjc.2014.5
Crujeiras, A. B., Diaz-Lagares, A., Stefansson, O. A., Macias-Gonzalez, M., Sandoval, J., Cueva, J., et al. (2017). Obesity and menopause modify the epigenomic profile of breast cancer. Endocr. Relat. Cancer 24, 351–363. doi: 10.1530/ERC-16-0565
Curtis, C., Shah, S. P., Chin, S. F., Turashvili, G., Rueda, O. M., Dunning, M. J., et al. (2012). The genomic and transcriptomic architecture of 2,000 breast tumours reveals novel subgroups. Nature 486, 346–352. doi: 10.1038/nature10983
Dai, X., Cheng, H., Bai, Z., and Li, J. (2017). Breast cancer cell line classification and its relevance with breast tumor subtyping. J. Cancer 8, 3131–3141. doi: 10.7150/jca.18457
Davalos, V., Martinez-Cardus, A., and Esteller, M. (2017). The epigenomic revolution in breast cancer: from single-gene to genome-wide next-generation approaches. Am. J. Pathol. 187, 2163–2174. doi: 10.1016/j.ajpath.2017.07.002
DeRose, Y. S., Wang, G., Lin, Y. C., Bernard, P. S., Buys, S. S., Ebbert, M. T., et al. (2011). Tumor grafts derived from women with breast cancer authentically reflect tumor pathology, growth, metastasis and disease outcomes. Nat. Med. 17, 1514–1520. doi: 10.1038/nm.2454
Djomehri, S. I., Burman, B., Gonzalez, M. E., Takayama, S., and Kleer, C. G. (2019). A reproducible scaffold-free 3D organoid model to study neoplastic progression in breast cancer. J. Cell Commun. Signal. 13, 129–143. doi: 10.1007/s12079-018-0498-7
Dobrolecki, L. E., Airhart, S. D., Alferez, D. G., Aparicio, S., Behbod, F., Bentires-Alj, M., et al. (2016). Patient-derived xenograft (PDX) models in basic and translational breast cancer research. Cancer Metastasis Rev. 35, 547–573. doi: 10.1007/s10555-016-9653-x
Doll, S., Gnad, F., and Mann, M. (2019). The case for proteomics and phospho-proteomics in personalized cancer medicine. Proteomics Clin. Appl. 13:e1800113. doi: 10.1002/prca.201800113
Dutta, D., Heo, I., and Clevers, H. (2017). Disease modeling in stem cell-derived 3D organoid systems. Trends Mol. Med. 23, 393–410. doi: 10.1016/j.molmed.2017.02.007
Elenbaas, B., Spirio, L., Koerner, F., Fleming, M. D., Zimonjic, D. B., Donaher, J. L., et al. (2001). Human breast cancer cells generated by oncogenic transformation of primary mammary epithelial cells. Genes Dev. 15, 50–65. doi: 10.1101/gad.828901
Evans, D. G., Shenton, A., Woodward, E., Lalloo, F., Howell, A., and Maher, E. R. (2008). Penetrance estimates for BRCA1 and BRCA2 based on genetic testing in a Clinical Cancer Genetics service setting: risks of breast/ovarian cancer quoted should reflect the cancer burden in the family. BMC Cancer 8:155. doi: 10.1186/1471-2407-8-155
Fallahpour, S., Navaneelan, T., De, P., and Borgo, A. (2017). Breast cancer survival by molecular subtype: a population-based analysis of cancer registry data. CMAJ Open 5, E734–E739. doi: 10.9778/cmajo.20170030
Fatehullah, A., Tan, S. H., and Barker, N. (2016). Organoids as an in vitro model of human development and disease. Nat. Cell Biol. 18, 246–254. doi: 10.1038/ncb3312
Fisher, B., Anderson, S., Bryant, J., Margolese, R. G., Deutsch, M., Fisher, E. R., et al. (2002). Twenty-year follow-up of a randomized trial comparing total mastectomy, lumpectomy, and lumpectomy plus irradiation for the treatment of invasive breast cancer. N. Engl. J. Med. 347, 1233–1241. doi: 10.1056/nejmoa022152
Fontaine, J. F., Priller, F., Barbosa-Silva, A., and Andrade-Navarro, M. A. (2011). Genie: literature-based gene prioritization at multi genomic scale. Nucleic Acids Res. 39, W455–W461. doi: 10.1093/nar/gkr246
Forbes, S. A., Beare, D., Boutselakis, H., Bamford, S., Bindal, N., Tate, J., et al. (2017). COSMIC: somatic cancer genetics at high-resolution. Nucleic Acids Res. 45, D777–D783. doi: 10.1093/nar/gkw1121
