- 1School of Biological Sciences, University of Bristol, Bristol, United Kingdom
- 2Department of Engineering Mathematics, University of Bristol, Bristol, United Kingdom
- 3School of Biosciences and Institute of Microbiology and Infection and Centre for Computational Biology, University of Birmingham, Birmingham, United Kingdom
- 4Max Plank Institute of Molecular Cell Biology and Genetics, Dresden, Germany
- 5Physics of Life, Cluster of Excellence, Technische Universität Dresden, Dresden, Germany
- 6School of Engineering, University of Edinburgh, Edinburgh, United Kingdom
- 7School of Biochemistry, University of Bristol, Bristol, United Kingdom
- 8Division of Biosciences, University College London, London, United Kingdom
- 9School of Computing, Newcastle University, Newcastle upon Tyne, United Kingdom
- 10Bateson Centre and School of Mathematics and Statistics, University of Sheffield, Sheffield, United Kingdom
- 11The European Molecular Biology Laboratory, Barcelona, Spain
Many complex behaviors in biological systems emerge from large populations of interacting molecules or cells, generating functions that go beyond the capabilities of the individual parts. Such collective phenomena are of great interest to bioengineers due to their robustness and scalability. However, engineering emergent collective functions is difficult because they arise as a consequence of complex multi-level feedback, which often spans many length-scales. Here, we present a perspective on how some of these challenges could be overcome by using multi-agent modeling as a design framework within synthetic biology. Using case studies covering the construction of synthetic ecologies to biological computation and synthetic cellularity, we show how multi-agent modeling can capture the core features of complex multi-scale systems and provide novel insights into the underlying mechanisms which guide emergent functionalities across scales. The ability to unravel design rules underpinning these behaviors offers a means to take synthetic biology beyond single molecules or cells and toward the creation of systems with functions that can only emerge from collectives at multiple scales.
Introduction
Many living organisms have evolved traits to exploit the capabilities that emerge from large interacting populations of molecules or cells, which go beyond those of the individual elements. From bacteria forming biofilms to fight antibiotic treatments to synchronizing their behaviors through quorum sensing during disease, emergent collective behaviors are pervasive in biology. Likewise, the engineering of emergent collective behaviors could offer an intriguing path to artificial biosystems with improved reliability, robustness and scalability. However, current approaches to biological design are ill-equipped for this task as they tend to focus on a single level of organization and ignore potential feedbacks between different aspects/levels of a system. A common example is the design of transcriptional gene regulatory networks where it is assumed that the function of the entire system can be understood solely by the steady state input-output transcriptional response of genetic devices (Nielsen et al., 2016). While this simplification is useful and powerful in some cases, if the genes regulated link to metabolic processes there is a chance that feedback via metabolism could break circuit function. Focusing purely on transcriptional networks makes it impossible to capture such behaviors.
In physics, great strides have been made through techniques from statistical mechanics to understand emergent phenomena. These include the Ising model used to capture magnetic phase transitions (Taroni, 2015) and the application of renormalization to understand how physical and biological constraints might underpin scaling laws that guide evolution (West et al., 2002; Kempes et al., 2019). There has also been growing interest over the past few decades in the field of complexity theory (Nicolis and Prigogine, 1989) and whether laws might exist that govern self-organization and emergence across diverse types of complex system composed of many interacting parts (Prigogine and Nicolis, 1985; Ashby, 1991; Goldstein, 1999; West et al., 2002).
An approach to capture and explore the emergent features of complex systems is multi-agent modeling (also termed agent-based or individual-based modeling) (Hellweger et al., 2016). This considers key components of a system as explicit entities/agents and allows for large and diverse interacting populations of these (Figure 1A). Specifically, a multi-agent model consists of autonomous agents that represent the lowest level components of the system. Common types of agent in biological systems include molecules, cells and whole multicellular organisms. Each agent is assigned a specific set of rules governing how it interacts with other agents and the local environment. The way these rules are modeled is flexible with the option to use basic finite state-machines, Boolean logic governing stimuli-response relationships, or more complex representations like differential equation models (e.g., capturing the biochemical reaction networks within a cell). Populations of these agents are then placed in a simulated environment that encompasses physical processes of relevance to the system. In biology, this might include the diffusion of chemicals, the flow of fluids, and the mechanical forces that cells can exert on one another. Again, the way that these environmental processes are modeled can vary, resulting in a final model that could potentially combine stochastic, deterministic, dynamic, discrete and continuous representations for different aspects of a system. The integration of such diverse modeling approaches allows for the most appropriate form of representation to be used for each aspect and helps simplify the specification of the multi-scale system, but often comes at the cost of reduced analytical tractability. Even so, multi-scale modeling has been shown capable of discovering some of the core ingredients needed for collective behaviors to emerge (Hellweger et al., 2016; Gorochowski and Richardson, 2017), but its use to date in synthetic biology has been limited (Gorochowski, 2016).
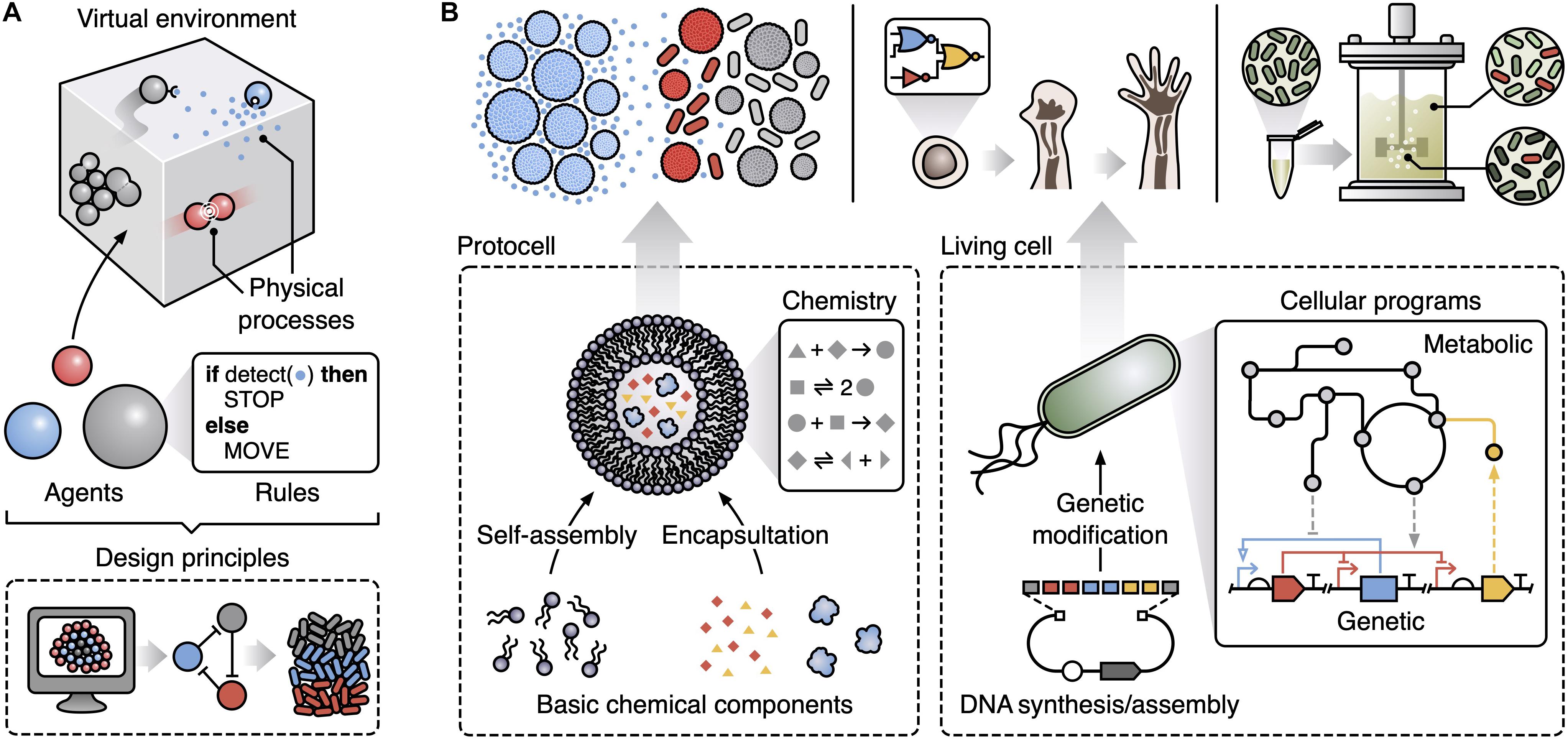
