- Department of Bioproducts and Biosystems, Aalto University, Espoo, Finland
Saccharomyces cerevisiae is a common platform for production of therapeutic proteins, but it is not intrinsically suited for the manufacturing of antibodies. Antibodies are naturally produced by plasma cells (PCs) and studies conducted on PC differentiation provide a comprehensive blueprint for the cellular transformations needed to create an antibody factory. In this study we mined transcriptomics data from PC differentiation to improve antibody secretion by S. cerevisiae. Through data exploration, we identified several new target genes. We tested the effects of 14 genetic modifications belonging to different cellular processes on protein production. Four of the tested genes resulted in improved antibody expression. The ER stress sensor IRE1 increased the final titer by 1.8-fold and smaller effects were observed with PSA1, GOT1, and HUT1 increasing antibody titers by 1. 6-, 1. 4-, and 1.4-fold. When testing combinations of these genes, the highest increases were observed when co-expressing IRE1 with PSA1, or IRE1 with PSA1 and HUT1, resulting in 3.8- and 3.1-fold higher antibody titers. In contrast, strains expressing IRE1 alone or in combination with the other genes produced similar or lower levels of recombinantly expressed endogenous yeast acid phosphatase compared to the controls. Using a genetic UPR responsive GFP reporter construct, we show that IRE1 acts through constitutive activation of the unfolded protein response. Moreover, the positive effect of IRE1 expression was transferable to other antibody molecules. We demonstrate how data exploration from an evolutionary distant, but highly specialized cell type can pinpoint new genetic targets and provide a novel concept for rationalized cell engineering.
Introduction
Baker’s yeast Saccharomyces cerevisiae has been successfully converted into cell factories for various biotechnological products. One of the most promising markets for microbial products is pharmaceutical proteins for therapeutic applications. S. cerevisiae is already a major platform for production of biopharmaceuticals, including over 20 different proteins ranging from vaccines and insulin products to growth hormones (Walsh, 2014). However, the market growth among biopharmaceuticals is concentrated on antibody products, which are currently produced in mammalian cell cultures (Walsh, 2014; Sanchez-Garcia et al., 2016). As the demand for antibodies continues to increase, efforts to develop alternative platforms to mammalian cells for manufacturing of antibodies have been extending to microbial hosts.
Saccharomyces cerevisiae is not intrinsically suited for therapeutic glycoprotein production, because the yeasts’ native N-glycan structures are structurally different from the N-glycans present on therapeutic antibodies. Advances in glycoengineering of different yeasts in general and of baker’s yeast in particular have demonstrated that production of antibodies with the desired N-glycan structure is feasible (Li et al., 2006; Jacobs P.P. et al., 2009; Nasab et al., 2013; Laukens et al., 2015; Piirainen et al., 2016). Besides approaches modifying the N-glycosylation pathway in vivo, also enzymatic in vitro transglycosylation of yeast produced Fc-fragments and full-length antibody was demonstrated as a feasible option (Wei et al., 2008; Liu et al., 2018). However, major other cellular obstacles still need to be overcome, in particular the limited capacity of S. cerevisiae to produce and secrete recombinant proteins and foremost the structurally complex full-length antibodies. Despite the fact that during the last two decades numerous studies have pinpointed bottlenecks along the secretory pathway (Idiris et al., 2010), up to now, only few studies addressed the problem of enhancing expression of antibody molecules, in particular of full-length antibodies. Increasing the size of the ER, populating the ER with chaperones or preventing missorting to the vacuoles have been identified as bottlenecks for antibody and antibody fragment expression (Shusta et al., 1998; Hackel et al., 2006; Rakestraw et al., 2009; de Ruijter et al., 2016a, b). Furthermore, designing secretory leaders specifically for antibody expression proved to be a successful strategy (Rakestraw et al., 2009). Metabolomics studies indicated also potential metabolic misbalances in antibody expressing strains (de Ruijter et al., 2017).
Antibodies are effective agents of the humoral immunity that are naturally produced by plasma cells (PCs). It was noted some time ago, that PCs outlay the guide map for genetic engineers to improve antibody production platforms, notably mammalian cells (Dinnis and James, 2005). PCs develop from naïve B cells, which first undergo antibody diversification and affinity maturation (Victora and Nussenzweig, 2012). After the mature B cell clone with the most efficient antibody is selected, it develops into a plasmablast (PB) and begins the plasmacytic phase of differentiation. Although PBs secrete low amounts of antibodies, the major preparations for high efficiency antibody production occur during the plasmacytic phase (Shaffer et al., 2004; Oracki et al., 2010). PCs transform their cellular machinery completely to support antibody production, but not all of the metabolic and cellular changes needed might be obvious per se.
Nature has explicated the cellular design for effective secretion. We have shown previously that adapting selected features from PCs, such as their expanded ER or overexpression of mammalian chaperons successfully increased the antibody secretion capacity of S. cerevisiae cells (de Ruijter et al., 2016b; Koskela et al., 2017). Capitalizing on this natural expression system, we explored transcriptomics data sets from PC differentiation to identify novel candidates to be used as engineering targets to improve full-length antibody secretion in yeast. We selected differentially regulated genes and manipulated the expression levels of their corresponding yeast homologs. The four genes IRE1, GOT1, HUT1, and PSA1 when expressed alone or in combinations were shown to be beneficial for antibody secretion in yeast. Further experiments established that the cell engineering strategy is applicable to the expression of other antibody molecules but not to the expression of the endogenous yeast acid phosphatase (AP).
Materials and Methods
Data Analysis Workflow for Plasma Cell Differentiation Phases
Data sets with GEO accession numbers GSE36975 (Le Gallou et al., 2012) and GSE41208 (Cocco et al., 2012) were downloaded to the R software environment by using the GEOquery-package (Davis and Meltzer, 2007). Both data sets were log2-transformed and GSE36975 was pre-processed with affyPLM-package (Bolstad, 2004) for quantile normalization. Probes without an EntrezID were removed from both data sets. R-package illuminaHumanv4.db (Dunning et al., 2015) was used for mapping probe annotations on the common microarray platform of the data sets. The conducted sample comparisons are depicted in the Supplementary Figure S1. In total four comparisons were made from PB phase (G1, G3, C2, and C1 in Supplementary Figure S1) and two from PC differentiation phase (C3 and C4 in Supplementary Figure S1). Differentially expressed genes were determined by using Student’s t-test and a p-value cutoff of 0.05. The differentially expressed genes were divided as follows: genes that had a mean fold-change >0 between the final and earlier states were considered to be upregulated and if mean fold-change was <0, the gene was considered as down-regulated. In the case of PB-specific comparisons, intersect of gene groups from the different data sets was selected in both subgroups (up- and downregulated).
The selected human EntrezIDs were converted to their S. cerevisiae homolog EntrezIDs when applicable with the idConverter()-function provided by AnnotationDbi (Pages et al., 2016) and enabled by organism-specific annotation packages org.Sc.sgd.db (Carlson, 2016) and hom.Hs.inp.db (Carlson and Pages, 2015). The total number of possible homologs from the microarray analysis was 1,364, covering approximately 25% of the yeast open reading frames. GO-term association analysis of the S. cerevisiae gene identifiers was realized with Cytoscape-software (Shannon et al., 2003) extended with the ClueGO-app (Bindea et al., 2009, 2013). Enrichment of the GO-terms was evaluated with a right-sided hypergeometric test without p-value correction from two clusters, the upregulated and downregulated subgroups.
Cloning of Overexpression Constructs
All recombinant DNA work has been done using Escherichia coli TOP10 (Invitrogen) as the cloning host. All plasmids used in this work are summarized in Table 1. Plasmid pEK032 is based on the integrative plasmid pRS305K (Taxis and Knop, 2006) and contains the S. cerevisiae PHO5 gene encoding yeast AP under control of GAL1 promoter and CYC1 terminator. The coding region of PHO5 was amplified from genomic DNA with oligonucleotides OAF219 and OAF220 (Supplementary Table S1) and inserted into plasmid pRS425-GAL1 (Mumberg et al., 1994). The resulting GAL1 promoter-PHO5 gene-CYC1 terminator fragment was inserted into SmaI BssHII sites of pRS305K.
Plasmids pAX512, pAX529, pAX535, and pAX538 are based on the low-copy number plasmid pRS416-GAL1 and contain URA3 selection marker. All antibody coding sequences were fused to wildtype MATα pre- and propeptide (MATα prepro) and were codon optimized for expression in S. cerevisiae (Geneart, Germany). Coding sequences were expressed under control of GAL1 promoter. Plasmids pAX512 and pAX538 encoding single-chain Fv-Fc (scFv-Fc) fusion and full-length IgG derived from anti-hen egg lysozyme Fab molecule (HyHEL-10) (Smith-Gill et al., 1984) have been described earlier (de Ruijter et al., 2017).
Plasmid pAX535 encoding full-length Herceptin antibody has been generated in two steps. First, MATα prepro-light chain (APZ76731.1) sequence-PGK1-terminator and MATα prepro-heavy chain (APZ76730.1) sequences were inserted into SacI Acc65I sites of plasmids pRS416-GAL1 (Mumberg et al., 1994) generating plasmid pAX519 and pAX521. Then, the expression cassette comprising GAL1 promoter-MATα prepro-heavy chain-CYC1 was inserted into PdiI NdeI sites of pAX519 generating plasmid pAX535.
Plasmid pAX529 encodes a scFv-Fc construct based on the Herceptin antibody. VL linked via three repeats of the sequence GGGS to the VH sequence were fused to the human CH2 and CH3 sequences using a previously described linker region (Powers et al., 2001) and appended to MATα prepro. The codon-optimized sequence was inserted into SpeI XhoI sites of pRS416-GAL1.
The coding regions of IRE1, SEC24, BXI1, and DER1 were amplified from genomic DNA with oligonucleotides as indicated (Supplementary Table S1) and cloned into low-copy number plasmid pRS415-TEF (Mumberg et al., 1995) by using restriction enzymes SpeI and XhoI. For IRE1 expression two modified plasmids were constructed by replacing the original TEF1 promoter with the PDI1 promoter and KAR2 promoter generating plasmids pMP29 and pMP30, respectively. The cloning of original plasmids is described earlier (de Ruijter et al., 2016b).
The coding regions of selected genes (ALG5, ALG7, GOT1, GSH21, HUT1, PSA1, and QRI1) were amplified from yeast genomic DNA by using oligonucleotides as indicated (Supplementary Table S1). The resulting products were subjected to another PCR reaction, where complementary overhangs to the plasmid were created with oligonucleotides 21 and 22. PCR-products were cloned between XbaI and XhoI sites of pRS415-TEF vector (Mumberg et al., 1995) by using exonuclease and ligation-independent cloning (Koskela and Frey, 2015).
For co-expression of multiple genes (GOT1, HUT1, and PSA1) vectors comprising URA3, HIS3, and TRP1 selection markers were generated. In brief, TEF promoter-gene-CYC1 terminator cassettes were inserted into SacI DraIII sites of pRS414 generating plasmids with TRP1 selection markers. For generation of vectors comprising URA3 and HIS3 selection markers, the genes were excised from existing plasmids and inserted into SpeI XhoI sites of plasmids pRS416-GAL1 and pRS413-GAL1, respectively.
The plasmid pMS109 for expression of the intronless active form of HAC1 (HAC1i) and its control plasmid pKK1 are described elsewhere (Valkonen et al., 2003a).
