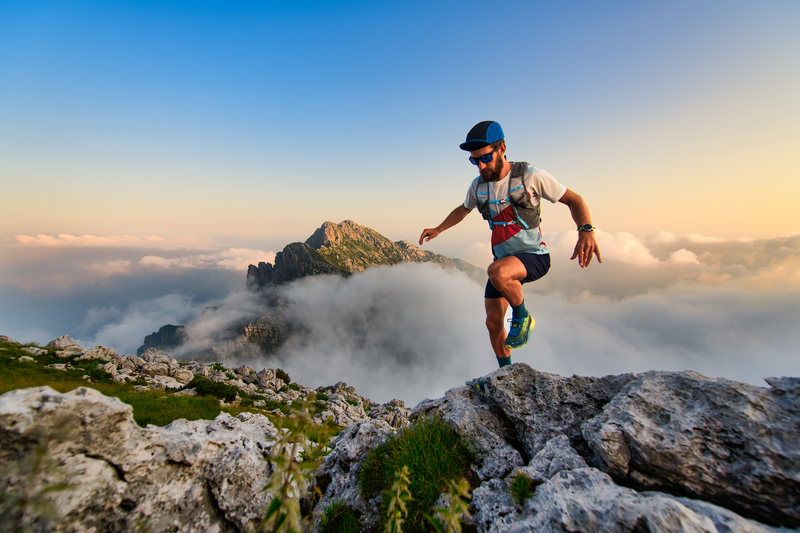
95% of researchers rate our articles as excellent or good
Learn more about the work of our research integrity team to safeguard the quality of each article we publish.
Find out more
REVIEW article
Front. Energy Res. , 20 December 2017
Sec. Sustainable Energy Systems
Volume 5 - 2017 | https://doi.org/10.3389/fenrg.2017.00034
Renewable energy planners in developing countries should be cautious in using analytical tools formulated in developed countries. Traditional energy consumption, economic and demography transitions, high-income inequality, and informal economy are some characteristics of developing countries that may contradict the assumptions of mainstream, widely used analytical tools. In this study, we synthesize the debate in previous review studies on energy models for developing countries and then extend the scope of the previous studies by highlighting emerging methods of system thinking, life cycle thinking, and decision support analysis. We then discuss how these tools have been used for renewable energy analysis in developing countries and found out that not all studies are aware of the emerging critical issues in developing countries. We offer here a guidance to select the most appropriate analytical tool, mainly when dealing with energy modeling and analysis for developing countries. We also suggest potential future improvements to the analytical tool for renewable energy modeling and analysis in the developing countries.
The need for widespread implementation of renewable energy technologies has become a global challenge as we transition to low carbon and green economy. Around 176 countries have stated their renewable energy targets, driven by various motivations (REN21, 2017). Renewable energy technologies are regarded as one of the pathways to decarbonize the energy sector and to reduce fossil fuel dependency (Dannenberg et al., 2008; Taylor et al., 2014; Ozcan, 2017). Traditionally, renewable energy plays a vital role as an energy source in rural areas of developing countries (Blum et al., 2013; Sovacool, 2013).
However, reaching individual country renewable energy targets comes up against various barriers (Nepal, 2012; Blum et al., 2013; Byrnes et al., 2013; Dulal et al., 2013; Masini and Menichetti, 2013; Sovacool, 2013; Goh et al., 2014; Marquardt, 2014; Zyadin et al., 2014; Erdinc et al., 2015; Luthra et al., 2015) whereby developed and developing countries have responded into different ways. Developed countries pull renewable energy investments by policy instruments, such as feed-in tariff (FIT) and a carbon tax to fossil fuel (Dannenberg et al., 2008). In the contrary, such cost increasing policies cannot be easily implemented in many developing countries, which usually have been overburdened from subsidizing fossil energy (Zyadin et al., 2014). Indonesia is an excellent example of this. Indonesian state-owned Electricity Company, which has been monopolizing the electricity market, rejected the FIT issued by the Indonesian Ministry of Energy and Mineral Resources and eventually, in 2017, the FIT has been replaced by the reference tariff scheme, which has a lower tariff than the oil-based electricity generation cost.
The differences of the energy system between the two country types have consequences when using analytical tools for renewable energy planning. Energy modeling is a standard analytical tool to establish a renewable energy target and policy; however, most energy models are initially designed for developed countries. Adopting energy models, without considering adequate adjustments, may produce bias in analyzing its use in developing countries’ cases. Besides energy system discrepancy, unique economic characteristics of developing countries, in particular—the informal economy, income inequality, and environment protection ability—also have major influences on the results of energy models (Van Ruijven et al., 2008b; Bhattacharyya and Timilsina, 2010a).
Therefore, several studies have attempted to review the most appropriate analytical tool for developing countries but their recommendations did not converge (Meier, 1984; Bhatia, 1987; Shukla, 1995; Pandey, 2002; Shukla et al., 2006; Hiremath et al., 2007; Urban et al., 2007; Van Ruijven et al., 2008b; Bhattacharyya and Timilsina, 2010a,b). Furthermore, most of them only discussed conventional energy models, and none of them examined the applicability of life cycle thinking, systems thinking, and agent-based modeling (ABM) methods. On the other hand, Ventosa et al. (2005) and Veselka et al. (2002) recommend the ABM for solving complex problems that could not be explained by the conventional energy models. Moreover, energy models should have the flexibility to allow model adjustments when an analysis is done in developing countries; and one of the modeling approaches with a high degree of flexibility is ABM (Chappin et al., 2017). Therefore, our study aims to update and extend previous studies by reviewing the more relevant analytical tools. We also attempt to provide clear guidance to select appropriate tools based on the purposes of analysis and features specific to developing countries.
Our paper is structured as follows: Section 2 discusses developing countries’ characteristics, which contradict the features assumed in conventional energy models. It also presents debates on the most appropriate analytical tools while the current debates still comprise on limited tool options. In Section 3, we review common analytical tools for energy systems and their applications in developing countries to understand the internalization of developing countries’ issues on the tools used. We provide recommendations for tool selection for developing countries in Section 4 while Section 5 concludes the analysis and recommendations for future renewable energy modeling work in developing countries.
As mentioned before, most of the previous studies limited their reviews on energy models, which can be categorized into economic-based (top-down) models, engineering-based (bottom-up) models, and hybrid energy models. Descriptions of those models and their applications are further discussed in the next section. In this section, we discuss the rationale of the incompatibility of existing conventional energy models for use in developing countries. Here, we define a developing country as “a country that generally lacks a high degree of industrialization, infrastructure, and other capital investment, sophisticated technology, widespread literacy, and advanced living standards among their populations as a whole” (Nfuka and Rusu, 2009).
In terms of analytical facility supports, Bhattacharyya and Timilsina (2010a,b) and Meier (1984) doubt the availability of computer infrastructure, data, and skilled human resources in a developing country. However, we hold the view that these barriers might not be relevant in recent years and, most importantly, some energy models, such as the Rogeaulito’s model (Benichou and Mayr, 2014), are already designed for easy use by using free and popular spreadsheet software.
In terms of the objective of the analysis, existing energy models aim for low carbon energy supply in developed countries while developing countries have additional concerns, such as energy access equity (Pandey, 2002; Shukla et al., 2006; Van Ruijven et al., 2008b). The issue of energy access calls for an analysis of decentralized energy systems, but most existing energy models do not integrate decentralized and centralized energy analyzes at the same time (Pandey, 2002; Hiremath et al., 2007). Furthermore, Bhatia (1987) and Urban et al. (2007) emphasize the differences between power system performances in the developed and developing countries. The electricity system in developing countries has characteristics of inadequate power supply, unreliable power plants, insufficient maintenance level, higher technical and non-technical losses, and subsidized electricity price. The characteristics are different from market-based and abundant energy supply in a developed country.