Forbes, S. A., Bhamra, G., Bamford, S., Dawson, E., Kok, C., Clements, J., et al. (2008). The catalogue of somatic mutations in cancer (COSMIC). Curr. Protoc. Hum. Genet. 57, 10.11.1–10.11.26. doi: 10.1002/0471142905.hg1011s57
Fujii, M., Shimokawa, M., Date, S., Takano, A., Matano, M., Nanki, K., et al. (2016). A colorectal tumor organoid library demonstrates progressive loss of niche factor requirements during tumorigenesis. Cell Stem Cell 18, 827–838. doi: 10.1016/j.stem.2016.04.003
Garcia-Becerra, R., Santos, N., Diaz, L., and Camacho, J. (2012). Mechanisms of resistance to endocrine therapy in breast cancer: focus on signaling pathways, miRNAs and genetically based resistance. Int. J. Mol. Sci. 14, 108–145. doi: 10.3390/ijms14010108
Garcia-Murillas, I., Schiavon, G., Weigelt, B., Ng, C., Hrebien, S., Cutts, R. J., et al. (2015). Mutation tracking in circulating tumor DNA predicts relapse in early breast cancer. Sci. Transl. Med. 7:302ra133. doi: 10.1126/scitranslmed.aab0021
Garrido-Castro, A. C., Lin, N. U., and Polyak, K. (2019). Insights into molecular classifications of triple-negative breast cancer: improving patient selection for treatment. Cancer Discov. 9, 176–198. doi: 10.1158/2159-8290.CD-18-1177
Gupta, A., Mutebi, M., and Bardia, A. (2015). Gene-expression-based predictors for breast cancer. Ann. Surg. Oncol. 22, 3418–3432. doi: 10.1245/s10434-015-4703-0
Hamdan, D., Nguyen, T. T., Leboeuf, C., Meles, S., Janin, A., and Bousquet, G. (2019). Genomics applied to the treatment of breast cancer. Oncotarget 10, 4786–4801. doi: 10.18632/oncotarget.27102
Hanahan, D., and Weinberg, R. A. (2011). Hallmarks of cancer: the next generation. Cell 144, 646–674. doi: 10.1016/j.cell.2011.02.013
Haque, M. M., and Desai, K. V. (2019). Pathways to endocrine therapy resistance in breast cancer. Front. Endocrinol. 10:573. doi: 10.3389/fendo.2019.00573
Hasgur, S., Aryee, K. E., Shultz, L. D., Greiner, D. L., and Brehm, M. A. (2016). Generation of immunodeficient mice bearing human immune systems by the engraftment of hematopoietic stem cells. Methods Mol. Biol. 1438, 67–78. doi: 10.1007/978-1-4939-3661-8_4
Haukaas, T. H., Euceda, L. R., Giskeodegard, G. F., Lamichhane, S., Krohn, M., Jernstrom, S., et al. (2016). Metabolic clusters of breast cancer in relation to gene- and protein expression subtypes. Cancer Metab. 4:12. doi: 10.1186/s40170-016-0152-x
Hidalgo, M., Amant, F., Biankin, A. V., Budinska, E., Byrne, A. T., Caldas, C., et al. (2014). Patient-derived xenograft models: an emerging platform for translational cancer research. Cancer Discov. 4, 998–1013. doi: 10.1158/2159-8290.CD-14-0001
Holen, I., Speirs, V., Morrissey, B., and Blyth, K. (2017). In vivo models in breast cancer research: progress, challenges and future directions. Dis. Model. Mech. 10, 359–371. doi: 10.1242/dmm.028274
Holliday, D. L., and Speirs, V. (2011). Choosing the right cell line for breast cancer research. Breast Cancer Res. 13:215. doi: 10.1186/bcr2889
Hong, S. P., Chan, T. E., Lombardo, Y., Corleone, G., Rotmensz, N., Bravaccini, S., et al. (2019). Single-cell transcriptomics reveals multi-step adaptations to endocrine therapy. Nat. Commun. 10:3840. doi: 10.1038/s41467-019-11721-9
Huang, F. K., Zhang, G., Lawlor, K., Nazarian, A., Philip, J., Tempst, P., et al. (2017). Deep coverage of global protein expression and phosphorylation in breast tumor cell lines using TMT 10-plex isobaric labeling. J. Proteome Res. 16, 1121–1132. doi: 10.1021/acs.jproteome.6b00374
Huang, K. L., Li, S., Mertins, P., Cao, S., Gunawardena, H. P., Ruggles, K. V., et al. (2017). Proteogenomic integration reveals therapeutic targets in breast cancer xenografts. Nat. Commun. 8:14864.