Figure 1. Multi-agent modeling can support the design of emergent collective functions in synthetic biology. (A) Key components of a multi-agent model. Populations of autonomous agents following user-prescribed rules are placed in a virtual environment that simulates relevant physical processes (e.g., physical collisions, chemical diffusion, movement, and fluid flows). Simulations of multi-agent models can be used to derive design principles that capture the basic ingredients (e.g., specific patterns of interactions) needed for a particular emergent behavior. (B) Potential applications of multi-agent modeling within synthetic biology and the underlying agents (bottom, dashed boxes) used to generate specific emergent collective behaviors: (top left) exploring how to create life-like behaviors from basic chemical components with sender protocells (blue) able to spatially propagate a signal to receiver protocells and bacteria (gray when inactive, red when active) using a small diffusive chemical (small blue dots); (top middle) understanding the developmental programs used during morphogenesis as a step toward the creation of synthetic multi-cellular life; (top right) improving scale-up of microbial fermentations by accounting for heterogeneity across a bioreactor and designing engineered microbes able to robustly function under these conditions.
Here, we aim to highlight some of the key areas of synthetic biology where multi-agent modeling offers a unique way to tackle longstanding problems (Figure 1B). While the examples we cover are diverse, they all share a core characteristic: the emergence of behaviors in the systems cannot be explained by looking solely at their basic parts in isolation. This essence makes such systems special yet difficult to engineer via traditional means. We propose to extend bioengineering methods to encompass principles gleaned from multi-agent models and use them to guide the design of synthetic biological systems displaying emergent phenomena. We end by discussing some of the practical challenges when using multi-agent modeling in synthetic biology and future directions for the marriage of these fields.
Understanding the Emergence of Life
When considering emergent phenomena, the quintessential example is the emergence of life. Putting aside the difficulty of defining precisely what life is, the ability of living systems to self-replicate and create order/information out of chaos is an inspiration for many engineers. Bottom-up synthetic biology attempts to build chemical systems that display life-like behaviors using a minimal set of components. The hope is that these simplified systems might help us understand how life emerged from first principles.
One attempt to reach this goal has been via the synthesis of artificial cells (protocells) with life-like properties. This requires the ability to bridge length scales by harnessing molecular self-assembly to create micron-sized compartments (Bayley et al., 2008; Li et al., 2014) and the intricate interactions between molecules and enzymes to form biochemical reaction networks (Hasty et al., 2002). The incorporation of these reaction networks within protocells has also been demonstrated (Adamala et al., 2017; Joesaar et al., 2019) and although chemically simple, such systems display an array of dynamical behaviors including pattern formation (Niederholtmeyer et al., 2015; Zadorin et al., 2017) and replication via controlled growth and division (Chen et al., 2004). By combining these systems with additional chemical modules and parts, this may offer a route to creating other key behaviors of living systems.
Building on these capabilities, functionalities can be scaled further by constructing systems composed of populations of protocells or through interacting natural and artificial cellular communities (Lentini et al., 2014; Adamala et al., 2017; Tang et al., 2018). While such extensions offer a promising platform for probing emergent behaviors using simple self-contained chemical units, it is difficult to know what parameters to engineer into these systems and the level of complexity required to drive a desired collective behavior. This is where multi-agent modeling, in combination with more traditional models of chemical reaction systems, could lead to a quantitative understanding of the key elements needed for the emergence of life-like behaviors. In particular, multi-agent models would allow for the rapid exploration of potential systems using physically realistic parameters until the right combination of parts was found that resulted in a desired emergent functionality.
Historically, mathematical models developed using differential equations have proved effective for understanding the dynamics of minimal chemical systems (Rovinskii and Zhabotinskii, 1984) and are widely and successfully used for modeling all types of biological system (Ellner and Guckenheimer, 2011; Raue et al., 2013). Furthermore, the application of bifurcation analysis to these dynamical models enables the rigorous characterization of emergent phenomena such as bi-stability, symmetry breaking, non-linear oscillations, chaos, and pattern formation (Kuznetsov, 2004). However, while it is possible to use partial differential equations (PDEs) to capture spatial aspects of a system, the high levels of heterogeneity in the complex environments of many biological system (e.g., cellular tissues) and the ability of both agents and the rules to change over time, can make practical use of PDEs a challenge (Hellweger et al., 2016; Perez-Carrasco et al., 2016; Glen et al., 2019).
In comparison, multi-agent modeling is able to explicitly capture such variation and consider simplified rules to express internal chemical reactions altering specific characteristics of each component. Due to the chemical simplicity and programmability of minimal protocells, this abstraction is a good fit, allowing iterative refinement of model and experimental system. For example, due to the limited number of possible chemical reactions present in a minimal system, comprehensive direct measurements can be made to create highly predictive rules for how a protocell’s chemical state will change over time. These can then drive simulations of accurate protocell behaviors in a multi-agent model to explore the specific combination of reactions required for the emergence of higher population-level functionalities. This two-way cycle of development would be difficult, if not impossible, when using natural cells where complex evolutionary baggage masks those features essential for emergence.
