- 1The Norwegian Centre for Movement Disorders, Stavanger University Hospital, Stavanger, Norway
- 2Department of Research, Section of Biostatistics, Stavanger University Hospital, Stavanger, Norway
- 3Department of Neurology, Haukeland University Hospital, Bergen, Norway
- 4Department of Clinical Medicine, University of Bergen, Bergen, Norway
- 5Department of Neurology, Stavanger University Hospital, Stavanger, Norway
- 6Department of Mathematics and Natural Sciences, University of Stavanger, Stavanger, Norway
- 7The Centre for Organelle Research, University of Stavanger, Stavanger, Norway
Introduction: Impulse control disorders (ICDs) are frequent non-motor symptoms in Parkinson’s disease (PD), with potential negative effects on the quality of life and social functioning. ICDs are closely associated with dopaminergic therapy, and genetic polymorphisms in several neurotransmitter pathways may increase the risk of addictive behaviors in PD. However, clinical differentiation between patients at risk and patients without risk of ICDs is still troublesome. The aim of this study was to investigate if genetic polymorphisms across several neurotransmitter pathways were associated with ICD status in patients with PD.
Methods: Whole-exome sequencing data were available for 119 eligible PD patients from the Norwegian ParkWest study. All participants underwent comprehensive neurological, neuropsychiatric, and neuropsychological assessments. ICDs were assessed using the self-report short form version of the Questionnaire for Impulsive-Compulsive Disorders in PD. Single-nucleotide polymorphisms (SNPs) from 17 genes were subjected to regression with elastic net penalization to identify candidate variants associated with ICDs. The area under the curve of receiver-operating characteristic curves was used to evaluate the level of ICD prediction.
Results: Among the 119 patients with PD included in the analysis, 29% met the criteria for ICD and 63% were using dopamine agonists (DAs). Eleven SNPs were associated with ICDs, and the four SNPs with the most robust performance significantly increased ICD predictability (AUC = 0.81, 95% CI 0.73–0.90) compared to clinical data alone (DA use and age; AUC = 0.65, 95% CI 0.59–0.78). The strongest predictive factors were rs5326 in DRD1, which was associated with increased odds of ICDs, and rs702764 in OPRK1, which was associated with decreased odds of ICDs.
Conclusion: Using an advanced statistical approach, we identified SNPs in nine genes, including a novel polymorphism in DRD1, with potential application for the identification of PD patients at risk for ICDs.
Introduction
Patients with Parkinson’s disease (PD) have a threefold increased odd for developing impulse control disorders (ICDs) and related compulsive behaviors when compared to controls (1, 2). These behaviors are characterized by lacking control of rewarding behaviors, such as gambling, sexual activity, eating, and buying. In addition, patients may also develop a preoccupation with hobbies, punding behaviors, and an addiction-like pattern of dopaminergic medication use. Although common in PD, ICDs are not merely a result of PD pathology (3), but are closely associated with the use of dopaminergic replacement therapy (DRT), such as dopamine agonists (DAs) (1, 2, 4). Still, not all patients develop ICDs when exposed to dopaminergic medications, arguing that some individuals are more susceptible to DRT than others. Previously identified demographic-risk factors, such as familial history of addiction, increased impulsivity, and novelty-seeking traits (1, 5), argue that the individual vulnerability may be of genetic origin.
To date, the evaluation of ICD susceptibility in PD has primarily focused on independent associations of single genetic variants. Several studies have reported an association between ICD development in PD patients and genetic polymorphisms in dopamine receptor (DRD1–3) and glutamate receptor (GRIN2B) genes (6–9), while individual studies also point toward a potential association with genetic polymorphisms in serotonin receptor (HTR2A), dopamine transporter (DAT1), and tryptophan hydroxylase 2 (TPH2) genes (10, 11). Recently, the spectrum of monoaminergic ICD candidate genes was expanded through the identification of a polymorphism in OPRK1, which encodes an opioid receptor, as the strongest genetic predictive factor in a clinical–genetic model designed to predict the occurrence of ICDs in early PD in the Parkinson’s Progression Markers Initiative (PPMI) cohort (12). The authors further reported that the inclusion of a panel of candidate-genetic variants improved the prediction of incident ICDs (identifying up to 76% of incident ICD cases in early-stage PD patients) compared to prediction based on clinical variables alone (12), arguing for the potential clinical utility of genetic testing. The authors estimated that common genetic variants accounted for 57% of the variance of ICD incidence among PD patients in the PPMI study. This heritability estimate is comparable to estimates from the general population, but current knowledge about individual risk genes is limited. We suggest that several neurotransmitter systems may contribute to ICD pathogenesis, and multiple genes within one system may play a crucial role in the pathogenesis of these behaviors.
To date, the identification of patients at risk of ICDs remains a primary aim in clinical research. Although several genetic polymorphisms have been suggested to aid clinical identification of ICD risk, most published studies utilize a candidate-gene approach based on previously published findings. In this study, we aimed to determine the association of genetic polymorphisms across several neurotransmitter pathways using an advanced statistical approach. A secondary aim was to investigate the clinical utility of a genetic panel in the prediction of ICD status in patients with PD.
Materials and Methods
Study Design
This cross-sectional study is based on participants from the Norwegian ParkWest study, a population-based longitudinal study of incident PD. The ParkWest cohort is composed of patients with newly diagnosed PD and normal control subjects recruited from four counties in Norway between 2004 and 2006, who were prospectively followed up by movement disorder neurologists. A detailed presentation of the diagnostic procedures and case ascertainment has previously been published (13). Screening for ICDs was first introduced at 5-year follow-up, and this study included 155 patients with PD who still remained in the study after 5 years of follow-up. Of these, 28 patients were excluded due to dementia and two due to missing data on Questionnaire for Impulsive-Compulsive Disorders in Parkinson’s Disease (QUIP), leaving 125 patients eligible for this study. Patients with missing information on relevant genetic variants (n = 6) were removed from this study.