Imamura, Y., Mukohara, T., Shimono, Y., Funakoshi, Y., Chayahara, N., Toyoda, M., et al. (2015). Comparison of 2D- and 3D-culture models as drug-testing platforms in breast cancer. Oncol. Rep. 33, 1837–1843. doi: 10.3892/or.2015.3767
Iqbal, N., and Iqbal, N. (2014). Human epidermal growth factor receptor 2 (HER2) in cancers: overexpression and therapeutic implications. Mol. Biol. Int. 2014:852748. doi: 10.1155/2014/852748
Jasbi, P., Wang, D., Cheng, S. L., Fei, Q., Cui, J. Y., Liu, L., et al. (2019). Breast cancer detection using targeted plasma metabolomics. J. Chromatogr. B Anal. Technol. Biomed. Life Sci. 1105, 26–37. doi: 10.1016/j.jchromb.2018.11.029
Jaspers, J. E., Kersbergen, A., Boon, U., Sol, W., van Deemter, L., Zander, S. A., et al. (2013). Loss of 53BP1 causes PARP inhibitor resistance in Brca1-mutated mouse mammary tumors. Cancer Discov. 3, 68–81. doi: 10.1158/2159-8290.CD-12-0049
Jerby, L., Wolf, L., Denkert, C., Stein, G. Y., Hilvo, M., Oresic, M., et al. (2012). Metabolic associations of reduced proliferation and oxidative stress in advanced breast cancer. Cancer Res. 72, 5712–5720. doi: 10.1158/0008-5472.CAN-12-2215
Jiang, Y. Z., Ma, D., Suo, C., Shi, J., Xue, M., Hu, X., et al. (2019). Genomic and transcriptomic landscape of triple-negative breast cancers: subtypes and treatment strategies. Cancer Cell 35, 428.e5–440.e5. doi: 10.1016/j.ccell.2019.02.001
Johansson, H. J., Socciarelli, F., Vacanti, N. M., Haugen, M. H., Zhu, Y., Siavelis, I., et al. (2019). Breast cancer quantitative proteome and proteogenomic landscape. Nat. Commun. 10:1600. doi: 10.1038/s41467-019-09018-y
Jones, P., Côté, R. G., Martens, L., Quinn, A. F., Taylor, C. F., Derache, W., et al. (2006). PRIDE: a public repository of protein and peptide identifications for the proteomics community. Nucleic Acids Res. 34, D659–D663.
Keren, L., Bosse, M., Marquez, D., Angoshtari, R., Jain, S., Varma, S., et al. (2018). A structured tumor-immune microenvironment in triple negative breast cancer revealed by multiplexed ion beam imaging. Cell 174, 1373.e19–1387.e19. doi: 10.1016/j.cell.2018.08.039
Kontani, K., Hashimoto, S., Murazawa, C., Norimura, S., Tanaka, H., Ohtani, M., et al. (2014). Factors responsible for long-term survival in metastatic breast cancer. World J. Surg. Oncol. 12:344. doi: 10.1186/1477-7819-12-344
Kornblum, N., Zhao, F., Manola, J., Klein, P., Ramaswamy, B., Brufsky, A., et al. (2018). Randomized phase II trial of fulvestrant plus everolimus or placebo in postmenopausal women with hormone receptor-positive, human epidermal growth factor receptor 2-negative metastatic breast cancer resistant to aromatase inhibitor therapy: results of PrE0102. J. Clin. Oncol. 36, 1556–1563. doi: 10.1200/JCO.2017.76.9331
Kus, T., Aktas, G., Kalender, M. E., Demiryurek, A. T., Ulasli, M., Oztuzcu, S., et al. (2016). Polymorphism of CYP3A4 and ABCB1 genes increase the risk of neuropathy in breast cancer patients treated with paclitaxel and docetaxel. Oncol. Targets Ther. 9, 5073–5080. doi: 10.2147/OTT.S106574
Larramendy, M. L., Lushnikova, T., Bjorkqvist, A. M., Wistuba, I. I., Virmani, A. K., Shivapurkar, N., et al. (2000). Comparative genomic hybridization reveals complex genetic changes in primary breast cancer tumors and their cell lines. Cancer Genet. Cytogenet. 119, 132–138. doi: 10.1016/s0165-4608(99)00226-5
