- CAS Key Laboratory of Quantitative Engineering Biology, Shenzhen Institute of Synthetic Biology, Shenzhen Institutes of Advanced Technology, Chinese Academy of Sciences, Shenzhen, China
Saccharomyces cerevisiae is a widely used eukaryotic model and microbial cell factory. RNA interference (RNAi) is a conserved regulatory mechanism among eukaryotes but absent from S. cerevisiae. Recent reconstitution of RNAi machinery in S. cerevisiae enables the use of this powerful tool for strain engineering. Here we first discuss the introduction of heterologous RNAi pathways in S. cerevisiae, and the design of various expression cassettes of RNAi precursor reagents for tunable, dynamic, and genome-wide regulation. We then summarize notable examples of RNAi-assisted functional genomics and metabolic engineering studies in S. cerevisiae. We conclude with the future challenges and opportunities of RNAi-based approaches, as well as the potential of other regulatory RNAs in advancing yeast engineering.
Introduction
As a unicellular eukaryotic microorganism, S. cerevisiae has become one of the most widely used microbial cell factory in the production of value-added chemicals, biofuels and biopharmaceuticals (Liu et al., 2013; Nielsen, 2019). S. cerevisiae has many desirable traits for industrial fermentation. With a long history in baking and brewing, S. cerevisiae is generally recognized as safe (GRAS). Compared with prokaryotes such as E. coli, S. cerevisiae possesses multiple organelles providing physical compartments for diverse biochemical reactions; it is also capable of post-translational modifications, which are required for heterologous synthesis of complex proteins (Tokmakov et al., 2012). S. cerevisiae is physiologically stable and highly robust toward harsh industrial conditions such as low pH, high osmotic pressure, and toxic inhibitors (Hong and Nielsen, 2012; Kavscek et al., 2015). Due to its popularity in fundamental and applied research, versatile tools have been developed in S. cerevisiae for genetic manipulation (Si et al., 2015b; Lian et al., 2018; Jiang et al., 2019) and bioprocess development (Hasunuma and Kondo, 2012; Tripathi and Shrivastava, 2019).
RNA interference (RNAi) is a post-transcriptional, gene-silencing mechanism broadly distributed in eukaryotic organisms. RNAi mediates many essential biological processes, including defense against viruses and transposons, maintenance of chromosome and genome integrity, and cellular differentiation and development (Castel and Martienssen, 2013; Gutbrod and Martienssen, 2020). Various organisms comprise different mechanisms for RNAi, but the basic process shared three common steps. First, small interfering RNA (siRNA) duplexes of 21–25 nucleotides are generated from long double-stranded RNA (dsRNA) precursors by a ribonuclease enzyme called Dicer. Second, siRNAs are loaded into Argonaute proteins to form a protein-RNA complex known as RNA-induced silencing complex (RISC), where the guide and passenger strands of siRNA are dissociated. Third, RISC finds and cleaves the cognate mRNA molecule, whose sequence is homologous to the siRNA loaded in the complex (Hannon, 2002; Wilson and Doudna, 2013; Ipsaro and Joshua-Tor, 2015). Thanks to its facile implementation and high specificity, RNAi has been widely used to knock down individual genes of interest, as well as to perform genome-wide reduction-of-function screening, since its first discovery in Caenorhabditis elegans (Fire et al., 1998; Agrawal et al., 2003; Boutros and Ahringer, 2008).
RNA interference is conserved in almost all eukaryotic organisms including fungi, plants and animals (Agrawal et al., 2003; Gutbrod and Martienssen, 2020), but it is evolutionarily lost in S. cerevisiae, possibly due to the incompatibility with a beneficial dsRNA “killer virus” (Drinnenberg et al., 2009, 2011). This theory is supported by the ecological significance of “killer viruses” (Boynton, 2019) and the importance of RNAi in antiviral defense (Li et al., 2013; Waldron et al., 2018). However, a non-canonical RNAi pathway exists in other budding yeasts, including Saccharomyces castellii, Candida albicans, and Kluyveromyces polysporus (Drinnenberg et al., 2009). Unlike canonical Dicers, which generate siRNAs of regular sizes from dsRNA termini, budding-yeast Dicers start processing in the interior of a dsRNA and work outward, with product size determined by the distance between neighboring active sites (Weinberg et al., 2011; Wilson and Doudna, 2013). On the other hand, other yeasts contain canonical RNAi machinery, including the fission yeast Schizosaccharomyces pombe (Volpe et al., 2002) and a human pathogenic yeast Cryptococcus neoformans (Loftus et al., 2005).
Since the discovery and characterization of the budding yeast pathways, RNAi has become an attractive platform for gene regulation in S. cerevisiae. Here we summarize the reconstitution of heterologous RNAi pathways in baker’s yeast and the design of RNAi cassettes for desirable potency and specificity. We also provide notable examples in implementing RNA-based engineering tools for tunable, dynamic, and genome-scale gene modulation in S. cerevisiae. To conclude, the future directions of RNAi-based tools and the potentials of other regulatory RNAs in S. cerevisiae strain engineering are discussed.
Tool Development
RNAi Pathway Reconstitution
Heterologous RNAi pathways from S. castelli and human were successfully reconstituted in S. cerevisiae. To evaluate RNAi effectiveness and efficiency, fluorescent proteins were commonly utilized as reporters (Drinnenberg et al., 2009; Suk et al., 2011; Crook et al., 2014; Si et al., 2014; Purcell et al., 2018; Kildegaard et al., 2019). For the S. castelli pathway, robust repression of green fluorescent protein (GFP) signals were observed when both DCR1 and AGO1 were present, but DCR1 alone was sufficient to generate GFP siRNAs in S. cerevisiae (Drinnenberg et al., 2009). On the other hand, all three components of the human RISC complex, Dicer, Argonaute-2 (Ago2), and HIV-1 transactivating response (TAR) RNA-binding protein (TRBP) (Gregory et al., 2005; Maniataki and Mourelatos, 2005), are necessary to reconstitute functional RNAi in S. cerevisiae (Suk et al., 2011). This difference between S. castelli and human pathways is probably because human TRBP is required to mediate interaction of Ago2 and siRNA bounded by Dicer, whereas budding yeast Dicers act as homodimers and did not require additional dsRBD domains (Chendrimada et al., 2005; Weinberg et al., 2011).
Due to evolutionary closeness and simplicity, the S. castellii RNAi pathway is commonly employed in S. cerevisiae (Drinnenberg et al., 2009; Crook et al., 2014; Si et al., 2014; Williams et al., 2015b; Purcell et al., 2018; Wang et al., 2019; Figure 1A). S. castellii Dicer and Argonaute were expressed either from a low-copy (centromeric) plasmid (Crook et al., 2014, 2016; Lee et al., 2016) or a single, integrated locus in the yeast genome (Drinnenberg et al., 2009; Si et al., 2014, 2017; Xiao and Zhao, 2014; Williams et al., 2015a, b; Purcell et al., 2018; Wang et al., 2019; Figure 1A and Table 1). To enable efficient gene silencing, gene expression of Dicer and Argonaute was driven by strong constitutive promoters, such as PTEF1, PGPD1, PTPI1, and PPGK1. Although no comprehensive comparisons have been performed among different expression formats, it is suggested that constitutive expression driven by a strong promoter from a genomic locus is sufficient for efficient RNAi silencing.
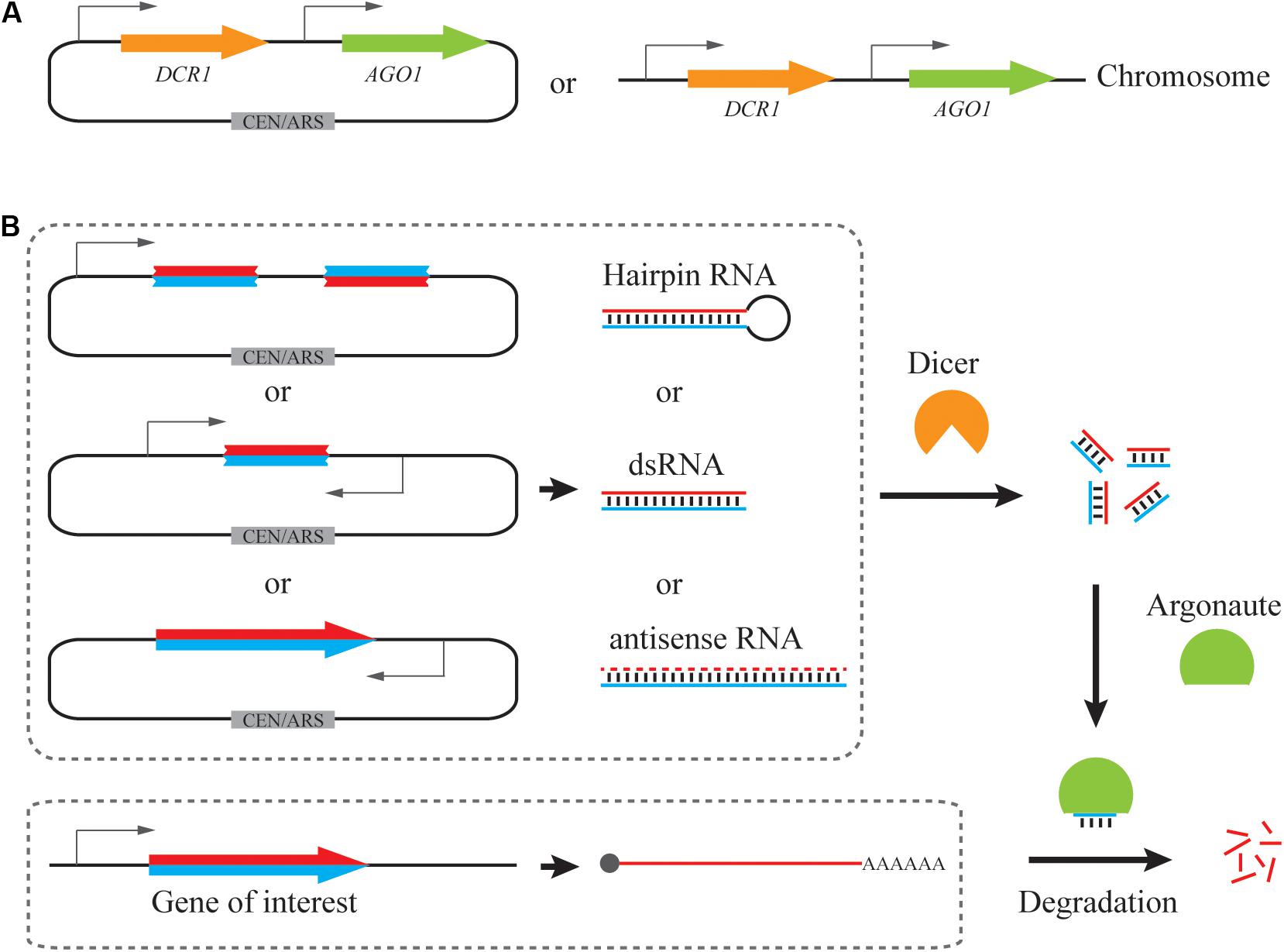
Figure 1. Reconstitution of a synthetic RNAi pathway in S. cerevisiae. (A) Expression cassettes of RNAi pathway. DCR1 and AGO1 from S. castellii are more commonly utilized, being cloned into a low-copy plasmid or integrated into the genome. (B) Expression cassettes of siRNA precursors. Hairpin RNA, dsRNA and full-length antisense RNA have been used for generating siRNAs by Dicer. siRNA duplexes mediate mRNA degradation and hence repression of a target gene with the help of Argonaute.