Distributed Computation During Development
Living cells continually monitor their environment and adapt their physiology in order to survive. This requires the processing of information gathered from sensors to make suitable changes to gene expression. Synthetic biology enables us to reprogram cells by writing our own genetic programs to exploit the cells’ computational capabilities in new ways (Greco et al., 2019; Grozinger et al., 2019). So far, the majority of research in biological computation has revolved around the concept of genetic circuits and attempted to repurpose tools and methodologies from electronic circuit design (Nielsen et al., 2016; Gorochowski et al., 2017) and automatic verification (Dunn et al., 2014). While this approach has enabled the automated design of cellular programs able to perform basic logic, much of the information processing in native biological systems is distributed, relying on collective decision making (e.g., quorum sensing) and interactions between large numbers of cells.
This feature is most evident in developmental biology where robust genetic programs must ensure that a complex multi-cellular organism emerges from a single cell. Cell growth, differentiation, migration and self-organization are coordinated by a developmental program with dynamics at both the intra- and inter-cellular levels. These enable the generation of precise deterministic patterns from stochastic underlying processes (Glen et al., 2019). In contrast to simple logic circuits, the complexity of the molecular interactions and mechanical forces underpinning these processes motivate the use of multi-agent modeling to better understand how developmental programs work in morphogenetic systems. In particular, multi-agent models are able to capture the role of cellular heterogeneity, proliferation and morphology, mechanical and environmental cues, movement of cells as well as the integration of multiple processes at diverse scales and the feedback between these (Montes-Olivas et al., 2019). Such models have helped deepen our understanding of early mammalian embryogenesis (Godwin et al., 2017), as well as the formation of vascular networks (Perfahl et al., 2017) and other complex structures and organs, including the skin, lung (Stopka et al., 2019), kidney (Lambert et al., 2018), and brain (Caffrey et al., 2014).
Although such work has provided insights into the computational architecture of native developmental programs, it has been difficult to apply this information to the creation of de novo morphogenetic systems because of a limited toolkit of parts available to build such systems. Synthetic biology may help solve this issue by facilitating the engineering of simplified multi-cellular systems (Velazquez et al., 2018) that implement developmental programs encompassing distributed feedback regulation (Ausländer and Fussenegger, 2016) and cell-to-cell communication (Bacchus et al., 2012), to better understand how these factors can be used to contribute to emergent self-organization (Morsut et al., 2016).
Collective Phenomena Driving Disease
Many of the challenges treating diseases result from the malfunction of emergent multi-cellular properties, be it carcinogenesis (Deisboeck and Couzin, 2009; Ward et al., 2020), viral infection (Jacob et al., 2004), bacterial biofilm formation (Wu et al., 2020) or microbiome imbalances (Shreiner et al., 2015; Kumar et al., 2019). Multi-agent modeling of these conditions has helped demystify how the collective behavior of large numbers of diverse cells and their interactions with each other and their environment can lead to negative clinical outcomes.
Cancer is a complex multi-scale disease that includes environmental factors, genetic mutations and clonal selection, and complex interactions with the immune and vascular system. As a result, computational models of cancer need to account for many of these factors considering the heterogeneity and interactions of single cells, yet contain sufficient numbers of them to predict emergent phenomena at a tumor scale (Metzcar et al., 2019). Using this approach, multi-agent models have been used to help understand metastasis (Waclaw et al., 2015) and show that cancer cells with stem cell-like properties can be a key determinant in cancer progression with fatal consequences (Scott et al., 2016, 2019).
Beyond understanding the emergence of some diseases, multi-agent models can also identify novel ways of fixing their dynamics by considering how to disrupt cellular behaviors, and their interactions in space and time (Waclaw et al., 2015; Gallaher et al., 2018). Treatments themselves can even be designed to have collective emergent properties. For example, bacteria have already been engineered to use quorum sensing to trigger their delivery of drugs (Din et al., 2016) or they can be controlled using magnetic fields to penetrate cancerous tissue (Schuerle et al., 2019). Other collective behaviors used in cancer nanomedicine include self-assembly of nanoparticles to anchor imaging agents in tumors, disassembly of nanoparticles to increase tissue penetration, nanoparticles that compute the state of a tumor, nanoparticle-based remodeling of tumor environments to improve secondary nanoparticle transport, or nanoparticle signaling of tumor location to amplify the accumulation of nanoparticles in tumors (Hauert et al., 2013; Hauert and Bhatia, 2014).
The emergent properties inherent in many diseases, and the potential to harness such behaviors for new treatments, highlight the need for multi-scale modeling tools. Moreover, with the rapidly expanding field of “systems medicine,” integrated modeling pipelines able to predict multi-scale disease dynamics and assess novel synthetic biology treatments via large-scale simulation and machine learning are positioned to revolutionize many areas of medicine (Stillman et al., 2020).
Challenges in Scaling-Up Biotechnology
The ability for synthetic biology to reprogram cellular metabolisms offers an opportunity to convert cheap substrates (or even waste) into valuable chemicals and materials via microbial fermentation (Nielsen and Keasling, 2016). To make this economically viable, large bioreactors are often used. However, while our use of fermentation stems back millennia (McGovern et al., 2004), we still struggle to reliably scale-up many processes from shake flasks in the lab to industrial-sized bioreactors (Lee and Kim, 2015).
A major reason for this problem is the increasing difficulty and power consumption of mixing or aerating reactors as their volume increases, causing pockets to form where nutrient concentration, temperature, oxygen, pH and other factors differ (Alvarez et al., 2005). As a microbe travels through the bioreactor, it becomes exposed to a wide variety of environments, each causing changes in its physiology. Because the path of each cell is unique, a population of cells will display a wide variety of physiological states. This differs from lab-scale experiments where environments are well-mixed and homogeneous, and causes predictions made from these conditions to significantly deviate from those observed during scale-up.
Capturing the combined environmental and cellular variability present in a large bioreactor is difficult using standard differential-equation models. In contrast, multi-agent models are able to explicitly capture and link gene regulation, metabolism, and the cells’ local environment (Nieß et al., 2017; Haringa et al., 2018), as well as differences between individual cells and how cells change over time (González-Cabaleiro et al., 2017). In particular, hybrid models in which continuous descriptions of complex physical processes like fluid flows have been coupled with multi-agent models to allow for the efficient simulation of these systems. This approach can accurately predict the emergence of population heterogeneity and overall production rates and help guide bioreactor design to further improve yields (Haringa et al., 2018). Some attempts have also been made to use control engineering principles to design cellular systems able to adapt to fluctuating environments (Hsiao et al., 2018). To date, these attempts have mostly focused on the basic genetic parts and regulatory motifs (e.g., negative feedback) needed to implement control algorithms (Ceroni et al., 2018; Aoki et al., 2019; Pedone et al., 2019; Bartoli et al., 2020). Moving forward, multi-agent models offer a means to make simulations of these systems more realistic by accurately capturing how individual cells and their complex environment change over time.
Another challenge faced during large-scale fermentation is the opportunity for mutants to arise or unwanted microbes to contaminate a process and out-compete their engineered counterparts (Kazamia et al., 2012; Louca and Doebeli, 2016). Multi-agent models of these complex environments and local competition when multiple types of organism are present, could help support the development of evolutionarily stable strategies (ESSs) that prevent the replacement of an engineered population by competitors (Schuster et al., 2010).