Clinical Measures
A standardized examination program was administered by trained members of the ParkWest study group. Information regarding demographic variables, lifestyle factors, clinical history, and medication was obtained using semi-structured interviews. Severity of motor symptoms was assessed using the Unified Parkinson’s Disease Rating Scale (UPDRS) part III (14). Self-evaluated functioning on activities of daily life and complications of dopaminergic therapy were assessed using UPDRS parts II and IV. Hoehn and Yahr (H&Y) was used to assess disease stage (15). Levodopa equivalent doses (LEDs) were calculated according to published recommendations (16). Mini-Mental State Examination (MMSE) was used to assess global cognitive functioning (17). The Montgomery and Aasberg Depression Rating Scale (MADRS) was used to assess depressive symptoms (18). Lastly, ICDs were assessed using the self-report short form version of the QUIP (19). Participants with a positive response to one or more screening questions of the QUIP were classified to have ICD (20).
Candidate Gene and Variant Selection
Of the 125 patients eligible for this study, 119 had previously been characterized by whole-exome sequencing (WES) (unpublished material). We selected 16 genes (ADRA2C, DRD1–5, SLC6A3/DAT1, DDC, COMT, SLC6A4/5HTTLPR, TPH2, HTR2A, OPRM1, OPRK1, GRIN2B, and BDNF) based on established roles in candidate neurotransmitter pathways, or a published involvement in ICD and related behaviors in either patients with PD or in non-PD populations. This was achieved by performing a literature search, and the genes identified were involved in four neurotransmitter pathways (dopaminergic, serotonergic, glutamatergic and opioid) (6–12). All variants (n = 185) present in the candidate-gene regions were extracted using ingenuity variant analysis (Qiagen, CA, USA) and filtered to retain only those with minor allele frequency (MAF) >0.5 in the ParkWest and the 1,000 genomes project (n = 71). A further 12 single-nucleotide polymorphisms (SNPs) were removed based on a high linkage disequilibrium (LD) measured using the Broad Institute SNP Annotation and Proxy Search (SNAP) (21). In addition, two SNPs that have frequently been studied in ICDs in PD, but which were not in the original data extraction, were also included: rs1800497 in ANKK1 was extracted from the WES data and rs6280 in DRD3 was genotyped using a custom-made TaqMan SNP-genotyping assay (Thermo Fisher Scientific), as described (22). For further analysis, the genotypes were converted to carrier status, and five variants removed due to a carrier frequency >95% in the study population.
Statistical Analyses
Statistical procedures were performed using IBM SPSS Statistics version 24.0.0.1, R 3.4.0 and STATA IC 14.2. Group differences were analyzed using t-tests, Mann–Whitney tests, χ2–tests, and Fisher exact tests as appropriate.
Performing an extensive investigation of genetic variants associated with ICDs is inherently difficult due to the large number of possible variants identified in a single neurotransmitter pathway. The number of variants (p) will often exceed the number of participants (n) in the study. In these cases (p >> n), the traditional strategies for multivariable regression modeling will fail. An option here is to assume a sparse solution, i.e., that only a small subset of variants are involved in a single neurotransmitter pathway. Recent advances in statistical modeling, such as elastic net (EN) regularized generalized linear regression, reduce the number of predictors by penalizing those that do not have enough prediction power. This allows one to reduce the risk of overfitted models and increase the generalizability to other cohorts (23, 24). In this study, regularized logistic regression with EN penalization was used to identify SNPs associated with ICDs. Regularized regression with EN is well suited for model selection of high-dimensional data, as is often the case in analyses of genetic polymorphisms in clinical cohorts (23, 25). In addition, EN handles variants with high LD and multiple SNPs from one neurotransmitter pathways well (26).
Elastic net analyses were performed in R, using the glmnet-package (27). The level of regularization parameter λ was chosen as the minimal λ that yielded prediction error estimated by cross-validation within one standard error from its minimal value. In the glmnet, the parameter α decides the balance between l1 and l2 regularizations, of which the former is the regularization used in Lasso regression (α = 1) and the latter is used in Ridge regression (α = 0). In our analyses, the EN was repeated for all α from 0 to 1, with 0.01 increments. Non-zero estimated coefficients consistent throughout the entire range of α support the evidence of associations between relevant SNPs and ICD status.
The discriminative ability of the biomarkers with regard to ICD diagnosis was assessed from receiver-operating characteristic (ROC) curve analysis. The test variable was the predicted probability from logistic regression with ICD diagnosis (yes/no) as outcome. In order to not overfit the model, the four SNPs with a most robust performance in EN analysis were selected as candidate SNPs. Robustness of candidate SNPs was defined by the consistency of the estimated B-values in EN analyses (which are visually represented by color in Figure 1). The ROC curve was plotted with preselected clinical variables alone (age and either DA use), for the genetic variables alone (genetic model), and with the clinical and candidate SNP data combined (clinical–genetic model). Area-under-the-curve (AUC) values were compared using DeLong test.