RNAi Reagent Cassette Design
In addition to RNAi pathways, RNAi precursor is another essential component for efficient gene silencing. We will limit our discussions to the design of RNAi reagent cassettes for the S. castellii RNAi pathway, which is widely employed for RNAi reconstitution in S. cerevisiae. In its native host, S. castellii siRNAs are originated from hairpin RNAs, which are transcribed from inverted genomic repeats and fold back on their own paired regions (Drinnenberg et al., 2009). These RNA hairpins were presumed to be composed of stems of 100–400 bp and loops ranging from 19 to >1600 nt (Drinnenberg et al., 2009). As expected, when co-expressed with S. castellii Dcr1 and Ago1 in S. cerevisiae, the hairpin RNA construct with inverted repeats of a 275 bp GFP fragment induced strong reduction of GFP mRNA and fluorescence levels (Drinnenberg et al., 2009). In addition to hairpin RNAs (Drinnenberg et al., 2009; Crook et al., 2014; Purcell et al., 2018), other forms of RNAi precursors, such as dsRNAs (Si et al., 2014; Crook et al., 2016) and antisense RNAs (Suk et al., 2011; Si et al., 2014, 2017), can also be accepted by the S. castellii RNA machinery for gene repression in S. cerevisiae. Notably, although a growing collection of computational tools is available to design RNAi targeting sequences, these tools are primarily developed for canonical RNAi pathways as recently summarized (Lagana et al., 2014; Jain and Wadhwa, 2018). Given the mechanistic differences of siRNA generation between canonical and budding-yeast RNAi machinery, it remains elusive if existing algorithms may help to identify RNAi targets for efficient gene silencing in S. cerevisiae. Here, we will focus on how different designs of expression cassettes may result in various levels of RNAi silencing (Table 1).
Hairpin RNA
The design of hairpin RNA cassettes, such as promoter, length of hairpin RNAs, and plasmid copy number, has a major impact on RNAi silencing efficiency (Table 1). To state a general observation, a low-copy auxotrophic plasmid expressing long hairpin by a strong promoter produces a potent RNAi reagent.
To investigate the impact of promoter strength, Crook et al. engineered reporter strains expressing yellow fluorescence protein (YFP) from a strong or weak promoter, which could mimic the different expression levels of native genes. Only when the hairpin RNA was expressed from the strong PTDH3 promoter, efficient repression (up to 80%) was obtained for strongly expressed YFP (Crook et al., 2014), indicating the amount of RNAi precursor was limited in this case. Moreover, three promoters with different strength (PCYC1, PTEF1 and PTDH3) were used to express a hairpin RNA targeting ADE3, the deletion of which can improve heterologous production of itaconic acid (IA). Various IA production levels were observed with different levels of hairpin RNA expression in three S. cerevisiae strains (BY4741, CEN.PK2-a, and Sigma 10560-4A), demonstrating the necessity of promoter screening to fine-tune RNAi efficacy (Crook et al., 2014). Similarly, the strong PTDH3 promoter elicited higher repression levels relative to the weak PRNR2 promoter when targeting the endogenous ZWF1 gene using hairpin RNAi (Kildegaard et al., 2019). The observation that the promoter activity of a hairpin RNA cassette modulates its gene silencing efficiency was exploited for dynamic, context-dependent gene knockdown, whereby an inducible promoter was used to drive hairpin RNA expression (Williams et al., 2015a).
Hairpin length also affects RNAi efficiency. When the length of hairpin increased from 100 bp to 200 bp, the downregulation efficiency was improved by 30% when YFP is strongly expressed (Crook et al., 2014). Notably, when YFP was weakly expressed, the inhibition level of 200 bp hairpin was 6-fold higher than that of the 100 bp hairpin, indicating that longer hairpin length is especially important for efficient silencing of low-abundance transcripts. The potency of long (∼250 bp) RNAi hairpins in S. cerevisiae was also confirmed in later studies (Williams et al., 2015a; Kildegaard et al., 2019).
For plasmid copy number, when using an auxotrophic marker (TRP1), a low-copy, centromeric plasmid achieved higher knockdown efficiency relative to a high-copy, 2-micron vector, for both strongly and weakly expressed YFP (Crook et al., 2014). This effect, however, cannot be observed when using an antibiotic resistance marker (KanMX). Improved RNAi efficiency by low-copy, centromeric plasmid may be related to reduced cell-to-cell variability.
It is also possible to introduce synthetic RNAi targets to a native transcript. A single, invariant target sequence was inserted into the 3′ untranslated region (UTR) of a yERFP reporter gene, and repression of fluorescence signal was achieved by introducing a corresponding hairpin RNA in an RNAi capable S. cerevisiae (Purcell et al., 2018). Gene silencing efficiency can be modulated via variations in the repeat number or sequence length of targeting siRNA sequences placed in the stem structure. For example, more repeats induced stronger gene repression. The authors also demonstrated that nucleus-residing non-coding RNA can be targeted by hairpin RNAs, but it was unlikely mediated by RNAi, because RNAi effector proteins are normally expressed in cytosol. In this way, any gene can be theoretically regulated by the same RNAi precursor upon insertion of a synthetic target sequence in the UTRs, offering a graded and scalable module of gene regulation.
Long dsRNA
Long dsRNA molecules without hairpin loop structures can also be used as siRNA precursors. The dsRNA molecules can be transcribed by convergent promoters, whereby the sense and antisense transcripts are individually expressed under the control of two opposing promoters. However, it was showed that the amount of siRNA from dsRNA was much lower than from hairpin RNA precursors, leading to weaker GFP repression (Drinnenberg et al., 2009). Like hairpin RNA, strong promoters achieved higher repression than weaker promoters when driving dsRNA expression (Crook et al., 2016). To investigate the influence of dsRNA length, we generated various dsRNA constructs on a low-copy, centromeric plasmid (pRS416) targeting different regions of the GFP gene, driven by two strong, convergent promoters PTEF1 and PTPI1. Among the dsRNAs corresponding to the 1–180, 1–360, 1–540, and 1–717 bp (full length) region of the GFP gene, knockdown efficiency was inversely related to dsRNA length, with the shortest (1–180 bp) dsRNA exhibited the strongest GFP repression (80%) (Si et al., 2014). As to the vector of dsRNA cassette, although efficient downregulation was observed from a centromeric plasmid, the silencing efficiency was even higher when integrated into the genome (Si et al., 2014; Crook et al., 2016). In addition, with the introduction of an intron from Schizosaccharomyces pombe RAD9 directly downstream of convergent promoters to enhance transcriptional strength, the extent of downregulation was significantly improved for weakly expressed YFP, but insignificant for strongly expressed YFP (Crook et al., 2016). Together, similar to hairpin RNA, the use of strong promoters and low-copy vectors is desirable for dsRNA. The length of dsRNAs should be carefully optimized, and ∼200 bp was frequently used (Si et al., 2014; Crook et al., 2016; Table 1).
Antisense RNA
Antisense RNAs can act as siRNA precursors by hybridizing with endogenous transcripts to form dsRNA substrates of Dicer. We expressed the full-length, antisense GFP transcript under control of the PTEF1 promoter on an episomal plasmid. Silencing effect is negligible without a RNA pathway, and a 95% repression of GFP signal was observed in an RNAi capable strain (Si et al., 2014; Table 1). When integrated into the genome, an even higher silencing efficiency and less population heterogeneity in fluorescence profile were observed (Si et al., 2014).
Taken together, hairpin RNA, dsRNA, and antisense RNA have been employed for RNAi silencing in S. cerevisiae (Figure 1B). Upon optimization, all three forms of siRNA precursor could induce strong gene knockdown efficiency (up to 95%). However, the design of hairpin RNA and dsRNA expression cassettes need more optimization, including the length and region of the targeting sequence. In addition, hairpin RNA structures with longer inverted repeats are difficult to build and unstable during cloning, probably due to interference with DNA replication (Voineagu et al., 2008). An intron-containing gap region could help to improve plasmid stability (Drinnenberg et al., 2009; Crook et al., 2014). Alternatively, full-length antisense RNA represents a simple yet effective RNAi cassette design.
