- 1Engineering School of Sustainable Infrastructure and Environment, University of Florida, Gainesville, FL, United States
- 2Civil and Environmental Engineering, Clarkson University, Potsdam, NY, United States
The Natural Hazards Engineering Research Infrastructure (NHERI) experimental facility at the University of Florida provides a diverse suite of experimental resources to support wind hazard research. The 40-m long Boundary Layer Wind Tunnel (BLWT) simulates boundary layer flows to characterize wind loading on rigid structural models and assess the response of aeroelastic structures. The use of experimental automation tools provides researchers unparalleled flexibility in their test configurations while supporting high throughput testing and data collection. The Terraformer, an array of 1116 roughness elements, can be rapidly reconfigured to generate terrain conditions in less than 90 s, the test section turntable can move automatically through a range of wind approach angles, and the instrumentation gantry can traverse preset paths to collect wind field measurements anywhere in the tunnel cross-section. These test automation tools, along with mechatronic structural models and real-time data transfer and processing, provide new opportunities in experimental wind tunnel testing. Recent cyber-physical wind tunnel testing projects highlight the benefits of these experimental automation tools. This paper will also discuss the most recent addition to the BLWT, the Flow Field Modulator (FFM). It consists of a 2D array of 319 individually controlled ducted fans driven by electronic speed controllers. The FFM expands the BLWT capabilities by supporting the simulation of non-monotonic profiles and non-stationary events such as gust fronts and downbursts, where mean velocity and turbulence distributions change over short spatial scales. The ability to simulate these flow conditions in a wind tunnel enables the investigation of a wide range of damaging conditions and the solutions for mitigating their impact on structures.
Introduction
The Natural Hazards Engineering Research Infrastructure, funded by the National Science Foundation (NSF), provides a geographically distributed network of shared use experimental resources for conducting natural hazards research. NSF’s investment in the experimental facilities (EFs) that house these resources enables their use by researchers that may not otherwise have access to such facilities, thereby broadening participation in natural hazards experimental research. The University of Florida (UF) NHERI EF offers experimental resources that support a range of wind hazard research activities. These resources provide unique testing capabilities and represent the state-of-the-art in experimental wind engineering. The UF EF is intended to facilitate research that provides a better understanding of how meteorological phenomena translate to loads on buildings and other infrastructure and how these loads may be best mitigated to improve the performance and enhance the safety of the built environment. The data generated by the NSF-supported researchers that use the UF EF are published and may be reused by the research community to further the NHERI mission and extend the outcomes of the research beyond the initial experiments. Beyond research outcomes, the UF EF is also dedicated to inspiring the next generation of wind hazard researchers through education and outreach activities that engage K-12 students and their educators, undergraduate and graduate students, and underrepresented members of the natural hazard research community.
This paper provides an overview of the experimental resources and services offered by the UF EF; however, the focus is on the enhanced experimental capabilities of the BLWT that are enabled by advances in automation and control. Two research projects are described that highlight the BLWT capabilities.
UF Experimental Facility Overview
The UF EF broadly supports researchers in the natural hazards community. Users have access to a diverse suite of wind engineering experimental research infrastructure; enhanced testing capabilities afforded by integrated high-performance computing; skilled and resourceful personnel that promote organization, productivity, and a culture of safety and collegiality; and effective and easy-to-use technologies to collaborate, document and share findings.
The UF EF is housed in the Powell Family Structures and Material Laboratory at the University of Florida. The EF offers five experimental resources for wind engineering research. Investigators can characterize loading on and dynamic response of a wide range of infrastructure in a large, reconfigurable boundary layer wind tunnel (BLWT) and conduct full-scale tests on large building systems with equipment capable of ultimate/collapse loads associated with a Saffir-Simpson Hurricane Wind Scale Category 5 hurricane or an Enhanced Fujita Scale 5 tornado.
Experimental Resources
The Self-Configuring Hybrid Boundary Layer Wind Tunnel (BLWT) offers a unique automated terrain roughness element system (Terraformer) which can reconfigure within minutes to achieve desired approach flow conditions over a large range of geometric scales. Furthermore, the Flow Field Modulator adds non-stationary capabilities to the BLWT. Detailed descriptions of the BLWT, the Terraformer, and the Flow Field Modulator, are provided in section “Boundary Layer Wind Tunnel.”
The remaining four experimental resources offered by the UF EF are the Multi-Axis Wind Load Simulator (MAWLS), the Dynamic Flow Simulator (DFS), the High Airflow Pressure Loading Actuator (HAPLA), and Spatiotemporal Pressure Loading Actuator (SPLA). The MAWLS is a pressure loading actuator that accommodates full size wall and cladding specimens for destructive testing. It applies time-varying out-of-plane pressure to simulate loads associated with buffeting or separated flow, while simultaneously applying static in-plane uplift or shear. The DFS is a high-speed wind tunnel that recreates building surface flows. The HAPLA accommodates moderately sized specimens that can be quickly interchanged and can impart dynamic wind pressure and simulate impinging rain conditions. The SPLA can rotate into a horizontal or vertical position and has the ability to simulate spatially varying pressure conditions by applying synchronized pressure loading to four reconfigurable chambers that span the specimen. All EF apparatus are interconnected in the lab through a high-speed network; thus, making it possible to characterize wind loading or velocity in the BLWT and instantly converting and applying those measurements as full-scale inputs to MAWLS, DFS, HAPLA, or SPLA.
Science Plan
Current policymaking and research trends signal that design, construction, and operation of civil infrastructure and lifelines will rapidly advance through the next few decades. Reliability-based design approaches that underpin current codes, regulations and engineering standards will mature into a resilience-based decision framework that establishes new performance goals for community functioning pre- and post-disaster. Hazard-centric design approaches will further homogenize (e.g., ASCE 7–10 shifting wind loading provisioning into alignment with its seismic counterpart) and collectively evolve into multihazard engineering guidelines that optimize the lifecycle cost of infrastructure. Concurrent adoption of sustainable design practices will influence this process, leading to long-term performance expectations for infrastructure as it physically ages and undergoes changes caused by operation and exposure to weather. Design tools will rapidly advance, shifting toward automation with an increasing reliance on machine learning and other AI-based agents to reduce cost, construction time, and environmental impact. Robotics, prefabrication, and additive manufacturing will become more widespread. Humans and machines will work with lighter, stronger, and greener materials, while application of conventional materials continue to be honed to overcome the technical limitations of today. The future of civil infrastructure presents multiple grand challenges and informs the science plan that guides the resources and services offered by the UF EF.
The UF EF science plan identified seven broad objectives, each of which embodies a grand challenge: (1) Reduce uncertainties in the wind loading chain, especially those related to predicting peak loads and structural response, (2) Advance computational wind engineering and reduce reliance on physical testing, (3) Develop methodologies that reliably predict performance as a function of building age and use, (4) Advance the state of knowledge regarding collapse limit state fragilities, (5) Advance automation and design of hazard resistant infrastructure, (6) Introduce high-performance and greener material, and (7) Find innovative and cost-effective solutions to retrofit existing infrastructure.