Engineering Synthetic Ecologies
At an even larger organizational level, synthetic biologists have begun to explore how to engineer interactions between communities to enable the future construction of synthetic ecologies (Ben Said and Or, 2017). With climate change, pollution and many other factors leading to the degradation of ecological systems, understanding how these systems emerge and function is crucial. Such knowledge would allow for effective restoration strategies (Solé et al., 2015) and potentially offer means to terraform other planets like Mars for future human inhabitation (Conde-Pueyo et al., 2020).
These applications require an understanding of how diverse organisms interact to create stable communities (Widder et al., 2016). This is difficult because the interactions that take place at the level of a population are governed by choices made by single organisms (Kreft et al., 2017). By using multi-agent modeling to rapidly test combinations of cell types, behaviors and interactions, and synthetic biology tools to engineer real-world microbial communities, it might become possible to design and test hypotheses regarding the principles for robust ecosystem design. For example, multi-agent modeling has been used to help understand how signaling and mutual cooperation can stabilize microbial communities (Kerényi et al., 2013). Furthermore, from a synthetic biology perspective many of the tools needed to engineer these systems already exist, e.g., biological parts able to implement cooperation (Shou et al., 2007), signaling (Bacchus et al., 2012), targeted death (Fedorec et al., 2019), and collective decision making (e.g., quorum sensing).
Beyond engineering interactions between organisms, spatial structure can also play a crucial role in the functionalities of microbial communities. Multi-agent modeling has demonstrated the significant impact that spatio-temporal organization can have on soil microbes and the success of auxotrophic interactions (Jiang et al., 2018). Such interactions are particularly important for engineering minimal functional synthetic communities as plant seed treatments and for vertical farming under defined conditions. In this context, whether or not a single cell or division of labor is the evolutionarily stable solution depends on the metabolic flux through the system, with high flux favoring division of labor (Kreft et al., 2020). Extending this modeling approach further to consider the thermodynamics of microbial growth and redox biochemistry could help ensure that resultant systems are ecologically and evolutionarily stable (Zerfaß et al., 2018). Alternatively, external control of the environment could be used to forcibly maintain a desired community structure (Treloar et al., 2020). In all cases, a combination of multi-agent modeling and engineerable biological systems provides a unique means to unravel how these complex systems function.
External feedback control has been proposed as another approach to control of cellular communities. By employing real-time single cell measurements (e.g., by time-lapse microscopy or flow-cytometry) and experimental systems able to send control signals to the cells via optogenetics (Toettcher et al., 2011) or chemical release in microfluidics (Menolascina et al., 2014), a computer can monitor and signal to a population of cells in order to maintain a desired behavior (e.g., the expression rate of a protein). More recently, it has been proposed to implement these control algorithms directly into cells, with the key aim of distributing tasks among different strains (Fiore et al., 2017; McCardell et al., 2017). Multi-agent modeling can be instrumental in the design of robust feedback mechanisms across multicellular populations, as it can reveal non-obvious effects of cell density, proliferation dynamics and spatial constraints on the effectiveness of control actions (Fiore et al., 2017).
Discussion
We have shown how multi-agent models can be applied to many areas of synthetic biology. The core features of these models provide insight into some of the basic building blocks and mechanisms needed for collective behaviors to emerge and, we believe, may offer a means to support the future predictive design of collective behaviors.
A major hurdle to the widespread use of multi-agent modeling is the need to define and simulate complex models (Grimm et al., 2006). Although computational frameworks have been available since the 1980s to support this process, it is only during the past decade that tools have been tailored for synthetic biology applications and reached sufficient performance (Gorochowski et al., 2012; Oishi and Klavins, 2014; Goñi-Moreno and Amos, 2015). More recently, the effective use of highly parallel computing resources has expanded the complexity of biological models that can be simulated (Rudge et al., 2012; Naylor et al., 2017; Li et al., 2019; Cooper et al., 2020). Automated coarse-graining of representations enable faster simulation without impacting on the accuracy of predictions (Graham et al., 2017), while advanced tools allow verification, validation and uncertainty quantification for such simulations (Richardson et al., 2020).
Improved simulations do not only speed up the time to an answer but may open up opportunities to create new types of computational design environments. For example, high-performance models coupled to virtual reality allow for multiple researchers to interactively manipulate a system and immediately observe the outcomes of their design decisions. Such capabilities have already begun to be used for molecular design (O’Connor et al., 2018) and when coupled to machine learning, offer a unique setting in which to explore complex high-dimensional datasets that are common in biology. They also allow for essential features to be distilled that can then be used to guide predictive design. Furthermore, hybrid approaches become possible where computational models dynamically augment an experimental setup by controlling physical features such as light (Rubio Denniss et al., 2019) or magnetism (Carlsen et al., 2014). If agents within the experimental system are responsive to these stimuli, then various forms of interaction can be externally programmed and rapidly explored to better understand the necessary conditions for a particular collective behavior to emerge. Once a desired set of rules for the interactions is found, the agents can be modified to implement these autonomously, removing the need for external control.
As synthetic biology moves beyond simple parts and circuits, and toward large-scale/multicellular systems, the available repertoire of design tools must also expand to support new requirements. Multi-agent modeling is perfectly placed to help make this leap and usher in new biological design methods focused on the engineering of emergent collective behaviors. Not only will this allow functionalities to span length scales, but it will also provide a way to engineer across the organizational levels of life through hierarchical composition of multi-scale models, from basic molecules and cells through to entire ecosystems.
Author Contributions
TG, SH, J-UK, LM, NS, and T-YT wrote the manuscript. All other authors helped with editing and provided the feedback.
Conflict of Interest
The authors declare that the research was conducted in the absence of any commercial or financial relationships that could be construed as a potential conflict of interest.
Funding
This work captured discussions between participants at the “Multi-agent modeling meets synthetic biology” workshop held on the 16–17 May 2019 at the University of Bristol, UK and funded by BrisSynBio, a BBSRC/EPSRC Synthetic Biology Research Centre (Grant No. BB/L01386X/1). TG was supported by a Royal Society University Research Fellowship (Grant No. UF160357). DD and VB were supported by the University of Bristol and the EPSRC & BBSRC Centre for Doctoral Training in Synthetic Biology (Grant No. EP/L016494/1). LB was supported by EPSRC (Grant No. EP/P017134/1-CONDSYC). AF received funding from the European Research Council under the European Union’s Horizon 2020 Research and Innovation Programme (Grant No. 770835). LM was supported by the Medical Research Council (Grant No. MR/N021444/1), and the Engineering and Physical Sciences Research Council (Grant Nos. EP/R041695/1 and EP/S01876X/1). SM was supported by a Mexico Consejo Nacional de Ciencia y Tecnología (CONACYT) Ph.D. scholarship. TF and J-UK are grateful to the UK National Centre for the Replacement, Refinement & Reduction of Animals in Research (NC3Rs) for funding their development of individual-based models (IBMs) for the gut environment (eGUT Grant No. NC/K000683/1 and Ph.D. training Grant No. NC/R001707/1). SH, NS, and SM received funding from the European Union’s Horizon 2020 FET Open programme (Grant No. 800983). T-YT acknowledged financial support from the MaxSynBio Consortium (jointly funded by the Federal Ministry of Education and Research, Germany and the Max Planck Society) and the MPI−CBG and the Cluster of Excellence Physics of Life of TU Dresden and EXC-1056 for funding.