Genome-Scale Library Design
A remarkable advantage of RNAi technology is genome-wide reduction-of-function screening (Boutros and Ahringer, 2008; Mohr et al., 2014). Genome-scale coverage, knockdown efficiency, and off-target effects are important considerations when designing large-scale RNAi experiments (Boutros and Ahringer, 2008; Horn et al., 2010). For heterologous RNAi in S. cerevisiae, these characteristics are related to the form of siRNA precursors, the DNA source of RNAi cassettes, the length of inserted fragments, and the library size (Figure 2). Due to the difficulty in construction of long inverted repeats and the issue of plasmid stability, it is challenging to generate genome-wide RNAi libraries based on hairpin RNAs. Alternatively, dsRNA and antisense RNA are commonly utilized to create comprehensive RNAi libraries in S. cerevisiae.
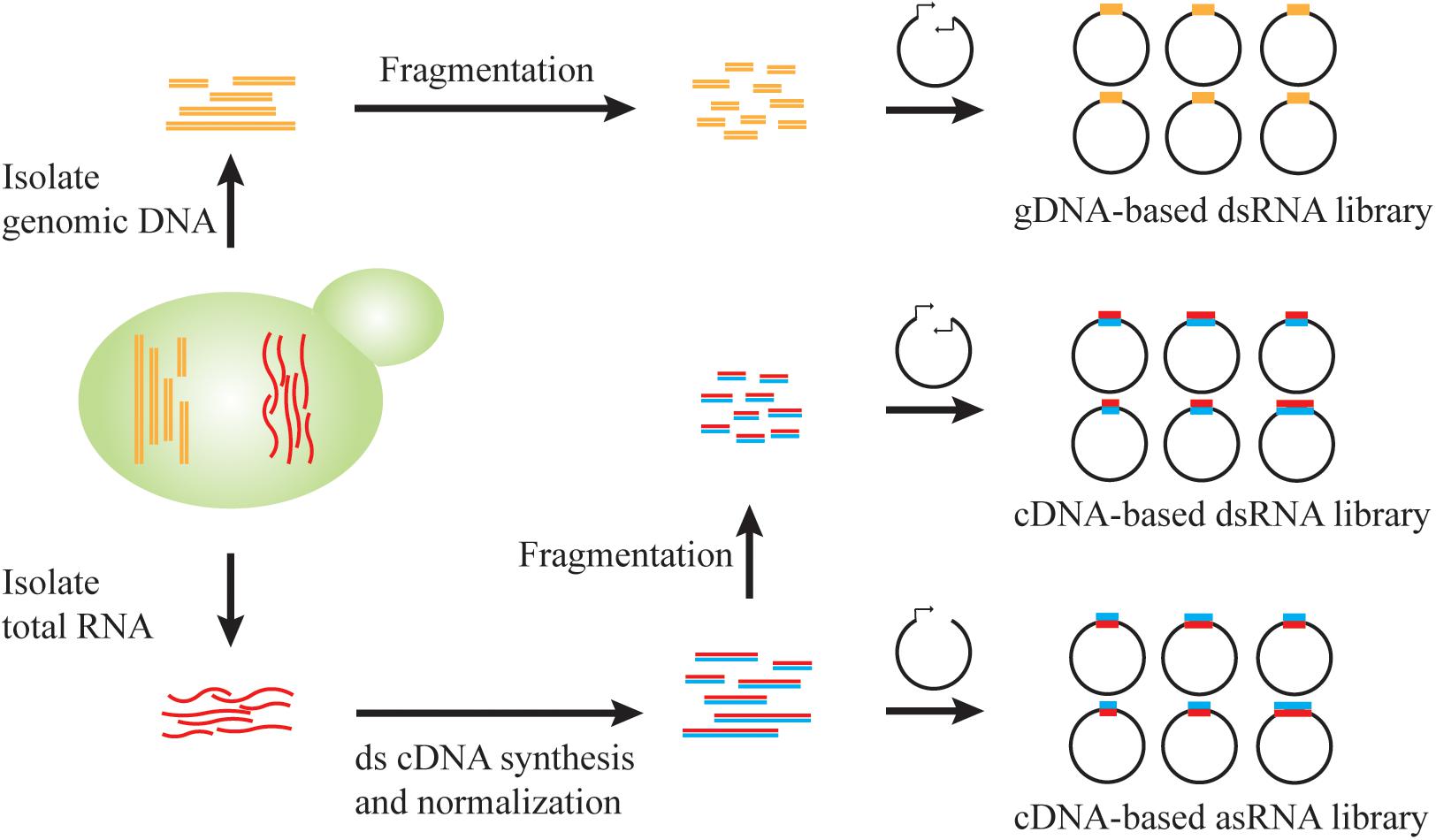
Figure 2. Genome-scale RNAi library construction in S. cerevisiae. RNAi library can be constructed from genomic DNA (gDNA) or total RNA. By cloning the fragmented genomic DNA into a vector with convergent promoters, a gDNA-based dsRNA library enables the generation of dsRNA precursors for RNAi pathway. After normalization of transcript abundance, the double-stranded cDNA could be fragmented and cloned into a vector with convergent promoters for a dsRNA library or be directionally cloned into a vector for antisense RNA transcription.
Pooled long-dsRNA libraries can be created by inserting genomic DNA or complementary DNA (cDNA) fragments between convergent promoters (Cheng and Jian, 2010; Si et al., 2014). Genomic DNA or cDNA fragments can be generated either through endonuclease-mediated, biochemical digestion, such as Sau3AI (Si et al., 2014), or using physical shearing method, such as ultrasonication (Crook et al., 2016). Fragmentation parameters should be carefully tuned to achieve a proper length distribution. For example, we previously constructed a strain library of >3 × 105 independent clones, where each clone contained ∼200 bp genomic DNA fragments. This library achieved a >5-fold redundancy of the 12 Mb yeast genome and hence >99% probability of a full genomic coverage (Si et al., 2014). Also, double-stranded cDNA were fragmented by ultrasonication to an average of 200 or 400 bp to minimize possible translation of open reading frames within dsRNA constructs (Crook et al., 2016). The resultant library contained over 105 distinct members and achieved a good coverage of yeast transcriptome (Lee et al., 2016). Notably, due to the heterogeneity of transcript abundance, cDNA normalization via selective degradation of abundant transcripts is necessary to improve genome-scale representation (Zhulidov et al., 2004; Si et al., 2017). On the other hand, genomic DNA-derived dsRNA libraries exhibit an even coverage of each gene, but such libraries will also contain non-transcribed regions that may be inefficient RNAi reagents in most cases. However, this feature may reveal unexpected RNAi targets such as tRNAs (Si et al., 2014), which are not commonly included in cDNA libraries.
Alternatively, antisense RNA libraries can be generated by cloning of cDNA molecules in the reverse direction downstream of a promoter. Based on the SMART (Switching Mechanism At 5′-end of RNA Template) mechanism (Zhu et al., 2001), two different adaptor sequences are included specifically to each end of a full-length cDNA molecule, and hence insertion direction can be controlled by arranging the homologous adaptor sequences in a desirable order in an expression cassette (Si et al., 2017). Upon cDNA normalization based on transcript abundance, a strain library of >106 independent clones achieved >92% coverage of all yeast genes, which was confirmed via next generation sequencing (NGS) analysis (Si et al., 2017). Taken together, genomic DNA and cDNA libraries can be readily sourced for generating RNAi reagents for genome-wide, reduction-of-function screening in S. cerevisiae.
Application
As one of the most widely used cell factory, S. cerevisiae is intensively engineered with different industrially relevant traits, including broadened substrate scope, improved titer, yield and productivity, and enhanced robustness (Kavscek et al., 2015; Lian et al., 2018). Due to the complexity of cellular networks, strain engineering requires concerted identification of genetic targets on a genome scale and fine-tuning of their expression with temporal precision (Si et al., 2015a; Lian et al., 2018). As a modular, scalable, and predictable tool for gene regulation, RNAi has been increasingly applied in yeast engineering, offering unique advantages such as dynamic regulation, genome-scale engineering, and multigene optimization.
Dynamic Regulation
Introduction of synthetic constructs and heterologous pathways typically causes metabolic burden, due to competition for cellular resources and accumulation of toxic intermediates and products (Keasling, 2008; Borkowski et al., 2016). To address this problem, the growth and production phases can be separated via dynamic gene regulation, which can be achieved by inducible RNAi expression.
GAL and Tet are well-characterized inducible promoters in S. cerevisiae, modulated by galactose and doxycycline, respectively (Blount et al., 2012). As a proof of concept, when a hairpin RNA cassette targeting URA3 was constructed under the control of PGAL1, addition of galactose reduced cell growth in the absence of uracil, and enabled cell growth in the presence of 5-fluoroorotic acid (5-FOA), which can be converted to a toxic product by Ura3p (Drinnenberg et al., 2009). Inducible expression of RNAi hairpins were also demonstrated using PTPGI, a promoter derived from PGAL1 and induced by galactose and anhydrotetracycline (aTc) (Purcell et al., 2018). Although dynamic RNAi regulation was achieved, the use of GAL promoters requires expensive inducers and suffers from leaky expression. Therefore, it is desirable to utilize promoters that are strongly repressed by glucose and activated by a cheap carbon source. Williams et al. found that during long-term fermentation, GFP expression from the SUC2 promoter was strongly repressed during the growth phase on glucose; once glucose concentration dropped below 5 nM, GFP expression was rapidly and strongly activated, exhibiting a dynamic range of 12.5-fold when the carbon source was switched to sucrose. Notably, dynamic repression of GFP expression was also achieved by coupling a full-length, antisense GFP cassette with the SUC2 promoter in an RNAi capable strain (Williams et al., 2015b).