User Services
The UF EF offers users a range of services to support the development, execution, and dissemination of their research. The EF PIs and staff are available to discuss experimental ideas, address the feasibility and refinement of experimental plans, identify appropriate co-PIs and assist in the preparation of proposals and budgets for submission to NSF. Once projects are funded, the EF PIs and staff work with users to refine and schedule their test plans, assess instrumentation needs, design a data collection and transfer plan, provide project oversight, ensure safety compliance, and implement risk management. The UF EF has staff dedicated to the various aspects of experimental planning and execution with expertise in: wind engineering testing and analysis, instrumentation and controls, civil/mechanical design and fabrication, and cyberinfrastructure and information technology. Multiple 3D printers, a 3-axis CNC router and a fully staffed machine shop are available at the EF to assist in the design and fabrication of models and customized test rigs. The facility manages the construction, staging, transport, and disposition of test specimens.
Boundary Layer Wind Tunnel
Background and Overview
BLWTs have served as important experimental tools in wind engineering research since the early 1960s (Cermak, 2003) and currently provide insight into the complex fluid motions observed around bluff bodies immersed in turbulent boundary layers – phenomena that are, in many cases, poorly captured by theoretical and computational fluid dynamics. Conventional BLWTs simulate natural winds in the first few hundred meters of the earth’s atmospheric boundary layer (ABL) at reduced geometric scales on the order of 1:50–1:400. To achieve similarity with full-scale ABL flows, air is forced through a large duct with a floor-mounted roughness element array to generate mechanical turbulence similar to the kind produced by buildings, trees, and other elements in the earth’s landscape (Cermak, 1982; Cook, 1982). This process naturally grows a boundary layer along the length of the tunnel’s development section to produce the desired mean velocity, turbulence intensity, power spectral density, and integral length scales at a downwind test section. The geometric dimensions, density and configuration of roughness elements in the development section are traditionally manually arranged to produce target approach flow conditions. Trips, spires, turbulence grids, and strakes are installed upwind of the roughness elements to artificially introduce large-scale motions and to promote mixing when required. A geometrically scaled model of the test subject installed on a turntable in the downwind test section is instrumented to record pressure, acceleration, displacement or base reaction data.
The UF EF BLWT infrastructure incorporates many features of traditional facilities and several key innovations. It is a long-fetch low-speed open circuit tunnel with dimensions of 6 m W × 3 m H × 38 m L (see Figure 1). Eight Aerovent 54D5 VJ vaneaxial fans driven by 75 hp (56 kW) AC induction motors – configured in a 2 × 4 array – generate axial flow, which is pre-conditioned by a nested honeycomb system to reduce fan-generated turbulence and ensure horizontal homogeneity of the velocity profile before entering the development section. A set of removable Irwin spires (Irwin, 1981) – i.e., vortex generators – are situated at the beginning of the development section to induce large scale mixing. The wind tunnel ceiling pitch is adjustable to produce approximately zero static pressure gradient flows (∂p/∂x≅0) along the length of the development and test sections – measured by an array of wall-mounted static tube and differential pressure transducer assemblies. Test section air temperature, humidity, and barometric pressure are monitored using a ceiling-mounted Omega iBTHX sensor centrally located above a mechanized turntable that can be rotated through 360 degrees to achieve user-specified wind directions. On this location, scale models are installed, instrumented, and tested. All tunnel systems are integrated into a command and control center with data collection and tunnel control computers.
Turbulent flow fields in the tunnel are measured using an automated multi-degree-of-freedom instrument gantry capable of traversing longitudinally, laterally, and vertically nearly the entire length, height, and width of the test section. The system is equipped with several fast-response four-hole Turbulent Flow Instrumentation (TFI) Series 100 Cobra Velocity Probes to capture discrete point measurements of u, v, and w fluctuating velocity components and static pressure within a ±45° acceptance cone (Tfi Catalogue, 2015). The gantry is also fitted with a Dantec Dynamics stereoscopic particle image velocimetry (PIV) system – a high-energy laser and high-speed camera assembly – that is actuated on linear guides for positioning over user-defined near-surface measurement locations. The gantry system is designed to minimize test section blockage at 4.63%. A bank of 10 fluid atomizers (i.e., seeders) is installed upwind of the test section to entrain 1–2 micron oil particles in the flow. Double frame images from two high-speed cameras – one on each side of an emitted laser light sheet – capture particle motion to produce time-resolved 3-D flow field measurements. Rigid body surface pressure monitoring is achieved through a 512 channel Scanivalve pressure scanning system. Rigid body base shears and overturning moment are measured using one of two ATI Industrial Automation six-axis load cells.
Data collected at the UF EF are stored at the High Performance Computing Center (HiPerGator supercomputer), which serves as the primary working storage for projects. Data are automatically transmitted to the DesignSafe CyberInfrastructure in the NHERI network. All hardware is connected through a 1 Gb/s dedicated high-volume data transfer network exclusively operated to support experimental and computational research. Data are curated using DesignSafe’s curation features including built-in support for the extraction and tagging of technical metadata from data files, aiding in the creation of well-documented datasets.
Terraformer
Unique to the UF EF, the Terraformer (Figures 1, 2) automates the roughness grid arrangement process by replacing hand-built terrains with a computer controlled terrain generator that can quickly change its configuration. The fully integrated system is an array of 1116 electronically actuated roughness element assemblies that independently rotate and translate to precisely control height and aspect ratio as shown in Figure 2C. Hardware for the assemblies include the Nanotec Linear Actuator LS4118S1404-T6 × 1–230, Motor Controller SMCI12, rectangular aluminum element, rotating disk, baseplate, supporting rods, slide, nylon sleeves, and endcaps. This system can configure the frontal projected area of each element over a continuous range between a minimum of 0 mm2 to a maximum of 16,256 mm2 in under 2 min. The individual elements have nominal lateral dimensions of 102 mm × 51 mm and a maximum actuated height of 160 mm yielding a range of drag coefficients from ∼0.97 to 1.21. The Terraformer is intended to represent approach terrain in the general sense of achieving desired flow parameters (e.g., profile, roughness coefficient, scale). The representation of particular landscape features such as individual buildings or trees surround the test subject can be achieved using the on-site fabrication facilities described in section “User Services.”
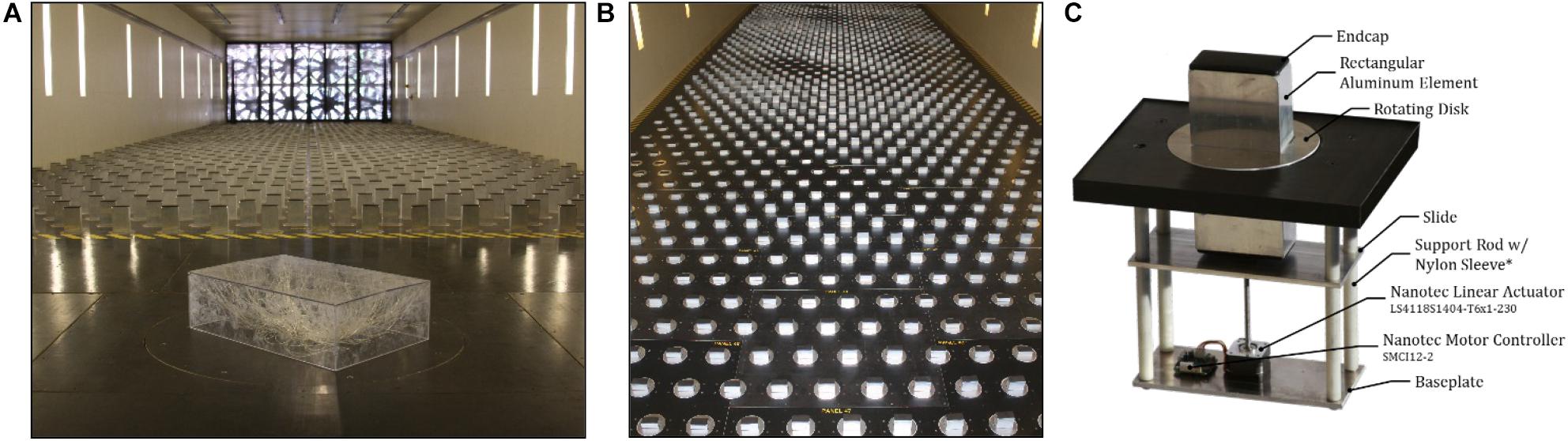
Figure 2. The Terraformer: (A) test subject installed in the foreground and roughness element field with elements uniformly actuated in the background; (B) heterogeneous terrain; and (C) annotated individual element assembly.