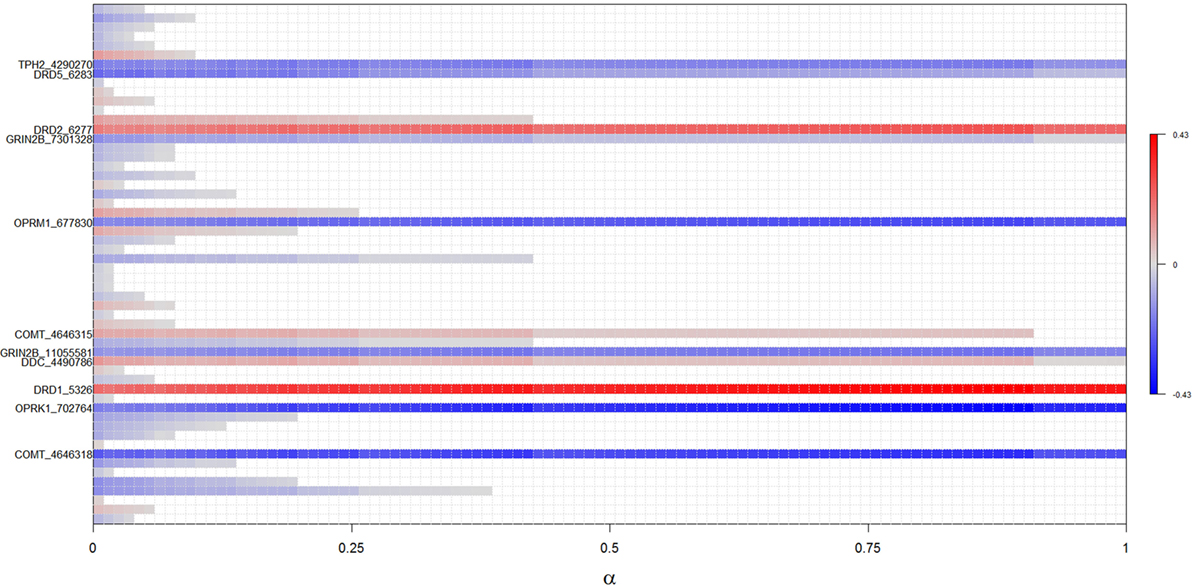
Figure 1. Results of regularized regression with elastic net penalization for α-values between 0 and 1. Polymorphisms positively associated with ICDs (i.e., increases risk) are highlighted with red, while polymorphisms negatively associated with ICDs (i.e., decreases risk) are highlighted in blue, with the intensity of color reflecting the strength of association. Polymorphisms not associated with ICDs are white. Identified polymorphisms demonstrate significant association across all levels of α.
Results
Demographic and Clinical Characteristics
Demographic and clinical characteristics are presented in Table 1. Of 119 patients in the study, 29.4% (35/119) reported at least one ICD. Patients with ICD did not differ from patients without ICD in terms of sex, education, duration of PD, MMSE scores, or scores on UPDRS II, III, or IV, but patients with ICDs tended to be younger (p = 0.050) and scored significantly higher on MADRS (p = 0.010). Patients with ICDs also used DA more frequently (p = 0.001) and had a higher total LED (p = 0.017). DA dosage was not different when comparing DA users with ICDs with those without ICDs (p = 0.958).
Variant Selection
The complete results from EN analyses are presented in Figure 1. Fifty-six SNPs were identified across the genes selected for analysis (Table S1 in Supplementary Material), and 11 SNPs from four neurotransmitter pathways were robustly associated with ICDs across all levels of α in the EN analysis (Figure 1; Table 2). Specifically, carriers of the minor alleles of the DRD1 rs5326, DRD2 rs6277, COMT rs4646315, and DDC rs4490786 SNPs were associated with an increased risk of ICDs. Carriers of the minor allele of the OPRM1 rs677830, OPRK1 rs702764, GRIN2B rs1105581 and rs7301328, COMT rs4646318, TPH2 rs4290270, DRD5 rs6283 SNPs were associated with a decreased risk of ICDs. Of these, the DRD1 rs5326, OPRK1 rs702764, OPRM1 rs677830, and COMT rs4646318 were most robustly associated with ICD status and thus considered candidate variants.
Prediction of ICDs
The prediction of ICDs was estimated by using ROC curves with AUC (Figure 2). In the clinical model, ROC curves plotted with the clinical variables age and DA use yielded an estimated AUC of 0.68 (95% CI 0.59–0.78). In this analysis, DA use [odds ratio (OR) 4.5; 95% CI 1.5–13.5; p = 0.006] was associated with the presence of ICDs. The genetic model, consisting of the SNPs DRD1 rs5326, OPRK1 rs702764, OPRM1 rs677830, and COMT rs4646318, yielded an estimated AUC of 0.70 (95% CI: 0.61–0.79). Of these, one variant, the DRD1 SNP rs5326, was significantly associated with ICDs (OR 2.9; 95% CI 1.1–7.6; p = 0.026).
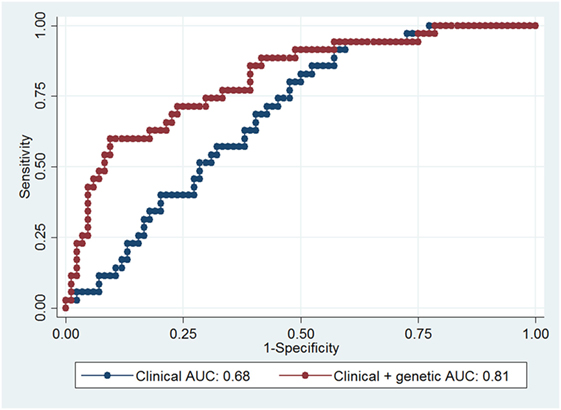
Figure 2. Receiver-operating characteristic (ROC) curves for prediction of impulse control disorders (ICDs). The blue curve was plotted with clinical variables (age and dopamine agonist use), while the red curve was plotted with clinical and the four candidate single-nucleotide polymorphisms. Area under the curve (AUC) for each model is indicated in the figure.