References
Adamala, K. P., Martin-Alarcon, D. A., Guthrie-Honea, K. R., and Boyden, E. S. (2017). Engineering genetic circuit interactions within and between synthetic minimal cells. Nat. Chem. 9, 431–439. doi: 10.1038/nchem.2644
Alvarez, M. M., Guzmán, A., and Elías, M. (2005). Experimental visualization of mixing pathologies in laminar stirred tank bioreactors. Chem. Eng. Sci. 60, 2449–2457. doi: 10.1016/j.ces.2004.11.049
Aoki, S. K., Lillacci, G., Gupta, A., Baumschlager, A., Schweingruber, D., and Khammash, M. (2019). A universal biomolecular integral feedback controller for robust perfect adaptation. Nature 570, 533–537. doi: 10.1038/s41586-019-1321-1
Ashby, W. R. (1991). “Principles of the self-organizing system,” in Facets of Systems Science, ed. G. J. Klir (Boston, MA: Springer US), 521–536. doi: 10.1007/978-1-4899-0718-9_38
Ausländer, S., and Fussenegger, M. (2016). Engineering gene circuits for mammalian cell–based applications. Cold Spring Harb. Perspect. Biol. 8:a023895. doi: 10.1101/cshperspect.a023895
Bacchus, W., Lang, M., El-Baba, M. D., Weber, W., Stelling, J., and Fussenegger, M. (2012). Synthetic two-way communication between mammalian cells. Nat. Biotechnol. 30, 991–996. doi: 10.1038/nbt.2351
Bartoli, V., Meaker, G. A., di Bernardo, M., and Gorochowski, T. E. (2020). Tunable genetic devices through simultaneous control of transcription and translation. Nat. Commun. 11:2095. doi: 10.1038/s41467-020-15653-7
Bayley, H., Cronin, B., Heron, A., Holden, M. A., Hwang, W. L., Syeda, R., et al. (2008). Droplet interface bilayers. Mol. Biosyst. 4, 1191–1208. doi: 10.1039/B808893D
Ben Said, S., and Or, D. (2017). Synthetic microbial ecology: engineering habitats for modular consortia. Front. Microbiol. 8:1125. doi: 10.3389/fmicb.2017.01125
Caffrey, J. R., Hughes, B. D., Britto, J. M., and Landman, K. A. (2014). An in silico agent-based model demonstrates Reelin function in directing lamination of neurons during cortical development. PLoS One 9:e110415. doi: 10.1371/journal.pone.0110415
Carlsen, R. W., Edwards, M. R., Zhuang, J., Pacoret, C., and Sitti, M. (2014). Magnetic steering control of multi-cellular bio-hybrid microswimmers. Lab. Chip 14, 3850–3859. doi: 10.1039/C4LC00707G
Ceroni, F., Boo, A., Furini, S., Gorochowski, T. E., Borkowski, O., Ladak, Y. N., et al. (2018). Burden-driven feedback control of gene expression. Nat. Methods 15, 387–393. doi: 10.1038/nmeth.4635
Chen, I. A., Roberts, R. W., and Szostak, J. W. (2004). The emergence of competition between model protocells. Science 305:1474. doi: 10.1126/science.1100757
Conde-Pueyo, N., Vidiella, B., Sardanyés, J., Berdugo, M., Maestre, T. F., de Lorenzo, V., et al. (2020). Synthetic biology for terraformation lessons from mars, earth, and the microbiome. Life 10:14. doi: 10.3390/life10020014
Cooper, F. R., Baker, R. E., Bernabeu, M. O., Bordas, R., Bowler, L., Bueno-Orovio, A., et al. (2020). Chaste: cancer, heart and soft tissue environment. J. Open Source Softw. 5:1848. doi: 10.21105/joss.01848
Deisboeck, T. S., and Couzin, I. D. (2009). Collective behavior in cancer cell populations. BioEssays 31, 190–197. doi: 10.1002/bies.200800084
Din, M. O., Danino, T., Prindle, A., Skalak, M., Selimkhanov, J., Allen, K., et al. (2016). Synchronized cycles of bacterial lysis for in vivo delivery. Nature 536, 81–85. doi: 10.1038/nature18930
Dunn, S.-J., Martello, G., Yordanov, B., Emmott, S., and Smith, A. G. (2014). Defining an essential transcription factor program for naïve pluripotency. Science 344:1156. doi: 10.1126/science.1248882
Ellner, S. P., and Guckenheimer, J. (2011). Dynamic Models in Biology. Princeton, NJ: Princeton University Press.