Generation of Yeast Strains
All strains used in this study are derived from YEK18, a previously engineered W303α based strain, comprising an expression cassette for the light and heavy chains of the anti-CD20 antibody fused to the PHO5 signal peptide sequence. Both coding sequences are under control of the GAL1-promoter and the construct was integrated into the his3-11,15 site (de Ruijter et al., 2016b).
Plasmids and disruption cassettes were transformed into the yeast cells by using the lithium-acetate method (Gietz et al., 1992). Gene disruptions were selected using YPD-agar medium, supplemented with 200 μg/mL G418 (Sigma-Aldrich, Helsinki, Finland) or 100 μg/mL nourseothricin (Jena Biosciences, Jena, Germany) and verified with colony-PCR. Plasmid containing transformants were selected on synthetic drop-out (SD) agar (0.67% yeast nitrogen base without amino acids) supplemented with a dropout mix lacking leucine, uracil, histidine or tryptophan or the required combinations.
A YEK18 derivative lacking the two proteases PRB1 and PEP4 was generated in two steps. LoxP-kanMX-loxP disruption modules for PRB1 and PEP4 were amplified from pUG6 (Güldener et al., 1996) with PCR using oligonucleotide pairs EK005/EK006 and OJR38/OJR39, respectively (Supplementary Table S1). After the first round of transformation, a Δprb1 deletion strain was obtained and confirmed with colony PCR. The KanMX cassette was removed using Cre-lox recombinase system as described (de Ruijter et al., 2016b). In a second round, deletion of the PEP4 gene was achieved generating the strain YEK66.
For generation of the UPR reporter strain containing an UPRE-GFP reporter, linearized plasmid pDEP17 (Pincus et al., 2010) was integrated into trp1-1 locus of YEK18 generating strain YMH13.
For generation of the anti-CD20 antibody and AP co-expressing strain YMH14, plasmid pEK32 was linearized with DraIII and integrated into leu2-3,112 locus of YEK18.
LoxP-kanMX-loxP disruption modules for ATE1, DPH2, and UTR4 were amplified from pUG6 (Güldener et al., 1996) with PCR using oligonucleotides 1-6 (Supplementary Table S1) and transformed into YEK18 generating strains YEK70, YEK71, and YEK72. All strains are listed in Table 2.
Cultivation Procedures
Strains were inoculated into 2 mL of synthetic drop-out (SD) medium (0.67% yeast nitrogen base without amino acids supplemented with a dropout mix lacking the appropriate amino acids and uracil, with 2% raffinose as the carbon source). The precultures were grown at 30°C, 220 rpm for 16 h. Cells were collected at 3,000 rcf for 5 min and resuspended in H2O to obtain a cell suspension of 5 OD600 per mL. Each well of a 24-deep well plate was filled with 3.84 mL of the expression medium (SD-medium lacking appropriate amino acids and uracil supplemented with 2% raffinose, 20 mM sodium phosphate buffer, pH 6.5 and 50 μg/mL BSA) and was inoculated with 160 μl of cell suspension to yield a starting cell concentration of 0.2 OD600 per mL. The 24-deep well plate was incubated at 30°C, 220 rpm for 6 h and induced with galactose to final concentration of 0.5%. After 16 h of cultivation, OD600 was measured, the culture medium was adjusted to 1× PBT (135 mM NaCl, 2.5 mM KCl, 10 mM Na2HPO4, 1.75 mM KH2PO4, 0.05% Tween-20) and the cultures were centrifuged at 3,000 rcf for 10 min and the cleared supernatant was stored at −20°C until analysis. Remaining medium was discarded and 24-deep well plates containing pelleted cells were stored at −20°C until analysis.
Initial experiments in 96-deep well plate format were done essentially as described above but with 1 mL of culture volume and expression for 24 h.
Samples for UPRE-GFP measurement were centrifuged at 5,000 rcf for 1 min and the cell pellets were resuspended in H2O to reach 5 OD600 per mL. 200 μl of cell suspension were transferred to 96-well plates and fluorescence was measured with an Eon Microplate Spectrophotometer (BioTek, Winooski, VT, United States) using appropriate filter set.
Cultivation of UPR reporter strains for continuous GFP measurements were conducted in 96-well microplates using an Eon Microplate Spectrophotometer (BioTek, Winooski, VT, United States). Precultures were diluted to 0.1 OD600 per mL and 100 μl of cell suspension were transferred to each well. The plate was incubated at 30°C, 220 rpm for 6 h and induced with galactose to final concentration of 0.5%. For repressing conditions 0.5% glucose were added. Growth (OD600) and GFP expression were monitored every 15 min for up to 40 h.
Enzyme-Linked Immunosorbent Assay and Acid Phosphatase Assay
Handling of the cleared supernatants and IgG titer determination with an enzyme-linked immunosorbent assay (ELISA) have been described previously (de Ruijter and Frey, 2015).
Secreted AP activity was measured from cleared supernatants collected prior to adjusting medium to 1× PBT. AP activity was measured using an endpoint method. Ten microliters of culture supernatants were dispensed in triplicates into a 96-well-assay plates. Prior to the assay, the plates were prewarmed for 5 min at 30°C. The assay was started by addition of 100 μL of 20 mM para-nitrophenyl-phosphate (pNPP) (Sigma) in 100 mM sodium acetate buffer, pH 4.2. The reactions were quenched by addition of 200 μL 2M Na2CO3 after 20 min of incubation at 30°C.
SDS-PAGE and Immunoblotting
Pellets for western blotting were prepared as described earlier. Briefly, plates were thawed on ice, cells were resuspended in 1 mL H2O containing 1 mM of PMSF. Ten OD600 units of cells were transferred to Eppendorf tubes and the cells were pelleted at 10,000 rcf for 5 min, +4°C. After this, cells were lysed and prepared for SDS-PAGE analysis as described (de Ruijter and Frey, 2015).
Equal amounts of cell extracts were resolved on SDS-polyacrylamide gels. Separated proteins were transferred onto nitrocellulose membranes at 25 mA and room temperature. Equal transfer of samples was confirmed with Ponceau staining of membranes. All incubation and washing steps were performed in 1% non-fat dry milk in Tris-buffered saline containing 0.1% Tween 20. After transfer, membranes were incubated with anti-human IgG (Fc specific)-peroxidase labeled antibody produced in goat (Sigma) for heavy chain detection, and anti-Human kappa light chains (bound and free)-peroxidase antibody produced in goat (Sigma) for light chain detection. Chemiluminescent detection was performed with Supersignal West Pico Plus detection reagent (Pierce, Rockford, IL, United States).
Results
Cues From B Cell Differentiation for Yeast Cell Engineering
Nature has developed the most sophisticated and efficient antibody expression factory –PCs. PC’s antibody expression and secretion capacity is estimated at 1,000 molecules per second per cell (Hendershot and Sitia, 2004). PCs derive from mature B cells through a complex differentiation process that has been characterized in several genome-wide studies at transcriptome and proteome level (Van Anken et al., 2003; Shaffer et al., 2004; Romijn et al., 2005; Cocco et al., 2012; Le Gallou et al., 2012). We hypothesized that the acquired data sets provide an underexplored repository of engineering targets and provide a guide map to improve antibody expression and secretion. We initiated our approach by conducting a bioinformatics analysis of PC development. We limited our analysis to data sets that included distinct phases of the differentiation process and thus selected two transcriptomics data sets (Cocco et al., 2012; Le Gallou et al., 2012). Although the majority of PCs are short-lived (Tarlinton et al., 2008), we deliberately selected a data set to explore the phenotype of long-lived PCs, as this subpopulation is truly adapted to the secretory burden (Cocco et al., 2012). We divided the differentiation process into two separate phases for the analysis: from mature B-cells to PB phenotype, and from PB to PC (Supplementary Figure S1). Although most of the cellular changes occur in the latter phase, we were interested to investigate whether some underlying changes are already initiated in the PB phase. We determined differentially expressed genes common to both data sets, and roughly divided them to up- and downregulated based on the mean fold-changes. From the differentially expressed genes in the PB phase, we found 45 and 94 yeast homologs corresponding to genes in the up- and downregulated groups, respectively, and in the PC phase we identified 255 and 219 yeast homologs, respectively, from the up- or downregulated genes. The lower numbers in PB phase might be due to strict data integration between the two data sets that we did not employ for the PC phase.
Next, we analyzed the yeast gene candidates with GO-term enrichment and some of the detected biological processes are illustrated in Figure 1. When mature B cells undergo selection and affinity maturation, the cell expressing the needed antibody will start to proliferate (Victora and Nussenzweig, 2012). This largely explains the domination of DNA replication and cell division related processes in the PB phase (Figure 1A). In contrast, the PC phase displayed differential regulation in several antibody expression and secretion related cellular processes illustrating the transformation needed for efficient protein secretion (Figure 1B). In general, upregulation of genes involved in translocation, lipid-linked oligosaccharide biosynthesis and protein N-glycosylation, nucleotide sugar metabolism and vesicle trafficking was prominent (Supplementary Table S2).
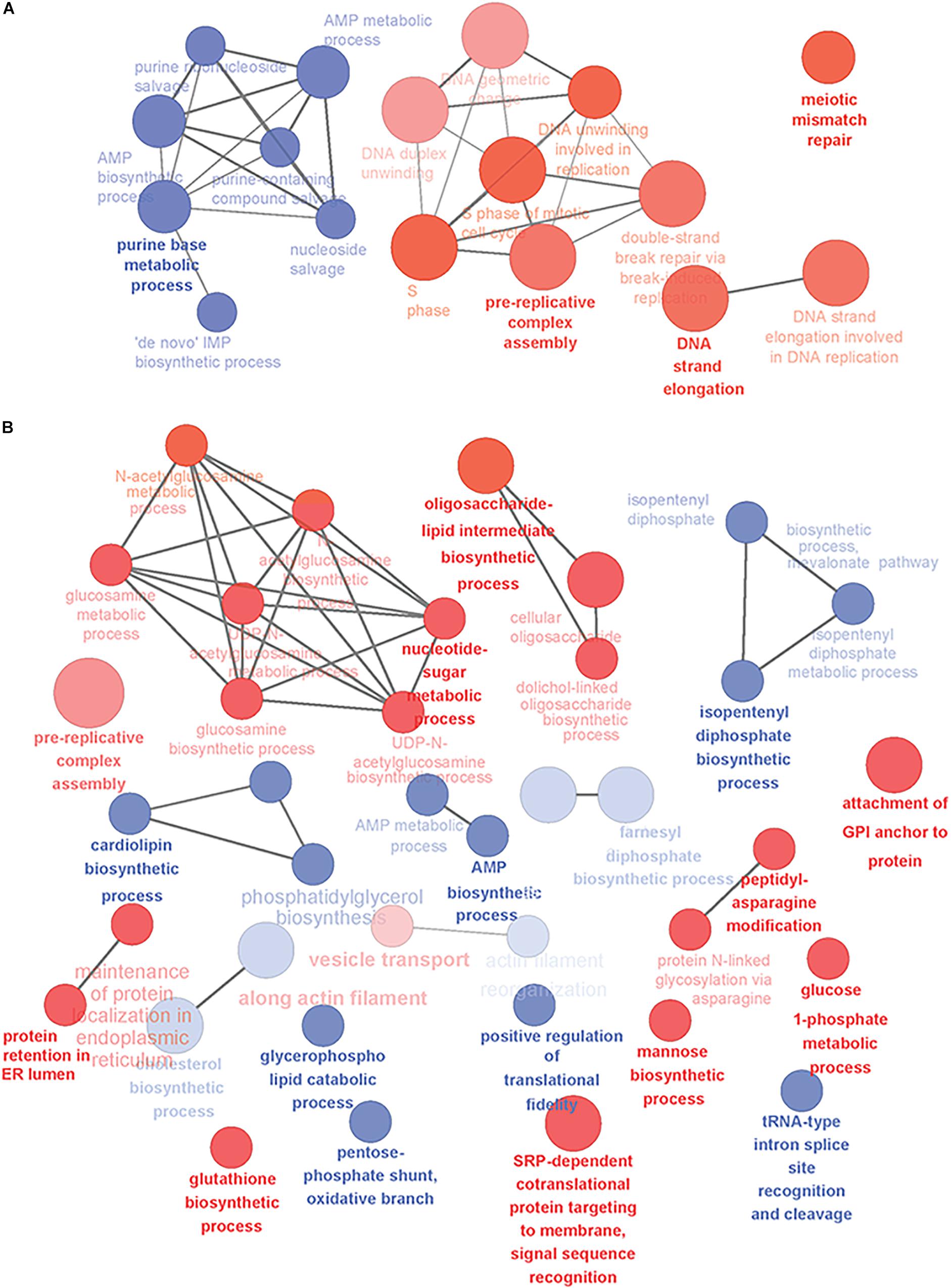
Figure 1. Overview of the yeast-relevant GO-terms displaying enrichment in plasma cell differentiation. Some of the enriched GO-terms of biological processes are visualized in (A) for early phases of plasma cell differentiation until the PB phenotype and (B) for plasmacytic phase of differentiation from PBs into long-lived plasma cells. Up-regulated processes are colored red and down-regulated blue. Size of the node reflects the number of genes identified for that process and the color intensity reflects the statistical significance of the enrichment. The showed networks are mainly descriptive and not directly comparable, as they were created with different settings of GO-levels, annotation requirements and network connectivity. Figure was created with Cytoscape and the ClueGO-app.