In the clinical–genetic model, we included four candidate SNPs identified in the EN analyses, resulting in an estimated AUC of 0.81 (95% CI 0.73–0.90). This 13% point increase in AUC between the clinical and the clinical–genetic model was statistically significant (p = 0.003). Similarly, the 11% point increase in AUC between the genetic and the clinical–genetic model was also significant (p = 0.008). In the clinical–genetic model, DA use (OR 7.4; 95% CI 2.1–26.2; p = 0.002) was again associated with increased odds of ICDs, and the significant genetic predictors DRD1 SNP rs5326 (OR 6.1; 95% CI 1.9–19.6; p = 0.003) and OPRK1 SNP rs702764 (OR 0.2; 95% CI 0.1–0.8; p = 0.040) were associated with an increased and a decreased risk of ICDs, respectively. Full details of the clinical and the clinical–genetic models are presented in Table 3.
Discussion
In this study, we identified an association between ICDs and SNPs in the dopaminergic, glutamatergic, serotonergic, and opioid neurotransmitter system using an advanced statistical procedure. Using four polymorphisms from this panel significantly increased the level of prediction of ICD status beyond known clinical risk factors. These results confirm and expand existing knowledge about the genetic architecture of ICDs in PD. To date, this is the most extensive investigation of polymorphisms in relation to ICDs in PD.
Guiding Clinical Practice Using Genetic Markers
Despite new insights into the pathophysiology of ICDs in PD, a consistent model for clinical differentiation between patients with high and low risk of ICDs has still not been developed. Although younger age has been associated with ICDs in several cohorts, DA is more often prescribed to younger patients than that to older. As evident in the clinical model of ICD risk, age is not significantly associated with ICDs when controlling for DA use (Table 3). Even though DA use is the predominant risk factor for ICDs in patients with PD, DA is still a preferred drug in the early stages of PD due to the diminishing effects of levodopa over time. Therefore, the identification of risk factors that predict ICDs before exposure to DA is important to guide clinical practice. Genetic panels have been advocated to be a clinically useful predictor of disease and may be especially important when investigating common polymorphisms, which may have a small effect size and be contingent upon gene-by-environment interactions. Recently, a predictive genetic panel for ICDs in PD has been proposed. Kraemmer and colleagues utilized a panel of 13 candidate polymorphisms, which in concert with clinical variables resulted in an AUC of 76% (95% CI 70–83%) for prediction of ICDs. Our findings support the use of a genetic and clinical model in the prediction of ICDs in PD and also advocate for an approach in which genetic variants are selected based on not only the previously published literature but also using a statistical approach that can handle a gamut of variants. Using such an approach, we have replicated the finding that OPRK1 rs702764 is associated with ICDs when controlling for DA use and identified a novel association between an SNP in DRD1 and ICDs. In addition, we also identified a sparse clinical–genetic model with a high degree of prediction [AUC of 81% (95% CI 73–90%)] of ICD status, using only four candidate SNPs.
Dopaminergic Pathways
When controlling for DA use and age, we identified two genes with polymorphisms that were independently associated with ICDs (Table 3). rs5326 is positioned in the 5′ untranslated region (UTR) of the DRD1 gene, which encodes the dopamine receptor D1, and was associated with an increased risk of ICDs. The D1 receptor is the most abundant dopamine receptor in the central nervous system, particularly expressed in the prefrontal areas, and is considered a modulator of dopaminergic activity (29). Stimulation of D1 receptors by agonists or illicit drugs (like cocaine and amphetamine) has been suggested to trigger punding and hobbyism behaviors in both patients with PD and patients with addiction (30). Previously, polymorphisms in the noncoding regions of DRD1 (rs4867798 in the 3'-UTR and rs4532 in the 5'-UTR) have been associated with ICDs in a Malaysian PD cohort (8). Furthermore, polymorphisms in DRD1 have been linked to ICDs, neuropsychiatric disease, problem gambling, addiction, and cognitive functioning in non-PD populations (31, 32). Risk variants of rs5326 have been associated with a decreased DRD1 expression, a reduced cognitive functioning in both healthy males and bipolar patients, and an increased risk of neuropsychiatric disorders, such as schizophrenia and heroin addiction (33–36).
Few studies have investigated the DRD1 gene with regard to ICDs in PD, while considerable effort has been made in identifying polymorphisms in DRD2 and DRD3, mostly due to the established importance of these genes in ICDs in the general population and the high affinity of DAs to these receptors (37, 38). In our data, the rs6277 SNP in DRD2 was robustly associated with ICDs in the EN analysis, but was not a strong individual predictor of ICD in regression analysis. rs6277 has previously been associated with individual differences in cognitive functioning, reward processing, and impulsivity (39–45). Although the association between ICDs and the rs6277 is novel, it should be noted that this SNP has not been included in previous studies of ICDs in PD. Several other genetic variants in DRD2, including rs6277 neighboring SNP rs1800497 (Taq1A), have been studied in PD and found to be associated with ICDs, although not in all studies (6–8, 12).
The D1 and D2 receptors have been suggested to have opposing roles in reward processing, modulating reward and avoidance-based learning, respectively (46). However, the precise interplay between polymorphisms in DRD1 and DRD2 and the presentation of ICDs is largely unknown. One theory suggests that polymorphisms in the promoter region of DRD1 can affect mRNA stability and result in a lower expression of the D1 receptor itself (8, 32). Given the modulating role of the DRD1 gene in dopaminergic signaling and reward processing, patients with polymorphisms may be prone to a hyperdopaminergic state when exposed to DRT. Similarly, some authors have speculated that polymorphisms in DRD2, like the Taq1A polymorphism, may result in modifications in the protein structure of the receptor and ultimately lead to a reduced expression of the D2 receptor (8). This theory is supported by neuroimaging studies that have identified low D2/D3 receptor availability in ventral striatum in patients with ICDs [see (47) for a review]. However, it is still unknown if polymorphisms in these SNPs can result in a reduced expression of D1 and D2 receptors and, if so, if these polymorphisms result in functional dysfunctions, like aberrant reward processing. In order to test these theories, studies at the cellular and molecular levels are needed.