In terms of the economic nature, the informal economy is a significant business activity in most developing countries, though it is not recorded as part of the gross domestic product (GDP). Energy models usually use GDP as one of the drivers for energy demands and, therefore, the inclusion of informal economy to GDP will change the parameter values in the energy models significantly. Compared to developed countries, income inequality in developing countries is higher; however, most existing energy models have neglected it by simply using the average income (Van Ruijven et al., 2008b; Bhattacharyya and Timilsina, 2010b). As a result, those energy models underestimate energy demand behavior of high-income households. Moreover, environmental analysis has been a standard feature in current energy models, though such models have also overlooked the financial ability and willingness of developing countries to implement recommended clean energy technologies, which usually imported at high costs (Pandey, 2002). Developing countries are also experiencing dynamic transitions, which are not entirely featured in existing energy models. These transitions include a shift from the traditional energy to modern energy system, rapid urbanization, industrialization, energy market transformation from monopoly to liberal market, and an increase of energy consumption intensity (Bhatia, 1987; Shukla, 1995; Pandey, 2002; Shukla et al., 2006; Urban et al., 2007; Van Ruijven et al., 2008b; Bhattacharyya and Timilsina, 2010a).
The fundamental differences between the two economies may preclude the adoption of existing energy models since most models that have been used are replications of energy systems in developed countries. Thus, deciding the most appropriate energy models for developing countries has been debated for decades. Bhatia (1987) and Urban et al. (2007) argue that most of the top-down approaches are not suitable for developing countries due to contradictive economic assumptions, such as market behavior, income distribution, informal economy, traditional energy, and most importantly continuous dynamic transition. Among the bottom-up approaches, Urban et al. (2007) suggest that a simulation model is more suitable for developing countries because the model does not assume optimal consumer behavior and the perfect market as compared to the optimization approaches do. The markets in developing countries are imperfect due to non-market-based economies and inadequate electricity supply. Moreover, the presence of rural households without access to modern energy does not represent optimal consumer behavior. However, the downside is that simulation models require intensive data and advanced skills, which are limited in developing countries. In this light, Bhattacharyya and Timilsina (2010a,b) recommend more simple accounting-based simulation models, such as Long-range Energy Alternatives Planning System (LEAP).
Moreover, Meier (1984) advises the top-down approaches, such as econometric, input-output analysis, and hybrid energy models, as well as the bottom-up approaches for applications in developing countries. Similarly, Shukla (1995) argues that adjusted top-down approaches should be complementary, especially to validate projections from the bottom-up models, which usually depend on exogenous energy demand assumptions. Meier (1984) and Pandey (2002) proclaim that all energy model approaches with adjustments will be suitable for developing countries’ characteristics. This argument is supported by Van Ruijven et al. (2008b), who conclude that six global top-down and hybrid models provide consistent results with economic theories for Asia’s developing countries though the models do not consider any critical issues faced by developing countries. On the other hand, Urban et al. (2007) encourage developing countries to make their specific energy models by modifying existing energy models.
While the debates continue and are more focused on energy models, Hiremath et al. (2007) and Pandey (2002) also review decision support analysis and, slightly, system dynamics (SD). Hiremath et al. (2007) discuss two SD-based studies for the Bangladesh case while Pandey (2002) only explains an SD study in the UK. Therefore, in the following section, we fill the gap by also reviewing system thinking, decision support analysis, and life cycle thinking approaches. These emerging methods have been commonly used for renewable energy analysis in developed countries (Hertwich et al., 2015; Rai and Robinson, 2015; Ishizaka et al., 2016). The reviews of each tool include definitions and several applications to assess on how they can incorporate developing countries’ characteristics.
A bottom-up energy modeling is an engineering approach that features a comprehensive technical database related to power plant technology, costs, and electricity demand patterns. Several applications of bottom-up models are for optimal expansion and operation of energy systems (Pfenninger et al., 2014), forecasting tools (Jebaraj and Iniyan, 2006; Suganthi and Samuel, 2012), energy market analysis (Ventosa et al., 2005; Foley et al., 2010; Möst and Keles, 2010) and clean energy system analysis (Bazmi and Zahedi, 2011).
The optimization model is mostly adopted to find the minimum electricity generation costs for given constraints, such as electricity demand, peak load, and resource availability. Examples of these optimization models are Energy Flow Optimization Model (EFOM), MARKet Allocation (MARKAL), Integrated MARKAL-EFOM System (TIMES), Model for Energy Supply Strategy Alternatives and their General Environmental Impact (MESSAGE), Wien Automatic System Planning Package (WASP), Open Source Energy Modeling System/(OSeMOSYS), and various optimization techniques, such as Linear Programming (LP), Mixed integer LP (MILP), and Mixed Objective LP (MOLP) (Banos et al., 2011; Bhandari et al., 2015). LP is a classic optimization technique commonly used for energy modeling in developing countries due to its simplicity. Some of its applications in developing countries are for analyzing renewable energy systems in rural areas (Ramakumar et al., 1986; Chauhan and Saini, 2015; Bhandari et al., 2016) and in the interconnected grid (Dudhani et al., 2006); and for optimizing biodiesel production (Leduc et al., 2009). Afful-Dadzie et al. (2017) employ MILP to optimize power plant capacity expansion in Ghana by considering budget limitation, which is a common problem in developing countries. Ramakumar et al. (1986) use LP to model firewood and solar stove in addition to modern renewable energy technologies, which include the solar photovoltaic (PV), wind, and hydropower technologies. On the other hand, WASP is a commercial optimization model, which focuses on engineering issues, so WASP studies usually ignore socio-economic problems in developing countries. For example, Chathuranga et al. (2016) focus on wind energy uncertainty in power plant planning in Sri Lanka while Hainoun et al. (2015) emphasize fuel availability to analyze optimal power plant expansion in Syria. A similar problem is found in EFOM application, Daniel et al. (2009) design a cost minimizing energy system for Tamil Nadu state – India by considering several constraints. However, the only analyzed issue on developing countries is the agriculture sector, which also exists in a developed country.
Most commercialized optimization models, such as MESSAGE, OSeMOSYS, and MARKAL, have been widely used for energy market and energy system analysis, including in the developing country setting. MESSAGE can reckon urban and rural dissimilarities such as the differences in income, infrastructure, and energy consumption pattern (Krey et al., 2012), though not all MESSAGE studies have modeled developing country issues (Liu et al., 2009; de Oliveira et al., 2016). Nerini et al. (2015) use OSeMOSYS to design an optimal rural electrification program in Timor-Leste by considering various traditional energies, such as open firewood and kerosene stoves. Most importantly, OSeMOSYS is an open-source energy model that is suitable for developing countries that are avoiding costly models. MARKAL-based studies already discuss more features of developing countries, such as fuel switching from traditional biomass to commercial energy, and also has urban and rural settings (Shakya et al., 2012; Yangka and Diesendorf, 2016).
Bottom-up models for forecasting purposes can have both narrow and broad analytical scope. Artificial Neural Network (ANN) has been used to predict the fluctuation of renewable energy productions, such as solar (Almaktar et al., 2015) and the wind (Ramasamy et al., 2015). Other common learning algorithms are Extreme Learning Machine (Golestaneh et al., 2016) and gray prediction model (Tsai et al., 2017). For broader applications, forecasting analysis typically uses social and economic data when integrating into optimization and energy system analysis. For example, Azadeh et al. (2013) apply ANN to set optimal renewables in Iran by considering environmental and economic factors, which are CO2, CO, NOx, energy prices, and GDP. In the meantime, Yu et al. (2012) use Particle Swam Optimization and Genetic Algorithm Optimal Energy Demand Forecasting (PSO-GA EDE) to estimate the impacts of urbanization, along with other factors, to forecast future energy demands in China.
Another type of bottom-up approach is the simulation modeling, which can be used for forecasting and energy system analysis. One of the well-known simulation models is LEAP, which provides energy system evolution scenarios instead of a single optimum path (Després et al., 2015). Another energy scenario model is of Rogeaulito, which forecast energy supply and demand separately in order to identify potential energy crises (Benichou and Mayr, 2014). Rogeaulito is claimed to solve the weakness of most conventional models, which only forecast energy demand (or supply) and assume that the forecast result will be automatically matched by supply (or demand) through energy market mechanism. As a global energy model, the analysis scope of Rogeaulito covers developing countries and biomass uses (Benichou et al., 2013); however, no study has applied Rogeaulito for analysis in developing country. On the other hand, Daioglou et al. (2012) and van Ruijven et al. (2011) establish simulation bottom-up energy models for developing countries. They differentiate the households based on rural–urban area and income quintiles. Rural households consume most energy for cooking while urban households consume significant amounts of energy for appliances and space cooling. Moreover, the low-income families are characterized to have a higher discount rate representing lower financing capability, and beyond the income issues, the families are assumed to have perceived costs, which are based on habit, convenience, culture, and other non-monetary factors, which eventually influence energy uses. Other discussed features of developing countries include the shift from traditional to modern energies and urbanization. The model then simulates the impacts of the carbon tax, rural electrification, and income distribution changes to energy consumptions, emissions, and energy market share.