Selecting Target Genes for Cell Engineering
To select the gene targets for experimental testing, we explored a detailed list of the enriched biological processes (Supplementary Table S2). In the quest to find potential engineering targets, we went through the gene candidates based on their GO-association. Keeping the risk of affecting viability in mind, we excluded genes associated with central biological processes, such as cell organization and division, basic metabolism, DNA, RNA, and cytoskeleton related terms. We excluded genes that have been reported to decrease fitness considerably (based on Saccharomyces genome database). In contrast to PC-phase, PB-phase comprised almost exclusively of essential processes. However, we decided to test the removal of DPH2, which participates in modifying translation initiation factors. All other candidates were derived from PC-phase.
In total, we selected 14 genetic modifications, where the type of modification, specifically deletion or overexpression, was largely determined by the direction of differential expression of the human homologs in the original analysis (Table 3). The selection of target genes to be overexpressed was biased toward genes with a direct or indirect link to process taking place along the secretory pathway, including genes involved in protein N-glycosylation (ALG5, ALG7, PSA1, and QRI1), transport (SEC24 and GOT1), ER associated protein degradation and ER homeostasis (BXI1, DER1, GSH2, HUT1, and IRE1). The genes IRE1, SEC24, and BXI1 had been identified in a preliminary version of the data analysis workflow of the same data sets. As targets for deletion we selected three genes, ATE1, DPH2, and UTR4, the function of these three genes has so far not been connected to recombinant protein production.
Initial Screening of the Candidate Genes
After defining the modifications, we analyzed the effects of their expression on antibody secretion in an IgG-expressing yeast strain, which had the expression cassette for an anti-CD20 antibody under the control of the GAL1-promoter integrated into its genome. For overexpression of genes, we amplified the coding region from genomic DNA and cloned them into an episomal vector under control of the TEF-promoter. Knock-out strains were created by deleting the complete open reading frames from the genome. After initial experiments in 96-deep well plates, all experiments were conducted in 24-deep well plate format. Antibody titers in culture supernatants were measured 16 h after induction. Four out of the eleven overexpressed genes had a positive effect on antibody secretion. The highest effect was obtained by expression of the UPR sensor IRE1 increasing the final titer by 1.8-fold reaching 178.79 ± 10.88 μg/L, smaller positive effects were observed with PSA1, GOT1, and HUT1 increasing antibody titers by 1. 6-, 1. 4-, and 1.4-fold, respectively, and reaching 157.40 ± 14.75 μg/L, 137.10 ± 13.18 μg/L, and 134.90 ± 9.50 μg/L, respectively (Figure 2A and Supplementary Table S3). In order to differentiate between an improved expression of the IgG and improved secretion, we compared the intracellular levels of heavy and light chains using immunoblotting. The data indicated that although variations in the amounts of intracellular heavy chains are visible, there is no indication that substantial amounts of IgG accumulated within the cells (Figure 2C).
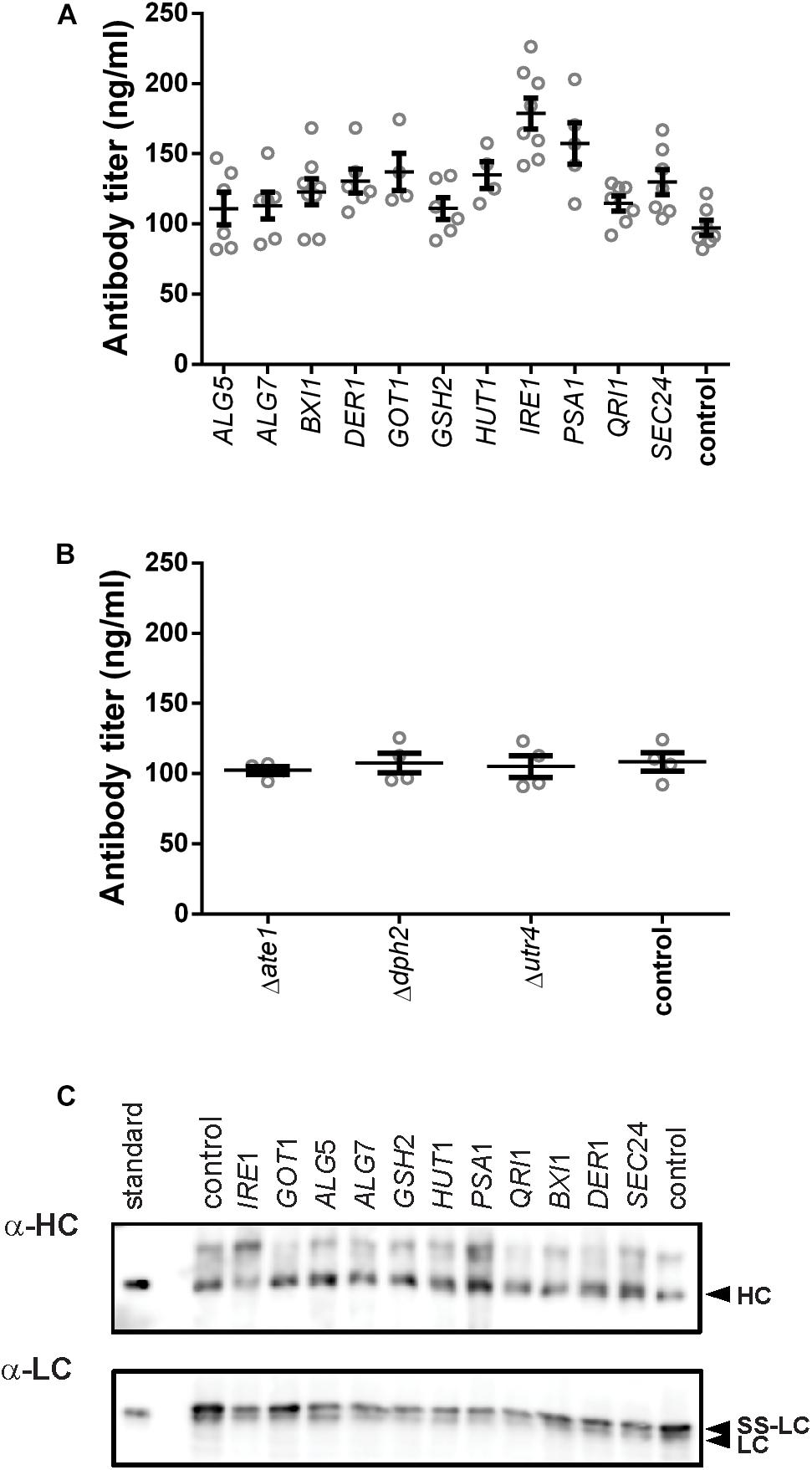
Figure 2. Effects of the tested genes on IgG secretion and expression. Mean antibody titers are displayed for each genetic modification for overexpression (A) and deletion strains (B). Overexpressed or deleted genes are indicated below the charts. Overexpression and corresponding control strains were derived from YEK66. Strains were grown in SD medium lacking leucine. Deletion strains are derived from YEK18 and were grown in complete SD medium. Data points of biological replicates together with mean and standard error are shown. N = 4–8. (C) Immunoblot analysis of cell extracts after 16 h of expression.
Although deletion of DPH2 improved titers in the initial 96-well screening format (Supplementary Figure S2), antibody secretion in the 24-well format did not result in any difference compared to the control strain (Figure 2B). None of the other two deletions resulted in any changes in the antibody expression or growth. Therefore, the three deletions strains were excluded from further experiments.
Synergistic Effects of Gene Overexpression on Antibody Secretion
Next, we created YEK66 strains for pairwise expression of IRE1, GOT1, HUT1, and PSA1 and tested their effects on antibody expression. Of the six strains, the strains co-expressing IRE1 together with either GOT1, HUT1, or PSA1 strongly increased antibody titers but also resulted in reduced final cell densities (Figures 3A,B and Supplementary Table S3). The highest titers were obtained in the strain co-expressing IRE1 and PSA1 reaching a mean titer of 137.68 ± 13.19 μg/L being 3.77-fold higher than the corresponding control (36.56 ± 1.63 μg/L). The two other pairs including IRE1 expression construct, reached final titers of 121.3 ± 13.80 μg/L (IRE1 and HUT1) and 107.08 ± 5.72 μg/L (IRE1 and GOT1) being 3.2- and 2.9-fold higher than the corresponding control strain. The other tested pairs resulted in smaller increases in final antibody titers ranging between 1.3 and 1.5-fold, but also in less than 20% reduction in final OD600 compared to the control (Figures 3A,B and Supplementary Table S3).