Quorum sensing (QS) describes a widespread phenomenon that couples gene expression with population density (Miller and Bassler, 2001; Choudhary and Schmidt-Dannert, 2010), commonly achieved via cell-cell communications mediated by extracellular signaling molecules. QS can help to separate growth phase and production phase, when activation of target pathway genes and repression of competing metabolic activities are only initiated after sufficient biomass is accumulated. As a proof of concept, Williams et al. designed a synthetic QS circuit (Williams et al., 2013, 2015a), where an aromatic amino acid (i.e., tryptophan) induces expression and subsequent secretion of α-pheromone peptide. When extracellular pheromone accumulates to a certain threshold due to increasing cell densities, gene expression from the FUS1J2 promoter is triggered and results in cell cycle arrest at the G1 phase (Figure 3). To achieve QS-regulated gene repression, RNAi constructs of ARO7, PYK, and CDC19, whose knockdown improves production of promoter para-hydroxybenzoic acid (PHBA) but impairs cell fitness, was expressed under the control of FUS1J2 promoter (Figure 3). In an RNAi capable strain, QS-coupled RNAi regulation achieved 1.07 mM PHBA titer, which represents a 37-fold increase over the base strain without the QS circuit (Williams et al., 2015a). This work demonstrates the capability of QS-linked RNAi circuit in dynamic and multiplex gene repression for metabolic engineering.
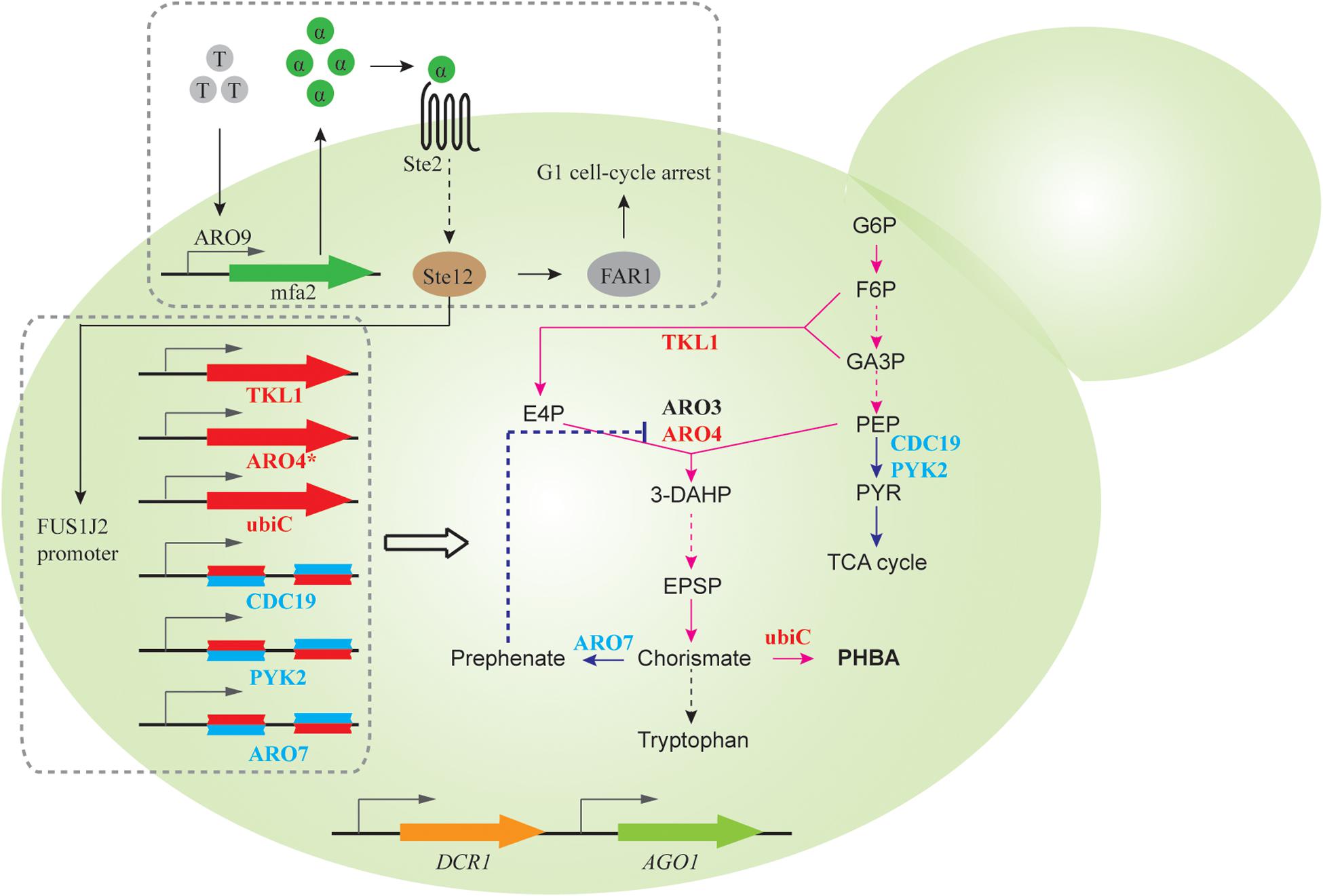
Figure 3. QS-linked, dynamic RNAi regulation during PHBA production. When the population density is high, the extracellular concentration of tryptophan-induced ARO9 promoter-dependent α-pheromone is high, which triggers the mitogen activated protein kinase (MAPK) phosphorylation cascade. The activated Ste12 transcription factor promotes the expression of FAR1, which leads to G1 cell-cycle arrest, and the expression of FUS1J2 promoter-mediated expression of TKL1, ARO4K229L and ubiC gene, and RNAi cassettes targeting CDC19, PYK2, and ARO7 gene, which enables the optimization of the PHBA pathway in the presence of a synthetic RNAi pathway.
Genome-Scale Regulation
Genome-scale engineering creates microbial strain libraries for large-scale genotype-phenotype mapping (Si et al., 2015b). For S. cerevisiae, non-essential genes have been individually deleted for functional genomic studies (Winzeler et al., 1999; Giaever et al., 2002). But gene-knockout libraries are only available in certain laboratory strains, because it is traditionally tedious to replace each gene sequence with a selection marker via homologous recombination (Scherens and Goffeau, 2004). It is necessary to perform genome-wide screening in custom genetic background due to substantial phenotypic variations among different strains (Van Dijken et al., 2000). Thanks to its trans-acting nature, genome-scale knockdown is readily achieved by delivery of RNAi reagents to a select strain on episomal plasmids or via genomic integration. RNAi screening is therefore widely applied to improve a range of target traits in S. cerevisiae.
Using a long dsRNA library constructed with digested genomic DNA and convergent promoters, we performed the first genome-scale RNAi screening in S. cerevisiae (Si et al., 2014). We first validated the screening process by identification of known and new knockdown suppressors (ret1, say1, ssa1, cst6, and mlp2) of yku70Δ mutation, which causes yeast growth arrest at elevated temperature. Notably, RET1 is an essential gene and hence cannot be identified using gene deletion collections. Moreover, inspired by directed protein evolution, we performed iterative rounds of RNAi screening for continuous improvement of acetic acid tolerance via accumulation of beneficial silencing cassettes, one at a time in each round (Figure 4A). Using this RNAi-assisted genome evolution (RAGE) method, we identified three knockdown targets (ptc6, ypr084w, and tRNAVal(AAC)) that synergistically enable yeast to grow in the presence of 1.0% (v/v) HAc. Similarly, the genomic DNA-derived RAGE library was applied to identify siz1 as a new gene knockdown target to improve furfural tolerance in S. cerevisiae (Xiao and Zhao, 2014).
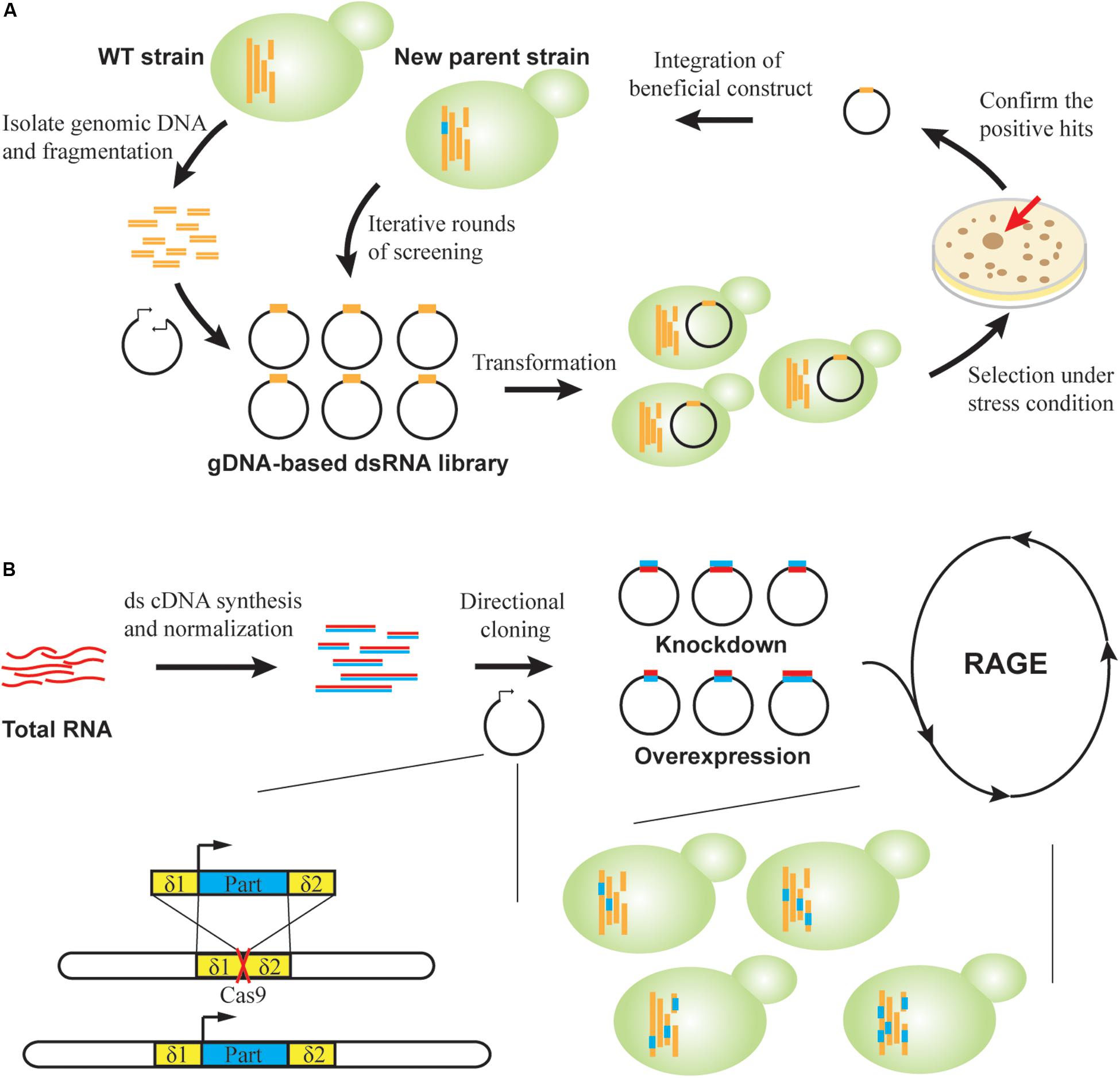
Figure 4. RNAi-assisted genome evolution in S. cerevisiae. (A) RAGE1.0. A dsRNA-encoding library is constructed by inserting genomic DNA fragments into convergent promoters. Strain libraries are created and screened on agar under selective pressure. For confirmed positive clones, corresponding beneficial RNA cassettes are genomically integrated to generate new parents for a subsequent round of selection. (B) RAGE2.0. Directional cloning creates overexpression and knockdown part libraries in a single step from cDNA libraries. Modulation parts are flanked by homologous δ sequences to direct multiplex genomic integration, which is facilitated by CRISPR-Cas. A standardized process of library creation and screening permits robotic iterations for directed genome evolution.