Lawson, D. A., Bhakta, N. R., Kessenbrock, K., Prummel, K. D., Yu, Y., Takai, K., et al. (2015). Single-cell analysis reveals a stem-cell program in human metastatic breast cancer cells. Nature 526, 131–135. doi: 10.1038/nature15260
Leelatian, N., Doxie, D. B., Greenplate, A. R., Mobley, B. C., Lehman, J. M., Sinnaeve, J., et al. (2017). Single cell analysis of human tissues and solid tumors with mass cytometry. Cytometry B Clin. Cytom. 92, 68–78. doi: 10.1002/cyto.b.21481
Legendre, C., Gooden, G. C., Johnson, K., Martinez, R. A., Liang, W. S., and Salhia, B. (2015). Whole-genome bisulfite sequencing of cell-free DNA identifies signature associated with metastatic breast cancer. Clin. Epigenet. 7:100. doi: 10.1186/s13148-015-0135-8
Lehmann, B. D., Jovanovic, B., Chen, X., Estrada, M. V., Johnson, K. N., Shyr, Y., et al. (2016). Refinement of triple-negative breast cancer molecular subtypes: implications for neoadjuvant chemotherapy selection. PLoS One 11:e0157368. doi: 10.1371/journal.pone.0157368
Li, J., Zhang, Z., Rosenzweig, J., Wang, Y. Y., and Chan, D. W. (2002). Proteomics and bioinformatics approaches for identification of serum biomarkers to detect breast cancer. Clin. Chem. 48, 1296–1304.
Linnarsson, S., and Teichmann, S. A. (2016). Single-cell genomics: coming of age. Genome Biol. 17:97.
Lisa, M., Cifkova, E., Khalikova, M., Ovcacikova, M., and Holcapek, M. (2017). Lipidomic analysis of biological samples: Comparison of liquid chromatography, supercritical fluid chromatography and direct infusion mass spectrometry methods. J. Chromatogr. A 1525, 96–108. doi: 10.1016/j.chroma.2017.10.022
Liu, K., Newbury, P. A., Glicksberg, B. S., Zeng, W. Z. D., Paithankar, S., Andrechek, E. R., et al. (2019). Evaluating cell lines as models for metastatic breast cancer through integrative analysis of genomic data. Nat. Commun. 10:2138. doi: 10.1038/s41467-019-10148-6
Liu, Y., Liu, H., Han, B., and Zhang, J. T. (2006). Identification of 14-3-3sigma as a contributor to drug resistance in human breast cancer cells using functional proteomic analysis. Cancer Res. 66, 3248–3255. doi: 10.1158/0008-5472.can-05-3801
Lowe, R., Shirley, N., Bleackley, M., Dolan, S., and Shafee, T. (2017). Transcriptomics technologies. PLoS Comput. Biol. 13:e1005457. doi: 10.1371/journal.pcbi.1005457
Ma, C. X., Reinert, T., Chmielewska, I., and Ellis, M. J. (2015). Mechanisms of aromatase inhibitor resistance. Nat. Rev. Cancer 15, 261–275. doi: 10.1038/nrc3920
Manem, V. S. K., Salgado, R., Aftimos, P., Sotiriou, C., and Haibe-Kains, B. (2018). Network science in clinical trials: A patient-centered approach. Semin. Cancer Biol. 52, 135–150. doi: 10.1016/j.semcancer.2017.12.006
Mardamshina, M., and Geiger, T. (2017). Next-generation proteomics and its application to clinical breast cancer research. Am. J. Pathol. 187, 2175–2184. doi: 10.1016/j.ajpath.2017.07.003
Marx, V. (2019). A dream of single-cell proteomics. Nat. Methods 16, 809–812. doi: 10.1038/s41592-019-0540-6
Massard, C., Michiels, S., Ferte, C., Le Deley, M. C., Lacroix, L., Hollebecque, A., et al. (2017). High-throughput genomics and clinical outcome in hard-to-treat advanced cancers: results of the MOSCATO 01 trial. Cancer Discov. 7, 586–595. doi: 10.1158/2159-8290.CD-16-1396
Masuda, H., Zhang, D., Bartholomeusz, C., Doihara, H., Hortobagyi, G. N., and Ueno, N. T. (2012). Role of epidermal growth factor receptor in breast cancer. Breast Cancer Res. Treat. 136, 331–345.