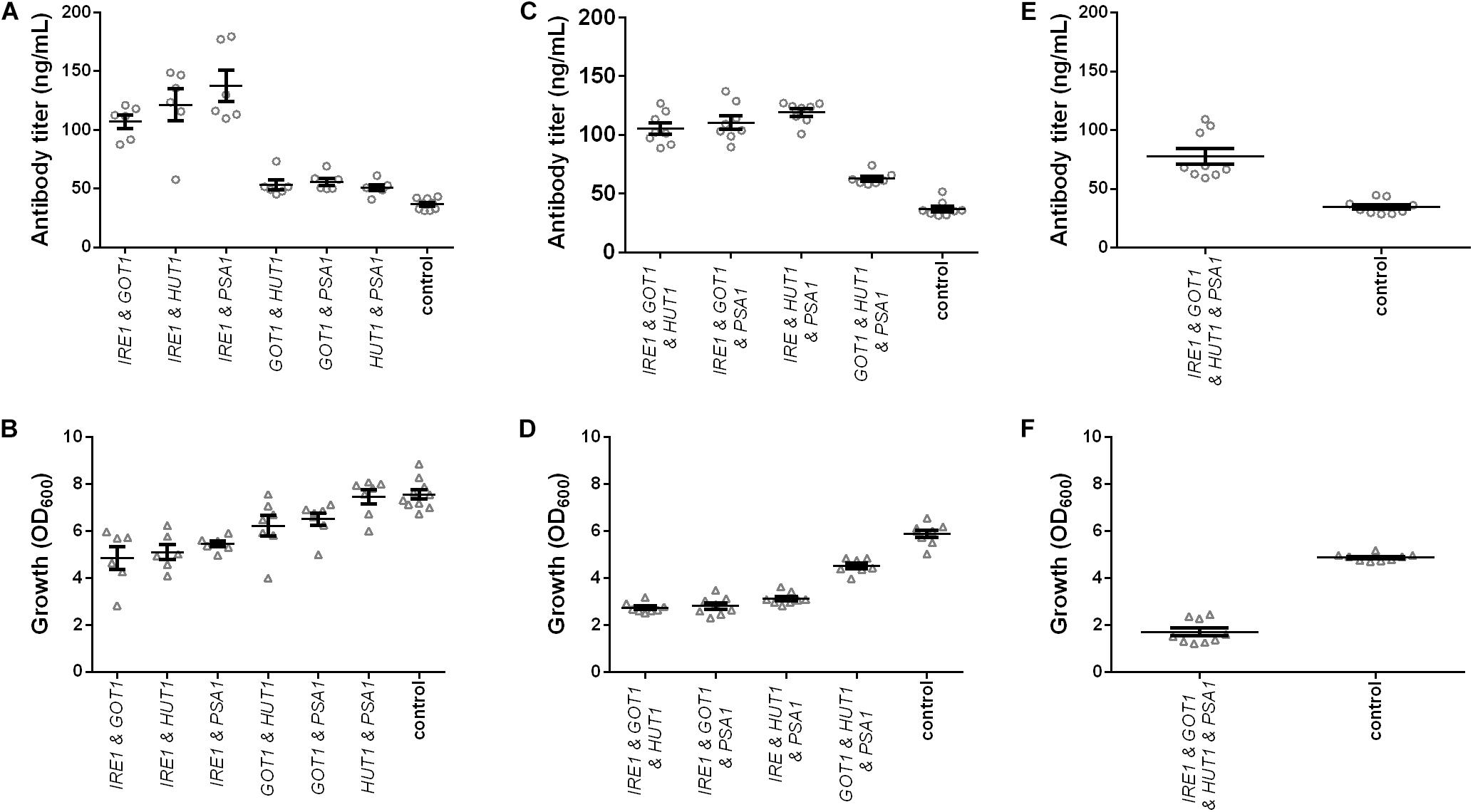
Figure 3. Effects of combined expression of IRE1, GOT1, HUT1, and PSA1 genes on IgG secretion and growth. Mean antibody titers and final cell density are displayed for each genetic modification for strains expressing combinations of two (A,B), three (C,D), and four genes (E,F). Overexpressed genes are indicated below the charts. Overexpression and corresponding control strains were derived from YEK66 and contain two, three, or four low copy number plasmids. Control strains contain corresponding number of empty plasmids. Strains were grown in SD medium lacking the appropriate amino acids (leucine, histidine, and tryptophan) and uracil. Data points of biological replicates together with mean and standard error are shown. N = 6–9.
In order to further improve antibody titers, co-expression of three and four genes at a time was tested. Despite the negative effects on growth, co-expression of three genes led to approximately threefold higher final antibody titers for strains co-expressing IRE1 together with either GOT1 and HUT1, GOT1 and PSA1, or HUT1 and PSA1 reaching final titers of 105.10 ± 4.80 μg/L, 110.50 ± 5.56 μg/L, 119.20 ± 3.19 μg/L (Figure 3C and Supplementary Table S3). However, also co-expression of GOT1, HUT1, and PSA1 resulted in a 1.6-fold increase in antibody concentration reaching 62.68 ± 2.13 μg/L. In the final experiments, all four genes were co-expressed. Antibody titer was 2.2-fold higher compared to the control strain and reached 77.61 ± 6.65 μg/L. However, growth was severely reduced reaching only 35% of the cell density of the corresponding control (Figures 3E,F and Supplementary Table S3).
On a per cell base, antibody productivity increased 6.5-fold for the strains co-expressing all four genes, 6.4-fold for the strain co-expressing IRE1, GOT1, and PSA1 and 5.8-fold for the strain co-expressing IRE1 and HUT1 compared to the corresponding control strains (Supplementary Figure S3).
In order to eliminate the variation in plasmid construct number and assess the contributions of each gene on production and growth a final set of strains was generated, which all contained four plasmids. Expression strains were complemented with the missing empty plasmids. Overall, we generated four strains expressing single genes, six strains expressing pairs, four strains expressing all possible combinations of three genes and one strain expressing all four genes and a control strain harboring four empty plasmids. All strains were grown under identical conditions and final cell density and antibody titers were determined. The measured antibody concentrations followed a similar pattern as observed in the earlier results, although the absolute titers were reduced (Figure 4A and Supplementary Table S3). The three best strains expressed IRE1 alone, IRE1 and HUT1 and IRE1 and PSA1 and reached antibody titers of 88.03 ± 2.85 μg/L, 103.00 ± 2.63 μg/L, 103.10 ± 7.55 μg/L, respectively, compared to the control strain. As expected, the presence of the four plasmids lowered final OD600. Expression of single genes had only minor negative effects on growth compared to the control. With the exception of one strain (IRE1 and PSA1), expression of IRE1 led to a noticeable growth phenotype. In contrast, for strains expressing gene combinations not including IRE1, the negative effects on growth were smaller (Figure 4B and Supplementary Table S3).
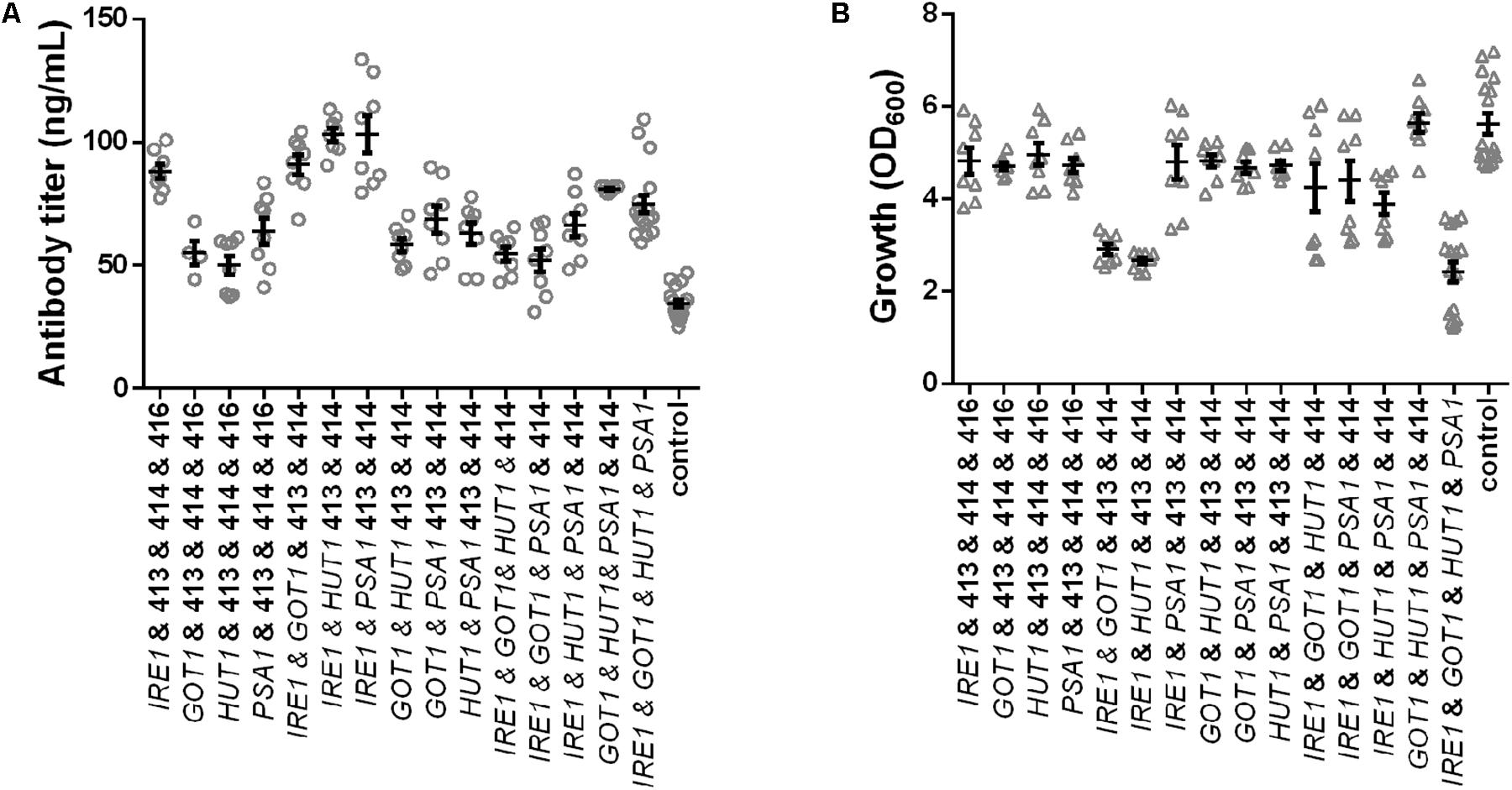
Figure 4. Effects of the selected genes on IgG secretion and growth. Mean antibody titers (A) and final cell density (B) are displayed for each strain expressing one or selected combinations of two, three, and four genes. Overexpressed genes are indicated below the charts. Overexpression and corresponding control strains were derived from YEK66 and all strains contain four low copy number plasmids. Empty plasmids are derived from pRS plasmid series and are labeled 413, 414, 415, and 416. Strains were grown in SD medium lacking leucine, histidine, tryptophan, and uracil. Data points of biological replicates together with mean and standard error are shown. N = 8–12.
Therefore, in order to test whether the expression of IRE1 can be optimized two additional constructs were generated where the TEF promoter was replaced with the KAR2 and PDI1 promoter, respectively. We previously showed that these promoters are less strong and were suitable for expression of molecular chaperones (de Ruijter et al., 2016b). When IRE1 was expressed alone under control of the three different promoters, the antibody titers and growth were very similar (Supplementary Figure S4). When combined with GOT1, HUT1, and PSA1 expression plasmids, antibody titers were similar in all three strains expressing IRE1, irrespective of the promoter controlling expression (Supplementary Figure S4). However, growth of the three strains varied, obtaining the lowest final OD600 with expression of IRE1 driven by PDI1 promoter, but none of the constructs alleviated the growth phenotype obtained with the initial construct.