Uniform actuation of the system (Figure 2A) – for the production of traditional homogeneous obstacle arrays – was calibrated using a predictive model that relates the morphometric properties of the roughness element grid to the aerodynamic roughness parameters (Catarelli et al., 2020). This provides a deterministic solution enabling the tunnel to accurately produce user-specified upwind target exposures for a range of model scales with minimal configuration time. The independently actuating roughness elements are also capable of configuring discrete random fields to simulate heterogeneous upwind terrain conditions as shown in Figure 2B. Control software was developed to model terrain based on stochastic simulation techniques from prescribed Fourier-based models and probabilistic targets.
Validation studies of the wind field and pressure monitoring system have recently been published. These include floor to freestream mean and turbulence profiles for 33 homogeneous terrain configurations (Fernández-Cabán and Masters, 2018a; Catarelli et al., 2020), ESDU target and measured longitudinal velocity spectra (Fernández-Cabán and Masters, 2018a, 2020; Fernández-Cabán et al., 2018), comparisons of measured bluff body pressure coefficients with NIST database benchmarks (Ho et al., 2003; Fernández-Cabán et al., 2018; Fernández-Cabán and Masters, 2020), and the sensitivity of pressure coefficients on a NIST benchmark building to fine changes in approach terrain (Fernández-Cabán and Masters, 2018a).
Flow Field Modulator
Modern wind tunnels have a limited ability to precisely and rapidly recreate complex wind fields associated with extreme weather, e.g., gust fronts and downbursts that exhibit non-monotonic mean velocity profiles and non-stationary amplitude and frequency components (Kwon and Kareem, 2009). These departures from traditional stationary straight-line wind models may alter the spatial distribution of instantaneous loads over the surface of a structure and thus impact structural vulnerability and habitability (Kijewski-Correa and Bentz, 2011; Nguyen and Manuel, 2013). Physical simulation techniques have been developed in proof-of-concept studies for turbulent flow control (e.g., Cao et al., 2002; Smith et al., 2012), which differ from standard BLWT simulations in that multi-fan arrays are used to generate user-specified mean velocity and turbulence characteristics downwind of a mixing region.
Building upon this prior work, the multi-fan Flow Field Modulator (Figure 3) was developed as a high resolution flow control device and integrated into the upwind portion of the tunnel to simulate complex extreme wind phenomena including non-monotonic mean velocity profiles, non-stationary flow properties, and transient flows. It is designed to operate in series with the existing vaneaxial fan bank (i.e., primary fan system) and consists of a computer controlled 2D array of 319 modular hexagonal aluminum cells containing shrouded three-blade corotating propeller pairs with high-performance 800 Watt brushless DC motors driven by electronic speed controllers (Figure 3). This hardware configuration permits a maximum frequency response of approximately 3 Hz, a maximum free discharge velocity of 23 m/s with the primary fan system running at full power, and a peak instantaneous flow acceleration of 100 m/s2. Individual cell assemblies incorporate pitot-static tube velocity monitoring and open-loop control. Closed-loop control of the individual cells is under development. The FFM 319 fan bank is located immediately upwind of the dimensionally identical 319 cell honeycomb shown in Figure 1. The FFM is mounted on a track system, allowing easy installation in or removal from the BLWT as needed.
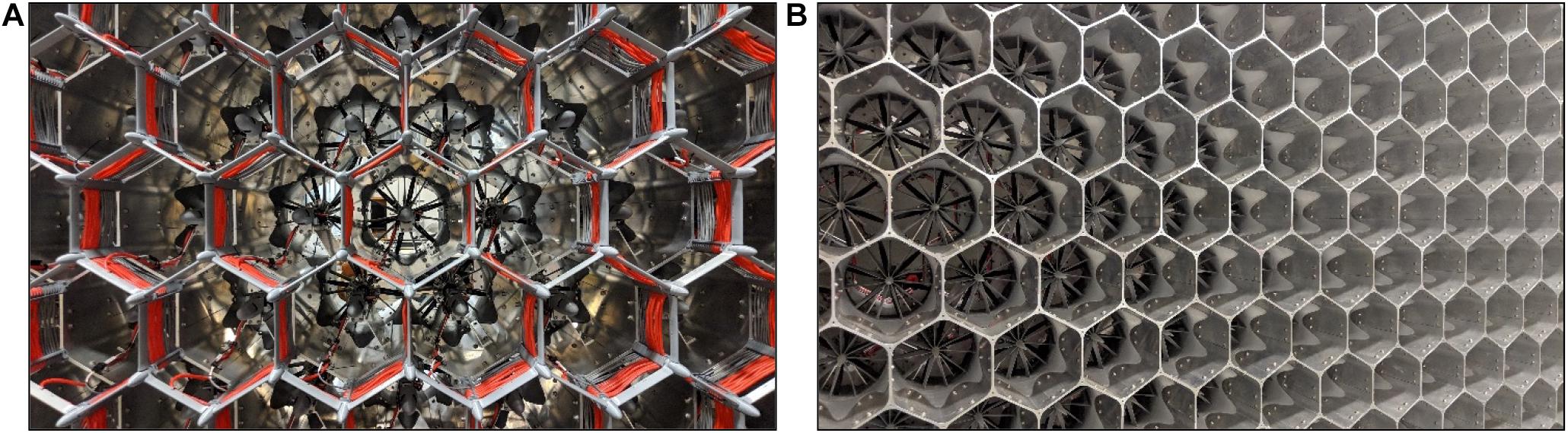
Figure 3. The Flow Field Modulator. Array of 319 modular hexagonal aluminum cells: (A) upwind face; (B) downwind face.
Variations in mean fan speeds of cell rows impart vertically stratified steady flows – mean velocity profiles – along the height of the tunnel, accounting for free shear layer interactions, as well as frictional and orifice losses. Individual instantaneous fan speeds are capable of fluctuating according to stochastic simulations (e.g., reproduced velocity time histories) based on target vertical, across, and along-wind integral length scales to achieve desired turbulence properties at the end of a mixing region downwind. Additional lateral and vertical turbulence can be introduced by shifting the phases of adjacent cells, decorrelating the gust structure.
The current status of validation and performance of the FFM for simulation of non-monotonic profiles (Vicroy, 1992) and non-stationary gusts (Balderrama et al., 2011) can be found in Catarelli (2019).