Fedorec, A. J. H., Karkaria, B. D., Sulu, M., and Barnes, C. P. (2019). Killing in response to competition stabilises synthetic microbial consortia. bioRxiv [Preprint]. doi: 10.1101/2019.12.23.887331
Fiore, G., Matyjaszkiewicz, A., Annunziata, F., Grierson, C., Savery, N. J., Marucci, L., et al. (2017). In-Silico analysis and implementation of a multicellular feedback control strategy in a synthetic bacterial consortium. ACS Synth. Biol. 6, 507–517. doi: 10.1021/acssynbio.6b00220
Gallaher, J. A., Enriquez-Navas, P. M., Luddy, K. A., Gatenby, R. A., and Anderson, A. R. A. (2018). Spatial heterogeneity and evolutionary dynamics modulate time to recurrence in continuous and adaptive cancer therapies. Cancer Res. 78:2127. doi: 10.1158/0008-5472.CAN-17-2649
Glen, C. M., Kemp, M. L., and Voit, E. O. (2019). Agent-based modeling of morphogenetic systems: advantages and challenges. PLoS Comput. Biol. 15:e1006577. doi: 10.1371/journal.pcbi.1006577
Godwin, S., Ward, D., Pedone, E., Homer, M., Fletcher, A. G., and Marucci, L. (2017). An extended model for culture-dependent heterogenous gene expression and proliferation dynamics in mouse embryonic stem cells. Npj Syst. Biol. Appl. 3:19. doi: 10.1038/s41540-017-0020-5
Goldstein, J. (1999). Emergence as a construct: history and issues. Emergence 1, 49–72. doi: 10.1207/s15327000em0101_4
Goñi-Moreno, A., and Amos, M. (2015). “DiSCUS: a simulation platform for conjugation computing,” in Unconventional Computation and Natural Computation, eds C. S. Calude and M. J. Dinneen (Cham: Springer International Publishing), 181–191. doi: 10.1007/978-3-319-21819-9_13
González-Cabaleiro, R., Mitchell, A. M., Smith, W., Wipat, A., and Ofiţeru, I. D. (2017). Heterogeneity in pure microbial systems: experimental measurements and modeling. Front. Microbiol. 8:1813. doi: 10.3389/fmicb.2017.01813
Gorochowski, T. E. (2016). Agent-based modelling in synthetic biology. Essays Biochem. 60:325. doi: 10.1042/EBC20160037
Gorochowski, T. E., Espah Borujeni, A., Park, Y., Nielsen, A. A., Zhang, J., Der, B. S., et al. (2017). Genetic circuit characterization and debugging using RNA-seq. Mol. Syst. Biol. 13:952. doi: 10.15252/msb.20167461
Gorochowski, T. E., Matyjaszkiewicz, A., Todd, T., Oak, N., Kowalska, K., Reid, S., et al. (2012). BSim: an agent-based tool for modeling bacterial populations in systems and synthetic biology. PLoS One 7:e42790. doi: 10.1371/journal.pone.0042790
Gorochowski, T. E., and Richardson, T. O. (2017). “How behaviour and the environment influence transmission in mobile groups,” in Temporal Network Epidemiology, eds N. Masuda and P. Holme (Singapore: Springer), 17–42. doi: 10.1007/978-981-10-5287-3_2
Graham, J. A., Essex, J. W., and Khalid, S. (2017). PyCGTOOL: automated generation of coarse-grained molecular dynamics models from atomistic trajectories. J. Chem. Inf. Model. 57, 650–656. doi: 10.1021/acs.jcim.7b00096
Greco, F. V., Tarnowski, M. J., and Gorochowski, T. E. (2019). Living computers powered by biochemistry. Biochemist 41, 14–18. doi: 10.1042/bio04103014
Grimm, V., Berger, U., Bastiansen, F., Eliassen, S., Ginot, V., Giske, J., et al. (2006). A standard protocol for describing individual-based and agent-based models. Ecol. Model. 198, 115–126. doi: 10.1016/j.ecolmodel.2006.04.023
Grozinger, L., Amos, M., Gorochowski, T. E., Carbonell, P., Oyarzún, D. A., Stoof, R., et al. (2019). Pathways to cellular supremacy in biocomputing. Nat. Commun. 10:5250. doi: 10.1038/s41467-019-13232-z
Haringa, C., Tang, W., Wang, G., Deshmukh, A. T., van Winden, W. A., Chu, J., et al. (2018). Computational fluid dynamics simulation of an industrial P. chrysogenum fermentation with a coupled 9-pool metabolic model: towards rational scale-down and design optimization. Chem. Eng. Sci. 175, 12–24. doi: 10.1016/j.ces.2017.09.020
Hasty, J., McMillen, D., and Collins, J. J. (2002). Engineered gene circuits. Nature 420, 224–230. doi: 10.1038/nature01257
Hauert, S., Berman, S., Nagpal, R., and Bhatia, S. N. (2013). A computational framework for identifying design guidelines to increase the penetration of targeted nanoparticles into tumors. Nano Today 8, 566–576. doi: 10.1016/j.nantod.2013.11.001
Hauert, S., and Bhatia, S. N. (2014). Mechanisms of cooperation in cancer nanomedicine: towards systems nanotechnology. Trends Biotechnol. 32, 448–455. doi: 10.1016/j.tibtech.2014.06.010
Hellweger, F. L., Clegg, R. J., Clark, J. R., Plugge, C. M., and Kreft, J.-U. (2016). Advancing microbial sciences by individual-based modelling. Nat. Rev. Microbiol. 14, 461–471. doi: 10.1038/nrmicro.2016.62
Hsiao, V., Swaminathan, A., and Murray, R. M. (2018). Control theory for synthetic biology: recent advances in system characterization, control design, and controller implementation for synthetic biology. IEEE Control Syst. Mag. 38, 32–62. doi: 10.1109/mcs.2018.2810459
Jacob, C., Litorco, J., and Lee, L. (2004). “Immunity through swarms: agent-based simulations of the human immune system,” in Artificial Immune Systems, eds G. Nicosia, V. Cutello, P. J. Bentley, and J. Timmis (Berlin: Springer), 400–412. doi: 10.1007/978-3-540-30220-9_32
Jiang, X., Zerfaß, C., Feng, S., Eichmann, R., Asally, M., Schäfer, P., et al. (2018). Impact of spatial organization on a novel auxotrophic interaction among soil microbes. ISME J. 12, 1443–1456. doi: 10.1038/s41396-018-0095-z
Joesaar, A., Yang, S., Bögels, B., van der Linden, A., Pieters, P., Kumar, B. V. V. S. P., et al. (2019). DNA-based communication in populations of synthetic protocells. Nat. Nanotechnol. 14, 369–378. doi: 10.1038/s41565-019-0399-9
Kazamia, E., Aldridge, D. C., and Smith, A. G. (2012). Synthetic ecology – A way forward for sustainable algal biofuel production? Photosynth. Microorg. Bio Fuel Prod. Sun Light 162, 163–169. doi: 10.1016/j.jbiotec.2012.03.022
Kempes, C. P., Koehl, M. A. R., and West, G. B. (2019). The scales that limit: the physical boundaries of evolution. Front. Ecol. Evol. 7:242. doi: 10.3389/fevo.2019.00242
Kerényi, Á, Bihary, D., Venturi, V., and Pongor, S. (2013). Stability of multispecies bacterial communities: signaling networks may stabilize microbiomes. PLoS One 8:e57947. doi: 10.1371/journal.pone.0057947
Kreft, J.-U., Griffin, B. M., and González-Cabaleiro, R. (2020). Evolutionary causes and consequences of metabolic division of labour: why anaerobes do and aerobes don’t. Curr. Opin. Biotechnol. 62, 80–87. doi: 10.1016/j.copbio.2019.08.008