Opioid Pathways
The second polymorphism having an independent association with ICDs was rs702764, located in the kappa-opioid receptor (OPRK1) gene. This polymorphism was negatively associated with ICDs in the clinical–genetic model. OPRK1 encodes the kappa-opioid receptor 1 (KOR1), which is one of four-related opioid receptors in the brain. KOR1 is involved in processes such as feeding behavior, pain management, and addiction. In rodent models, the OPRK1 gene has been shown to modulate dopaminergic tone, suggesting that OPRK1 is involved in reward processing (48, 49). Previously, the TC genotype of the OPRK1 SNP rs702764 has been associated with incident ICDs (12). The neurophysiology between KOR1 and dopamine signaling is not fully understood, but some authors have suggested that the opioid receptors mu1 (MOR1) and KOR1 have opposing roles in the modulation of basal dopaminergic tone in the nucleus accumbens (50–52). Thus, the involvement of the OPRK1 in modifying the risk of ICDs may be of special interest due to the potential for pharmacological interventions with opioid antagonists. The opioid antagonist naltrexone, which has high affinity to the MOR1 and KOR1, has been deemed efficacious in reducing the severity of other ICDs, such as hoarding and compulsive disorders in the general population. To date, only one trial with PD patients has been published (53). Although naltrexone was not associated with change on the Clinical Global Impression scale, naltrexone was associated with significant changes in QUIP score, arguing that further studies are warranted.
The possible association between polymorphisms in dopamine and opioid receptors and ICDs is interesting, as they are also considered candidate genes for what has been termed “reward deficiency syndrome,” a hypothesized neuropsychological state characterized by decreased feelings of satisfaction caused by gene-by-environment interactions (37, 54, 55). This theory, composed of evidence from ICD patients without PD, suggests that polygenic variability, given the right environmental factors, could result in a hypodopaminergic state that causes insensitivity to reward and results in an atypical reward-seeking behavior, as often seen in patients with behavioral or chemical addictions. However, the current models of ICDs in PD suggest that ICDs in PD are a result of a hyperdopaminergic state, caused by exogenous dopamine and possibly exacerbated by frontal cognitive dysfunctions (56, 57). Based on these observations, one might argue that although ICDs in patients with PD and patients without PD are similar in terms of phenotype and share genetic risk profiles, the gene-by-environment profiles and pathophysiology might differ in the two populations.
Strengths and Limitations
There are several limitations that should be considered. First, we have not validated our findings in an external cohort, making generalization or clinical utility of these findings impossible before replication. Despite this, our approach positively identifies variants previously associated with ICDs in the PPMI study (12) and provides new insights into the genetic architecture of ICDs in PD. A second limitation is the use of QUIP as a definition of ICDs. This measure has high sensitivity, but lacks specificity and may inflate the frequency estimates of ICDs. Third, causative relations between the identified genetic polymorphisms and ICDs are difficult to infer based on the current research design. Due to the involvement of DA in ICD development, one might argue that the identified SNPs could increase the risk of DA use, rather than ICDs. We have attempted to meet this challenge by adopting a clinical–genetic model that controls for DA use. Strengths of this study include the use of patients with and without ICDs that are matched in terms of motor impairment and H&Y stage. As argued by Cormier and colleagues, investigations into the genetic architecture of ICDs in PD should include matched groups in terms of motor impairment, H&Y stage, and DA LED (58). Although patients differed in terms of total LED, patients with ICDs were not significantly different than patients without ICDs in terms of DA LED. Lastly, we argue that using an advanced statistical approach that yields robust findings when analyzing a large amount of variants is a major strength of this study.
Conclusion
Our findings demonstrate that a genetic panel (DRD1, OPRK1, OPRM1, and COMT) can provide valuable information with regard to the clinical differentiation between PD patients at risk of ICDs and PD patients without risk. Using an advanced statistical approach, we also identified one novel polymorphism associated with ICDs in PD. Although promising, our results need replication in other, larger cohorts.
Ethics Statement
All subjects gave written informed consent in accordance with the Declaration of Helsinki. The protocol was approved by the Regional Committee for Medical and Health Research Ethics, Western Norway.
Author Contributions
AE was involved in the conception, design, statistical analysis, interpretation of data, and writing of the first draft. JG and KP were involved in the conception, design, interpretation of data, and supervision of the study. ID and AU were involved in statistical analysis and interpretation of data. JG, CT, and JC were involved in the analysis of genetic data. GA and OT were involved in the conception and study supervision. All authors made critical contributions and approved this manuscript.
Conflict of Interest Statement
The authors declare that the research was conducted in the absence of any commercial or financial relationships that could be construed as a potential conflict of interest.
Acknowledgments
The authors are grateful to all patients for their willingness to participate in this study and thank all personnel involved in planning and conducting the Norwegian ParkWest study.
Funding
This study was supported by the Research Council of Norway (grant# 177966) and the Western Norway Regional Health Authority (grant# 911218 and grant# 912014).
Supplementary Material
The Supplementary Material for this article can be found online at http://www.frontiersin.org/articles/10.3389/fneur.2018.00109/full#supplementary-material.
Table S1. Included genes in regularized regression with elastic net penalization.
Abbreviations
PD, Parkinson’s disease; ICD, impulse control disorder; QUIP, Questionnaire for Impulsive-Compulsive Disorders in Parkinson’s Disease; SNP, single-nucleotide polymorphism; ROC, receiver-operating characteristics; AUC, area under the curve; DRT, dopaminergic replacement therapy; DA, dopamine agonist; PPMI, Parkinson’s progression markers initiative; UPDRS, Unified Parkinson’s Disease Rating Scale; LED, levodopa equivalent dose; MAF, minor allele frequency; EN, elastic net; LD, linkage disequilibrium.