Transferability of Effects to Other Antibodies
In order to test whether the positive effects were restricted to the anti-CD20 antibody expressed in the screening strains, we evaluated whether the positive effects are more general. Therefore, we included four additional antibody constructs, two full-length antibodies (anti-hen egg lysozyme and Herceptin) and two single-chain-Fc constructs. In contrast to the anti-CD20 antibody, Matα signal sequence is utilized to direct these molecules to the secretory pathway. As IRE1 expression was identified as the main contributor to the positive effects, we limited the experiments to co-expressing IRE1 together with the antibody constructs in the wild-type strain W303α applying our standard 24-well plate cultivation protocol. The control strains contained the corresponding empty plasmid. The expression of all four antibodies was improved by co-expression of IRE1, although the magnitude of the effect varied. The relative improvement was higher for the full-length antibodies than for the less complex scFv-Fc’s (Figure 5 and Supplementary Table S3). IRE1 expression led to 1.3- and 1.4-fold higher expression of scFv-Fc’s and to 4.5- and 3.7-fold higher expression of full-length Herceptin antibody and full-length HyHEL antibody reaching a final titers of 75.44 ± 0.68 μg/L and 89.38 ± 1.60 μg/L, respectively, confirming that the effects are transferable to other antibody molecules.
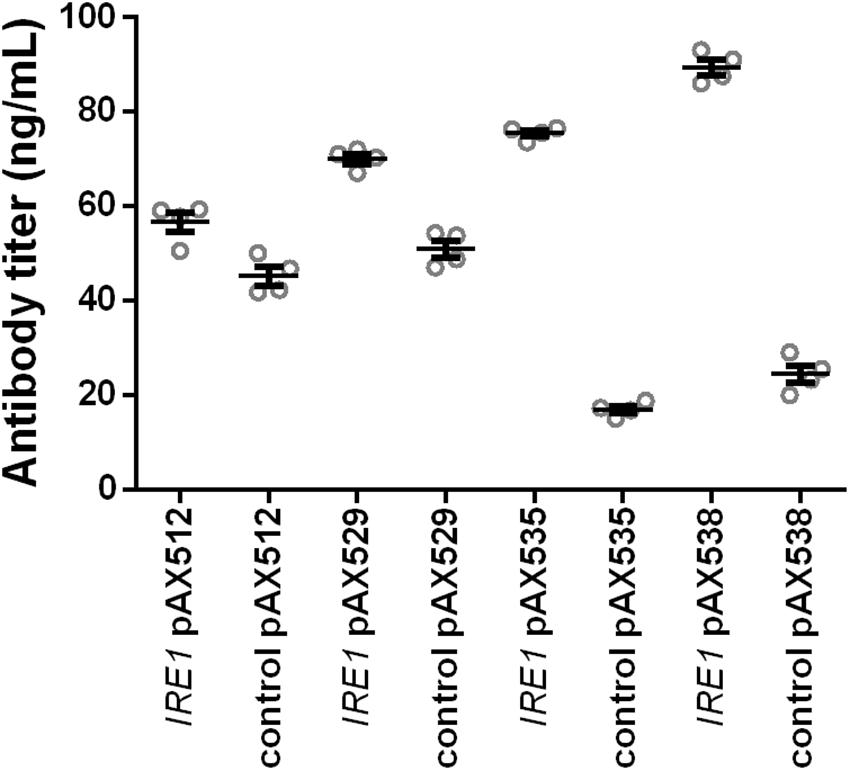
Figure 5. Expression of IRE1 increases secretion of various IgGs. Two full-length and two scFv-Fc antibodies were co-expressed with IRE1 or a control plasmid. Mean antibody titers are displayed for each strain expressing scFv-Fc antibodies (pAX512 and pAX529) or full-length antibodies (pAX535 and pAX538). Overexpression and corresponding control strains were derived from W303α and all strains contain two low copy number plasmids. Antibody expression is under control of PGAL1. Strains were grown in SD medium lacking leucine and uracil. Data points of biological replicates together with mean and standard error are shown. N = 4.
Expression of the Endogenous Protein Acid Phosphatase Is Not Improved by the Cell Engineering Approach
Next we wondered whether the positive effects are specific for recombinant antibodies or also observable for the expression of other proteins. In order to test this we generated a yeast strain harboring the expression cassette for the anti-CD20 antibody under control of GAL1 promoter in the HIS3 locus and an additional expression cassette for the endogenous AP expressing PHO5 gene under control of GAL1 promoter in the LEU2 locus. Preliminary tests with this strain showed that the AP activity increased with increasing galactose concentration from 0 to 4%. In contrast, IgG secretion was limited and the titers reached a plateau above an induction level of 1% galactose (Supplementary Figure S5). Thus, while secretion capacity of antibodies is clearly limited, the secretion of the endogenous AP activity is not limited under the tested conditions.
In the experiments we tested expression of IRE1, co-expression of IRE1 with GOT1, HUT1, or PSA1 and co-expression of all four genes and measured secreted antibody titers and AP activity. Antibody secretion and growth were influenced in similar ways as observed earlier, yielding increased titers, but lower final OD600 for the engineered strains (Figure 6). Furthermore, higher induction levels did not increase antibody titers. In contrast to antibody concentrations, the secreted AP activity was highest in the control strain and reduced to different levels in the other strains. Moreover, AP activity increased when using the higher induction levels. Notably, the measured AP activities correlated well with the final OD600 (Figures 6B,C). Overall, the results imply that the cell engineering approach is highly specific for increasing antibody expression.
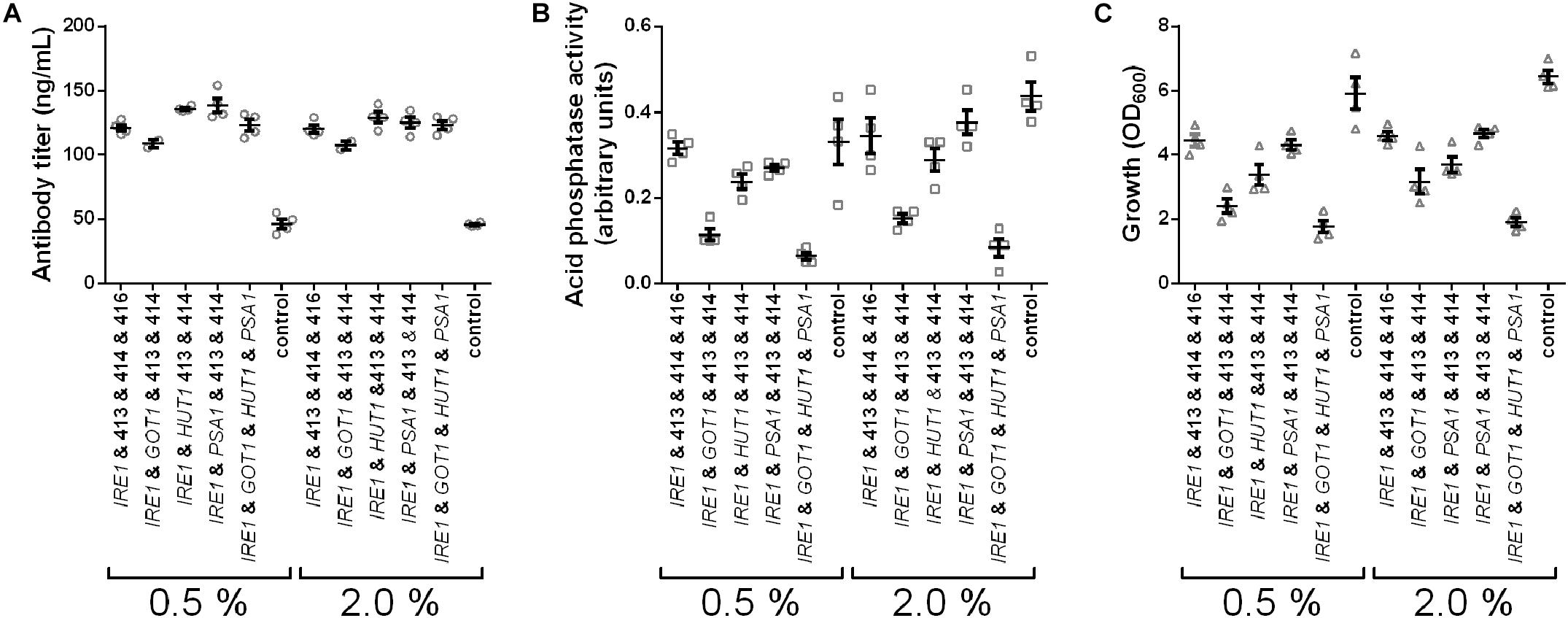
Figure 6. IRE1, GOT1, HUT1, and PSA1 enhance secretion of IgG but not of overexpressed endogenous AP. Antibody titers (A), secreted AP activity (B), and final cell density (C) are displayed for each strain expressing one or selected combinations of two, or all four genes. Overexpressed genes are indicated below the charts. All strains were derived from YMH14 and contain four low copy number plasmids. Expression of antibody and AP are under control of PGAL1. Expression strains were grown in SD medium lacking leucine, histidine, tryptophan, and uracil. Protein expression was induced with 0.5 and 2.0% galactose. Data points of biological replicates together with mean and standard error are shown. N = 4.
Expression of IRE1 Activates the Unfolded Protein Response
Induction of premature or constant UPR has been shown to enhance secretion, but the induction was established with overexpression of HAC1i encoding active spliced Hac1p, the protein downstream of Ire1p (Sidrauski and Walter, 1997; Valkonen et al., 2003a). We set out to test whether our novel gene identified through the data exploration would have similar effects on IgG secretion as this known approach. Two strains together with their corresponding control strains were generated and effects of Hac1p and Ire1p on antibody secretion and growth were analyzed. Our data showed that expression of both the sensor kinase as well as the active transcription factor increased antibody secretion with only a small effect on growth (Figure 7 and Supplementary Table S3). Antibody concentrations increased 1.6- and 1.8-fold, whereas final OD600 reached 83 and 93% of the corresponding control strains for HAC1i and IRE1 expression strains, respectively (Figures 7A,B). Next we investigated UPR induction in the antibody expressing strain using a previously published UPR responsive GFP reporter construct (UPRE-GFP) (Pincus et al., 2010). HAC1i expression leads to constitutive UPR induction and thus served as a reference. We compared the HAC1i and IRE1 expression strains in two different settings, in repressive glucose medium inhibiting antibody expression and under inducing conditions in microtiter plate cultivations. As expected HAC1 expression led to activation of UPR under both repressive and inducing conditions. A similar response was observed for IRE1 expressing strain, indicating that overexpression of UPR sensor leads to activation of the stress response irrespective of the antibody expression. The control strains expressing antibody alone showed detectable responses only when grown on galactose (Supplementary Figure S6). Next we compared UPR induction in our standard expression set-up. Based on the preliminary data, we selected five time points for measuring UPR activation. Samples were measured at time points 0, 2.5, 5, 8, and 16 h after induction. Overexpression of UPR signaling proteins induced measurable UPR. UPR induction was higher in both strains expressing the UPR sensing and signaling molecules (Figure 7).