Limitations
The previous sections describe unique capabilities that facilitate high throughput and expand the breadth of wind phenomena that can be simulated. We now round out the facility description with a discussion of current known limitations of the UF EF.
The strength of this wind tunnel facility is the precise control and measurement of the flow field. For this reason, the BLWT is not a destructive testing apparatus (e.g., Chowdhury et al., 2017). The windspeed is nominally limited to 20 m/s freestream. Typical tests include rigid pressure-tapped models, rigid high-frequency base balance models, and aeroelastic models. Other equipment at UF, including the multi-axis wind load simulator, dynamic flow simulator, high airflow pressure loading actuator, and spatiotemporal pressure loading actuator, provide destructive testing capabilities. The flow limits on the FFM include a maximum frequency response is approximately 3 Hz, maximum instantaneous velocity is 23 m/s, and maximum instantaneous flow acceleration is 100 m/s2.
The Terraformer is limited to the representation of homogeneous or heterogeneous upwind terrain in a general sense. If needed, detailed proximity models can be added to capture the impact of discrete near-field terrain features. Based on the range of possible roughness element frontal areas, the Terraformer can create upwind terrain conditions for model scales ranging from approximately 1:20 (e.g., a low-rise building) to 1:3100 (e.g., a topographic model). At the larger model scales, additional flow conditioning devices (or the FFM) may be required to create the appropriate low-frequency turbulence.
The PIV provides time-correlated 3D flow measurements within the viewing window, however it comes with some limitations. The PIV viewing window is limited to 150 mm in the x-axis and 215 mm in the z-axis, so multiple sample windows may be required to cover larger areas. Measurements will not be time correlated across windows. The window can be positioned at any x-position in the test section and at any y-position within ±50 mm of the center of the test section (coordinate system shown in Figure 1). The BLWT is open circuit, requiring a baffle at the exit for safety when running the PIV laser. This baffle increases the static pressure in the tunnel and has a small impact on other flow characteristics, all of which can be characterized. Additionally, special model preparations may be needed to avoid damage under the high-power laser.
Example Research Projects
Two recent projects are highlighted to demonstrate the automated, high throughput capabilities of the UF EF BLWT.
Cyber-Physical Design and Optimization in the Wind Tunnel
Cyber-physical systems link the real world to the cyber-world by leveraging the ability of computers to monitor and control physical systems. Components include sensors, actuators, data communication, computers for executing numerical models or algorithms, and a physical phenomenon of interest. In wind engineering, advances in cyber-physical systems coupled with UF EF’s push for automated and high-throughput testing offer the unprecedented opportunity to integrate BLWT modeling into the engineering design process.
Researchers at the University of Maryland (UMD) and UF pioneered the first cyber-physical systems approach to the optimal design of structures subjected to wind hazards. This approach combines the accuracy of physical wind tunnel testing with the efficient exploration of the design space using numerical optimization algorithms. The approach is fully automated, with experiments executed in the BLWT, sensor feedback monitored by a computer, and actuators used to bring about changes to a mechatronic building model as dictated by the optimization algorithm. The model undergoes physical changes in dynamics or aerodynamics as it approaches the optimal solution, accurately capturing the impacts of these changes on its response. As part of the project, cyber-infrastructure was developed to seamlessly connect the BLWT operations (e.g., turntable angle, metadata collection), instrumentation (triggering and data transfer), specimen actuation, data post-processing, finite element modeling, and optimization algorithms. The interconnectivity was critical to creating an automated high-throughput system that, once set up, would drive the specimen to the optimal design given user-specified objectives and constraints.
Proof-of-concept was demonstrated for a low-rise building model with a parapet wall of variable height (Whiteman et al., 2018a, b). Parapet walls alter the location of the roof corner vortices, reducing suction loads on the windward facing roof corners and edges and setting up an interesting optimal design problem. In the BLWT, the parapet height was actuated using servo-motors (see Figure 4). Exploration of the design space was conducted using multi-objective heuristic optimization algorithms to achieve a design that minimized loads on both components and cladding and the main wind force resisting system. The optimal design repeatedly and quickly converged, and the influence of the objectives and constraints were clearly seen in the results.
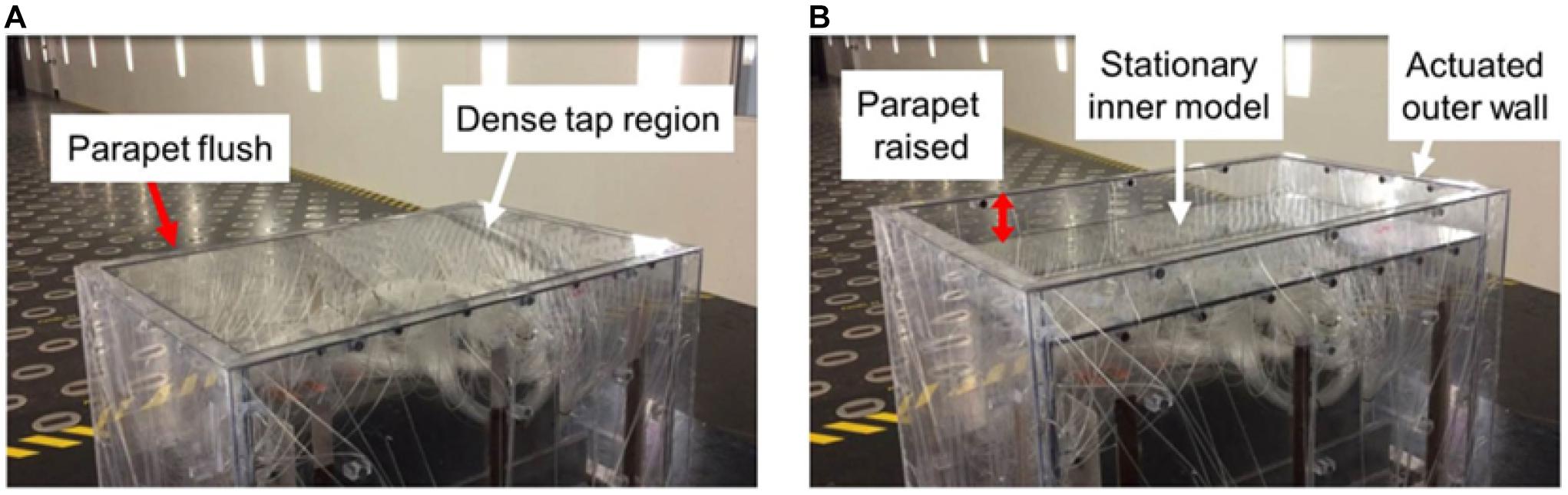
Figure 4. (A) Building model with a flush parapet wall and (B) a raised parapet wall (from Whiteman et al., 2018a under the Creative Commons CC BY license).