Nevertheless, many studies have still used an accounting-based approach just for simplicity and data availability reasons without further characterizing the issues faced by developing countries. For example, Huang et al. (2011) utilize LEAP to simulate several scenarios for Taiwan’s energy demand but the only issue being modeled for developing countries is the presence of the agriculture sector in the model. Similarly, McPherson and Karney (2014), comparing the scenario-based projections of Panama’s electricity supply, use LEAP because of its simplicity and ready-to-use characteristics.
The bottom-up approaches ignore macroeconomic interactions by assuming exogenous energy prices, demand, and other economic theory-related inputs (Li et al., 2015). In the contrary, the second category—economic-based approaches—or the so-called top-down approaches endogenize energy demand to other macro and microeconomic variables. Top-down approaches, such as computable general equilibrium (CGE), econometrics and input–output (I-O) analysis, are praised for their consistency with prevailing economic theories and empirical data.
With these features, top-down approaches are useful for analyzing the economic impacts of an energy policy (Wianwiwat and Asafu-Adjaye, 2011; Nguyen, 2012) and energy crisis (Vasconcelos and Carpio, 2015). Dai et al. (2016) use CGE to estimate economic and employment growths from renewable energy addition in China. However, by using time series analysis, Zhao and Luo (2017) reject the hypothesis of renewable energy as a job creator in China. Wesseh and Lin (2016), estimating translog production model, find out that renewable energy use in African countries has higher economic impacts than fossil fuels.
The complexity of renewable energy systems calls for a systems science-based understanding and approach, such as system dynamics (SD) and ABM (Möst and Keles, 2010; Nakata et al., 2011; Pfenninger et al., 2014). SD could be defined as a methodology to visualize, learn, manage, and communicate complex systems rigorously (Maani, 2009; Kelly et al., 2013). SD includes identification of problems, primary variables, and interactions between variables; model validation; sensitivity analysis, and scenario simulations.
System dynamics has the flexibility to have properties of the bottom-up approach, top-down approach or both. Ahmad and bin Mat Tahar (2014) perform a SD analysis to assess renewable energy targets in Malaysia by considering decision process, planning, construction and operational capacity without further considering specific issues of developing countries. Hsu (2012), in assessing the effectiveness of PV policy in Taiwan, narrow the analysis to financial problems such as cost, incentives, and learning rate. In analyzing Colombia biodiesel market, Espinoza et al. (2017) discuss political, environmental, social, economic, technology, and especially social issues of energy and food security. Nevertheless, most of those studies still have not incorporated developing countries’ characteristics in their analyses (Hsu, 2012; Ahmad and Tahar, 2014; Espinoza et al., 2017).
Another well-known model for systems approach is ABM, which is a dynamic model consisting of agents with an ability for learning, adaptive capacity, heterogeneity, autonomy, local interaction, bounded rationality, and non-equilibrium dynamic characteristics (Fagiolo et al., 2007; Ringler et al., 2016). ABM also could possess bottom-up and top-down features at the same time (Ehlen and Scholand, 2005; Gerst et al., 2013). Therefore, ABM could model the heterogeneous agents from different approaches, for example, microeconomic approach for profit maximization problem, space theory for site selection problem, evolutionary programming for adaptive process problem, and experience-based decision models (An, 2012). These features allow modeling of how the different incomes of rural and urban households can influence energy systems, as pointed out by Meier (1984), Pandey (2002), Urban et al. (2007), and Van Ruijven et al. (2008b) when analyzing rural electrification.
With regard to ABM applications, Smajgl and Bohensky (2013) estimate the impacts of fuel subsidy policy to poverty and deforestation patterns in East Kalimantan, Indonesia. Other issues considered include traditional energy, informal economy and rural-urban households’ characteristics (e.g., income and education profiles). The model is based on survey data that are scaled-up to the provincial average. Alfaro et al. (2017) apply ABM for analyzing rural electrification in Liberia by considering issues of job creation and income generation. Tang (2013) simulates the significance of CDM scheme for wind turbine investment in Brazil, China, and India.
Another tool used to understand the complexity of energy social system is decision support analysis, such as multi-criteria decision analysis (MCDA) (Pohekar and Ramachandran, 2004). MCDA usually extracts opinions from multidisciplinary stakeholders in analyzing energy issues. The most commonly used MCDA families are the analytical hierarchy process (AHP), preference ranking organization method for enrichment evaluation (PROMETHEE), and the elimination and choice translating reality (ELECTRE) (Pohekar and Ramachandran, 2004). AHP organizes a complex system into the main objective at the top, criteria in the level, and sub-criteria in the sub-levels of hierarchy. By using underlying information and stakeholders’ judgments, elements at each level are then weighted to calculate priorities of each decision alternative. Similarly, PROMETHEE is a simple ranking method to rank alternative actions from the best to the worst, and the rank is arranged by using weights and preference functions (Pohekar and Ramachandran, 2004; Behzadian et al., 2010). On the other hand, ELECTRE uses binary outranking relations to identify and eliminate alternatives which are unacceptable (Pohekar and Ramachandran, 2004; Vahdani et al., 2013). Compared to AHP, PROMETHEE, and ELECTRE have better performance to manage imprecise information due to the utilization of thresholds and probability distributions (Cinelli et al., 2014).
Multi-criteria decision analysis studies generally search for the optimal option in multi-perspectives and, therefore, could consider criteria from both bottom-up and top-down perspectives. For example, Ahmad and Tahar (2014) employ AHP analysis when concluding that solar energy is the best renewable energy to be developed in Malaysia. Their study involves bottom-up data, such as investment costs, emission, efficiency, and the land requirement for renewable energy technologies, as well as top-down data, for example, job creation. However, Ahmad and Tahar (2014) do not further account the unique characteristics of developing countries, and all of the data are obtained from the US and other developed nations. By contrast, Sovacool (2013) undertakes qualitative factor analysis to assess success and failure factors for renewable energy in rural areas of 10 Asia-Pacific developing countries. The study encompasses primary data from 441 interviews, 90 site visits, and discussions with 800 local community members.
Life cycle thinking methods are systemic tools to compare the characteristics of renewable energy technologies from cradle to grave in a comprehensive manner. For example, life cycle costing (LCC) estimates all direct costs of a technology while environmental life cycle analysis (LCA) has a similar purpose but focuses on the estimation of environmental impacts, instead of the monetary values. In a triple dimensional analysis of sustainability, social LCA (SLCA) is accounted with the other two (LCA and LCC) when analyzing the sustainability impacts of technology utilizations in the society. For example, Manik et al. (2013) do a SLCA analysis to estimate the social effects of palm oil-based biodiesel industry in Indonesia. Their analysis covers 24 criteria and weighted the criteria by interviewing workers, local community, society, growers, transporter, and mill owners in the palm-oil biodiesel supply chain.
The integration of LCC, LCA, SLCA, and other tools, such as MCDA, SD, and ABM, will result in life cycle sustainability assessment for the analysis of the complexity of emerging sustainable systems. However, these methods are challenged by data availability as a result of detailed calculations in each phase of technology lifetime (Halog and Manik, 2011). Therefore, in terms of data requirement, life cycle thinking demands more detailed technical data than the bottom-up model. As a consequence, the results of life cycle studies become input data for previously discussed tools, for example, life cycle emission in a MCDA study by Ahmad and Tahar (2014). Not only as a data-generating tool but the life cycle thinking approach is also commonly combined with other methods to form hybrid energy analytical tools.
Each discussed analytical tool (as explained above) has advantages and disadvantages and, therefore, using a single analytical tool is not adequate when analyzing a complex system such as energy system. As shown in Table 1, bottom-up models have detailed specifications of energy demand, but the demand is usually exogenous without interactions to energy prices, income, and other factors. On the other hand, top-down approaches typically have a high aggregated energy sector (Urban et al., 2007; Herbst et al., 2012; Li et al., 2015). Aggregating all of the highly diverse power plant technologies into a single electricity sector will produce inaccurate results (de Koning et al., 2015).