To achieve multi-mode, genome-scale engineering, we designed RAGE 2.0 libraries that permitted genome-wide overexpression and knockdown screening in a single step (Si et al., 2017; Figure 4B). Briefly, a normalized, full-length-enriched cDNA library was first constructed to cover >92% of yeast genes. To the 5′ and 3′ ends of cDNA molecules, we attached two different adaptor sequences that allowed directional cloning of cDNAs under a strong constitutive promoter PTEF1. Therefore, gene overexpression was achieved when cDNA molecules were transcribed in the sense direction, whereas gene repression was achieved through transcription of antisense RNAs in the presence of a heterologous RNAi pathway. Using this collection of modulation parts, both gene overexpression and knockdown targets were successfully identified in laboratory and industrial yeast strains for improved cellulase expression, isobutanol production, and xylose utilization (Si et al., 2017; HamediRad et al., 2018). Notably, in addition to isolating individual mutant strains, genome-wide mapping of gene–trait relations can be also achieved. We targeted glycerol substrate utilization, and improved mutants were enriched during serial transfer in glycerol media. Before and after growth enrichment, NGS was applied to track frequency changes of individual modulation parts, which was used to calculate enrichment ratios of corresponding mutants. In this way, the functional impact of every RAGE2.0 overexpression or knockdown mutation on glycerol utilization was quantified (Si et al., 2017).
Furthermore, the standardized and modular design of RAGE2.0 parts enabled accumulation of multiplex, genome-wide mutations in an evolving yeast genome (Si et al., 2017). We utilized the yeast genomic δ sites as landing pads for integrating multiple parts, where genomic integration efficiency was greatly enhanced by introduction of specific double-stranded breaks within δ sequences using the clustered regularly interspaced short palindromic repeats (CRISPR)/CRISPR-associated (Cas) system (Figure 4B). We then designed a standardized process where strain library creation and screening can be iteratively and robotically performed. After three rounds of selection under increasing HAc concentrations of 0.9, 1.0, and 1.1% (v/v), a mutant strain harboring at least 26 different modulation parts was isolated, indicating the success in creating multiplex genomic diversity. This strain was able to ferment glucose into ethanol in 1.1% (v/v) HAc media, which is the highest reported HAc tolerance to the best of our knowledge.
Alternatively, the Alper group pioneered in creating cDNA-derived RNAi libraries for tunable, genome-wide screening in S. cerevisiae (Crook et al., 2016). Using this library, Hsp70 family proteins were identified as key regulators of isobutanol tolerance. Reduced expression of these proteins increased biomass accumulation by 64% under 12 g/L isobutanol stress. Moreover, two rounds of screening identified two RNAi reagents targeting alcohol dehydrogenase and enolase. When combined, these two mutations exhibited a 31-fold improvement in cell growth in the presence of 10 g/L 1-butanol. Furthermore, reduced expression of the ribosome-associated chaperone Ssb1p was discovered to confer a 52% increase in lactic acid tolerance using the same RNAi library (Lee et al., 2016).
In a recent study, the Nielsen group integrated RNAi screening, microfluidic sorting, and Cas9-mediated genome recombineering to establish a workflow for identification and optimization of multiple gene targets (Wang et al., 2019). Two RNAi libraries were constructed, whereas the genomic DNA-derived library and full-length cDNA-derived library exhibited low and high knockdown efficiencies, respectively. Using droplet microfluidics, 1,500,000 cells covering ∼243,000 knockdown effectors were sorted and enriched in three rounds for improved α-amylase secretion, and genes involved in cellular metabolism, protein modification and degradation, and cell cycle were found to exert substantial impact on recombinant protein production.
Taken together, a variety of genome-scale RNAi libraries were created to screen a wide range of industrially relevant phenotypes in S. cerevisiae. These studies demonstrated several unique advantages. First, unlike gene deletion collections that are available only in certain laboratory strains (Scherens and Goffeau, 2004), genome-wide RNAi libraries can be readily introduced in a custom strain background, such as CEN.PK (Si et al., 2014, 2017; Wang et al., 2019), BY4741 (Xiao and Zhao, 2014; Crook et al., 2016; Lee et al., 2016), and industrial strains (Si et al., 2017; HamediRad et al., 2018). Notably, this feature enables the use of directed evolution strategies in genome-wide engineering, whereby beneficial mutations can be continuously accumulated in an evolving yeast genome (Si et al., 2014). Second, the multiplex nature of RNAi silencing permits simultaneous modulation of many gene targets, whose synergistic actions may lead to superior traits (Si et al., 2014, 2017; Wang et al., 2019). Third, mediating mRNA degradation, RNAi is particularly suited to screen gene silencing targets in polyploid industrial strains, whereby multiple gene copies often produce the same transcript (Si et al., 2017; HamediRad et al., 2018). Fourth, the use of RNAi expands the range of gene regulation in genome-scale screening, whereby not only various repression levels can be achieved by using different RNAi designs (Wang et al., 2019), but also inclusion of both overexpression and knockdown mutations in a single genome-wide library has been realized (Si et al., 2017).
On the other hand, there are certain limitations of genome-scale RNAi screening in S. cerevisiae. Due to the random nature of genomic DNA and cDNA-derived libraries, it is essential to perform necessary quality control to ensure sufficient genomic coverage and representation are achieved (Si et al., 2017). Also, due to the prevalence of off-target effects (Jackson et al., 2003), RNAi targets identified from large-scale screening need to be further confirmed by complementary approaches. For example, one can investigate whether the observed phenotype can be generated by a second RNAi reagent designed to target a distinct region of the same gene relative to the isolated RNAi (Si et al., 2014), as it is highly unlikely that two independent RNAi sequences exhibit the same off-target effect. Moreover, most RNAi screening studies in S. cerevisiae target “easy-to-screen” phenotypes such as chemical tolerance (Si et al., 2014, 2017; Xiao and Zhao, 2014; Crook et al., 2016; Lee et al., 2016) and substrate utilization (Si et al., 2017; HamediRad et al., 2018), because desirable mutant strains can be facilely identified by growth-based selection or enrichment. The integration of robotics and microfluidics will help to expand the scope of phenotypes that can be engineered using genome-scale RNAi screening (Si et al., 2017; Wang et al., 2019). Finally, for multiplex gene silencing (Si et al., 2017), simultaneous introduction of many RNAi reagents may saturate the RNAi machinery and resulted in inefficient silencing of individual targets, but such complication has not been rigorously assessed. Also, it is inherent challenging to speculate how multiple mutations may confer an improved trait, so that mechanistic studies are necessary to analyze the functional impact of single mutations and their interactions.
Conclusion and Future Directions
RNA interference has long been a versatile tool for studying eukaryotic functional genomics studies but is absent from S. cerevisiae. Recent advances have greatly expanded the toolkit and application of RNAi in this model yeast, exhibiting many advantages including efficiency, specificity, graded and dynamic repression, genome-scale coverage, and multiplex targeting. Currently, a few groups have been routinely utilizing RNAi as a functional genomics and strain engineering tool in S. cerevisiae, although other potential applications such as genetic circuit design (Purcell et al., 2018) and gene delivery (Duman-Scheel, 2019) are also explored. One major factor limiting a wider use of RNAi by the S. cerevisiae communities is the availability of ample gene regulation tools in this model organism. Other gene repression methods include but are not limited to promoter engineering (Nevoigt et al., 2006), mRNA destabilization via 3′ insertion (Breslow et al., 2008), and CRISPR interference (CRISPRi) (Gilbert et al., 2013). However, the ease of creating genome-wide libraries directly from genomic DNA and cDNA molecules makes RNAi an attractive tool even in the era of CRISPR/Cas. Creating genome-wide deletion libraries by CRISPR/Cas requires genome sequencing data, algorithm to predict and rank guide RNA (gRNA) sequences, as well as introduction of homologous repair donors for efficient genome editing (Bao et al., 2018; Sharon et al., 2018). Using protein fusions between nuclease-dead mutants of Cas9 (dCas9) and transcriptional repressors, CRISPRi was successfully developed for genome-scale reduction-of-function screening in S. cerevisiae (Lian et al., 2019). Many prerequisites of CRISPR/Cas are not always available in polyploid industrial strains, but they do not limit the use of RNAi (HamediRad et al., 2018). Nonetheless, the ability to prototype gRNA collections based on array-synthesized oligo pools confers a greater potential of CRISPRi over RNAi, because the coverage and efficacy of gRNA libraries may be improved in “design-build-test” iterations. Notably, because both genome-scale CRISRPi and RNAi screening are currently performed in pooled formats, so that the silencing efficiency and off-target effect of individual reagents (gRNA or siRNA) remains unknown, which warrants detailed characterization in future studies.