McCann, K. E., and Hurvitz, S. A. (2018). Advances in the use of PARP inhibitor therapy for breast cancer. Drugs Context 7:212540. doi: 10.7573/dic.212540
Mertins, P., Mani, D. R., Ruggles, K. V., Gillette, M. A., Clauser, K. R., Wang, P., et al. (2016). Proteogenomics connects somatic mutations to signalling in breast cancer. Nature 534, 55–62. doi: 10.1038/nature18003
Michailidou, K., Lindström, S., Dennis, J., Beesley, J., Hui, S., Kar, S., et al. (2017). Association analysis identifies 65 new breast cancer risk loci. Nature 551, 92–94. doi: 10.1038/nature24284
Milioli, H. H., Tishchenko, I., Riveros, C., Berretta, R., and Moscato, P. (2017). Basal-like breast cancer: molecular profiles, clinical features and survival outcomes. BMC Med. Genom. 10:19. doi: 10.1186/s12920-017-0250-9
Minn, A. J., Kang, Y., Serganova, I., Gupta, G. P., Giri, D. D., Doubrovin, M., et al. (2005). Distinct organ-specific metastatic potential of individual breast cancer cells and primary tumors. J. Clin. Investig. 115, 44–55. doi: 10.1172/jci22320
Mohammed, H., Russell, I. A., Stark, R., Rueda, O. M., Hickey, T. E., Tarulli, G. A., et al. (2015). Progesterone receptor modulates ERα action in breast cancer. Nat. 523, 313–317.
Molyneux, G., Geyer, F. C., Magnay, F. A., McCarthy, A., Kendrick, H., Natrajan, R., et al. (2010). BRCA1 basal-like breast cancers originate from luminal epithelial progenitors and not from basal stem cells. Cell Stem Cell 7, 403–417. doi: 10.1016/j.stem.2010.07.010
Nair, N. U., Das, A., Rogkoti, V. M., Fokkelman, M., Marcotte, R., de Jong, C. G., et al. (2019). Migration rather than proliferation transcriptomic signatures are strongly associated with breast cancer patient survival. Sci. Rep. 9:10989. doi: 10.1038/s41598-019-47440-w
Neve, R. M., Chin, K., Fridlyand, J., Yeh, J., Baehner, F. L., Fevr, T., et al. (2006). A collection of breast cancer cell lines for the study of functionally distinct cancer subtypes. Cancer Cell 10, 515–527. doi: 10.1016/j.ccr.2006.10.008
Nik-Zainal, S., Davies, H., Staaf, J., Ramakrishna, M., Glodzik, D., Zou, X., et al. (2016). Landscape of somatic mutations in 560 breast cancer whole-genome sequences. Nature 534:47. doi: 10.1038/nature17676
Omenn, G. S. (2014). The strategy, organization, and progress of the HUPO human proteome project. J. Proteomics 100, 3–7. doi: 10.1016/j.jprot.2013.10.012
Park, S. T., and Kim, J. (2016). Trends in Next-generation sequencing and a new Era for whole genome sequencing. Int. Neurourol. J. 20, S76–S83.
Pernas, S., Tolaney, S. M., Winer, E. P., and Goel, S. (2018). CDK4/6 inhibition in breast cancer: current practice and future directions. Ther. Adv. Med. Oncol. 10:1758835918786451.