Further studies focused on tall building design, for which a 1.5 m 1:200 scale multi-degree-of-freedom aeroelastic model was created (Fernández-Cabán et al., 2020; see Figure 5). Aeroelastic models directly simulate the scaled dynamic behavior of the building including effects of aerodynamic damping, vortex shedding, coupling within modes, and higher modes. The model’s responses were monitored using accelerometers and displacement transducers. The model was equipped with a series of variable stiffness devices (adjustable leaf springs) in the base connected to internal steel tendons to enable quick and automated adjustments to the model’s dynamics. Additionally, the model had a set of mechanized corner fins to tune the aerodynamic shape. The fins were designed to reduce vortex-induced vibration and can be adjusted based on windspeed and direction to keep occupants safe and comfortable. Multiple design problems were explored where the model’s dynamics and aerodynamics were refined using heuristic optimization algorithms to minimize costs while satisfying acceleration and drift limits.
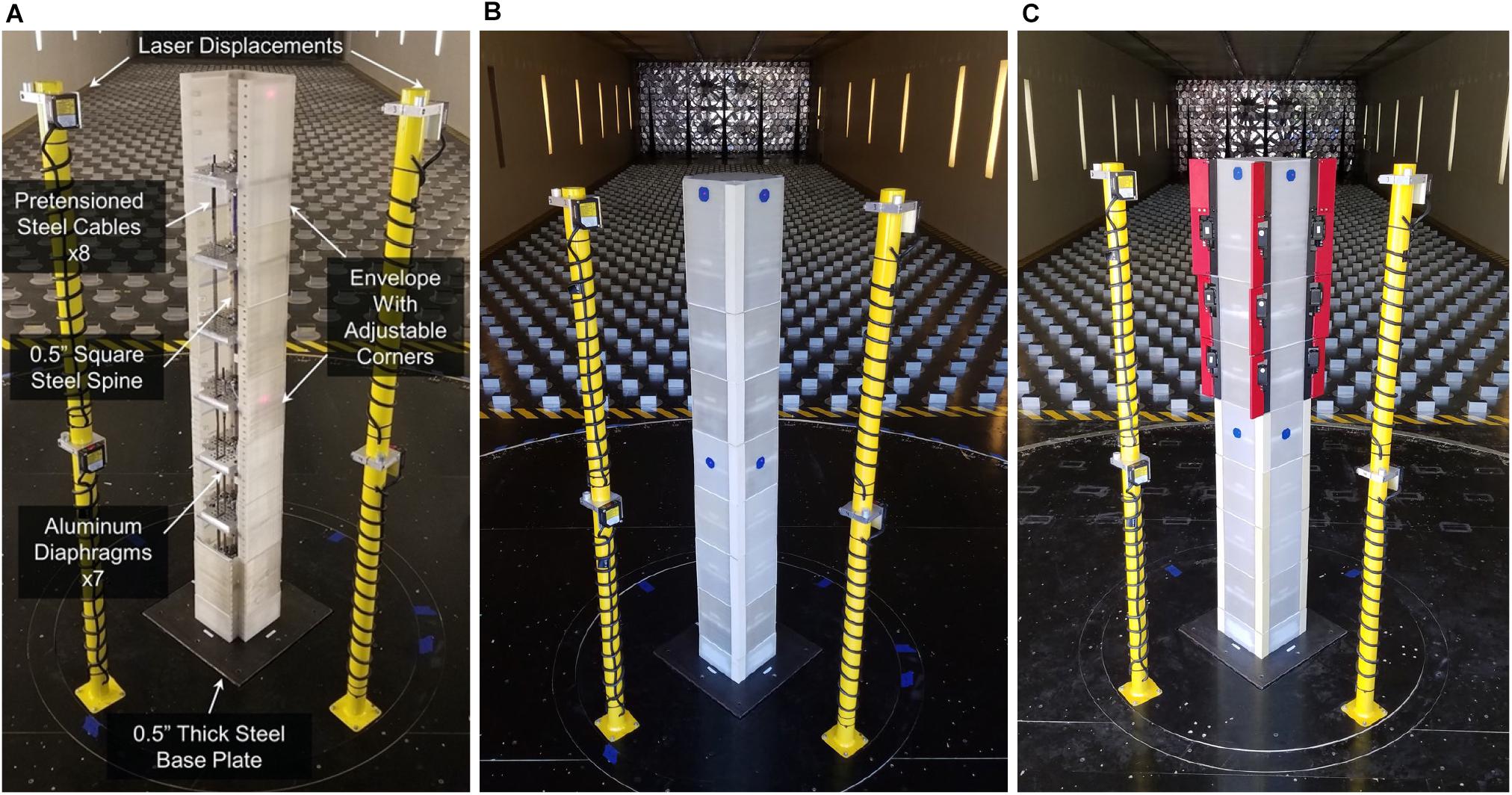
Figure 5. 1.5 m tall multi-degree-of-freedom aeroelastic model: (A) inner mechanics exposed, (B) enclosed model without fins, and (C) enclosed model with fins.
This project advances the capacity to build stronger, lighter, and more resilient structures in the face of wind hazards. The traditional design process requires a lengthy collaboration between designers and wind tunnel operators. This process may include the construction of a very limited set of building models, leading to a non-exhaustive exploration of potential designs. The use of scaled building models with physically adjustable properties (e.g., geometry, stiffness, damping, etc.) allows optimum designs to be attained faster than conventional methods and eliminates the need to reconstruct new models and perform additional wind tunnel tests. Mechatronic specimens connected to cyber-infrastructure greatly enhances the capacity of BLWT facilities. In wind engineering, cyber-physical design enables engineers to more exhaustively explore a range of candidate designs and replace trial-and-error approaches with automation.
Characterization and Prediction of Upstream Terrain Effects on Wind Pressure Loading
Previous research in wind tunnels (e.g., Gartshore, 1973; Hillier and Cherry, 1981; Saathoff and Melbourne, 1997) have revealed a strong linkage between the surface pressure field acting on sharp-edged bluff bodies (e.g., low-rise buildings) and the turbulent characteristics of freestream approach flows. In particular, the intensity and distribution of wind-induced loads developed in regions experiencing extreme suction pressures, such as roof edges/corners of buildings, are highly sensitive to mechanical turbulence largely driven by the morphometric features of the upstream terrain. The Terraformer provides unique capabilities to rapidly and accurately control upstream turbulent flow conditions in the BLWT, enabling prompt quantification of pressure loading on civil infrastructure for a wide range of upwind terrain conditions (e.g., a continuum between marine to dense suburban exposures).
A holistic assessment of upstream terrain effects was performed at the UF NHERI EF through a series of aerodynamic BLWT experiments on three scaled (1:20, 1:30, and 1:50) low-rise building models. Geometric building properties (i.e., plan dimensions, roof eave height, and roof slope) for the three models were based on full-scale dimensions of the Wind Engineering Research Field Laboratory (WERFL) experimental building, which is located at Texas Tech University (Levitan and Mehta, 1992). The three WERFL models were tested under 33 unique turbulent boundary layer flows through fine adjustment of the Terraformer roughness element grid. The initial series of baseline aerodynamic tests only employ homogeneous (i.e., uniform) roughness element arrays. That is, in each test, all 1116 roughness elements were set to the same height and orientation. Homogeneous terrains consisted of the bare floor (i.e., “flush,” h = 0 mm) case, 16 element heights (h = 10–160 mm using 10 mm increments) and two element orientations namely wide and narrow edge windward. For each upwind terrain configuration, surface pressures were recorded by 266 pressure taps located on the roof and walls of the low-rise models. Figure 6 includes pressure coefficient contours of standard deviation for the 1:20 WERFL model for three representative roughness element heights (h = 10, 80, and 160 mm).