Certain cautions should be taken during the design and implementation of RNAi in S. cerevisiae. The length and targeting position of hairpin RNA or dsRNA cassettes exhibit substantial impact on RNAi efficiency (Crook et al., 2014, 2016; Si et al., 2014), but there is currently no design rules. In addition, the expression level and mRNA structure of target gene may affect RNAi efficacy. For example, inefficient repression of ZWF1 was attributed to the interference from mRNA secondary structure (Williams et al., 2015a). Moreover, RNAi often suffers from off-target effects, which may be particularly severe due to longer dsRNA precursors in budding yeasts for siRNA production (Weinberg et al., 2011; Wilson and Doudna, 2013; Ipsaro and Joshua-Tor, 2015). Systematic experimental evaluation and computer-aided design may help to understand and avoid the abovementioned issues (Lagana et al., 2014).
To further improve RNAi tools in S. cerevisiae, a more potent RNAi pathway can be engineered. For example, Xrn1 orthologs were reported to be involved in multiple steps of RNAi in Arabidopsis thaliana, Caenorhabditis elegans, Drosophila melanogaster, and Homo sapiens (Souret et al., 2004; Orban and Izaurralde, 2005; Chatterjee et al., 2011; Lima et al., 2016). In a genetic selection to discover novel components of RNAi in S. castellii, Xrn1p was found to play multiple roles to enhance silencing efficiency, including affecting the ratio of different types of siRNA precursors and duplexes, increasing siRNA loading into Ago1p, and enhancing degradation of the passenger strand (Getz et al., 2019). It is interesting to see if introduction of S. castellii Xrn1p can improve RNAi processing capabilities in S. cerevisiae, which may be limiting when multiplex RNAi regulation is performed (Si et al., 2017).
In addition to RNAi, other regulatory RNAs have been or can be potentially implemented for yeast strain engineering, such as CRISPR RNA, antisense non-coding RNAs, and tRNAs (David et al., 2006; Samanta et al., 2006; Donaldson and Saville, 2012; Si et al., 2015a; Bakowska-Zywicka et al., 2016). For example, antisense non-coding RNAs have been revealed for gene-specific transcriptional regulation, targeting SER3 (Martens et al., 2004), IME4 (Hongay et al., 2006), PHO5 (Uhler et al., 2007), PHO84 (Camblong et al., 2007, 2009; Castelnuovo et al., 2013), GAL (Houseley et al., 2008), IME1 (Van Werven et al., 2012; Moretto et al., 2018), and CDC28 (Nadal-Ribelles et al., 2014). Distinct mechanisms are employed, including mRNA interference, competition with transcriptional machinery, histone modification, and gene loop (Yamashita et al., 2016; Niederer et al., 2017; Till et al., 2018; Novačić et al., 2020). Systematic examination of antisense transcriptome will provide comprehensive insights into the mechanisms of antisense RNA-dependent gene regulation to guide engineering tool development (Huber et al., 2016; Nevers et al., 2018). With a deeper understanding, we envision the development of versatile RNA tools that are complementary to RNAi for facilitating strain engineering in S. cerevisiae.
Author Contributions
YC and TS conceived the scope of this work. YC and EG drafted the manuscript. JZ and TS involved in revising and editing the manuscript. All authors approved the submitted version and agreed both to be personally accountable for the author’s own contributions and to ensure that questions related to the accuracy or integrity of any part of the work.
Conflict of Interest
The authors declare that the research was conducted in the absence of any commercial or financial relationships that could be construed as a potential conflict of interest.
Acknowledgments
We apologize to those whose findings are relevant but not cited in this review due to space limitation. We acknowledged the financial support from Shenzhen Institute of Synthetic Biology Scientific Research Program JCHZ20190003.
References
Agrawal, N., Dasaradhi, P. V., Mohmmed, A., Malhotra, P., Bhatnagar, R. K., and Mukherjee, S. K. (2003). RNA interference: biology, mechanism, and applications. Microbiol. Mol. Biol. Rev. 67, 657–685. doi: 10.1128/mmbr.67.4.657-685.2003
Bakowska-Zywicka, K., Mleczko, A. M., Kasprzyk, M., Machtel, P., Zywicki, M., and Twardowski, T. (2016). The widespread occurrence of tRNA-derived fragments in Saccharomyces cerevisiae. FEBS Open Bio. 6, 1186–1200. doi: 10.1002/2211-5463.12127
Bao, Z., HamediRad, M., Xue, P., Xiao, H., Tasan, I., Chao, R., et al. (2018). Genome-scale engineering of Saccharomyces cerevisiae with single-nucleotide precision. Nat. Biotechnol. 36, 505–508. doi: 10.1038/nbt.4132
Blount, B. A., Weenink, T., and Ellis, T. (2012). Construction of synthetic regulatory networks in yeast. FEBS Lett. 586, 2112–2121. doi: 10.1016/j.febslet.2012.01.053
Borkowski, O., Ceroni, F., Stan, G. B., and Ellis, T. (2016). Overloaded and stressed: whole-cell considerations for bacterial synthetic biology. Curr. Opin. Microbiol. 33, 123–130. doi: 10.1016/j.mib.2016.07.009
Boutros, M., and Ahringer, J. (2008). The art and design of genetic screens: RNA interference. Nat. Rev. Genet. 9, 554–566. doi: 10.1038/nrg2364
Boynton, P. J. (2019). The ecology of killer yeasts: Interference competition in natural habitats. Yeast 36, 473–485. doi: 10.1002/yea.3398
Breslow, D. K., Cameron, D. M., Collins, S. R., Schuldiner, M., Stewart-Ornstein, J., Newman, H. W., et al. (2008). A comprehensive strategy enabling high-resolution functional analysis of the yeast genome. Nat. Methods 5, 711–718. doi: 10.1038/nmeth.1234
Camblong, J., Beyrouthy, N., Guffanti, E., Schlaepfer, G., Steinmetz, L. M., and Stutz, F. (2009). Trans-acting antisense RNAs mediate transcriptional gene cosuppression in S. cerevisiae. Genes Dev. 23, 1534–1545. doi: 10.1101/gad.522509
Camblong, J., Iglesias, N., Fickentscher, C., Dieppois, G., and Stutz, F. (2007). Antisense RNA stabilization induces transcriptional gene silencing via histone deacetylation in S. cerevisiae. Cell 131, 706–717. doi: 10.1016/j.cell.2007.09.014
Castel, S. E., and Martienssen, R. A. (2013). RNA interference in the nucleus: roles for small RNAs in transcription, epigenetics and beyond. Nat. Rev. Genet. 14, 100–112. doi: 10.1038/nrg3355
Castelnuovo, M., Rahman, S., Guffanti, E., Infantino, V., Stutz, F., and Zenklusen, D. (2013). Bimodal expression of PHO84 is modulated by early termination of antisense transcription. Nat. Struct. Mol. Biol. 20, 851–858. doi: 10.1038/nsmb.2598
Chatterjee, S., Fasler, M., Bussing, I., and Grosshans, H. (2011). Target-mediated protection of endogenous microRNAs in C. elegans. Dev. Cell 20, 388–396. doi: 10.1016/j.devcel.2011.02.008
Chendrimada, T. P., Gregory, R. I., Kumaraswamy, E., Norman, J., Cooch, N., Nishikura, K., et al. (2005). TRBP recruits the Dicer complex to Ago2 for microRNA processing and gene silencing. Nature 436, 740–744. doi: 10.1038/nature03868
Cheng, X., and Jian, R. (2010). Construction and application of random dsRNA interference library for functional genetic screens in embryonic stem cells. Methods Mol. Biol. 650, 65–74. doi: 10.1007/978-1-60761-769-3_5
Choudhary, S., and Schmidt-Dannert, C. (2010). Applications of quorum sensing in biotechnology. Appl. Microbiol. Biotechnol. 86, 1267–1279. doi: 10.1007/s00253-010-2521-7
Crook, N., Sun, J., Morse, N., Schmitz, A., and Alper, H. S. (2016). Identification of gene knockdown targets conferring enhanced isobutanol and 1-butanol tolerance to Saccharomyces cerevisiae using a tunable RNAi screening approach. Appl. Microbiol. Biotechnol. 100, 10005–10018. doi: 10.1007/s00253-016-7791-2
Crook, N. C., Schmitz, A. C., and Alper, H. S. (2014). Optimization of a yeast RNA interference system for controlling gene expression and enabling rapid metabolic engineering. ACS Synth. Biol. 3, 307–313. doi: 10.1021/sb4001432
David, L., Huber, W., Granovskaia, M., Toedling, J., Palm, C. J., Bofkin, L., et al. (2006). A high-resolution map of transcription in the yeast genome. Proc. Natl. Acad. Sci. U.S.A. 103, 5320–5325. doi: 10.1073/pnas.0601091103
Donaldson, M. E., and Saville, B. J. (2012). Natural antisense transcripts in fungi. Mol. Microbiol. 85, 405–417. doi: 10.1111/j.1365-2958.2012.08125.x