Pfefferle, A. D., Herschkowitz, J. I., Usary, J., Harrell, J. C., Spike, B. T., Adams, J. R., et al. (2013). Transcriptomic classification of genetically engineered mouse models of breast cancer identifies human subtype counterparts. Genome Biol. 14:R125. doi: 10.1186/gb-2013-14-11-r125
Pierobon, M., Petricoin, E. F., and Wulfkuhle, J. D. (2018). Phosphoprotein-based drug target activation mapping for precision oncology: a view to the future. Expert Rev. Proteom. 15, 851–853. doi: 10.1080/14789450.2018.1531709
Pinker, K., Chin, J., Melsaether, A. N., Morris, E. A., and Moy, L. (2018). Precision medicine and radiogenomics in breast cancer: new approaches toward diagnosis and treatment. Radiology 287, 732–747. doi: 10.1148/radiol.2018172171
Playdon, M. C., Ziegler, R. G., Sampson, J. N., Stolzenberg-Solomon, R., Thompson, H. J., Irwin, M. L., et al. (2017). Nutritional metabolomics and breast cancer risk in a prospective study. Am. J. Clin. Nutr. 106, 637–649. doi: 10.3945/ajcn.116.150912
Raso, C., Cosentino, C., Gaspari, M., Malara, N., Han, X., McClatchy, D., et al. (2012). Characterization of breast cancer interstitial fluids by TmT labeling, LTQ-Orbitrap Velos mass spectrometry, and pathway analysis. J. Proteome Res. 11, 3199–3210. doi: 10.1021/pr2012347
Rosato, R. R., Davila-Gonzalez, D., Choi, D. S., Qian, W., Chen, W., Kozielski, A. J., et al. (2018). Evaluation of anti-PD-1-based therapy against triple-negative breast cancer patient-derived xenograft tumors engrafted in humanized mouse models. Breast Cancer Res. 20:108. doi: 10.1186/s13058-018-1037-4
Rudnick, P. A., Markey, S. P., Roth, J., Mirokhin, Y., Yan, X., Tchekhovskoi, D. V., et al. (2016). A description of the clinical proteomic tumor analysis consortium (CPTAC) common data analysis pipeline. J Proteome Res. 15, 1023–1032. doi: 10.1021/acs.jproteome.5b01091
Saadatmand, S., Bretveld, R., Siesling, S., and Tilanus-Linthorst, M. M. A. (2015). Influence of tumour stage at breast cancer detection on survival in modern times: population based study in 173 797 patients. BMJ 351:h4901. doi: 10.1136/bmj.h4901
Sachs, N., de Ligt, J., Kopper, O., Gogola, E., Bounova, G., Weeber, F., et al. (2018). A living biobank of breast cancer organoids captures disease heterogeneity. Cell 172, 373.e10–386.e10. doi: 10.1016/j.cell.2017.11.010
Saha Roy, S., and Vadlamudi, R. K. (2012). Role of estrogen receptor signaling in breast cancer metastasis. Int. J. Breast Cancer 2012, 8.
Schmid, P., Adams, S., Rugo, H. S., Schneeweiss, A., Barrios, C. H., Iwata, H., et al. (2018). Atezolizumab and nab-paclitaxel in advanced triple-negative breast cancer. N. Engl. J. Med. 379, 2108–2121. doi: 10.1056/NEJMoa1809615
Sefrioui, D., Perdrix, A., Sarafan-Vasseur, N., Dolfus, C., Dujon, A., Picquenot, J. M., et al. (2015). Short report: Monitoring ESR1 mutations by circulating tumor DNA in aromatase inhibitor resistant metastatic breast cancer. Int. J. Cancer 137, 2513–2519. doi: 10.1002/ijc.29612
Silva, C., Perestrelo, R., Silva, P., Tomas, H., and Camara, J. S. (2019). Breast cancer metabolomics: from analytical platforms to multivariate data analysis. a review. Metabolites 9:102. doi: 10.3390/metabo9050102
Sørlie, T., Perou, C. M., Tibshirani, R., Aas, T., Geisler, S., Johnsen, H., et al. (2001). Gene expression patterns of breast carcinomas distinguish tumor subclasses with clinical implications. Proc. Natl. Acad. Sci. U.S.A. 98, 10869–10874. doi: 10.1073/pnas.191367098
Speake, C., Presnell, S., Domico, K., Zeitner, B., Bjork, A., Anderson, D., et al. (2015). An interactive web application for the dissemination of human systems immunology data. J. Transl. Med. 13:196. doi: 10.1186/s12967-015-0541-x