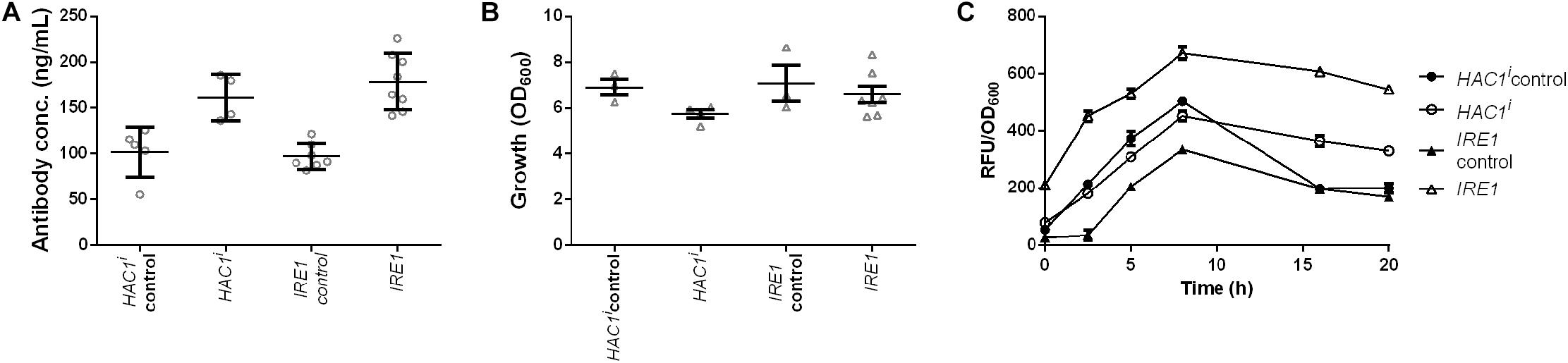
Figure 7. Expression of UPR sensor and UPR specific transcription factor Hac1p enhances antibody production. Mean antibody titers (A), final cell density (B), and UPR activation (C) are displayed for strains constitutively expressing active spliced HAC1i and IRE1. For each expression construct the corresponding control plasmid was included. All strains were derived from YEK66. Strains were grown in SD medium lacking leucine. Data points of biological replicates together with mean and standard error are shown. N = 4–8.
Discussion
In this study we demonstrated the potential of using PC differentiation as a blueprint to guide cell engineering. Through data exploration, we identified 300 and 315 yeast homologs in the group of up- and downregulated genes, respectively. From these genes, we selected 14 for experimental testing. Out of the 14 target genes, the manipulation of four genes resulted in positive effects on antibody expression. All four genes were selected from the group of upregulated genes during PC differentiation and were therefore overexpressed in our experiments.
Especially the analysis of the PC-phase unearthed several genes that have been successfully tested in engineering recombinant protein production. For example, the ER chaperone BiP (yeast KAR2) and the nucleotide exchange factor SIL1 (yeast SIL1) were in the upregulated group associated to several of the identified biological processes (Supplementary Table S2). Both functionalities, human BiP or KAR2 and yeast SIL1 have been overexpressed in antibody producing S. cerevisiae strains (Shusta et al., 1998; Hackel et al., 2006; de Ruijter et al., 2016b). Moreover, the homologs of the vacuolar sorting proteins Vps41p and Vps45p appeared in the category of downregulated genes, corresponding to previous reports where deletion of several of the VPS genes has been found beneficial for recombinant protein secretion (Zhang et al., 2001; Rakestraw et al., 2009; de Ruijter et al., 2016a).
The strongest effect was obtained by expression of the UPR sensor, the transmembrane protein Ire1p. The positive effect of IRE1 expression was confirmed by co-expression with four different antibody molecules showing that its positive effect was not restricted to enhancing secretion of the anti-CD20 antibody in the screening strain but was applicable to other antibody molecules and formats (Figure 5). The effects were notably higher with the structurally more complex tetrameric full-length IgGs than the dimeric scFv-Fc constructs. Moreover, the effects of IRE1 on antibody secretion was independent of the signal sequence used, the 19 amino acids long PHO5 derived signal peptide in the case of anti-CD20 antibody and the Matα signal sequence for the other four antibody molecules. It is interesting to note in this context that expression of different antibody formats led to distinctive reshaping of intracellular metabolome (de Ruijter et al., 2017). Furthermore, relative rate of secretion decreased with increasing complexity of the protein in Pichia pastoris (Love et al., 2012). Furthermore, it was reported that IRE1 expression led to a higher intracellular level of a Hepatitis B small antigen GFP fusion in yeast (Sheng et al., 2017). In contrast, co-expression of the endogenous AP did not benefit from IRE1 expression under the tested conditions (Figure 6). Ire1p senses and signals ER stress by splicing the HAC1 transcript into its active form. Activation of Ire1p is a two-stage process in which first Kar2p is released followed by binding of unfolded proteins inducing oligomerization of Ire1p (Kimata et al., 2007). Experiments using a UPRE-GFP reporter showed that IRE1 expression led to a higher basal GFP level under repressing conditions, indicating that UPR is preactivated. It is plausible that the overexpression of IRE1 shifts the ratio between Kar2p and Ire1p, therefore making Ire1p more susceptible to ER stress. Thus, our finding is in contrast to earlier results, where the overexpression of IRE1 from a high-copy number plasmid did not result in UPR activation as shown through analyzing HAC1 splicing (Kimata et al., 2007).
Genetic induction of UPR by overexpression of the spliced transcript of HAC1, HAC1i, has been shown to enhance protein secretion in yeasts and fungi (Valkonen et al., 2003a, b; Gasser et al., 2007; Payne et al., 2008). Therefore, we have also compared these two approaches to induce UPR. Our data indicate that expression of IRE1 is slightly more successful in increasing antibody secretion relative to HAC1i expression. In contrast to expression of active Hac1p, cells overexpressing Ire1p can putatively still sense physiological ER stress, which can be important in order to tune the UPR according to cellular needs. Although replicating the events taking place in PCs, induction of UPR via expression of either IRE1 or HAC1i resembles more a shotgun approach than a designed genetic modification.
The essential protein Psa1p catalyzes the last step of the GDP-mannose biosynthesis. GDP-mannose is used as substrate in the cytoplasmic steps of the LLO biosynthesis, in all mannosylation steps in the Golgi apparatus and serves as a donor for dolichol-phosphate mannose synthesis. Expression of the PSA1 gene in S. cerevisiae was shown earlier to complement defects in the LLO biosynthesis rescuing temperature sensitive mutations of alg1, alg2, and dpm1 genes and restoring protein N-glycosylation (Janik et al., 2003). As the expressed antibody is not a heavily glycosylated protein, having only one glycosylation site in each heavy chain, it is likely that observed improvements are due to indirect effects on cellular glycosylation status and viability. Interestingly, expression of Kluyveromyces lactis homolog KlPsa1 enhanced secretion of the non-glycosylated protein human serum albumin and of the N-glycosylated Arxula adeninivorans glucoamylase in K. lactis (Uccelletti et al., 2005). Opposite effects on protein secretion were reported when studying a GDP-mannose phosphorylase in Hansenula polymorpha where inactivation of the gene led to an increased secretion of two proteins (Agaphonov et al., 2001). Taking together the previous and our current results, the molecular bases underlying the observed increased protein secretion are not yet clear. Moreover, the other genes with links to protein glycosylation, ALG5, ALG7, and QRI1 did not result in any effects.
GOT1 overexpression led to a moderate increase in antibody expression. The function of Got1p in the COPII-coated vesicles is less defined (Conchon et al., 1999; Malsam et al., 2008; Lorente-Rodríguez et al., 2009). Conchon et al. (1999) suggested that Got1p contributes to vesicle fusion on the cis-Golgi membrane, but Lorente-Rodríguez et al. (2009) showed that most of Got1p localizes to the ER. Strong overexpression of Got1p induced elaboration of ER membranes, which Lorente-Rodríguez et al. (2009) inferred to be caused by a block in secretion. We show here that overexpression of Got1p in fact increased secretion, which implies that Got1p is an active player in vesicle trafficking.
The cellular function of the multi-pass membrane protein Hut1p is the least known of the four proteins. Due to sequence similarity with the human UDP-galactose transporter it had been studied in the context of cellular glycosylation (Kainuma et al., 2001), but other studies on Hut1p from S. cerevisiae and Schizosaccharomyces cerevisiae implied that Hut1p is involved in protein folding in the ER maintaining optimal conditions for folding of secretory pathway proteins (Nakanishi et al., 2001). This hypothesis was corroborated by genetic interaction of HUT1 with ERO1 and increased sensitivity to DTT of a Δhut1 deletion strain.
When combining the expression of multiple genes, the highest increases were observed combining IRE1 with PSA1 or IRE1 with PSA1 and HUT1, reaching 3.8- and 3.1-fold higher antibody titers compared to the corresponding control strains. We also tested whether the expression of IRE1 together with ER folding factors such as KAR2, PDI1, and CPR5 would result in synergistic effect, although a small additional increase was overserved for coexpression of CPR5, the effect was of a smaller magnitude than one obtained with coexpression of PSA1 (data not shown). When all four genes were co-expressed final titers decreased again, implying a strong drain on the cellular resource pool. In particular, the co-expression with IRE1 had strong negative effects on final cell densities (Figures 3, 4), which might result from the additional activation of UPR. Also expressing IRE1 under control of weaker promotors did not alleviate the costs of IRE1 coexpression on strain fitness. However despite a reduced fitness, the productivity per amount of cells increased (Supplementary Figure S3). Moreover, a negative correlation between final OD600 and increasing plasmid number was observed. Growth of the control strains containing one, two, three, or four plasmids resulted in average final OD600 of 6.9, 7.55, 5.74, and 4.89, respectively. In the most severely affected strains expressing the genes, growth reached 90% (one plasmid), 64% (two plasmids), 47% (three plasmids), and 35% (four plasmids) of the final OD600 obtained of the corresponding control strains indicating that there is a cumulative effect resulting from the burden of carrying extra plasmids and from expression of the overexpressed proteins.
Interestingly, expression of AP followed a different pattern than expression of antibody. Although we used the same genetic constructs for expression, a single copy of the gene was integrated into the genome at either LEU2 or HIS3 locus, expression was controlled by the GAL1 promoter and the identical signal peptide was used for translocation, the effects of IRE1, PSA1, GOT1, or HUT1 on the secretion of either the antibody or the AP were very different. As the secreted AP activity, in contrast to the secreted anti-CD20 antibody amounts, increased with increasing induced concentration, we believe that in our set-up the expression of AP is mostly limited at transcriptional level whereas anti-CD20 antibody secretion is limited at the post-translational level (Supplementary Figure S5) and thus expression of IRE1, PSA1, GOT1, or HUT1 which are all involved in biological processes taking place post-translationally only improve secretion of the antibody.
Conclusion
In conclusion, by mining transcriptomics data sets from B cell differentiation we identified four new modifications, which enhanced antibody secretion in yeast. Thus, such data exploration is effective in finding novel engineering targets, even when employed to an evolutionary distant organism. So far, comparable experimental approaches had been limited to analyzing the expression host’s own transcriptome and selective overexpression of up-regulated genes (Gasser et al., 2007; Jacobs D.I. et al., 2009). In order to raise the antibody titers to competitive levels further improvements are required. In particular, it will be crucial to identify more engineering targets producing synergistic effects and to use advanced secretion optimized strains as a basis for strain engineering (Wang et al., 2019). Furthermore, it will be essential to generate antibodies with proper N-glycan structures. Unless using post-production in vitro glycosylation (Wei et al., 2008; Liu et al., 2018), it needs to be ensured that glycoengineering strategies are compatible with production optimized strains.
Data Availability Statement
All datasets generated for this study are included in the article/Supplementary Material.
Author Contributions
AF and EK designed the study and wrote the manuscript. EK conducted the bioinformatics analysis, contributed to cloning of the expression constructs and experimental testing. HI contributed to bioinformatics analysis, cloning of the expression constructs and participated in preliminary experiments. AG created deletion strains and participated in cloning of the expression constructs and preliminary experiments. MP contributed to the gene target selection and cloning of expression constructs. HS contributed to the experimental testing. AF contributed to cloning of the expression constructs, created and analyzed the experimental data. All authors have read the manuscript.