Drinnenberg, I. A., Fink, G. R., and Bartel, D. P. (2011). Compatibility with killer explains the rise of RNAi-deficient fungi. Science 333:1592. doi: 10.1126/science.1209575
Drinnenberg, I. A., Weinberg, D. E., Xie, K. T., Mower, J. P., Wolfe, K. H., Fink, G. R., et al. (2009). RNAi in Budding Yeast. Science 326, 544–550. doi: 10.1126/science.1176945
Duman-Scheel, M. (2019). Saccharomyces cerevisiae (Baker’s Yeast) as an Interfering RNA expression and delivery system. Curr. Drug Targets 20, 942–952. doi: 10.2174/1389450120666181126123538
Fire, A., Xu, S., Montgomery, M. K., Kostas, S. A., Driver, S. E., and Mello, C. C. (1998). Potent and specific genetic interference by double-stranded RNA in Caenorhabditis elegans. Nature 391, 806–811. doi: 10.1038/35888
Getz, M. A., Weinberg, D. E., Drinnenberg, I. A., Fink, G. R., and Bartel, D. P. (2019). Xrn1p acts at multiple steps in the budding-yeast RNAi pathway to enhance the efficiency of silencing. bioRxiv. [Preprint]. doi: 10.1101/2019.12.12.873604
Giaever, G., Chu, A. M., Ni, L., Connelly, C., Riles, L., Veronneau, S., et al. (2002). Functional profiling of the Saccharomyces cerevisiae genome. Nature 418, 387–391. doi: 10.1038/nature00935
Gilbert, L. A., Larson, M. H., Morsut, L., Liu, Z., Brar, G. A., Torres, S. E., et al. (2013). CRISPR-mediated modular RNA-guided regulation of transcription in eukaryotes. Cell 154, 442–451. doi: 10.1016/j.cell.2013.06.044
Gregory, R. I., Chendrimada, T. P., Cooch, N., and Shiekhattar, R. (2005). Human RISC couples microRNA biogenesis and posttranscriptional gene silencing. Cell 123, 631–640. doi: 10.1016/j.cell.2005.10.022
Gutbrod, M. J., and Martienssen, R. A. (2020). Conserved chromosomal functions of RNA interference. Nat. Rev. Genet. 21, 311–331. doi: 10.1038/s41576-019-0203-6
HamediRad, M., Lian, J., Li, H., and Zhao, H. (2018). RNAi assisted genome evolution unveils yeast mutants with improved xylose utilization. Biotechnol. Bioeng. 115, 1552–1560. doi: 10.1002/bit.26570
Hasunuma, T., and Kondo, A. (2012). Development of yeast cell factories for consolidated bioprocessing of lignocellulose to bioethanol through cell surface engineering. Biotechnol. Adv. 30, 1207–1218. doi: 10.1016/j.biotechadv.2011.10.011
Hong, K. K., and Nielsen, J. (2012). Metabolic engineering of Saccharomyces cerevisiae: a key cell factory platform for future biorefineries. Cell. Mol. Life Sci. 69, 2671–2690. doi: 10.1007/s00018-012-0945-1
Hongay, C. F., Grisafi, P. L., Galitski, T., and Fink, G. R. (2006). Antisense transcription controls cell fate in Saccharomyces cerevisiae. Cell 127, 735–745. doi: 10.1016/j.cell.2006.09.038
Horn, T., Sandmann, T., and Boutros, M. (2010). Design and evaluation of genome-wide libraries for RNA interference screens. Genome Biol. 11:R61. doi: 10.1186/gb-2010-11-6-r61
Houseley, J., Rubbi, L., Grunstein, M., Tollervey, D., and Vogelauer, M. (2008). A ncRNA modulates histone modification and mRNA induction in the yeast GAL gene cluster. Mol. Cell 32, 685–695. doi: 10.1016/j.molcel.2008.09.027
Huber, F., Bunina, D., Gupta, I., Khmelinskii, A., Meurer, M., Theer, P., et al. (2016). Protein abundance control by non-coding antisense transcription. Cell Rep. 15, 2625–2636. doi: 10.1016/j.celrep.2016.05.043
Ipsaro, J. J., and Joshua-Tor, L. (2015). From guide to target: molecular insights into eukaryotic RNA-interference machinery. Nat. Struct. Mol. Biol. 22, 20–28. doi: 10.1038/nsmb.2931
Jackson, A. L., Bartz, S. R., Schelter, J., Kobayashi, S. V., Burchard, J., Mao, M., et al. (2003). Expression profiling reveals off-target gene regulation by RNAi. Nat. Biotechnol. 21, 635–637. doi: 10.1038/nbt831
Jain, C. K., and Wadhwa, G. (2018). “Computational Tools: RNA Interference in Fungal Therapeutics,” in Current Trends in Bioinformatics: An Insight, eds G. Wadhwa, P. Shanmughavel, A. K. Singh, and J. R. Bellare (Singapore: Springer), 207–225. doi: 10.1007/978-981-10-7483-7_12
Jiang, S., Si, T., and Dai, J. (2019). Whole-Genome Regulation for Yeast Metabolic Engineering. Small Methods 4:1900640. doi: 10.1002/smtd.201900640
Kavscek, M., Strazar, M., Curk, T., Natter, K., and Petrovic, U. (2015). Yeast as a cell factory: current state and perspectives. Microb. Cell Fact. 14:94. doi: 10.1186/s12934-015-0281-x
Keasling, J. D. (2008). Synthetic biology for synthetic chemistry. ACS Chem. Biol. 3, 64–76. doi: 10.1021/cb7002434
Kildegaard, K. R., Tramontin, L. R. R., Chekina, K., Li, M., Goedecke, T. J., Kristensen, M., et al. (2019). CRISPR/Cas9-RNA interference system for combinatorial metabolic engineering of Saccharomyces cerevisiae. Yeast 36, 237–247. doi: 10.1002/yea.3390
Lagana, A., Shasha, D., and Croce, C. M. (2014). Synthetic RNAs for Gene Regulation: Design Principles and Computational Tools. Front. Bioeng. Biotechnol. 2:65. doi: 10.3389/fbioe.2014.00065
Lee, J. J., Crook, N., Sun, J., and Alper, H. S. (2016). Improvement of lactic acid production in Saccharomyces cerevisiae by a deletion of ssb1. J. Ind. Microbiol. Biotechnol. 43, 87–96. doi: 10.1007/s10295-015-1713-7
Li, Y., Lu, J., Han, Y., Fan, X., and Ding, S. W. (2013). RNA interference functions as an antiviral immunity mechanism in mammals. Science 342, 231–234. doi: 10.1126/science.1241911
Lian, J., Mishra, S., and Zhao, H. (2018). Recent advances in metabolic engineering of Saccharomyces cerevisiae: New tools and their applications. Metab. Eng. 50, 85–108. doi: 10.1016/j.ymben.2018.04.011
Lian, J., Schultz, C., Cao, M., HamediRad, M., and Zhao, H. (2019). Multi-functional genome-wide CRISPR system for high throughput genotype-phenotype mapping. Nat. Commun. 10:5794. doi: 10.1038/s41467-019-13621-4
Lima, W. F., De Hoyos, C. L., Liang, X. H., and Crooke, S. T. (2016). RNA cleavage products generated by antisense oligonucleotides and siRNAs are processed by the RNA surveillance machinery. Nucleic Acids Res. 44, 3351–3363. doi: 10.1093/nar/gkw065
Liu, L., Redden, H., and Alper, H. S. (2013). Frontiers of yeast metabolic engineering: diversifying beyond ethanol and Saccharomyces. Curr. Opin. Biotechnol. 24, 1023–1030. doi: 10.1016/j.copbio.2013.03.005
Loftus, B. J., Fung, E., Roncaglia, P., Rowley, D., Amedeo, P., Bruno, D., et al. (2005). The genome of the basidiomycetous yeast and human pathogen Cryptococcus neoformans. Science 307, 1321–1324. doi: 10.1126/science.1103773
Maniataki, E., and Mourelatos, Z. (2005). A human, ATP-independent, RISC assembly machine fueled by pre-miRNA. Genes Dev. 19, 2979–2990. doi: 10.1101/gad.1384005
Martens, J. A., Laprade, L., and Winston, F. (2004). Intergenic transcription is required to repress the Saccharomyces cerevisiae SER3 gene. Nature 429, 571–574. doi: 10.1038/nature02538
Miller, M. B., and Bassler, B. L. (2001). Quorum sensing in bacteria. Annu. Rev. Microbiol. 55, 165–199. doi: 10.1146/annurev.micro.55.1.165
Mohr, S. E., Smith, J. A., Shamu, C. E., Neumuller, R. A., and Perrimon, N. (2014). RNAi screening comes of age: improved techniques and complementary approaches. Nat. Rev. Mol. Cell Biol. 15, 591–600. doi: 10.1038/nrm3860
Moretto, F., Wood, N. E., Kelly, G., Doncic, A., and van Werven, F. J. (2018). A regulatory circuit of two lncRNAs and a master regulator directs cell fate in yeast. Nat. Commun. 9:780. doi: 10.1038/s41467-018-03213-z
Nadal-Ribelles, M., Sole, C., Xu, Z., Steinmetz, L. M., de Nadal, E., and Posas, F. (2014). Control of Cdc28 CDK1 by a stress-induced lncRNA. Mol. Cell 53, 549–561. doi: 10.1016/j.molcel.2014.01.006
Nevers, A., Doyen, A., Malabat, C., Néron, B., Kergrohen, T., Jacquier, A., et al. (2018). Antisense transcriptional interference mediates condition-specific gene repression in budding yeast. Nucleic Acids Res. 46, 6009–6025. doi: 10.1093/nar/gky342
Nevoigt, E., Kohnke, J., Fischer, C. R., Alper, H., Stahl, U., and Stephanopoulos, G. (2006). Engineering of promoter replacement cassettes for fine-tuning of gene expression in Saccharomyces cerevisiae. Appl. Environ. Microbiol. 72, 5266–5273. doi: 10.1128/AEM.00530-06
Niederer, R. O., Hass, E. P., and Zappulla, D. C. (2017). Long Noncoding RNAs in the Yeast S. cerevisiae. Adv. Exp. Med. Biol. 1008, 119–132. doi: 10.1007/978-981-10-5203-3_4