References
1. Weintraub D, Koester J, Potenza MN, Siderowf AD, Stacy M, Voon V, et al. Impulse control disorders in Parkinson disease: a cross-sectional study of 3,090 patients. Arch Neurol (2010) 67(5):589–95. doi:10.1001/archneurol.2010.65
2. Erga AH, Alves G, Larsen JP, Tysnes OB, Pedersen KF. Impulsive and compulsive behaviors in Parkinson’s disease: the Norwegian ParkWest study. J Parkinsons Dis (2017) 7(1):183–91. doi:10.3233/JPD-160977
3. Weintraub D, Papay K, Siderowf AD. Screening for impulse control symptoms in patients with de novo Parkinson disease—a case–control study. Neurology (2013) 80:176–80. doi:10.1212/WNL.0b013e31827b915c
4. Grosset DG, Cardoso F, Lees A. Dopamine agonists vs levodopa in impulse control disorders. Arch Neurol (2011) 68(4):544–6. doi:10.1001/archneurol.2011.41
5. Voon V, Thomsen T, Miyasaki JM, de Souza M, Shafro A, Fox SH, et al. Factors associated with dopaminergic drug-related pathological gambling in Parkinson disease. Arch Neurol (2007) 64(2):212–6. doi:10.1001/archneur.64.2.212
6. Lee JY, Lee EK, Park SS, Lim JY, Kim HJ, Kim JS, et al. Association of DRD3 and GRIN2B with impulse control and related behaviors in Parkinson’s disease. Mov Disord (2009) 24(12):1803–10. doi:10.1002/mds.22678
7. Hoenicka J, Garcia-Ruiz PJ, Ponce G, Herranz A, Martinez-Rubio D, Perez-Santamarina E, et al. The addiction-related gene ANKK1 in parkinsonian patients with impulse control disorder. Neurotox Res (2015) 27(3):205–8. doi:10.1007/s12640-014-9504-x
8. Zainal Abidin S, Tan EL, Chan SC, Jaafar A, Lee AX, Abd Hamid MH, et al. DRD and GRIN2B polymorphisms and their association with the development of impulse control behaviour among Malaysian Parkinson’s disease patients. BMC Neurol (2015) 15:59. doi:10.1186/s12883-015-0316-2
9. Krishnamoorthy S, Rajan R, Banerjee M, Kumar H, Sarma G, Krishnan S, et al. Dopamine D3 receptor Ser9Gly variant is associated with impulse control disorders in Parkinson’s disease patients. Parkinsonism Relat Disord (2016) 30:13–7. doi:10.1016/j.parkreldis.2016.06.005
10. Lee JY, Jeon BS, Kim HJ, Park SS. Genetic variant of HTR2A associates with risk of impulse control and repetitive behaviors in Parkinson’s disease. Parkinsonism Relat Disord (2012) 18(1):76–8. doi:10.1016/j.parkreldis.2011.08.009
11. Cilia R, Benfante R, Asselta R, Marabini L, Cereda E, Siri C, et al. Tryptophan hydroxylase type 2 variants modulate severity and outcome of addictive behaviors in Parkinson’s disease. Parkinsonism Relat Disord (2016) 29:96–103. doi:10.1016/j.parkreldis.2016.05.017
12. Kraemmer J, Smith K, Weintraub D, Guillemot V, Nalls MA, Cormier-Dequaire F, et al. Clinical–genetic model predicts incident impulse control disorders in Parkinson’s disease. J Neurol Neurosurg Psychiatry (2016) 87(10):1106–11. doi:10.1136/jnnp-2015-312848
13. Alves G, Muller B, Herlofson K, HogenEsch I, Telstad W, Aarsland D, et al. Incidence of Parkinson’s disease in Norway: the Norwegian ParkWest study. J Neurol Neurosurg Psychiatry (2009) 80(8):851–7. doi:10.1136/jnnp.2008.168211
14. Fahn S, Elton R. Unified Parkinson’s Disease Rating Scale. In: Fahn S, Marsden C, Calne D, editors. Recent Development in Parkinson’s Disease. Florham Park, NJ: MacMillan Health Care Information (1987). p. 153–63.