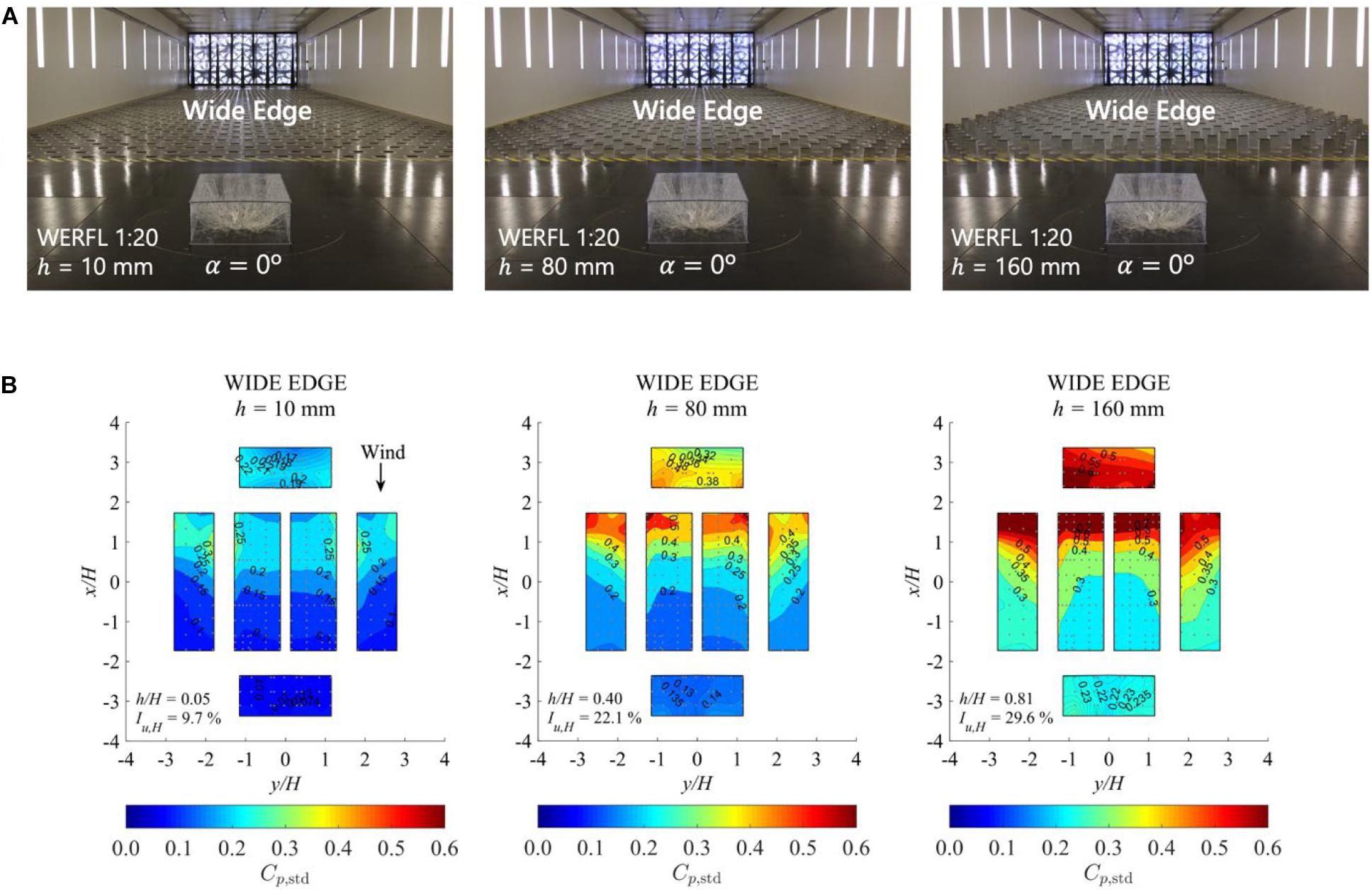
Figure 6. (A) Aerodynamic BLWT tests on the 1:20 WERFL model for three homogeneous Terraformer configurations (h = 10, 80, and 160 mm); (B) pressure coefficient contours of standard deviation (from Fernández-Cabán and Masters, 2018a under the Creative Commons CC BY license).
Clear distinctions are observed in both the magnitude and distribution of the wind pressure field for the three cases shown in Figure 6, particularly near the leading windward edge of the roof. Similar pressure distributions were obtained for the 1:50 and 1:30 WERFL models. However, scale dependencies were detected when comparing the magnitude of the pressures under similar freestream turbulence flow conditions, which may be attributed to Reynolds number discrepancies in the BLWT (Lim et al., 2007). Therefore, the data are well suited for further examination of scaling effects in the BLWT when testing low building models (Stathopoulos and Surry, 1983). The complete aerodynamic BLWT dataset is publicly accessible in the DesignSafe-CI repository (Fernández-Cabán and Masters, 2018b, 2020). The data can be re-used to validate computational fluid dynamic models (e.g., LES) and train machine learning algorithms such as artificial neural networks (ANN; see Figure 7) to develop analytical tools for predicting wind-induced loads on low-rise structures (Fernández-Cabán et al., 2018; Tian et al., 2020).
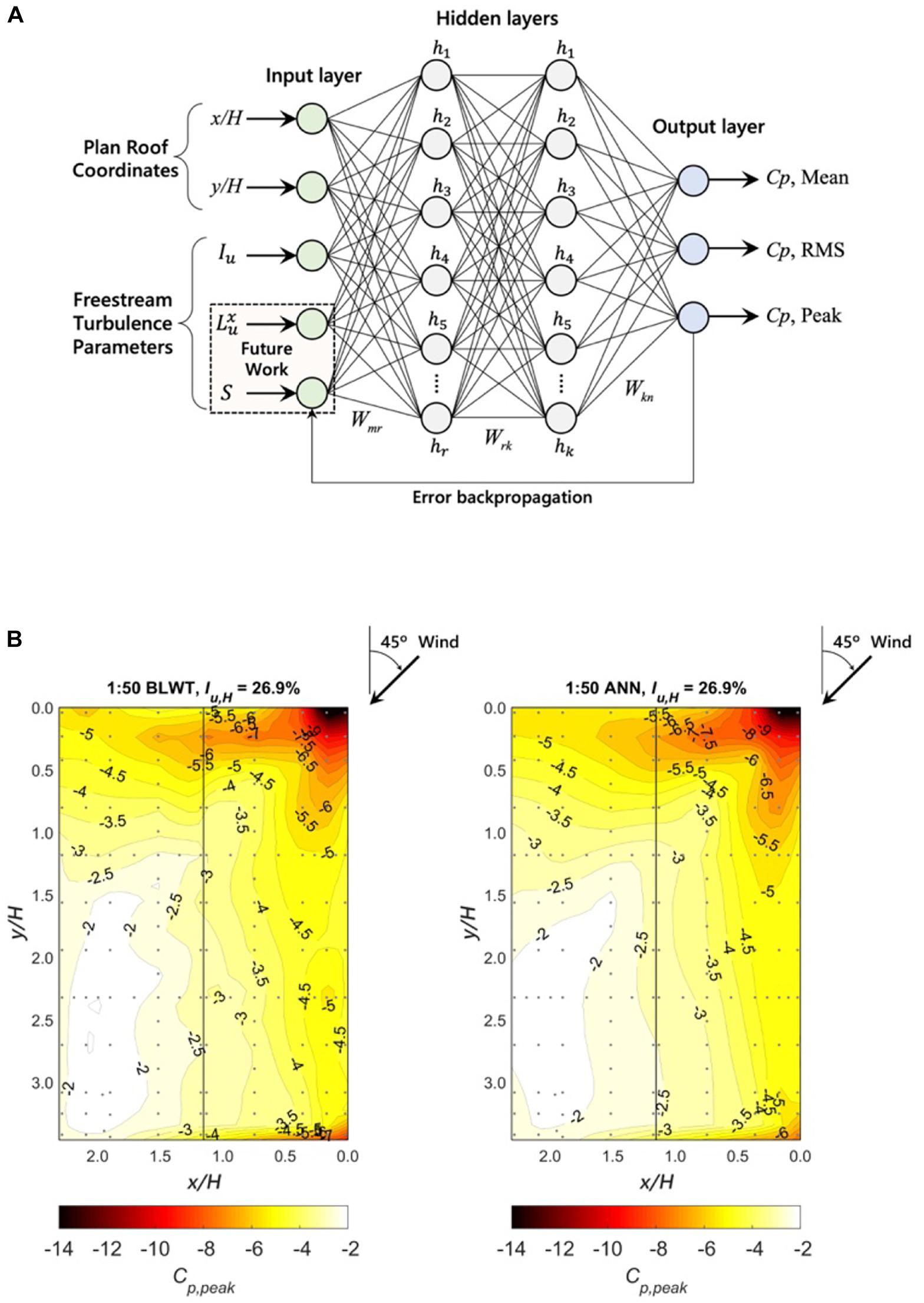
Figure 7. (A) Architecture of an artificial neural network (ANN) for wind pressure prediction accounting for turbulence parameters. (B) Peak pressure contours of the 1:50 WERFL model: BLWT experimental data (left) and ANN model prediction (right) (from Fernández-Cabán et al., 2018 under the Creative Commons CC BY license).