Stender, J. D., Frasor, J., Komm, B., Chang, K. C., Kraus, W. L., and Katzenellenbogen, B. S. (2007). Estrogen-regulated gene networks in human breast cancer cells: involvement of E2F1 in the regulation of cell proliferation. Mol. Endocrinol. 21, 2112–2123. doi: 10.1210/me.2006-0474
Stephens, P. J., Tarpey, P. S., Davies, H., Van Loo, P., Greenman, C., Wedge, D. C., et al. (2012). The landscape of cancer genes and mutational processes in breast cancer. Nature 486, 400–404. doi: 10.1038/nature11017
Tate, J. G., Bamford, S., Jubb, H. C., Sondka, Z., Beare, D. M., Bindal, N., et al. (2019). COSMIC: the catalogue of somatic mutations in cancer. Nucleic Acids Res. 47, D941–D947. doi: 10.1093/nar/gky1015
Timms, J. F., White, S. L., O’Hare, M. J., and Waterfield, M. D. (2002). Effects of ErbB-2 overexpression on mitogenic signalling and cell cycle progression in human breast luminal epithelial cells. Oncogene 21, 6573–6586. doi: 10.1038/sj.onc.1205847
Tirier, S. M., Park, J., Preusser, F., Amrhein, L., Gu, Z., Steiger, S., et al. (2019). Pheno-seq - linking visual features and gene expression in 3D cell culture systems. Sci. Rep. 9:12367. doi: 10.1038/s41598-019-48771-4
Tomczak, K., Czerwinska, P., and Wiznerowicz, M. (2015). The Cancer Genome Atlas (TCGA): an immeasurable source of knowledge. Contemp. Oncol. 19, A68–A77. doi: 10.5114/wo.2014.47136
Tsoutsou, P. G., Vozenin, M. C., Durham, A. D., and Bourhis, J. (2017). How could breast cancer molecular features contribute to locoregional treatment decision making? Crit. Rev. Oncol. Hematol. 110, 43–48. doi: 10.1016/j.critrevonc.2016.12.006
Tyanova, S., Albrechtsen, R., Kronqvist, P., Cox, J., Mann, M., and Geiger, T. (2016). Proteomic maps of breast cancer subtypes. Nat. Commun. 7:10259. doi: 10.1038/ncomms10259
Varešlija, D., Priedigkeit, N., Fagan, A., Purcell, S., Cosgrove, N., O’Halloran, P. J., et al. (2018). Transcriptome characterization of matched primary breast and brain metastatic tumors to detect novel actionable targets. J. Natl. Cancer Instit. 111, 388–398. doi: 10.1093/jnci/djy110
Velasco-Velazquez, M. A., Popov, V. M., Lisanti, M. P., and Pestell, R. G. (2011). The role of breast cancer stem cells in metastasis and therapeutic implications. Am. J. Pathol. 179, 2–11. doi: 10.1016/j.ajpath.2011.03.005
Vieira, A. F., and Schmitt, F. (2018). An update on breast cancer multigene prognostic tests—emergent clinical biomarkers. Front. Med. 5:248. doi: 10.3389/fmed.2018.00248
Vikas, P., Borcherding, N., and Zhang, W. (2018). The clinical promise of immunotherapy in triple-negative breast cancer. Cancer Manag. Res. 10, 6823–6833. doi: 10.2147/CMAR.S185176
Vincent, K. M., Findlay, S. D., and Postovit, L. M. (2015). Assessing breast cancer cell lines as tumour models by comparison of mRNA expression profiles. Breast Cancer Res, 17:114. doi: 10.1186/s13058-015-0613-0
von Ahlfen, S., Missel, A., Bendrat, K., and Schlumpberger, M. (2007). Determinants of RNA quality from FFPE samples. PLoS One 2:e1261. doi: 10.1371/journal.pone.0001261
von Stechow, L., Francavilla, C., and Olsen, J. V. (2015). Recent findings and technological advances in phosphoproteomics for cells and tissues. Expert Rev. Proteomics 12, 469–487. doi: 10.1586/14789450.2015.1078730
Vu, T., and Claret, F. X. (2012). Trastuzumab: updated mechanisms of action and resistance in breast cancer. Front. Oncol. 2:62. doi: 10.3389/fonc.2012.00062
Wagner, J., Rapsomaniki, M. A., Chevrier, S., Anzeneder, T., Langwieder, C., Dykgers, A., et al. (2019). A single-cell atlas of the tumor and immune ecosystem of human breast cancer. Cell 177, 1330.e18–1345.e18. doi: 10.1016/j.cell.2019.03.005
Wahba, H. A., and El-Hadaad, H. A. (2015). Current approaches in treatment of triple-negative breast cancer. Cancer Biol. Med. 12, 106–116. doi: 10.7497/j.issn.2095-3941.2015.0030