Therefore, developing hybrid approaches is warranted to solve the weaknesses of the bottom-up and top-down approaches (Nakata et al., 2011). The integration could explore three alternative strategies. First, exogenous electricity demand in bottom-up approaches is modified into endogenous variables (Murphy et al., 2007; Strachan and Kannan, 2008; Murphy and Jaccard, 2011; Sarica and Tyner, 2013). Second, a hybrid energy model could disaggregate the energy sector in the top-down data into several specific energy technologies (McFarland et al., 2004; Wing, 2006; Wing and Joint Program on the Science and Policy of Global Change MIT, 2008; Dai et al., 2011). Third, one of the energy models used data resulting from other independent models (Koopmans and te Velde, 2001; Giraudet et al., 2012).
An example of the first type of hybrid energy model for developing countries is provided by Meier and Mubayi (1983), who modify a highly complex LP model from developed countries into a simpler, but a comprehensive model by reducing energy end-use sector details. Basically, Meier and Mubayi (1983) set iterative interactions between reference energy system (RES) and I-O table to update energy demand and economic output. To capture issues in developing countries, Meier and Mubayi (1983) stipulate the upper and lower bounds on variables to consider non-industry-based energy demands and transition from traditional to commercial energy utilization, including renewable energy. MARKAL–MACRO is another example where MARKAL as a bottom-up approach provides energy demand projection to MACRO as a top-down model to estimate the energy costs. The costs are then inputted back to the MARKAL to refine the energy demand projection (Ko et al., 2010). Chen (2005) and Ko et al. (2010) run MARKAL–MACRO for assessing the renewable energy share in future energy consumptions of China and Taiwan, respectively. Compared to Ko et al. (2010), Chen (2005) can relatively capture the developing countries’ characteristics by dividing energy demand into agriculture sector, urban, and rural households.
The second type of hybrid energy model attempts to disaggregate the energy sector into several energy technologies. In a CGE model for Thailand, Wianwiwat and Asafu-Adjaye (2011) not only model traditional biomass energy but also further divide it to bagasse, firewood, paddy husk, crop residues and paper production residues. However, due to issue of data availability, elasticity parameters for these energies are obtained from the Global Trade Analysis Project (GTAP) 6 database.
In the third type of hybrid energy models, Extended Snapshot model combines I-O analysis estimating new economic outputs, and bottom-up model processing the outputs into new energy demand and emissions (Hak et al., 2017). In its application, Hak et al. (2017) consider the family size of urban and rural households when estimating emission reduction from renewable energy development in Cambodia. Tomaschek et al. (2016) analyze four renewable energy policy scenarios in South Africa by combining three analytical tools, which are TIMES—Gauteng Energy and Emissions Cost Optimisation (TIMES-GEECO), Transport Emissions Modelling Tool and Geographic Information System (GIS). Tomaschek et al. (2016) also reckon various characteristics of developing countries, such as different road characteristics in rural and urban areas, electrification ratio, income distribution, economic and employment transitions from primary sector to service sector. Anger et al. (2016) use Energy-Environment-Economy Model at the Global (E3MG) and Chemistry-Transport model and Atmospheric Chemistry model (CTM pTOMCAT) to evaluate the influence of renewable energy target to emission reduction in China.
Nevertheless, as Anger et al. (2016)’s drawbacks, several hybrid energy models do not further include any key developing countries’ issues (Cai et al., 2011; Dai et al., 2011; Duan et al., 2014; Rivera et al., 2015; Bosello et al., 2016). For examples, in an I-O analysis, Cai et al. (2011) disaggregate the electricity sector into nine power plant types to estimate green economy and green jobs created from renewable energy in China. Dai et al. (2011) analyze the impacts of carbon intensity reduction scenarios to renewable development in China by dividing the energy sector in hybrid AIM/CGE model into seven sub energy sectors and 12 power plant technologies. Similarly, Duan et al. (2014) develop CE3METL (Chinese Energy- Economy-Environment Model with Endogenous Technological change by employing Logistic curves) in assessing optimal renewable energy policy in China. Rivera et al. (2015) evaluate the transition pathway to low-carbon energy system in Mexico by disaggregating electricity sectors into five renewable energy power plants and four fossil energy power plants in the ThreeME (Multi-sector Macroeconomic Model for the Evaluation of Environmental and Energy policy) model. Bosello et al. (2016) integrate Intertemporal Computable Equilibrium System and World Induced Technical Change Hybrid models to estimate the impacts of four climate change strategy scenarios for renewable energy consumptions in Southeast Asia countries.
Common practices to analyze characteristics of developing countries are to use the characteristics directly, for example firewood use, as one of the variables in the model (Wianwiwat and Asafu-Adjaye, 2011) or to translate the characteristics into scenarios. Shukla et al. (2006) convert transitions of the population, GDP, urbanization, land-use patterns, and structural changes in agriculture and livestock sectors into four emission scenarios. The scenarios are then simulated on Edmonds–Reilly–Barns (ERB) model for analyzing renewable energy growth in India. The ERB model eventually is renamed GCAM, an open source license model, which had been used to investigate energy demand in the building sector (Chaturvedi et al., 2014) and the effectiveness of renewable energy policy (Shukla and Chaturvedi, 2012) in India. Chaturvedi et al. (2014) directly count developing countries’ issues by modeling rural and urban differences and traditional biomass consumption in assessing long-term path of energy consumption in the building sector. Moreover, Shukla and Chaturvedi (2012) estimate renewable energy generation from policy scenarios, which consider transitions in technology development and international cooperation.
Hybrid energy models are not only integrating top-down and bottom-up approaches but also other analytical tools. Those tools are commonly used to generate inputs, which replace exogenous scenarios in bottom-up models. Bala (1997) works on SD to forecast population, animal and wood resources and then the forecasting results are inputted to LEAP for estimating energy demands, including firewood, animal waste, and crop residues in Bangladesh households. Similarly, Robalino-López et al. (2014) employ the Hodrick–Prescott (HP) filter to generate smooth time series data while SD then uses the data to investigate emission changes from renewables growth in Ecuador. Van Ruijven et al. (2008a) use the system dynamic TIMER to evaluate the impacts of hydrogen energy uses in India and Western European countries. Though TIMER initially has various developing countries’ features (De Vries et al., 2001), Van Ruijven et al. (2008a) do not further discuss how to incorporate these features into the model.
In a hybrid decision support analysis, Kabak and Dağdeviren (2014) combine the Benefits, Opportunities, Costs, and Risks and Analytic Network Process (ANP) to select the prioritized renewable energy technology in Turkey. The ANP involves 19 criteria whose weights are determined by eight national experts and conclude that, as Kabak and Dağdeviren (2014) suggested, hydropower as the most important renewable energy. Rahman et al. (2016) integrate Stochastic Multicriteria Acceptability Analysis and an accounting-based simulation model to propose biomass as the prioritized energy in Bangladesh. Several of the criteria used represented the characteristics of developing countries, for example, availability of local skill and resources. Tahri et al. (2015) carry out integrated GIS—AHP analysis to determine a specific location for a 500 MW solar farm in Morocco. Weights of criteria, such as location, orography, land use, and climate, are derived from a group of experts.
In a hybrid life cycle thinking, Varun et al. (2010) use a hybrid IO—LCA model to estimate total energy uses and emission production from several hydropower plants in India. Nevertheless, Varun et al. (2010) exclude specific issues of developing countries in their model and indeed, use the US data due to similar production costs in the steel industry in India and the US. Kursun et al. (2015) design a renewables-based rural electrification system in India by using three integrated tools, which are MOLP, LCA, and emergy analysis. The MOLP has multiple objectives, whose parameters derived from LCA (i.e., minimum land use, water use and global warming potential) and emergy analysis (i.e., minimum environmental loading ratio, maximum renewability, and maximum emergy yield ratio).
The above-discussed tools are just a few of a wide range of analytical tools in the energy sector with potential applications to developing country needs. More detailed descriptions of energy models are reviewed by Bhowmik et al. (2017), Calvin et al. (2012), Chen et al. (2016), Connolly et al. (2010), Després et al. (2015), Foley et al. (2010), Pfenninger et al. (2014), Suganthi and Samuel (2012), and Weijermars et al. (2012).