Both the holistic quantification of the influence of upwind terrain and the development of the ANN model required a large dataset enabled by high-throughput testing. The three BLWT models were each evaluated under 33 unique turbulent boundary layer flows at three angles of attack for a total of almost 300 experiments. Automation in the Terraformer, turntable, and data transfer made it possible to complete all tests within four working days. It is estimated that without the Terraformer, it would take an order of magnitude longer to complete the same test matrix.
Summary and Conclusion
As part of the NSF NHERI network, the UF EF offers five shared use resources that support a range of wind hazard research activities. The research supported by the UF EF provides a better understanding of how meteorological phenomena translate to loads on buildings and other infrastructure and how these loads may be best mitigated to improve the performance and enhance the safety of the built environment. The focus of this paper is on the enhanced experimental capabilities of the Self-Configuring Hybrid BLWT, which simulates boundary layer flows to characterize wind loading on rigid structural models and assess the response of aeroelastic structures. The development of unique automation tools provides researchers unparalleled flexibility in their test configurations while supporting high throughput testing and data collection. Terrain roughness can be rapidly reconfigured to alter approach flow turbulence, and the instrumentation gantry can traverse preset paths to collect wind field measurements anywhere in the tunnel cross-section. The Flow Field Modulator adds non-stationary and non-monotonic boundary layer capabilities to the BLWT. These test automation tools, along with mechatronic structural models and real-time data transfer and processing, provide new opportunities in experimental wind tunnel testing.
Two recent projects are highlighted to demonstrate the automated, high throughput capabilities of the UF EF BLWT. In wind engineering, advances in cyber-physical systems coupled with UF EF’s push for automated and high-throughput testing offer the unprecedented opportunity to integrate BLWT modeling into the engineering design process. Cyber-infrastructure was developed to seamlessly connect the BLWT operations (e.g., turntable angle, metadata collection), instrumentation (triggering and data transfer), specimen actuation, data post-processing, finite element modeling, and optimization algorithms. The interconnectivity was critical to creating an automated high-throughput system that would drive the specimen to the optimal design given user specified objectives and constraints. The rapidly reconfigurable and automated Terraformer facilitated a study of pressure sensitivity to approach flow with unprecedented resolution, illuminating the limitations of the current standard of coarsely discretized terrain characterization.
More information about the NHERI UF EF can be found at https://ufl.designsafe-ci.org/ and through contact with the corresponding author.
Data Availability Statement
Publicly available datasets were analyzed in this study. This data can be found here: doi: 10.17603/DS2W670.
Author Contributions
All authors listed have made a substantial, direct and intellectual contribution to the work, and approved it for publication.
Funding
The University of Florida NHERI Experimental Facility was supported by a grant from the National Science Foundation, Award No. CMMI 1520843. Research discussed herein was also supported by the NSF Award Nos. CMMI 1636039 and CMMI 0928563.
Conflict of Interest
The authors declare that the research was conducted in the absence of any commercial or financial relationships that could be construed as a potential conflict of interest.
Acknowledgments
We wish to recognize the Powell Structures and Materials Laboratory staff, with special thanks to Jon Sinnreich, Steve Schein, Eric Agostinelli, Kevin Stultz, Shelby Brothers, and Scott Powell for their contribution in wind tunnel testing. We would also like to acknowledge Michael Whiteman of the University of Maryland and Justin Davis of the University of Florida for their contributions to the cyber-physical testing. Any opinions, findings, and conclusions or recommendations expressed in this manuscript are those of the authors and do not necessarily reflect the views of the sponsors, partners, and contributors.
References
Balderrama, J. A., Masters, F. J., Gurley, K. R., Prevatt, D. O., Aponte-Bermúdez, L. D., Reinhold, T. A., et al. (2011). The Florida Coastal Monitoring Program (FCMP): a review. J. Wind Eng. Ind. Aerodynamics 99, 979–995. doi: 10.1016/j.jweia.2011.07.002
Cao, S., Nishi, A., Kikugawa, H., and Matsuda, Y. (2002). Reproduction of wind velocity history in a multiple fan wind tunnel. J. Wind Eng. Ind. Aerodynamics 90, 1719–1729. doi: 10.1016/s0167-6105(02)00282-9
Catarelli, R. A. (2019). Enhancing the Modalities of Boundary Layer Wind Tunnel Modeling and Experimental Flow Simulation Over Complex Topography. Gainesville, FL: University of Florida. Ph.D. Dissertation.
Catarelli, R. A., Fernaìndez-Cabaìn, P. L., Masters, F. J., Bridge, J. A., Gurley, K. R., and Matyas, C. J. (2020). Automated terrain generation for precise atmospheric boundary layer simulation in the wind tunnel. J. Wind Eng. Ind. Aerodynamics
Cermak, J. E. (1982). “Physical modeling of the atmospheric boundary layer (ABL) in long boundary-layer wind tunnels (BLWT),” in Proceedings of the International Workshop on Wind Tunnel Modeling Criteria and Techniques in Civil Engineering Applications. Gaithersburg: Cambridge University Press, 97–125.