Wakabayashi, M., Yoshihara, H., Masuda, T., Tsukahara, M., Sugiyama, N., and Ishihama, Y. (2014). Phosphoproteome analysis of formalin-fixed and paraffin-embedded tissue sections mounted on microscope slides. J. Proteome Res. 13, 915–924. doi: 10.1021/pr400960r
Weaver, V. M., Howlett, A. R., Langton-Webster, B., Petersen, O. W., and Bissell, M. J. (1995). The development of a functionally relevant cell culture model of progressive human breast cancer. Semin. Cancer Biol. 6, 175–184. doi: 10.1006/scbi.1995.0021
Weeber, F., Ooft, S. N., Dijkstra, K. K., and Voest, E. E. (2017). Tumor organoids as a pre-clinical cancer model for drug discovery. Cell Chem. Biol. 24, 1092–1100. doi: 10.1016/j.chembiol.2017.06.012
Wu, L., Shi, W., Long, J., Guo, X., Michailidou, K., Beesley, J., et al. (2018). A transcriptome-wide association study of 229,000 women identifies new candidate susceptibility genes for breast cancer. Nat. Genet. 50, 968–978. doi: 10.1038/s41588-018-0132-x
Xu, H., Lyu, X., Yi, M., Zhao, W., Song, Y., and Wu, K. (2018). Organoid technology and applications in cancer research. J. Hematol. Oncol. 11:116.
Yang, Y., Yang, H. H., Hu, Y., Watson, P. H., Liu, H., Geiger, T. R., et al. (2017). Immunocompetent mouse allograft models for development of therapies to target breast cancer metastasis. Oncotarget 8, 30621–30643. doi: 10.18632/oncotarget.15695
Yanovich, G., Agmon, H., Harel, M., Sonnenblick, A., Peretz, T., and Geiger, T. (2018). Clinical proteomics of breast cancer reveals a novel layer of breast cancer classification. Cancer Res. 78, 6001–6010. doi: 10.1158/0008-5472.CAN-18-1079
Yates, L. R., Gerstung, M., Knappskog, S., Desmedt, C., Gundem, G., Van Loo, P., et al. (2015). Subclonal diversification of primary breast cancer revealed by multiregion sequencing. Nat. Med. 21, 751–759. doi: 10.1038/nm.3886
Yates, L. R., Knappskog, S., Wedge, D., Farmery, J. H. R., Gonzalez, S., Martincorena, I., et al. (2017). Genomic evolution of breast cancer metastasis and relapse. Cancer Cell 32, 169.e7–184.e7.
Zagorac, I., Fernandez-Gaitero, S., Penning, R., Post, H., Bueno, M. J., Mouron, S., et al. (2018). In vivo phosphoproteomics reveals kinase activity profiles that predict treatment outcome in triple-negative breast cancer. Nat. Commun. 9:3501. doi: 10.1038/s41467-018-05742-z
Keywords: breast cancer, system biology, proteomics, transcriptomics, genomics, organoids, PDX
Citation: Parsons J and Francavilla C (2020) ‘Omics Approaches to Explore the Breast Cancer Landscape. Front. Cell Dev. Biol. 7:395. doi: 10.3389/fcell.2019.00395
Received: 30 October 2019; Accepted: 30 December 2019;
Published: 22 January 2020.
Edited by:
Zuzana Koledova, Masaryk University, CzechiaReviewed by:
Sajib Chakraborty, University of Dhaka, BangladeshCopyright © 2020 Parsons and Francavilla. This is an open-access article distributed under the terms of the Creative Commons Attribution License (CC BY). The use, distribution or reproduction in other forums is permitted, provided the original author(s) and the copyright owner(s) are credited and that the original publication in this journal is cited, in accordance with accepted academic practice. No use, distribution or reproduction is permitted which does not comply with these terms.
*Correspondence: Chiara Francavilla, Y2hpYXJhLmZyYW5jYXZpbGxhQG1hbmNoZXN0ZXIuYWMudWs=
Disclaimer: All claims expressed in this article are solely those of the authors and do not necessarily represent those of their affiliated organizations, or those of the publisher, the editors and the reviewers. Any product that may be evaluated in this article or claim that may be made by its manufacturer is not guaranteed or endorsed by the publisher.
Research integrity at Frontiers
Learn more about the work of our research integrity team to safeguard the quality of each article we publish.