Funding
This work was supported by the Aalto University Bioeconomy facilities, Aalto University School of Chemical Engineering and Academy of Finland (grant number 298476). EV Koskela and MA Piirainen are recipients of doctoral study grants from the School of Chemical Engineering, Aalto University.
Conflict of Interest
EK was employed by the company Euretos B.V and HI was employed by Byinfo Oy while the manuscript was finalized.
The remaining authors declare that the research was conducted in the absence of any commercial or financial relationships that could be construed as a potential conflict of interest.
Acknowledgments
We would like to thank Technical Research Center of Finland for providing the plasmid pMS109. In addition, we acknowledge Sofia Julin, Jaakko Sahlsten, Ara Taalas, Henni Auvinen, Vili Auvinen, and Hele Haapaniemi, who contributed to this study.
Supplementary Material
The Supplementary Material for this article can be found online at: https://www.frontiersin.org/articles/10.3389/fbioe.2020.00255/full#supplementary-material
FIGURE S1 | Selected transcriptomics data sets and comparisons included in the analysis. Gene expression omnibus identifiers for the data sets and microarrays in each sample are shown. Comparisons are shown as arrows and common genes were identified from each of the two phases.
FIGURE S2 | Effects of the tested genes on IgG secretion in 96-deep well plate format. Mean antibody titers are displayed for each genetic modification for overexpression strain (A) and deletion strains (B). Overexpressed or deleted genes are indicated below the charts. Overexpression and corresponding control strains a derived from YEK66. Strains were grown in SD-Leu medium. Deletion strains are derived from YEK18 and were grown in complete SD medium. Data points of biological replicates together with mean and standard error are shown. N = 8, IRE1: N = 16.
FIGURE S3 | Effects of combined expression of IRE1, GOT1, HUT1, and PSA1 genes on biomass normalized IgG titers. Data from Figure 3 depicting IgG titers normalized by final OD600 for strains expressing combinations of two (A), three (B), and four genes (C). Overexpressed genes are indicated below the charts. Data points of biological replicates together with mean and standard error are shown. N = 6–8.
FIGURE S4 | Optimization of IRE1 expression. Mean antibody titers (A,C) and final cell density (B,D) are displayed for three YEK66 derived strains expressing IRE1 gene under control of PTEF1, PPDI1, and PKAR2 (A,B) or IRE1 gene under control of PTEF1, PPDI1, and PKAR2 together with GOT1, HUT1, and PSA1 genes (C,D). Data points of biological replicates together with mean and standard error are shown. N = 6.
FIGURE S5 | Effect of increasing inducer concentrations on secretion of antibody and AP in 96-deep well plate format. Strain YMH14 was grown in in 96-deep well plate format and expression was induced with 0, 0.5, 1.0, 2.0, and 4.0% galactose. Antibody titers (A), secreted AP activity (B) normalized to cell density is reported. Data points of biological replicates together with mean and standard error are shown. N = 14–16.
FIGURE S6 | Unfolded protein response in strains overexpressing UPR signaling proteins. Growth and activation of UPR under repressive (glucose) and inducing (galactose) conditions. Glucose and galactose were added to the cultures 6 h after inoculation. Results represents mean and standard error of three biological replicates. For each biological replicate four parallel cultures were grown.
TABLE S1 | Oligonucleotides used in this study.
TABLE S2 | Analysis of the yeast homologs in PC and PB phase and their GO-associations.
TABLE S3 | Compilation of numeric data including antibodies titers and final cell densities.
Abbreviations
LLO, lipid-linked oligosaccharide; PB, plasmablast; PC, plasma cell; UPR, unfolded protein response.
References
Agaphonov, M. O., Packeiser, A. N., Chechenova, M. B., Choi, E. S., and Ter-Avanesyan, M. D. (2001). Mutation of the homologue of GDP-mannose pyrophosphorylase alters cell wall structure, protein glycosylation and secretion in Hansenula polymorpha. Yeast 18, 391–402. doi: 10.1002/yea.678
Bindea, G., Galon, J., and Mlecnik, B. (2013). CluePedia Cytoscape plugin: pathway insights using integrated experimental and in silico data. Bioinformatics 29, 661–663. doi: 10.1093/bioinformatics/btt019
Bindea, G., Mlecnik, B., Hackl, H., Charoentong, P., Tosolini, M., Kirilovsky, A., et al. (2009). ClueGO: a Cytoscape plug-in to decipher functionally grouped gene ontology and pathway annotation networks. Bioinformatics 25, 1091–1093. doi: 10.1093/bioinformatics/btp101
Bolstad, B. (2004). Low Level Analysis of High-Density Oligonucleotide Array Data: Background, Normalization And Summarization. Berkeley, CA: University of California.
Carlson, M. (2016). org.Sc.sgd.db: Genome Wide Annotation for Yeast. Avaliable at: http://bioconductor.org/packages/org.Sc.sgd.db. Version: 3.2.3. (accessed July 06, 2017).
Carlson, M., and Pages, H. (2015). Hom.Hs.inp.db: Homology Information for Homo Sapiens from Inparanoid, R package version 3.1.2. (accessed July 06, 2017).
Cocco, M., Stephenson, S., Care, M. A., Newton, D., Barnes, N. A., Davison, A., et al. (2012). In vitro generation of long-lived human plasma cells. J. Immunol. 189, 5773–5785. doi: 10.4049/jimmunol.1103720
Conchon, S., Cao, X., Barlowe, C., and Pelham, H. R. B. (1999). Got1p and Sft2p: membrane proteins involved in traffic to the Golgi complex. EMBO J. 18, 3934–3946. doi: 10.1093/emboj/18.14.3934
Davis, S., and Meltzer, P. S. (2007). GEOquery: a bridge between the gene expression omnibus (GEO) and bioconductor. Bioinformatics 14, 1846–1847. doi: 10.1093/bioinformatics/btm254
de Ruijter, J. C., and Frey, A. D. (2015). Analysis of antibody production in Saccharomyces cerevisiae: effects of ER protein quality control disruption. Appl. Microbiol. Biotechnol. 99, 9061–9071. doi: 10.1007/s00253-015-6807-7
de Ruijter, J. C., Jurgens, G., and Frey, A. D. (2016a). Screening for novel genes of Saccharomyces cerevisiae involved in recombinant antibody production. FEMS Yeast Res. 17, 1–10. doi: 10.1093/femsyr/fow104
de Ruijter, J. C., Koskela, E. V., and Frey, A. D. (2016b). Enhancing antibody folding and secretion by tailoring the Saccharomyces cerevisiae endoplasmic reticulum. Microb. Cell Fact. 15, 87. doi: 10.1186/s12934-016-0488-5
de Ruijter, J. C., Koskela, E. V., Nandania, J., Frey, A. D., and Velagapudi, V. (2017). Understanding the metabolic burden of recombinant antibody production in Saccharomyces cerevisiae using a quantitative metabolomics approach. Yeast 35, 1–11. doi: 10.1002/yea.3298
Dinnis, D. M., and James, D. C. (2005). Engineering mammalian cell factories for improved recombinant monoclonal antibody production: lessons from nature? Biotechnol. Bioeng. 91, 180–189. doi: 10.1002/bit.20499
Dunning, M., Lynch, A., and Eldridge, M. (2015). Illuminahumanv4.db: Illumina HumanHT12v4 Annotation data (chip illuminaHumanv4). R Package Version 1.26.0.
Gasser, B., Sauer, M., Maurer, M., Stadlmayr, G., and Mattanovich, D. (2007). Transcriptomics-based identification of novel factors enhancing heterologous protein secretion in yeasts. Appl. Env. Microbiol. 73, 6499–6507. doi: 10.1128/aem.01196-07
Gietz, D., St Jean, A., Woods, R. A., and Schiestl, R. H. (1992). Improved method for high efficiency transformation of intact yeast cells. Nucleic Acid Res. 20:1425. doi: 10.1093/nar/20.6.1425
Güldener, U., Heck, S., Fiedler, T., Beinhauer, J., and Hegemann, J. H. (1996). A new efficient gene disruption cassette for repeated use in budding yeast. Nucleic Acids Res. 24, 2519–2524. doi: 10.1093/nar/24.13.2519
Hackel, B. J., Huang, D., Bubolz, J. C., Wang, X. X., and Shusta, E. V. (2006). Production of soluble and active transferrin receptor-targeting single-chain antibody using Saccharomyces cerevisiae. Pharm. Res. 23, 790–797. doi: 10.1007/s11095-006-9778-7
Hendershot, L. M., and Sitia, R. (2004). “Immunoglobulin assembly and secretion,” in Molecular Biology of B Cells, eds M. Reth, A. Radbruch, F. Alt, T. Honjo, and M. Neuberger (Amsterdam: Elsevier), 261–273. doi: 10.1016/b978-012053641-2/50018-6
Idiris, A., Tohda, H., Kumagai, H., and Takegawa, K. (2010). Engineering of protein secretion in yeast: strategies and impact on protein production. Appl. Microbiol. Biotechnol. 86, 403–417. doi: 10.1007/s00253-010-2447-0
Jacobs, D. I., Olsthoorn, M. M., Maillet, I., Akeroyd, M., Breestraat, S., Donkers, S., et al. (2009). Effective lead selection for improved protein production in Aspergillus niger based on integrated genomics. Fungal. Genet. Biol. 46, (Suppl. 1), S141–S152. doi: 10.1016/j.fgb.2008.08.012
Jacobs, P. P., Geysens, S., Vervecken, W., Contreras, R., and Callewaert, N. (2009). Engineering complex-type N-glycosylation in Pichia pastoris using GlycoSwitch technology. Nat. Protoc. 4, 58–70. doi: 10.1038/nprot.2008.213
Janik, A., Sosnowska, M., Kruszewska, J., Krotkiewski, H., and Lehle, L. (2003). Overexpression of GDP-mannose pyrophosphorylase in Saccharomyces cerevisiae corrects defects in dolichol-linked saccharide formation and protein glycosylation. Biochim. Biophys. Acta 1621, 22–30. doi: 10.1016/s0304-4165(03)00026-6
Kainuma, M., Chiba, Y., Takeuchi, M., and Jigami, Y. (2001). Overexpression of HUT1 gene stimulates in vivo galactosylation by enhancing UDP – galactose transport activity in Saccharomyces cerevisiae. Yeast 18, 533–541. doi: 10.1002/yea.708
Kimata, Y., Ishiwata-Kimata, Y., Ito, T., Hirata, A., Suzuki, T., Oikawa, D., et al. (2007). Two regulatory steps of ER-stress sensor Ire1 involving its cluster formation and interaction with unfolded proteins. J. Cell Biol. 179, 75–86. doi: 10.1083/jcb.200704166
Koskela, E. V., de Ruijter, J. C., and Frey, A. D. (2017). Following nature’s roadmap: folding factors from plasma cells led to improvements in antibody secretion in S. cerevisiae. Biotechnol. J. 12, 1–13.