Cermak, J. E. (2003). Wind-tunnel development and trends in applications to civil engineering. J. Wind Eng. Ind. Aerodynamics 91, 355–370. doi: 10.1016/s0167-6105(02)00396-3
Chowdhury, A. G., Zisis, I., Irwin, P., Bitsuamlak, G., Pinelli, J.-P., Hajra, B., et al. (2017). Large scale experimentation using the 12-Fan wall of wind to assess and mitigate hurricane wind and rain impacts on buildings and infrastructure systems. ASCE J. Struct. Eng. 143:04017053. doi: 10.1061/(ASCE)ST.1943-541X.0001785
Cook, N. J. (1982). “Simulation techniques for short test-section wind tunnels: roughness, barrier and mixing-device methods,” in Proceedings of the International Workshop on Wind Tunnel Modeling Criteria and Techniques in Civil Engineering Applications. Gaithersburg: Cambridge University Press, 126–136.
Fernández-Cabán, P. L., and Masters, F. J. (2018a). Effects of freestream turbulence on the pressure acting on a low-rise building roof in the separated flow region. Front. Built Environ. 4:17. doi: 10.3389/fbuil.2018.00017
Fernández-Cabán, P. L., and Masters, F. J. (2018b). Upwind terrain effects on low- rise building pressure loading observed in the boundary layer wind tunnel. DesignSafe-CI doi: 10.17603/DS2W670
Fernández-Cabán, P. L., and Masters, F. J. (2020). Experiments in a large boundary layer wind tunnel: upstream terrain effects on surface pressures acting on a low-rise structure. J. Struct. Eng. ASCE 20:146. doi: 10.1061/(ASCE)ST.1943-541X.0002690
Fernández-Cabán, P. L., Masters, F. J., and Phillips, B. M. (2018). Predicting roof pressures on a low-rise structure from freestream turbulence using artificial neural networks. Front. Built Environ. 4:68. doi: 10.3389/fbuil.2018.00068
Fernández-Cabán, P. L., Whiteman, M. L., Phillips, B. M., Masters, F. J., Davis, J. R., and Bridge, J. A. (2020). Cyber-physical design and optimization of tall building dynamics using aeroelastic wind tunnel modeling. J. Wind Eng. Ind. Aerodynamics 198:104092. doi: 10.1016/j.jweia.2020.104092
Gartshore, I. S. (1973). The Effects of Free Stream Turbulence on the Drag of Rectangular Two-Dimensional Prisms. London, ON: University of Western Ontario, Faculty of Engineering Science, Boundary Layer Wind Tunnel Laboratory.
Hillier, R., and Cherry, N. J. (1981). The effects of stream turbulence on separation bubbles. J. Wind Eng. Ind. Aerodynamics 8, 49–58. doi: 10.1016/0167-6105(81)90007-6
Ho, T. C. E., Surry, D., and Morrish, D. P. (2003). NIST/TTU Cooperative Agreement–Windstorm Mitigation Initiative: Wind Tunnel Experiments on Generic Low Buildings. London, ON: The Boundary Layer Wind Tunnel Laboratory, The University of Western Ontario.
Irwin, H. P. A. H. (1981). The design of spires for wind simulation. J. Wind Eng. Ind. Aerodynamics 7, 361–366. doi: 10.1016/0167-6105(81)90058-1
Kijewski-Correa, T., and Bentz, A. (2011). Wind-induced vibrations of buildings: role of transient events. Proc. Institution Civil Eng. Struct. Build. 164, 273–284. doi: 10.1680/stbu.2011.164.4.273
Kwon, D., and Kareem, A. (2009). Gust-front factor: new framework for wind load effects on structures. J. Struct. Eng. ASCE 135, 717–732. doi: 10.1061/(asce)0733-9445(2009)135:6(717)
Levitan, M. L., and Mehta, K. C. (1992). Texas tech field experiments for wind loads part 1: building and pressure measuring system. J. Wind Eng. Ind. Aerodynamics 43, 1565–1576. doi: 10.1016/0167-6105(92)90372-h
Lim, H. C., Castro, I. P., and Hoxey, R. P. (2007). Bluff bodies in deep turbulent boundary layers: reynolds-number issues. J. Fluid Mechan. 571, 97–118. doi: 10.1017/s0022112006003223
Nguyen, H. H., and Manuel, L. (2013). Thunderstorm downburst risks to wind farms. J. Renew. Sustain. Energy 5:013120. doi: 10.1063/1.4792497
Saathoff, P. J., and Melbourne, W. H. (1997). Effects of free-stream turbulence on surface pressure fluctuations in a separation bubble. J. Fluid Mechan. 337, 1–24. doi: 10.1017/s0022112096004594
Smith, J. T., Masters, F. J., Liu, Z., and Reinhold, T. A. (2012). A simplified approach to simulate prescribed boundary layer flow conditions in a multiple controlled fan wind tunnel. J. Wind Eng. and Ind. Aerodynamics 109, 79–88. doi: 10.1016/j.jweia.2012.04.010
Stathopoulos, T., and Surry, D. (1983). Scale effects in wind tunnel testing of low buildings. J. Wind Eng. Ind. Aerodynamics 13, 313–326. doi: 10.1016/b978-0-444-42340-5.50038-5
Tfi Catalogue (2015). Catalogue Section - Cobra Probe. http://www.turbulentflow.com.au/Downloads/Cat_CobraProbe.pdf (accessed August 21, 2020).
Tian, J., Gurley, K. R., Diaz, M. T., Fernández-Cabán, P. L., Masters, F. J., and Fang, R. (2020). Low-rise gable roof buildings pressure prediction using deep neural networks. J. Wind Eng. Ind. Aerodynamics 196:104026. doi: 10.1016/j.jweia.2019.104026
Vicroy, D. D. (1992). Assessment of microburst models for downdraft estimation. J. Aircraft 29, 1043–1048. doi: 10.2514/3.46282
Whiteman, M. L., Fernández-Cabán, P. L., Phillips, B. M., Masters, F. J., Bridge, J. A., and Davis, J. R. (2018a). Multi-objective optimal design of a building envelope and structural system using cyber-physical modeling in a wind tunnel. Front. Built Environ. 4:13. doi: 10.3389/fbuil.2018.00013
Keywords: NHERI, boundary layer wind tunnel, experimental wind engineering, structural optimization, automation
Citation: Catarelli RA, Fernández-Cabán PL, Phillips BM, Bridge JA, Masters FJ, Gurley KR and Prevatt DO (2020) Automation and New Capabilities in the University of Florida NHERI Boundary Layer Wind Tunnel. Front. Built Environ. 6:558151. doi: 10.3389/fbuil.2020.558151
Received: 01 May 2020; Accepted: 28 August 2020;
Published: 16 September 2020.
Edited by:
Franklin Lombardo, University of Illinois at Urbana-Champaign, United StatesReviewed by:
Haitham Aboshosha, Ryerson University, CanadaTanya Michelle Brown-Giammanco, Insurance Institute for Business & Home Safety, United States
Copyright © 2020 Catarelli, Fernández-Cabán, Phillips, Bridge, Masters, Gurley and Prevatt. This is an open-access article distributed under the terms of the Creative Commons Attribution License (CC BY). The use, distribution or reproduction in other forums is permitted, provided the original author(s) and the copyright owner(s) are credited and that the original publication in this journal is cited, in accordance with accepted academic practice. No use, distribution or reproduction is permitted which does not comply with these terms.
*Correspondence: Kurtis R. Gurley, a2d1cmxAY2UudWZsLmVkdQ==