15. Hoehn MM, Yahr MD. Parkinsonism: onset, progression and mortality. Neurology (1967) 17(5):427–42. doi:10.1212/WNL.17.5.427
16. Tomlinson C, Stowe R, Patel S, Rick C, Gray R, Clarke C. Systematic review of levodopa dose equivalency reporting in Parkinson’s disease. Mov Disord (2010) 25(15):2649–53. doi:10.1002/mds.23429
17. Folstein MF, Folstein SE, McHugh PR. Mini mental state. A practical method for grading the cognitive state of patients for the clinician. J Psychiatr Res (1975) 12(3):189–98. doi:10.1016/0022-3956(75)90026-6
18. Montgomery SA, Asberg M. A new depression scale designed to be sensitive to change. Br J Psychiatry (1979) 134:382–9. doi:10.1192/bjp.134.4.382
19. Weintraub D, Mamikonyan E, Papay K, Shea JA, Xie SX, Siderowf A. Questionnaire for Impulsive-Compulsive Disorders in Parkinson’s Disease-Rating Scale. Mov Disord (2012) 27(2):242–7. doi:10.1002/mds.24023
20. Weintraub D, Hoops S, Shea JA, Lyons KE, Pahwa R, Driver-Dunckley ED, et al. Validation of the Questionnaire for Impulsive-Compulsive Disorders in Parkinson’s Disease. Mov Disord (2009) 24(10):1461–7. doi:10.1002/mds.22571
21. Johnson AD, Handsaker RE, Pulit SL, Nizzari MM, O’Donnell CJ, de Bakker PI. SNAP: a web-based tool for identification and annotation of proxy SNPs using HapMap. Bioinformatics (2008) 24(24):2938–9. doi:10.1093/bioinformatics/btn564
22. Lange J, Lunde KA, Sletten C, Moller SG, Tysnes OB, Alves G, et al. Association of a BACE1 gene polymorphism with Parkinson’s disease in a Norwegian population. Parkinsons Dis (2015) 2015:973298. doi:10.1155/2015/973298
23. Lange K, Papp JC, Sinsheimer JS, Sobel EM. Next generation statistical genetics: modeling, penalization, and optimization in high-dimensional data. Annu Rev Stat Appl (2014) 1(1):279–300. doi:10.1146/annurev-statistics-022513-115638
24. Pavlou M, Ambler G, Seaman S, De Iorio M, Omar RZ. Review and evaluation of penalised regression methods for risk prediction in low-dimensional data with few events. Stat Med (2016) 35(7):1159–77. doi:10.1002/sim.6782
25. Wei Z, Wang W, Bradfield J, Li J, Cardinale C, Frackelton E, et al. Large sample size, wide variant spectrum, and advanced machine-learning technique boost risk prediction for inflammatory bowel disease. Am J Hum Genet (2013) 92(6):1008–12. doi:10.1016/j.ajhg.2013.05.002
26. Huls A, Ickstadt K, Schikowski T, Kramer U. Detection of gene–environment interactions in the presence of linkage disequilibrium and noise by using genetic risk scores with internal weights from elastic net regression. BMC Genet (2017) 18:55. doi:10.1186/s12863-017-0519-1
27. Schelldorfer J, Meier L, Bühlmann P. GLMMLasso: an algorithm for high-dimensional generalized linear mixed models using l1-penalization. J Comput Graph Stat (2014) 23(2):460–77. doi:10.1080/10618600.2013.773239
28. van Dunnen J, Antonarakis S. Mutation nomenclature extensions and suggestions to describe complex mutations: a discussion. Hum Mutat (2000) 15:7–12. doi:10.1002/(SICI)1098-1004(200001)15:1<7::AID-HUMU4>3.0.CO;2-N
29. Rothmond DA, Weickert CS, Webster MJ. Developmental changes in human dopamine neurotransmission: cortical receptors and terminators. BMC Neurosci (2012) 13:18. doi:10.1186/1471-2202-13-18
30. Fasano A, Petrovic I. Insights into pathophysiology of punding reveal possible treatment strategies. Mol Psychiatry (2010) 15(6):560–73. doi:10.1038/mp.2009.95
31. Comings DE, Gade R, Wu S, Chiu C, Dietz G, Muhleman D, et al. Studies of the potential role of the dopamine D1 receptor gene in addictive behaviors. Mol Psychiatry (1997) 2(1):44–56. doi:10.1038/sj.mp.4000207
32. Huang W, Ma JZ, Payne TJ, Beuten J, Dupont RT, Li MD. Significant association of DRD1 with nicotine dependence. Hum Genet (2008) 123(2):133–40. doi:10.1007/s00439-007-0453-9
33. Pan Y, Yao J, Wang B. Association of dopamine D1 receptor gene polymorphism with schizophrenia: a meta-analysis. Neuropsychiatr Dis Treat (2014) 10:1133–9. doi:10.2147/NDT.S63776
34. Levran O, Randesi M, da Rosa JC, Ott J, Rotrosen J, Adelson M, et al. Overlapping dopaminergic pathway genetic susceptibility to heroin and cocaine addictions in African Americans. Ann Hum Genet (2015) 79(3):188–98. doi:10.1111/ahg.12104
35. Tsang J, Fullard JF, Giakoumaki SG, Katsel P, Katsel P, Karagiorga VE, et al. The relationship between dopamine receptor D1 and cognitive performance. NPJ Schizophr (2015) 1:14002. doi:10.1038/npjschz.2014.2
36. Zhao L, Lin Y, Lao G, Wang Y, Guan L, Wei J, et al. Association study of dopamine receptor genes polymorphism with cognitive functions in bipolar I disorder patients. J Affect Disord (2015) 170:85–90. doi:10.1016/j.jad.2014.08.039
37. Blum K, Sheridan P, Wood R, Braverman E, Chen TJ, Cull J, et al. The D2 dopamine receptor gene as a determinant of reward deficiency syndrome. J R Soc Med (1996) 89:396–400.