Koskela, E. V., and Frey, A. D. (2015). Homologous recombinatorial cloning without the creation of single-stranded ends: exonuclease and ligation-independent cloning (ELIC). Mol. Biotechnol. 57, 233–240. doi: 10.1007/s12033-014-9817-2
Laukens, B., De Visscher, C., and Callewaert, N. (2015). Engineering yeast for producing human glycoproteins: where are we now? Future Microbiol. 10, 21–34. doi: 10.2217/fmb.14.104
Le Gallou, S., Caron, G., Delaloy, C., Rossille, D., Tarte, K., and Fest, T. (2012). IL-2 requirement for human plasma cell generation: coupling differentiation and proliferation by enhancing MAPK–ERK signaling. J. Immunol. 189, 161–173. doi: 10.4049/jimmunol.1200301
Li, H., Sethuraman, N., Stadheim, T. A., Zha, D., Prinz, B., Ballew, N., et al. (2006). Optimization of humanized IgGs in glycoengineered Pichia pastoris. Nat. Biotechnol. 24, 210–215. doi: 10.1038/nbt1178
Liu, C. P., Tsai, T. I., Cheng, T., Shivatare, V. S., Wu, C. Y., Wu, C. Y., et al. (2018). Glycoengineering of antibody (Herceptin) through yeast expression and in vitro enzymatic glycosylation. Proc. Natl. Acad. Sci. U.S.A. 115, 720–725. doi: 10.1073/pnas.1718172115
Lorente-Rodríguez, A., Heidtman, M., and Barlowe, C. (2009). Multicopy suppressor analysis of thermosensitive YIP1 alleles implicates GOT1 in transport from the ER. J. Cell Sci. 122, 1540–1550. doi: 10.1242/jcs.042457
Love, K. R., Politano, T. J., Panagiotou, V., Jiang, B., Stadheim, T. A., and Love, J. C. (2012). Systematic single-cell analysis of Pichia pastoris reveals secretory capacity limits productivity. PLoS One 7:e37915. doi: 10.1371/journal.pone.0037915
Malsam, J., Kreye, S., and Söllner, T. H. (2008). Membrane traffic in the secretory pathway: Membrane fusion: SNAREs and regulation. Cell. Mol. Life Sci. 65, 2814–2832. doi: 10.1007/s00018-008-8352-3
Mumberg, D., Muller, R., and Funk, M. (1994). Regulatable promoters of Saccharomyces cerevisiae: comparison of transcriptional activity and their use for heterologous expression. Nucleic Acids Res. 22, 5767–5768. doi: 10.1093/nar/22.25.5767
Mumberg, D., Müller, R., and Funk, M. (1995). Yeast vectors for the controlled expression of heterologous proteins in different genetic backgrounds. Gene 156, 119–122. doi: 10.1016/0378-1119(95)00037-7
Nakanishi, H., Nakayama, K. I., Yokota, A., Tachikawa, H., Takahashi, N., and Jigami, Y. (2001). HutI proteins identified in Saccharomyces cerevisiae and Schizosaccharomyces pombe are functional homologues involved in the protein-folding process at the endoplasmic reticulum. Yeast 18, 543–554. doi: 10.1002/yea.707
Nasab, F. P., Aebi, M., Bernhard, G., and Frey, A. D. (2013). A combined system for engineering glycosylation efficiency and glycan structure in Saccharomyces cerevisiae. Appl. Environ. Microbiol. 79, 997–1007. doi: 10.1128/AEM.02817-12
Oracki, S. A., Walker, J. A., Hibbs, M. L., Corcoran, L. M., Tarlinton, D. M., and Tarlinton, D. M. (2010). Plasma cell development and survival. Immunol. Rev. 237, 140–159. doi: 10.1111/j.1600-065x.2010.00940.x
Pages, H., Carlson, M., Falcon, S., and Li, N. (2016). AnnotationDbi: Annotation Database Interface. R Package Version 1.34.4.
Payne, T., Finnis, C., Evans, L. R., Mead, D. J., Avery, S. V., Archer, D. B., et al. (2008). Modulation of chaperone gene expression in mutagenized Saccharomyces cerevisiae strains developed for recombinant human albumin production results in increased production of multiple heterologous proteins. Appl Env. Microbiol. 74, 7759–7766. doi: 10.1128/AEM.01178-08
Piirainen, M. A., Boer, H., De Ruijter, J. C., and Frey, A. D. (2016). A dual approach for improving homogeneity of a human-type N-glycan structure in Saccharomyces cerevisiae. Glycoconj. J. 33, 189–199. doi: 10.1007/s10719-016-9656-4
Pincus, D., Chevalier, M. W., Aragón, T., van Anken, E., Vidal, S. E., El-Samad, H., et al. (2010). BiP binding to the ER-stress sensor Ire1 tunes the homeostatic behavior of the unfolded protein response. PLoS Biol. 8:e1000415. doi: 10.1371/journal.pbio
Powers, D. B., Amersdorfer, P., Poul, M.-A., Nielsen, U. B., Shalaby, M. R., Adams, G. P., et al. (2001). Expression of single-chain Fv-Fc fusions in Pichia pastoris. J. Immunol. Methods 251, 123–135. doi: 10.1016/s0022-1759(00)00290-8
Rakestraw, J. A., Sazinsky, S. L., Piatesi, A., Antipov, E., and Wittrup, K. D. (2009). Directed evolution of a secretory leader for the improved expression of heterologous proteins and full-length antibodies in Saccharomyces cerevisiae. Biotechnol. Bioeng. 103, 1192–1201. doi: 10.1002/bit.22338
Romijn, E. P., Christis, C., Wieffer, M., Gouw, J. W., Fullaondo, A., van der Sluijs, P., et al. (2005). Expression clustering reveals detailed co-expression patterns of functionally related proteins during B cell differentiation. Mol. Cell. Proteomics 4, 1297–1310. doi: 10.1074/mcp.m500123-mcp200
Sanchez-Garcia, L., Martín, L., Mangues, R., Ferrer-Miralles, N., Vázquez, E., and Villaverde, A. (2016). Recombinant pharmaceuticals from microbial cells: a 2015 update. Microb. Cell Fact. 15, 33. doi: 10.1186/s12934-016-0437-3
Shaffer, A. L., Shapiro-Shelef, M., Iwakoshi, N. N., Lee, A. H., Qian, S. B., Zhao, H., et al. (2004). XBP1, downstream of Blimp-1, expands the secretory apparatus and other organelles, and increases protein synthesis in plasma cell differentiation. Immunity 21, 81–93. doi: 10.1016/j.immuni.2004.06.010
Shannon, P., Markiel, A., Ozier, O., Baliga, N., Wang, J., Ramage, D., et al. (2003). Cytoscape: a software environment for integrated models of biomolecular interaction networks. Genome Res. 13, 2498–2504. doi: 10.1101/gr.1239303
Sheng, J., Flick, H., and Feng, X. (2017). Systematic optimization of protein secretory pathways in Saccharomyces cerevisiae to increase expression of hepatitis B Small Antigen. Front. Microbiol. 8:875. doi: 10.3389/fmicb.2017.00875
Shusta, E. V., Raines, R. T., Pluckthun, A., and Wittrup, K. D. (1998). Increasing the secretory capacity of Saccharomyces cerevisiae for production of single-chain antibody fragments. Nat Biotechnol 16, 773–777. doi: 10.1038/nbt0898-773
Sidrauski, C., and Walter, P. (1997). The transmembrane kinase Ire1p is a site-specific endonuclease that initiates mRNA splicing in the unfolded protein response. Cell 90, 1031–1039. doi: 10.1016/s0092-8674(00)80369-4
Smith-Gill, S. J., Mainhart, C. R., Lavoie, T. B., Rudikoff, S., and Potter, M. (1984). VL-VH expression by monoclonal antibodies recognizing avian lysozyme. J. Immunol. 132, 963–967.
Tarlinton, D., Radbruch, A., Hiepe, F., and Dörner, T. (2008). Plasma cell differentiation and survival. Curr. Opin. Immunol. 20, 162–169. doi: 10.1016/j.coi.2008.03.016
Taxis, C., and Knop, M. (2006). System of centromeric, episomal, and integrative vectors based on drug resistance markers for Saccharomyces cerevisiae. Biotechniques 40, 73–78. doi: 10.2144/000112040
Uccelletti, D., Staneva, D., Rufini, S., Venkov, P., and Palleschi, C. (2005). Enhanced secretion of heterologous proteins in Kluyveromyces lactis by overexpression of the GDP-mannose pyrophosphorylase. KlPsa1p. FEMS Yeast Res. 5, 735–746. doi: 10.1016/j.femsyr.2005.01.004
Valkonen, M., Penttilä, M., and Saloheimo, M. (2003a). Effects of inactivation and constitutive expression of the unfolded- protein response pathway on protein production in the yeast Saccharomyces cerevisiae. Appl. Environ. Microbiol. 69, 2065–2072. doi: 10.1128/aem.69.4.2065-2072.2003
Valkonen, M., Ward, M., Wang, H., Penttilä, M., and Saloheimo, M. (2003b). Improvement of foreign-protein production in Aspergillus niger var. awamori by Constitutive Induction of the Unfolded-Protein Response. Appl. Environ. Microbiol. 69, 6979–6986. doi: 10.1128/aem.69.12.6979-6986.2003
Van Anken, E., Romijn, E. P., Maggioni, C., Mezghrani, A., Sitia, R., Braakman, I., et al. (2003). Sequential waves of functionally related proteins are expressed when B cells prepare for antibody secretion. Immunity 18, 243–253. doi: 10.1016/s1074-7613(03)00024-4
Victora, G. D., and Nussenzweig, M. C. (2012). Germinal centers. Annu. Rev. Immunol. 30, 429–457. doi: 10.1146/annurev-immunol-020711-075032
Walsh, G. (2014). Biopharmaceutical benchmarks 2014. Nat. Biotechnol. 32, 992–1000. doi: 10.1038/nbt.3040
Wang, G., Björk, S. M., Huang, M., Liu, Q., Campbell, K., Nielsen, J., et al. (2019). RNAi expression tuning, microfluidic screening, and genome recombineering for improved protein production in Saccharomyces cerevisiae. Proc. Natl. Acad. Sci. U.S.A. 116, 9324–9332. doi: 10.1073/pnas.1820561116
Wei, Y., Li, C., Huang, W., Li, B., Strome, S., and Wang, L. X. (2008). Glycoengineering of human IgG1-Fc through combined yeast expression and in vitro chemoenzymatic glycosylation. Biochemistry 47, 10294–10304. doi: 10.1021/bi800874y
Keywords: transcriptomics, plasma cell differentiation, antibody, Saccharomyces cerevisiae, synthetic biology
Citation: Koskela EV, Gonzalez Salcedo A, Piirainen MA, Iivonen HA, Salminen H and Frey AD (2020) Mining Data From Plasma Cell Differentiation Identified Novel Genes for Engineering of a Yeast Antibody Factory. Front. Bioeng. Biotechnol. 8:255. doi: 10.3389/fbioe.2020.00255
Received: 30 October 2019; Accepted: 12 March 2020;
Published: 31 March 2020.
Edited by:
Chun Li, Beijing Institute of Technology, ChinaCopyright © 2020 Koskela, Gonzalez Salcedo, Piirainen, Iivonen, Salminen and Frey. This is an open-access article distributed under the terms of the Creative Commons Attribution License (CC BY). The use, distribution or reproduction in other forums is permitted, provided the original author(s) and the copyright owner(s) are credited and that the original publication in this journal is cited, in accordance with accepted academic practice. No use, distribution or reproduction is permitted which does not comply with these terms.
*Correspondence: Alexander D. Frey, alexander.frey@aalto.fi
†Present address: Essi V. Koskela, Euretos B.V., Utrecht, the Netherlands; Alina Gonzalez Salcedo, Institute for Technical Chemistry, Leibniz University Hannover, Hanover, Germany; Heidi A. Iivonen, Bynfo Oy, Helsinki, Finland