Nielsen, J. (2019). Yeast Systems Biology: Model Organism and Cell Factory. Biotechnol. J. 14:e1800421. doi: 10.1002/biot.201800421
Novačić, A., Vučenović, I., Primig, M., and Stuparević, I. (2020). Non-coding RNAs as cell wall regulators in Saccharomyces cerevisiae. Crit. Rev. Microbiol. 46, 1–11. doi: 10.1080/1040841X.2020.1715340
Orban, T. I., and Izaurralde, E. (2005). Decay of mRNAs targeted by RISC requires XRN1, the Ski complex, and the exosome. RNA 11, 459–469. doi: 10.1261/rna.7231505
Purcell, O., Cao, J., Muller, I. E., Chen, Y. C., and Lu, T. K. (2018). Artificial repeat-structured siRNA precursors as tunable regulators for Saccharomyces cerevisiae. ACS Synth. Biol. 7, 2403–2412. doi: 10.1021/acssynbio.8b00185
Samanta, M. P., Tongprasit, W., Sethi, H., Chin, C. S., and Stolc, V. (2006). Global identification of noncoding RNAs in Saccharomyces cerevisiae by modulating an essential RNA processing pathway. Proc. Natl. Acad. Sci. U.S.A. 103, 4192–4197. doi: 10.1073/pnas.0507669103
Scherens, B., and Goffeau, A. (2004). The uses of genome-wide yeast mutant collections. Genome Biol. 5:229. doi: 10.1186/gb-2004-5-7-229
Sharon, E., Chen, S. A., Khosla, N. M., Smith, J. D., Pritchard, J. K., and Fraser, H. B. (2018). Functional genetic variants revealed by massively parallel precise genome editing. Cell 175, 544–557.e16. doi: 10.1016/j.cell.2018.08.057
Si, T., Chao, R., Min, Y., Wu, Y., Ren, W., and Zhao, H. (2017). Automated multiplex genome-scale engineering in yeast. Nat. Commun. 8:15187. doi: 10.1038/ncomms15187
Si, T., HamediRad, M., and Zhao, H. (2015a). Regulatory RNA-assisted genome engineering in microorganisms. Curr. Opin. Biotechnol. 36, 85–90. doi: 10.1016/j.copbio.2015.08.003
Si, T., Xiao, H., and Zhao, H. (2015b). Rapid prototyping of microbial cell factories via genome-scale engineering. Biotechnol. Adv. 33, 1420–1432. doi: 10.1016/j.biotechadv.2014.11.007
Si, T., Luo, Y., Bao, Z., and Zhao, H. (2014). RNAi-assisted genome evolution in Saccharomyces cerevisiae for complex phenotype engineering. ACS Synth. Biol. 4, 283–291. doi: 10.1021/sb500074a
Si, T., and Zhao, H. (2016). RNAi-assisted genome evolution (RAGE) in Saccharomyces cerevisiae. Methods Mol. Biol. 1470, 183–198. doi: 10.1007/978-1-4939-6337-9_15
Souret, F. F., Kastenmayer, J. P., and Green, P. J. (2004). AtXRN4 degrades mRNA in Arabidopsis and its substrates include selected miRNA targets. Mol. Cell 15, 173–183. doi: 10.1016/j.molcel.2004.06.006
Suk, K., Choi, J., Suzuki, Y., Ozturk, S. B., Mellor, J. C., Wong, K. H., et al. (2011). Reconstitution of human RNA interference in budding yeast. Nucleic Acids Res. 39:e43. doi: 10.1093/nar/gkq1321
Till, P., Mach, R. L., and Mach-Aigner, A. R. (2018). A current view on long noncoding RNAs in yeast and filamentous fungi. Appl. Microbiol. Biotechnol. 102, 7319–7331. doi: 10.1007/s00253-018-9187-y
Tokmakov, A. A., Kurotani, A., Takagi, T., Toyama, M., Shirouzu, M., Fukami, Y., et al. (2012). Multiple post-translational modifications affect heterologous protein synthesis. J. Biol. Chem. 287, 27106–27116. doi: 10.1074/jbc.M112.366351
Tripathi, N. K., and Shrivastava, A. (2019). Recent developments in bioprocessing of recombinant proteins: expression hosts and process development. Front. Bioeng. Biotechnol. 7:420. doi: 10.3389/fbioe.2019.00420
Uhler, J. P., Hertel, C., and Svejstrup, J. Q. (2007). A role for noncoding transcription in activation of the yeast PHO5 gene. Proc. Natl. Acad. Sci. U.S.A. 104, 8011–8016. doi: 10.1073/pnas.0702431104
Van Dijken, J. P., Bauer, J., Brambilla, L., Duboc, P., Francois, J. M., Gancedo, C., et al. (2000). An interlaboratory comparison of physiological and genetic properties of four Saccharomyces cerevisiae strains. Enzyme Microb. Technol. 26, 706–714. doi: 10.1016/s0141-0229(00)00162-9
Van Werven, F. J., Neuert, G., Hendrick, N., Lardenois, A., Buratowski, S., van Oudenaarden, A., et al. (2012). Transcription of two long noncoding RNAs mediates mating-type control of gametogenesis in budding yeast. Cell 150, 1170–1181. doi: 10.1016/j.cell.2012.06.049
Voineagu, I., Narayanan, V., Lobachev, K. S., and Mirkin, S. M. (2008). Replication stalling at unstable inverted repeats: interplay between DNA hairpins and fork stabilizing proteins. Proc. Natl. Acad. Sci. U.S.A. 105, 9936–9941. doi: 10.1073/pnas.0804510105
Volpe, T. A., Kidner, C., Hall, I. M., Teng, G., Grewal, S. I., and Martienssen, R. A. (2002). Regulation of heterochromatic silencing and histone H3 lysine-9 methylation by RNAi. Science 297, 1833–1837. doi: 10.1126/science.1074973
Waldron, F. M., Stone, G. N., and Obbard, D. J. (2018). Metagenomic sequencing suggests a diversity of RNA interference-like responses to viruses across multicellular eukaryotes. PLoS Genet. 14:e1007533. doi: 10.1371/journal.pgen.1007533
Wang, G., Björk, S. M., Huang, M., Liu, Q., Campbell, K., Nielsen, J., et al. (2019). RNAi expression tuning, microfluidic screening, and genome recombineering for improved protein production in Saccharomyces cerevisiae. Proc. Natl. Acad. Sci. U.S.A. 116, 9324–9332. doi: 10.1073/pnas.1820561116
Weinberg, D. E., Nakanishi, K., Patel, D. J., and Bartel, D. P. (2011). The inside-out mechanism of Dicers from budding yeasts. Cell 146, 262–276. doi: 10.1016/j.cell.2011.06.021
Williams, T. C., Averesch, N. J. H., Winter, G., Plan, M. R., Vickers, C. E., Nielsen, L. K., et al. (2015a). Quorum-sensing linked RNA interference for dynamic metabolic pathway control in Saccharomyces cerevisiae. Metab. Eng. 29, 124–134. doi: 10.1016/j.ymben.2015.03.008
Williams, T. C., Espinosa, M. I., Nielsen, L. K., and Vickers, C. E. (2015b). Dynamic regulation of gene expression using sucrose responsive promoters and RNA interference in Saccharomyces cerevisiae. Microb. Cell Fact. 14:43. doi: 10.1186/s12934-015-0223-7
Williams, T. C., Nielsen, L. K., and Vickers, C. E. (2013). Engineered quorum sensing using pheromone-mediated cell-to-cell communication in Saccharomyces cerevisiae. ACS Synth. Biol. 2, 136–149. doi: 10.1021/sb300110b
Wilson, R. C., and Doudna, J. A. (2013). Molecular mechanisms of RNA interference. Annu. Rev. Biophys. 42, 217–239. doi: 10.1146/annurev-biophys-083012-130404
Winzeler, E. A., Shoemaker, D. D., Astromoff, A., Liang, H., Anderson, K., Andre, B., et al. (1999). Functional characterization of the S. cerevisiae genome by gene deletion and parallel analysis. Science 285, 901–906. doi: 10.1126/science.285.5429.901
Xiao, H., and Zhao, H. (2014). Genome-wide RNAi screen reveals the E3 SUMO-protein ligase gene SIZ1 as a novel determinant of furfural tolerance in Saccharomyces cerevisiae. Biotechnol. Biofuels 7:78. doi: 10.1186/1754-6834-7-78
Yamashita, A., Shichino, Y., and Yamamoto, M. (2016). The long non-coding RNA world in yeasts. BBA Gene Regul. Mech. 1859, 147–154. doi: 10.1016/j.bbagrm.2015.08.003
Zhu, Y. Y., Machleder, E. M., Chenchik, A., Li, R., and Siebert, P. D. (2001). Reverse transcriptase template switching: a SMART approach for full-length cDNA library construction. Biotechniques 30, 892–897. doi: 10.2144/01304pf02
Keywords: RNAi, Saccharomyces cerevisiae, strain engineering, functional genomics, synthetic biology
Citation: Chen Y, Guo E, Zhang J and Si T (2020) Advances in RNAi-Assisted Strain Engineering in Saccharomyces cerevisiae. Front. Bioeng. Biotechnol. 8:731. doi: 10.3389/fbioe.2020.00731
Received: 17 April 2020; Accepted: 10 June 2020;
Published: 02 July 2020.
Edited by:
Yunzi Luo, Sichuan University, ChinaReviewed by:
Zihe Liu, Beijing University of Chemical Technology, ChinaXinqing Zhao, Shanghai Jiao Tong University, China
Copyright © 2020 Chen, Guo, Zhang and Si. This is an open-access article distributed under the terms of the Creative Commons Attribution License (CC BY). The use, distribution or reproduction in other forums is permitted, provided the original author(s) and the copyright owner(s) are credited and that the original publication in this journal is cited, in accordance with accepted academic practice. No use, distribution or reproduction is permitted which does not comply with these terms.
*Correspondence: Tong Si, dG9uZy5zaUBzaWF0LmFjLmNu