The selection of the most appropriate analytical tool should consider the purpose of the analysis (Kelly et al., 2013; Cinelli et al., 2014). Therefore, we synthesize possible tools for developing countries based on the purposes of the analysis and the issues in the developing countries as shown in Table 2. Developing countries tend to minimize total energy costs, which could be estimated by using optimization model and total LCC, even though MCDA and LCA could also suggest the most favorable energy supply by considering other factors. By contrast, those tools are inappropriate for analyzing the macroeconomic impacts of a clean energy policy. The capability to quantitatively measure environmental impacts of a policy is a standard feature of energy analytical tools except for MCDA, which commonly uses expert opinions. Meanwhile, seeking alternative energy transition pathways could benefit from the bottom-up approaches and system thinking. One of the modeling benefits is to have knowledge and insights on how the tools can be used (DeCarolis et al., 2012). Each analytical tool will give a new understanding of energy system, except the top-down approaches, which have less energy system specification. Moreover, system thinking provides more knowledge by structurally determining the relationships of influencing factors in the complex energy system.
The reviewed tools can incorporate most of the key developing countries’ issues, especially traditional way of using energy as well as availability of free or inexpensive software. Nevertheless, the top-down approaches are not suitable for rural electrification analysis because they are usually applied at the national level. Moreover, the approaches rely on the variable of per capita electricity demands, which is incorrectly measured by ignoring unequal electricity access. In this respect, developing countries should use the number of electricity customers instead of using the per capita variable. On the other hand, bottom-up approaches and SD are not suitable for assessing the impacts of income inequality to the energy system because they usually use a homogenous energy consumer in the analysis. Most of the tools could also analyze affordability of renewable energy policy, for example, by using a budget constraint in the bottom-up approaches, the cost criteria in MCDA, and the different incomes in ABM. Hybrid energy tools surely can solve the weakness of each approach but integrating multiple approaches will be hampered by data availability and modeling ability. Similarly, life cycle thinking needs more rigorous data, ranging from energy extraction data to power plant decommissioning data.
As Van Ruijven et al. (2008b) conclude, we hold the view that most of the studies across all tool types cannot consider the informal economy in their tools. Shukla et al. (2006) discuss the informal economy issue in their analysis but they then translate it into a scenario rather than changing the structure of the energy model. Ignoring informal economy would have a significant adverse impact on the top-down approaches, which mainly use per GDP variables. MCDA potentially consider the issue, but it will be challenged by the availability of data and experts who could link the interaction of the informal economy and energy sector. As a suggestion, energy analytical tool should adopt the multiple-indicators multiple-causes (MIMIC) and dynamic MIMIC (DYMIMIC) methods which can be used to assess the size of the informal economy (Karaca, 2016; Davidescu, 2017).
Beyond developing countries’ issues as shown in Table 2, another emerging problem is energy market transition from monopoly to liberal energy market. The number of emerging economies liberalizing their electricity market is growing, and such action should cautiously consider the negative impact of electricity price increase (Nepal and Jamasb, 2015). Though the bottom-up approaches may simulate liberal energy market, the conventional energy models cannot capture learning effect, asymmetric information, imperfect competition, and strategic interaction including collusions between companies in the market (Sensfuß et al., 2007; Weidlich and Veit, 2008). In this case, ABM is preferable because it could simulate strategic behaviors of electricity companies especially for pricing analysis (Koesrindartoto et al., 2005).
Agent-based modeling is also a powerful tool to analyze renewable energy adoption in households. Rai and Robinson (2015) provide a useful ABM example to be adopted by developing countries. Their ABM is based on survey data of social, economic, and environmental factors to characterize household behavior. Households will invest in rooftop PV by considering a rebate scheme, their values to the environment and their social network. Rai and Robinson (2015) deduce that the economic feasibility alone is sufficient to explain investment behaviors. However, Alfaro et al. (2017) contend that ABM should be a complementary analytical tool instead of a substitute for an integrated power plant planning system.
Though the renewable energy targets for developing countries have increased, these countries seem to have limited renewable energy policy options. They are not able to automatically adopt the global renewable energy policy since they have different characteristics from developed countries. Consequently, analyzing inherent characteristics of developing countries is necessary to formulate effective and efficient energy policy but, unfortunately, most energy analytical tools do not incorporate those characteristics.
This problem has been recognized and debated by several published review studies. Bhatia (1987), Bhattacharyya and Timilsina (2010a,b), Hiremath et al. (2007), and Urban et al. (2007) do not recommend economic approach-based energy models because the economic assumptions used are different from conditions in developing countries. Instead, they advocate the use of engineering approach-based models, which have detailed descriptions of energy systems. By contrast, Meier (1984), Pandey (2002), Shukla (1995), and Van Ruijven et al. (2008b) advise that the top-down models still can be used by modifying the model’s assumptions to developing country’s characteristics. Nevertheless, we found out that those studies have limited discussions on decision support analysis, life cycle thinking, and system thinking approaches. Our review shows that these new analytical tools have been widely used for analyzing renewable energy systems in developing countries. In fact, integrating the new tools and conventional energy modeling will improve the robustness of results when conducting energy systems modeling and analysis.
We also believe that all analytical tools could be applied for analysis in the case of developing countries, as long as adjustments are made accordingly. Most importantly, tools selection should consider the objective and the scope of the study. For that, we provide a guideline to select the appropriate analytical tool based on analysis purpose and developing country issues. As an example, for forecasting purposes, the accounting-based bottom-up model is suitable to simulate dynamic transition in developing countries and, on the other hand, top-down approaches are the most suitable tools for fiscal policy analysis. Moreover, analyzing rural household participation in renewable energy development could employ ABM while selecting prioritized renewable energy could benefit from decision support analysis. Most analytical tools can take into account developing countries issues. Common practices to adjust the energy model use in developing countries are by disaggregating energy sector data in top-down approaches and by disaggregating single household sector into poor-rich households. However, only a few studies can consider the informal economy, whose data are difficult to measure. Therefore, energy policy analysis in developing countries is being challenged to quantity the informal economy, which has caused bias GDP variables on the energy models.
For modeling recommendations, developing countries should develop hybrid ABM, combining four perspectives, which are engineering, economic, social, and environmental issues. ABM is an excellent modeling platform, which can generate heterogeneous agents in the simulation. In conducting social analysis, the agents could represent households with differences in income, electricity access, and location; while in engineering perspectives, the agents could be power plants with differences in cost, emission, and capacity factor. The hybrid ABM should also identify optimal policies, which consider not only generation costs and environmental constraints but also macroeconomic impacts and social acceptance. The proposed model should cover both on-grid and off-grid renewable energy systems at the same time to understand the interactions of two different renewable energy markets. Moreover, for general energy modeling in developing countries, we suggest paying attention to the number of electricity customers. This indicator is necessary to measure the impacts of economic structure shifting or rural electrification program to the electricity demand of a nation. This indicator has been neglected in most existing electricity demand estimations (Adom et al., 2012; Arisoy and Ozturk, 2014; Atalla and Hunt, 2016).
All authors have significantly contributed to the article. AH is the leader of the research group and the main supervisor who gives the final approval of what to be published. MI is a Research Higher Degree student who analyzed data and drafted the article as part of his PhD project. RN and DK give guidance and critical revisions of the article.
The authors declare that the research was conducted in the absence of any commercial or financial relationships that could be construed as a potential conflict of interest.
The authors acknowledge the funding support from the Indonesia Endowment Fund for Education (LPDP), Ministry of Finance—the Republic of Indonesia (Grant no: 20141122092191); and the research grant from the School of Earth and Environmental Sciences at the University of Queensland.