Kreft, J.-U., Plugge, C. M., Prats, C., Leveau, J. H. J., Zhang, W., and Hellweger, F. L. (2017). From genes to ecosystems in microbiology: modeling approaches and the importance of individuality. Front. Microbiol. 8:2299. doi: 10.3389/fmicb.2017.02299
Kumar, M., Ji, B., Zengler, K., and Nielsen, J. (2019). Modelling approaches for studying the microbiome. Nat. Microbiol. 4, 1253–1267. doi: 10.1038/s41564-019-0491-9
Lambert, B., MacLean, A. L., Fletcher, A. G., Combes, A. N., Little, M. H., and Byrne, H. M. (2018). Bayesian inference of agent-based models: a tool for studying kidney branching morphogenesis. J. Math. Biol. 76, 1673–1697. doi: 10.1007/s00285-018-1208-z
Lee, S. Y., and Kim, H. U. (2015). Systems strategies for developing industrial microbial strains. Nat. Biotechnol. 33, 1061–1072. doi: 10.1038/nbt.3365
Lentini, R., Santero, S. P., Chizzolini, F., Cecchi, D., Fontana, J., Marchioretto, M., et al. (2014). Integrating artificial with natural cells to translate chemical messages that direct E. coli behaviour. Nat. Commun. 5:4012. doi: 10.1038/ncomms5012
Li, B., Taniguchi, D., Gedara, J. P., Gogulancea, V., Gonzalez-Cabaleiro, R., Chen, J., et al. (2019). NUFEB: a massively parallel simulator for individual-based modelling of microbial communities. PLoS Comput. Biol. 15:e1007125. doi: 10.1371/journal.pcbi.1007125
Li, M., Huang, X., Tang, T.-Y. D., and Mann, S. (2014). Synthetic cellularity based on non-lipid micro-compartments and protocell models. Synth. Biol. Synth. Biomol. 22, 1–11. doi: 10.1016/j.cbpa.2014.05.018
Louca, S., and Doebeli, M. (2016). Transient dynamics of competitive exclusion in microbial communities. Environ. Microbiol. 18, 1863–1874. doi: 10.1111/1462-2920.13058
McCardell, R. D., Huang, S., Green, L. N., and Murray, R. M. (2017). Control of bacterial population density with population feedback and molecular sequestration. bioRxiv [Preprint]. doi: 10.1101/225045
McGovern, P. E., Zhang, J., Tang, J., Zhang, Z., Hall, G. R., Moreau, R. A., et al. (2004). Fermented beverages of pre- and proto-historic China. Proc. Natl. Acad. Sci. U.S.A. 101:17593. doi: 10.1073/pnas.0407921102
Menolascina, F., Fiore, G., Orabona, E., De Stefano, L., Ferry, M., Hasty, J., et al. (2014). In-Vivo real-time control of protein expression from endogenous and synthetic gene networks. PLoS Comput. Biol. 10:e1003625. doi: 10.1371/journal.pcbi.1003625
Metzcar, J., Wang, Y., Heiland, R., and Macklin, P. (2019). A review of cell-based computational modeling in cancer biology. JCO Clin. Cancer Inform. 3, 1–13. doi: 10.1200/CCI.18.00069
Montes-Olivas, S., Marucci, L., and Homer, M. (2019). Mathematical models of organoid cultures. Front. Genet. 10:873. doi: 10.3389/fgene.2019.00873
Morsut, L., Roybal, K. T., Xiong, X., Gordley, R. M., Coyle, S. M., Thomson, M., et al. (2016). Engineering customized cell sensing and response behaviors using synthetic notch receptors. Cell 164, 780–791. doi: 10.1016/j.cell.2016.01.012
Naylor, J., Fellermann, H., Ding, Y., Mohammed, W. K., Jakubovics, N. S., Mukherjee, J., et al. (2017). Simbiotics: a multiscale integrative platform for 3D modeling of bacterial populations. ACS Synth. Biol. 6, 1194–1210. doi: 10.1021/acssynbio.6b00315
Nicolis, G., and Prigogine, I. (1989). Exploring Complexity: An Introduction. New York, NY: W.H. Freeman and Company.
Niederholtmeyer, H., Sun, Z. Z., Hori, Y., Yeung, E., Verpoorte, A., Murray, R. M., et al. (2015). Rapid cell-free forward engineering of novel genetic ring oscillators. eLife 4:e09771. doi: 10.7554/eLife.09771
Nielsen, A. A. K., Der, B. S., Shin, J., Vaidyanathan, P., Paralanov, V., Strychalski, E. A., et al. (2016). Genetic circuit design automation. Science 352:aac7341. doi: 10.1126/science.aac7341
Nielsen, J., and Keasling, J. D. (2016). Engineering cellular metabolism. Cell 164, 1185–1197. doi: 10.1016/j.cell.2016.02.004
Nieß, A., Löffler, M., Simen, J. D., and Takors, R. (2017). Repetitive short-term stimuli imposed in poor mixing zones induce long-term adaptation of E. coli cultures in large-scale bioreactors: experimental evidence and mathematical model. Front. Microbiol. 8:1195. doi: 10.3389/fmicb.2017.01195
O’Connor, M., Deeks, H. M., Dawn, E., Metatla, O., Roudaut, A., Sutton, M., et al. (2018). Sampling molecular conformations and dynamics in a multiuser virtual reality framework. Sci. Adv. 4:eaat2731. doi: 10.1126/sciadv.aat2731
Oishi, K., and Klavins, E. (2014). Framework for engineering finite state machines in gene regulatory networks. ACS Synth. Biol. 3, 652–665. doi: 10.1021/sb4001799
Pedone, E., Postiglione, L., Aulicino, F., Rocca, D. L., Montes-Olivas, S., Khazim, M., et al. (2019). A tunable dual-input system for on-demand dynamic gene expression regulation. Nat. Commun. 10:4481. doi: 10.1038/s41467-019-12329-9
Perez-Carrasco, R., Guerrero, P., Briscoe, J., and Page, K. M. (2016). Intrinsic noise profoundly alters the dynamics and steady state of morphogen-controlled bistable genetic switches. PLoS Comput. Biol. 12:e1005154. doi: 10.1371/journal.pcbi.1005154
Perfahl, H., Hughes, B. D., Alarcón, T., Maini, P. K., Lloyd, M. C., Reuss, M., et al. (2017). 3D hybrid modelling of vascular network formation. J. Theor. Biol. 414, 254–268. doi: 10.1016/j.jtbi.2016.11.013
Prigogine, I., and Nicolis, G. (1985). “Self-organisation in nonequilibrium systems: towards a dynamics of complexity,” in Bifurcation Analysis: Principles, Applications and Synthesis, eds M. Hazewinkel, R. Jurkovich, and J. H. P. Paelinck (Dordrecht: Springer Netherlands), 3–12. doi: 10.1007/978-94-009-6239-2_1
Raue, A., Schilling, M., Bachmann, J., Matteson, A., Schelke, M., Kaschek, D., et al. (2013). lessons learned from quantitative dynamical modeling in systems biology. PLoS One 8:e74335. doi: 10.1371/journal.pone.0074335
Richardson, R. A., Wright, D. W., Edeling, W., Jancauskas, V., Lakhlili, J., and Coveney, P. V. (2020). EasyVVUQ: a library for verification, validation and uncertainty quantification in high performance computing. J. Open Res. Softw. 8:11. doi: 10.5334/jors.303
Rovinskii, A. B., and Zhabotinskii, A. M. (1984). Mechanism and mathematical model of the oscillating bromate-ferroin-bromomalonic acid reaction. J. Phys. Chem. 88, 6081–6084. doi: 10.1021/j150669a001
Rubio Denniss, A. M., Gorochowski, T. E., and Hauert, S. (2019). “Augmented reality for the engineering of collective behaviours in microsystems,” in Proceedings of the 2019 International Conference on Manipulation, Automation and Robotics at Small Scales (MARSS), Helsinki.