38. Seeman P. Parkinson’s disease treatment may cause impulse-control disorder via dopamine D3 receptors. Synapse (2015) 69(4):183–9. doi:10.1002/syn.21805
39. Ritchie T, Noble EP. Association of seven polymorphisms of the D2 dopamine receptor-binding characteristics. Neurochem Res (2003) 28(1):73–82. doi:10.1023/a:1021648128758
40. Rodriguez-Jimenez R, Hoenicka J, Jimenez-Arriero MA, Ponce G, Bagney A, Aragues M, et al. Performance in the Wisconsin Card Sorting Test and the C957T polymorphism of the DRD2 gene in healthy volunteers. Neuropsychobiology (2006) 54(3):166–70. doi:10.1159/000098652
41. Doehring A, Hentig N, Graff J, Salamat S, Schmidt M, Geisslinger G, et al. Genetic variants altering dopamine D2 receptor expression or function modulate the risk of opiate addiction and the dosage requirements of methadone substitution. Pharmacogenet Genomics (2009) 19(6):407–14. doi:10.1097/FPC.0b013e328320a3fd
42. Colzato LS, van den Wildenberg WP, Van der Does AJ, Hommel B. Genetic markers of striatal dopamine predict individual differences in dysfunctional, but not functional impulsivity. Neuroscience (2010) 170(3):782–8. doi:10.1016/j.neuroscience.2010.07.050
43. Markett SA, Montag C, Reuter M. The association between dopamine DRD2 polymorphisms and working memory capacity is modulated by a functional polymorphism on the nicotinic receptor gene CHRNA4. J Cogn Neurosci (2010) 22(9):1944–54. doi:10.1162/jocn.2009.21354
44. Stelzel C, Basten U, Montag C, Reuter M, Fiebach CJ. Frontostriatal involvement in task switching depends on genetic differences in d2 receptor density. J Neurosci (2010) 30(42):14205–12. doi:10.1523/JNEUROSCI.1062-10.2010
45. Markett S, Reuter M, Montag C, Weber B. The dopamine D2 receptor gene DRD2 and the nicotinic acetylcholine receptor gene CHRNA4 interact on striatal gray matter volume: evidence from a genetic imaging study. Neuroimage (2013) 64:167–72. doi:10.1016/j.neuroimage.2012.08.059
46. Cox SM, Frank MJ, Larcher K, Fellows LK, Clark CA, Leyton M, et al. Striatal D1 and D2 signaling differentially predict learning from positive and negative outcomes. Neuroimage (2015) 109:95–101. doi:10.1016/j.neuroimage.2014.12.070
47. Aracil-Bolanos I, Strafella AP. Molecular imaging and neural networks in impulse control disorders in Parkinson’s disease. Parkinsonism Relat Disord (2016) 22(Suppl 1):S101–5. doi:10.1016/j.parkreldis.2015.08.003
48. Chefer VI, Czyzyk T, Bolan EA, Moron J, Pintar JE, Shippenberg TS. Endogenous kappa-opioid receptor systems regulate mesoaccumbal dopamine dynamics and vulnerability to cocaine. J Neurosci (2005) 25(20):5029–37. doi:10.1523/JNEUROSCI.0854-05.2005
49. Levran O, Yuferov V, Kreek MJ. The genetics of the opioid system and specific drug addictions. Hum Genet (2012) 131(6):823–42. doi:10.1007/s00439-012-1172-4
50. Spanagel R, Herz A, Shippenberg TS. Opposing tonical active endogenous opioid systems modulate the mesolimbic dopaminergic pathway. Proc Natl Acad Sci U S A (1992) 89:2046–50. doi:10.1073/pnas.89.6.2046
51. Huiping Zhang ZL. Analyzing interaction of μ-, δ- and κ-opioid receptor gene variants on alcohol or drug dependence using a pattern discovery-based method. J Addict Res Ther (2012) 7:007. doi:10.4172/2155-6105.s7-007
52. Crowley NA, Kash TL. Kappa opioid receptor signaling in the brain: circuitry and implications for treatment. Prog Neuropsychopharmacol Biol Psychiatry (2015) 62:51–60. doi:10.1016/j.pnpbp.2015.01.001
53. Papay K, Xie SX, Stern M, Hurtig H, Siderowf A, Duda JE, et al. Naltrexone for impulse control disorders in Parkinson disease: a placebo-controlled study. Neurology (2014) 83(9):826–33. doi:10.1212/WNL.0000000000000729
54. Blum K, Braverman ER, Holder JM, Lubar JF, Monastra VJ, Miller D, et al. The reward deficiency syndrome: a biogenetic model for the diagnosis and treatment of impulsive, addictive and compulsive behaviors. J Psychoactive Drugs (2000) 32(Sup 1):1–112. doi:10.1080/02791072.2000.10736099
55. Blum K, Febo M, Badgaiyan RD, Demetrovics Z, Simpatico T, Fahlke C, et al. Common neurogenetic diagnosis and meso-limbic manipulation of hypodopaminergic function in reward deficiency syndrome (RDS): changing the recovery landscape. Curr Neuropharmacol (2017) 15(1):184–94. doi:10.2174/1570159X13666160512150
56. Santangelo G, Raimo S, Barone P. The relationship between impulse control disorders and cognitive dysfunctions in Parkinson’s disease: a meta-analysis. Neurosci Biobehav Rev (2017) 77:129–47. doi:10.1016/j.neubiorev.2017.02.018
57. Voon V, Napier TC, Frank MJ, Sgambato-Faure V, Grace AA, Rodriguez-Oroz M, et al. Impulse control disorders and levodopa-induced dyskinesias in Parkinson’s disease: an update. Lancet Neurol (2017) 16(3):238–50. doi:10.1016/S1474-4422(17)30004-2
Keywords: Parkinson’s disease, impulse control disorders, addiction, elastic net, OPRK1, DRD1
Citation: Erga AH, Dalen I, Ushakova A, Chung J, Tzoulis C, Tysnes OB, Alves G, Pedersen KF and Maple-Grødem J (2018) Dopaminergic and Opioid Pathways Associated with Impulse Control Disorders in Parkinson’s Disease. Front. Neurol. 9:109. doi: 10.3389/fneur.2018.00109
Received: 21 November 2017; Accepted: 14 February 2018;
Published: 28 February 2018
Edited by:
Mayela Rodríguez-Violante, Instituto Nacional de Neurología y Neurocirugía (INNN), MexicoReviewed by:
Daniel Martinez-Ramirez, University of Florida, United StatesFélix Javier Jiménez-Jiménez, Hospital Universitario del Sureste, Spain
Copyright: © 2018 Erga, Dalen, Ushakova, Chung, Tzoulis, Tysnes, Alves, Pedersen and Maple-Grødem. This is an open-access article distributed under the terms of the Creative Commons Attribution License (CC BY). The use, distribution or reproduction in other forums is permitted, provided the original author(s) and the copyright owner are credited and that the original publication in this journal is cited, in accordance with accepted academic practice. No use, distribution or reproduction is permitted which does not comply with these terms.
*Correspondence: Aleksander H. Erga, aleksander.erga@gmail.com