Adom, P. K., Bekoe, W., and Akoena, S. K. K. (2012). Modelling aggregate domestic electricity demand in Ghana: an autoregressive distributed lag bounds cointegration approach. Energy Policy 42, 530–537. doi: 10.1016/j.enpol.2011.12.019
Afful-Dadzie, A., Afful-Dadzie, E., Awudu, I., and Banuro, J. K. (2017). Power generation capacity planning under budget constraint in developing countries. Appl. Energy 188, 71–82. doi:10.1016/j.apenergy.2016.11.090
Ahmad, S., and bin Mat Tahar, R. (2014). Using system dynamics to evaluate renewable electricity development in Malaysia. Kybernetes 43, 24–39. doi:10.1108/K-10-2012-0092
Ahmad, S., and Tahar, R. M. (2014). Selection of renewable energy sources for sustainable development of electricity generation system using analytic hierarchy process: a case of Malaysia. Renew. Energy 63, 458–466. doi:10.1016/j.renene.2013.10.001
Alfaro, J. F., Miller, S., Johnson, J. X., and Riolo, R. R. (2017). Improving rural electricity system planning: an agent-based model for stakeholder engagement and decision making. Energy Policy 101, 317–331. doi:10.1016/j.enpol.2016.10.020
Almaktar, M., Abdul Rahman, H., Hassan, M. Y., and Saeh, I. (2015). Artificial neural network-based photovoltaic module temperature estimation for tropical climate of Malaysia and its impact on photovoltaic system energy yield. Prog. Photovoltaics Res. Appl. 23, 302–318. doi:10.1002/pip.2424
An, L. (2012). Modeling human decisions in coupled human and natural systems: review of agent-based models. Ecol. Model. 229, 25–36. doi:10.1016/j.ecolmodel.2011.07.010
Anger, A., Dessens, O., Xi, F., Barker, T., and Wu, R. (2016). China’s air pollution reduction efforts may result in an increase in surface ozone levels in highly polluted areas. Ambio 45, 254–265. doi:10.1007/s13280-015-0700-6
Arisoy, I., and Ozturk, I. (2014). Estimating industrial and residential electricity demand in Turkey: a time varying parameter approach. Energy 66, 959–964. doi:10.1016/j.energy.2014.01.016
Atalla, T. N., and Hunt, L. C. (2016). Modelling residential electricity demand in the GCC countries. Energy Econ. 59, 149–158. doi:10.1016/j.eneco.2016.07.027
Azadeh, A., Babazadeh, R., and Asadzadeh, S. (2013). Optimum estimation and forecasting of renewable energy consumption by artificial neural networks. Renew. Sustain. Energ. Rev. 27, 605–612. doi:10.1016/j.rser.2013.07.007
Bala, B. (1997). Computer modelling of the rural energy system and of CO2 emissions for Bangladesh. Energy 22, 999–1003. doi:10.1016/S0360-5442(97)00025-X
Banos, R., Manzano-Agugliaro, F., Montoya, F., Gil, C., Alcayde, A., and Gómez, J. (2011). Optimization methods applied to renewable and sustainable energy: a review. Renew. Sustain. Energ. Rev. 15, 1753–1766. doi:10.1016/j.rser.2010.12.008
Bazmi, A. A., and Zahedi, G. (2011). Sustainable energy systems: role of optimization modeling techniques in power generation and supply – a review. Renew. Sustain. Energ. Rev. 15, 3480–3500. doi:10.1016/j.rser.2011.05.003
Behzadian, M., Kazemzadeh, R. B., Albadvi, A., and Aghdasi, M. (2010). PROMETHEE: a comprehensive literature review on methodologies and applications. Eur. J. Oper. Res. 200, 198–215. doi:10.1016/j.ejor.2009.01.021
Benichou, L., and Mayr, S. (2014). Rogeaulito: a world energy scenario modeling tool for transparent energy system thinking. Front. Energy Res. 1, 1–11. doi:10.3389/fenrg.2013.00013
Benichou, L., Praz, B., Hajjar, J., Le Treut, G., Meillan, M.-P., Waterhouse, S., et al. (2013). Rogeaulito – Transparent Energy Scenario Thinking. Technical Description of Rogeaulito Model and Framework. Paris, France: The Shift Project.
Berglund, C., and Söderholm, P. (2006). Modeling technical change in energy system analysis: analyzing the introduction of learning-by-doing in bottom-up energy models. Energy Policy 34, 1344–1356. doi:10.1016/j.enpol.2004.09.002
Bhandari, B., Ahn, S.-H., and Ahn, T.-B. (2016). Optimization of hybrid renewable energy power system for remote installations: case studies for mountain and island. Int. J. Precis. Eng. Manuf. 17, 815–822. doi:10.1007/s12541-016-0100-2
Bhandari, B., Lee, K.-T., Lee, G.-Y., Cho, Y.-M., and Ahn, S.-H. (2015). Optimization of hybrid renewable energy power systems: a review. Int. J. Precis. Eng. Manuf. Green Technol. 2, 99–112. doi:10.1007/s40684-015-0013-z
Bhatia, R. (1987). Energy demand analysis in developing countries: a review. Energy J. 8, 1–33. doi:10.5547/ISSN0195-6574-EJ-Vol8-NoSI-2
Bhattacharyya, S. C., and Timilsina, G. R. (2010a). Modelling energy demand of developing countries: are the specific features adequately captured? Energy Policy 38, 1979–1990. doi:10.1016/j.enpol.2009.11.079
Bhattacharyya, S. C., and Timilsina, G. R. (2010b). A review of energy system models. Int. J. Energy Sect. Manag. 4, 494–518. doi:10.1108/17506221011092742
Bhowmik, C., Bhowmik, S., Ray, A., and Pandey, K. M. (2017). Optimal green energy planning for sustainable development: a review. Renew. Sustain. Energ. Rev. 71, 796–813. doi:10.1016/j.rser.2016.12.105
Blum, N. U., Wakeling, R. S., and Schmidt, T. S. (2013). Rural electrification through village grids – assessing the cost competitiveness of isolated renewable energy technologies in Indonesia. Renew. Sustain. Energ. Rev. 22, 482–496. doi:10.1016/j.rser.2013.01.049
Böhringer, C. (1998). The synthesis of bottom-up and top-down in energy policy modeling. Energy Econ. 20, 233–248. doi:10.1016/S0140-9883(97)00015-7
Böhringer, C., and Rutherford, T. F. (2008). Combining bottom-up and top-down. Energy Econ. 30, 574–596. doi:10.1016/j.eneco.2007.03.004
Bosello, F., Marangoni, G., Orecchia, C., Raitzer, D., and Tavoni, M. (2016). The Cost of Climate Stabilization in Southeast Asia, a Joint Assessment with Dynamic Optimization and CGE Models. Fondazione Eni Enrico Mattei Working Papers, Milan, 39.
Byrnes, L., Brown, C., Foster, J., and Wagner, L. D. (2013). Australian renewable energy policy: barriers and challenges. Renew. Energy 60, 711–721. doi:10.1016/j.renene.2013.06.024
Cai, W., Wang, C., Chen, J., and Wang, S. (2011). Green economy and green jobs: myth or reality? The case of China’s power generation sector. Energy 36, 5994–6003. doi:10.1016/j.energy.2011.08.016
Calvin, K., Clarke, L., Krey, V., Blanford, G., Jiang, K., Kainuma, M., et al. (2012). The role of Asia in mitigating climate change: results from the Asia modeling exercise. Energy Econ. 34, S251–S260. doi:10.1016/j.eneco.2012.03.008
Chappin, E. J. L., de Vries, L. J., Richstein, J. C., Bhagwat, P., Iychettira, K., and Khan, S. (2017). Simulating climate and energy policy with agent-based modelling: the energy modelling laboratory (EMLab). Environ. Model. Software 96, 421–431. doi:10.1016/j.envsoft.2017.07.009
Chathuranga, J. K., Wijayapala, W. S., and Wijayatunga, P. (2016). “Mainstreaming renewable energy developments into traditional planning: a Sri Lanka case study,” in IEEE Electrical Engineering Conference (EECon), Colombo, 59–67.