Rudge, T. J., Steiner, P. J., Phillips, A., and Haseloff, J. (2012). Computational modeling of synthetic microbial biofilms. ACS Synth. Biol. 1, 345–352. doi: 10.1021/sb300031n
Schuerle, S., Soleimany, A. P., Yeh, T., Anand, G. M., Häberli, M., Fleming, H. E., et al. (2019). Synthetic and living micropropellers for convection-enhanced nanoparticle transport. Sci. Adv. 5:eaav4803. doi: 10.1126/sciadv.aav4803
Schuster, S., Kreft, J.-U., Brenner, N., Wessely, F., Theißen, G., Ruppin, E., et al. (2010). Cooperation and cheating in microbial exoenzyme production – Theoretical analysis for biotechnological applications. Biotechnol. J. 5, 751–758. doi: 10.1002/biot.200900303
Scott, J. G., Fletcher, A. G., Anderson, A. R. A., and Maini, P. K. (2016). Spatial metrics of tumour vascular organisation predict radiation efficacy in a computational model. PLoS Comput. Biol. 12:e1004712. doi: 10.1371/journal.pcbi.1004712
Scott, J. G., Maini, P. K., Anderson, A. R., and Fletcher, A. G. (2019). Inferring tumor proliferative organization from phylogenetic tree measures in a computational model. Syst. Biol. syz070. doi: 10.1093/sysbio/syz070
Shou, W., Ram, S., and Vilar, J. M. G. (2007). Synthetic cooperation in engineered yeast populations. Proc. Natl. Acad. Sci. U.S.A. 104:1877. doi: 10.1073/pnas.0610575104
Shreiner, A. B., Kao, J. Y., and Young, V. B. (2015). The gut microbiome in health and in disease. Curr. Opin. Gastroenterol. 31, 69–75.
Solé, R. V., Montañez, R., and Duran-Nebreda, S. (2015). Synthetic circuit designs for earth terraformation. Biol. Direct. 10:37. doi: 10.1186/s13062-015-0064-7
Stillman, N. R., Kovacevic, M., Balaz, I., and Hauert, S. (2020). In silico modelling of cancer nanomedicine, across scales and transport barriers. Npj Comput. Mater. (in press).
Stopka, A., Kokic, M., and Iber, D. (2019). Cell-based simulations of biased epithelial lung growth. Phys. Biol. 17:016006. doi: 10.1088/1478-3975/ab5613
Tang, T.-Y. D., Cecchi, D., Fracasso, G., Accardi, D., Coutable-Pennarun, A., Mansy, S. S., et al. (2018). Gene-mediated chemical communication in synthetic protocell communities. ACS Synth. Biol. 7, 339–346. doi: 10.1021/acssynbio.7b00306
Toettcher, J. E., Gong, D., Lim, W. A., and Weiner, O. D. (2011). Light-based feedback for controlling intracellular signaling dynamics. Nat. Methods 8, 837–839. doi: 10.1038/nmeth.1700
Treloar, N. J., Fedorec, A. J. H., Ingalls, B., and Barnes, C. P. (2020). Deep reinforcement learning for the control of microbial co-cultures in bioreactors. PLoS Comput. Biol. 16:e1007783. doi: 10.1371/journal.pcbi.1007783
Velazquez, J. J., Su, E., Cahan, P., and Ebrahimkhani, M. R. (2018). Programming morphogenesis through systems and synthetic biology. Trends Biotechnol. 36, 415–429. doi: 10.1016/j.tibtech.2017.11.003
Waclaw, B., Bozic, I., Pittman, M. E., Hruban, R. H., Vogelstein, B., and Nowak, M. A. (2015). A spatial model predicts that dispersal and cell turnover limit intratumour heterogeneity. Nature 525, 261–264. doi: 10.1038/nature14971
Ward, D., Montes Olivas, S., Fletcher, A., Homer, M., and Marucci, L. (2020). Cross-talk between Hippo and Wnt signalling pathways in intestinal crypts: insights from an agent-based model. Comput. Struct. Biotechnol. J. 18, 230–240. doi: 10.1016/j.csbj.2019.12.015
West, G. B., Woodruff, W. H., and Brown, J. H. (2002). Allometric scaling of metabolic rate from molecules and mitochondria to cells and mammals. Proc. Natl. Acad. Sci. U.S.A. 99:2473. doi: 10.1073/pnas.012579799
Widder, S., Allen, R. J., Pfeiffer, T., Curtis, T. P., Wiuf, C., Sloan, W. T., et al. (2016). Challenges in microbial ecology: building predictive understanding of community function and dynamics. ISME J. 10, 2557–2568. doi: 10.1038/ismej.2016.45
Wu, S., Liu, J., Liu, C., Yang, A., and Qiao, J. (2020). Quorum sensing for population-level control of bacteria and potential therapeutic applications. Cell. Mol. Life Sci. 77, 1319–1343. doi: 10.1007/s00018-019-03326-8
Zadorin, A. S., Rondelez, Y., Gines, G., Dilhas, V., Urtel, G., Zambrano, A., et al. (2017). Synthesis and materialization of a reaction–diffusion French flag pattern. Nat. Chem. 9, 990–996. doi: 10.1038/nchem.2770
Keywords: synthetic biology, multi-agent modeling, systems biology, emergence, multi-scale, bioengineering, consortia, collectives
Citation: Gorochowski TE, Hauert S, Kreft J-U, Marucci L, Stillman NR, Tang T-YD, Bandiera L, Bartoli V, Dixon DOR, Fedorec AJH, Fellermann H, Fletcher AG, Foster T, Giuggioli L, Matyjaszkiewicz A, McCormick S, Montes Olivas S, Naylor J, Rubio Denniss A and Ward D (2020) Toward Engineering Biosystems With Emergent Collective Functions. Front. Bioeng. Biotechnol. 8:705. doi: 10.3389/fbioe.2020.00705
Received: 02 May 2020; Accepted: 05 June 2020;
Published: 26 June 2020.
Edited by:
Pablo Ivan Nikel, Novo Nordisk Foundation Center for Biosustainability (DTU Biosustain), DenmarkReviewed by:
Dae-Hee Lee, Korea Research Institute of Bioscience and Biotechnology (KRIBB), South KoreaIrene Otero Muras, Consejo Superior de Investigaciones Científicas (CSIC), Spain
Copyright © 2020 Gorochowski, Hauert, Kreft, Marucci, Stillman, Tang, Bandiera, Bartoli, Dixon, Fedorec, Fellermann, Fletcher, Foster, Giuggioli, Matyjaszkiewicz, McCormick, Montes Olivas, Naylor, Rubio Denniss and Ward. This is an open-access article distributed under the terms of the Creative Commons Attribution License (CC BY). The use, distribution or reproduction in other forums is permitted, provided the original author(s) and the copyright owner(s) are credited and that the original publication in this journal is cited, in accordance with accepted academic practice. No use, distribution or reproduction is permitted which does not comply with these terms.
*Correspondence: Thomas E. Gorochowski, thomas.gorochowski@bristol.ac.uk
†These authors have contributed equally to this work