Chaturvedi, V., Eom, J., Clarke, L. E., and Shukla, P. R. (2014). Long term building energy demand for India: disaggregating end use energy services in an integrated assessment modeling framework. Energy Policy 64, 226–242. doi:10.1016/j.enpol.2012.11.021
Chauhan, A., and Saini, R. (2015). Renewable energy based off-grid rural electrification in Uttarakhand state of India: technology options, modelling method, barriers and recommendations. Renew. Sustain. Energ. Rev. 51, 662–681. doi:10.1016/j.rser.2015.06.043
Chen, W. (2005). The costs of mitigating carbon emissions in China: findings from China MARKAL-MACRO modeling. Energy Policy 33, 885–896. doi:10.1016/j.enpol.2003.10.012
Chen, W., Yin, X., and Zhang, H. (2016). Towards low carbon development in China: a comparison of national and global models. Clim. Change 136, 95–108. doi:10.1007/s10584-013-0937-7
Cinelli, M., Coles, S. R., and Kirwan, K. (2014). Analysis of the potentials of multi criteria decision analysis methods to conduct sustainability assessment. Ecol. Indic. 46, 138–148. doi:10.1016/j.ecolind.2014.06.011
Connolly, D., Lund, H., Mathiesen, B. V., and Leahy, M. (2010). A review of computer tools for analysing the integration of renewable energy into various energy systems. Appl. Energy 87, 1059–1082. doi:10.1016/j.apenergy.2009.09.026
Dai, H., Masui, T., Matsuoka, Y., and Fujimori, S. (2011). Assessment of China’s climate commitment and non-fossil energy plan towards 2020 using hybrid AIM/CGE model. Energy Policy 39, 2875–2887. doi:10.1016/j.enpol.2011.02.062
Dai, H., Xie, X., Xie, Y., Liu, J., and Masui, T. (2016). Green growth: the economic impacts of large-scale renewable energy development in China. Appl. Energy 162, 435–449. doi:10.1016/j.apenergy.2015.10.049
Daioglou, V., Van Ruijven, B. J., and Van Vuuren, D. P. (2012). Model projections for household energy use in developing countries. Energy 37, 601–615. doi:10.1016/j.energy.2011.10.044
Daniel, J., Dicorato, M., Forte, G., Iniyan, S., and Trovato, M. (2009). A methodology for the electrical energy system planning of Tamil Nadu state (India). Energy Policy 37, 904–914. doi:10.1016/j.enpol.2008.10.039
Dannenberg, A., Mennel, T., and Moslener, U. (2008). What does Europe pay for clean energy? Review of macroeconomic simulation studies. Energy Policy 36, 1318–1330. doi:10.1016/j.enpol.2007.12.010
Davidescu, A. A. (2017). What Are the Main Determinants of the Romanian Shadow Economy? An Empirical Analysis Based on Structural Equation Models, Development, Growth and Finance of Organizations from an Eastern European Context. Cham: Springer, 159–170.
de Koning, A., Bruckner, M., Lutter, S., Wood, R., Stadler, K., and Tukker, A. (2015). Effect of aggregation and disaggregation on embodied material use of products in input-output analysis. Ecol. Econ. 116, 289–299. doi:10.1016/j.ecolecon.2015.05.008
de Oliveira, L. P. N., Rochedo, P. R. R., Portugal-Pereira, J., Hoffmann, B. S., Aragão, R., Milani, R., et al. (2016). Critical technologies for sustainable energy development in Brazil: technological foresight based on scenario modelling. J. Clean. Prod. 130, 12–24. doi:10.1016/j.jclepro.2016.03.010
De Vries, B. J. M., van Vuuren, D. P., Den Elzen, M. G. J., and Janssen, M. A. (2001). The Targets IMage Energy Regional (TIMER) Model, Technical Documentation, RIVM Report 461502024 2001. Bilthoven, Netherlands: National Institute of Public Health and the Environment.
DeCarolis, J. F., Hunter, K., and Sreepathi, S. (2012). The case for repeatable analysis with energy economy optimization models. Energy Econ. 34, 1845–1853. doi:10.1016/j.eneco.2012.07.004
Després, J., Hadjsaid, N., Criqui, P., and Noirot, I. (2015). Modelling the impacts of variable renewable sources on the power sector: reconsidering the typology of energy modelling tools. Energy 80, 486–495. doi:10.1016/j.energy.2014.12.005
Duan, H.-B., Zhu, L., and Fan, Y. (2014). Optimal carbon taxes in carbon-constrained China: a logistic-induced energy economic hybrid model. Energy 69, 345–356. doi:10.1016/j.energy.2014.03.022
Dudhani, S., Sinha, A., and Inamdar, S. (2006). Renewable energy sources for peak load demand management in India. Int. J. Electric. Power Energy Syst. 28, 396–400. doi:10.1016/j.ijepes.2005.12.011
Dulal, H. B., Shah, K. U., Sapkota, C., Uma, G., and Kandel, B. R. (2013). Renewable energy diffusion in Asia: can it happen without government support? Energy Policy 59, 301–311. doi:10.1016/j.enpol.2013.03.040
Ehlen, M. A., and Scholand, A. J. (2005). “Modeling interdependencies between power and economic sectors using the N-ABLE agent based model,” in Proceedings of the IEEE Power Engineering Society General Meeting, San Francisco, 2842–2846.
Erdinc, O., Paterakis, N. G., and Catalão, J. P. (2015). Overview of insular power systems under increasing penetration of renewable energy sources: opportunities and challenges. Renew. Sustain. Energ. Rev. 52, 333–346. doi:10.1016/j.rser.2015.07.104
Espinoza, A., Bautista, S., Narváez, P., Alfaro, M., and Camargo, M. (2017). Sustainability assessment to support governmental biodiesel policy in Colombia: a system dynamics model. J. Clean. Prod. 141, 1145–1163. doi:10.1016/j.jclepro.2016.09.168
Fagiolo, G., Moneta, A., and Windrum, P. (2007). A critical guide to empirical validation of agent-based models in economics: methodologies, procedures, and open problems. Comput. Econ. 30, 195–226. doi:10.1007/s10614-007-9104-4
Foley, A., Gallachóir, B. Ó, Hur, J., Baldick, R., and McKeogh, E. (2010). A strategic review of electricity systems models. Energy 35, 4522–4530. doi:10.1016/j.energy.2010.03.057
Frei, C. W., Haldi, P.-A., and Sarlos, G. (2003). Dynamic formulation of a top-down and bottom-up merging energy policy model. Energy Policy 31, 1017–1031. doi:10.1016/S0301-4215(02)00170-2
Gerst, M. D., Wang, P., Roventini, A., Fagiolo, G., Dosi, G., Howarth, R. B., et al. (2013). Agent-based modeling of climate policy: an introduction to the ENGAGE multi-level model framework. Environ. Model. Software 44, 62–75. doi:10.1016/j.envsoft.2012.09.002
Giraudet, L.-G., Guivarch, C., and Quirion, P. (2012). Exploring the potential for energy conservation in French households through hybrid modeling. Energy Econ. 34, 426–445. doi:10.1016/j.eneco.2011.07.010
Goh, H. H., Lee, S., Chua, Q., Goh, K. C., Kok, B. C., and Teo, K. (2014). Renewable energy project: project management, challenges and risk. Renew. Sustain. Energ. Rev. 38, 917–932. doi:10.1016/j.rser.2014.07.078
Golestaneh, F., Pinson, P., and Gooi, H. B. (2016). Very short-term nonparametric probabilistic forecasting of renewable energy generation – with application to solar energy. IEEE Trans. Power Syst. 31, 3850–3863. doi:10.1109/TPWRS.2015.2502423
Hainoun, A., Aldin, M. K. S., Saba, G., and Almoustafa, S. (2015). Long-term expansion planning for the Syrian electric system using the optimisation model WASP-IV. Int. J. Global Energy Issues 38, 164–195. doi:10.1504/IJGEI.2015.069483
Hak, M., Matsuoka, Y., and Gomi, K. (2017). A qualitative and quantitative design of low-carbon development in Cambodia: energy policy. Energy Policy 100, 237–251. doi:10.1016/j.enpol.2016.10.017
Halog, A., and Manik, Y. (2011). Advancing integrated systems modelling framework for life cycle sustainability assessment. Sustainability 3, 469–499. doi:10.3390/su3020469
Herbst, A., Toro, F., Reitze, F., and Jochem, E. (2012). Introduction to energy systems modelling. Swiss J. Econ. Stat. 148, 111–135.
Hertwich, E. G., Gibon, T., Bouman, E. A., Arvesen, A., Suh, S., Heath, G. A., et al. (2015). Integrated life-cycle assessment of electricity-supply scenarios confirms global environmental benefit of low-carbon technologies. Proc. Natl. Acad. Sci. U.S.A. 112, 6277–6282. doi:10.1073/pnas.1312753111
Hiremath, R., Shikha, S., and Ravindranath, N. (2007). Decentralized energy planning; modeling and application – a review. Renew. Sustain. Energ. Rev. 11, 729–752. doi:10.1016/j.rser.2005.07.005
Hsu, C.-W. (2012). Using a system dynamics model to assess the effects of capital subsidies and feed-in tariffs on solar PV installations. Appl. Energy 100, 205–217. doi:10.1016/j.apenergy.2012.02.039
Huang, Y., Bor, Y. J., and Peng, C.-Y. (2011). The long-term forecast of Taiwan’s energy supply and demand: LEAP model application. Energy Policy 39, 6790–6803. doi:10.1016/j.enpol.2010.10.023
Ishizaka, A., Siraj, S., and Nemery, P. (2016). Which energy mix for the UK (United Kingdom)? An evolutive descriptive mapping with the integrated GAIA (graphical analysis for interactive aid)-AHP (analytic hierarchy process) visualization tool. Energy 95, 602–611. doi:10.1016/j.energy.2015.12.009
Jacobsen, H. K. (1998). Integrating the bottom-up and top-down approach to energy-economy modelling: the case of Denmark. Energy Econ. 20, 443–461. doi:10.1016/S0140-9883(98)00002-4
Jebaraj, S., and Iniyan, S. (2006). A review of energy models. Renew. Sustain. Energ. Rev. 10, 281–311. doi:10.1016/j.rser.2004.09.004
Kabak, M., and Dağdeviren, M. (2014). Prioritization of renewable energy sources for Turkey by using a hybrid MCDM methodology. Energy Convers. Manag. 79, 25–33. doi:10.1016/j.enconman.2013.11.036
Karaca, C. (2016). The Comparison of the Shadow Economy in Turkey and European Countries, Comparative Economics and Regional Development in Turkey. IGI Global, Hershey, 73–105.
Kelly, R. A., Jakeman, A. J., Barreteau, O., Borsuk, M. E., ElSawah, S., Hamilton, S. H., et al. (2013). Selecting among five common modelling approaches for integrated environmental assessment and management. Environ. Model. Software 47, 159–181. doi:10.1016/j.envsoft.2013.05.005
Ko, F.-K., Huang, C.-B., Tseng, P.-Y., Lin, C.-H., Zheng, B.-Y., and Chiu, H.-M. (2010). Long-term CO2 emissions reduction target and scenarios of power sector in Taiwan. Energy Policy 38, 288–300. doi:10.1016/j.enpol.2009.09.018
Koesrindartoto, D., Sun, J., and Tesfatsion, L. (2005). An agent-based computational laboratory for testing the economic reliability of wholesale power market designs. Paper presented at the IEEE Power Engineering Society General Meeting 2005. San Francisco.
Koopmans, C. C., and te Velde, D. W. (2001). Bridging the energy efficiency gap: using bottom-up information in a top-down energy demand model. Energy Econ. 23, 57–75. doi:10.1016/S0140-9883(00)00054-2
Krey, V., O’Neill, B. C., van Ruijven, B., Chaturvedi, V., Daioglou, V., Eom, J., et al. (2012). Urban and rural energy use and carbon dioxide emissions in Asia. Energy Econ. 34, S272–S283. doi:10.1016/j.eneco.2012.04.013
Kursun, B., Bakshi, B. R., Mahata, M., and Martin, J. F. (2015). Life cycle and emergy based design of energy systems in developing countries: centralized and localized options. Ecol. Model. 305, 40–53. doi:10.1016/j.ecolmodel.2015.03.006
Leduc, S., Natarajan, K., Dotzauer, E., McCallum, I., and Obersteiner, M. (2009). Optimizing biodiesel production in India. Appl. Energy 86, S125–S131. doi:10.1016/j.apenergy.2009.05.024
Li, F. G., Trutnevyte, E., and Strachan, N. (2015). A review of socio-technical energy transition (STET) models. Technol. Forecast. Soc. Change 100, 290–305. doi:10.1016/j.techfore.2015.07.017
Liu, Q., Shi, M., and Jiang, K. (2009). New power generation technology options under the greenhouse gases mitigation scenario in China. Energy Policy 37, 2440–2449. doi:10.1016/j.enpol.2009.02.044
Luthra, S., Kumar, S., Garg, D., and Haleem, A. (2015). Barriers to renewable/sustainable energy technologies adoption: Indian perspective. Renew. Sustain. Energ. Rev. 41, 762–776. doi:10.1016/j.rser.2014.08.077
Maani, K. (2009). System Dynamics and Organizational Learning, Complex Systems in Finance and Econometrics. Larkspur: Springer, 738–752.
Manik, Y., Leahy, J., and Halog, A. (2013). Social life cycle assessment of palm oil biodiesel: a case study in Jambi Province of Indonesia. Int. J. Life Cycle Assess. 18, 1386–1392. doi:10.1007/s11367-013-0581-5
Marquardt, J. (2014). A struggle of multi-level governance: promoting renewable energy in Indonesia. Energy Proc. 58, 87–94. doi:10.1016/j.egypro.2014.10.413
Masini, A., and Menichetti, E. (2013). Investment decisions in the renewable energy sector: an analysis of non-financial drivers. Technol. Forecast. Soc. Change 80, 510–524. doi:10.1016/j.techfore.2012.08.003
McFarland, J. R., Reilly, J. M., and Herzog, H. J. (2004). Representing energy technologies in top-down economic models using bottom-up information. Energy Econ. 26, 685–707. doi:10.1016/j.eneco.2004.04.026
McPherson, M., and Karney, B. (2014). Long-term scenario alternatives and their implications: LEAP model application of Panama’s electricity sector. Energy Policy 68, 146–157. doi:10.1016/j.enpol.2014.01.028
Meier, P., and Mubayi, V. (1983). Modelling energy-economic interactions in developing countries: a linear programming approach. Eur. J. Oper. Res. 13, 41–59. doi:10.1016/0377-2217(83)90006-1
Möst, D., and Keles, D. (2010). A survey of stochastic modelling approaches for liberalised electricity markets. Eur. J. Oper. Res. 207, 543–556. doi:10.1016/j.ejor.2009.11.007
Murphy, R., and Jaccard, M. (2011). Energy efficiency and the cost of GHG abatement: a comparison of bottom-up and hybrid models for the US. Energy Policy 39, 7146–7155. doi:10.1016/j.enpol.2011.08.033
Murphy, R., Rivers, N., and Jaccard, M. (2007). Hybrid modeling of industrial energy consumption and greenhouse gas emissions with an application to Canada. Energy Econ. 29, 826–846. doi:10.1016/j.eneco.2007.01.006
Nakata, T., Silva, D., and Rodionov, M. (2011). Application of energy system models for designing a low-carbon society. Prog. Energy Combust. Sci. 37, 462–502. doi:10.1016/j.pecs.2010.08.001
Nepal, R. (2012). Roles and potentials of renewable energy in less-developed economies: the case of Nepal. Renew. Sustain. Energ. Rev. 16, 2200–2206. doi:10.1016/j.rser.2012.01.047
Nepal, R., and Jamasb, T. (2015). Caught between theory and practice: government, market, and regulatory failure in electricity sector reforms. Econ. Anal. Policy 46, 16–24. doi:10.1016/j.eap.2015.03.001
Nerini, F. F., Dargaville, R., Howells, M., and Bazilian, M. (2015). Estimating the cost of energy access: the case of the village of Suro Craic in Timor Leste. Energy 79, 385–397. doi:10.1016/j.energy.2014.11.025
Nfuka, E. N., and Rusu, L. (2009). IT Governance in the Public Sector in a Developing Country, Handbook of Research on ICT-Enabled Transformational Government: A Global Perspective. Hershey: IGI Global, 452–486.
Nguyen, Q. K. (2012). “Study on the impacts of electricity tariff increase on the national economy of Vietnam,” in Energy Market Integration in East Asia: Theories, Electricity Sector and Subsidies, ERIA Research Project Report 2011-17 ed, eds Y. Wu, X. Shi, and F. Kimura (Jakarta: ERIA), 253–267.
Ozcan, M. (2017). The role of renewables in increasing Turkey’s self-sufficiency in electrical energy. Renew. Sustain. Energ. Rev. doi:10.1016/j.rser.2017.09.111
Pandey, R. (2002). Energy policy modelling: agenda for developing countries. Energy Policy 30, 97–106. doi:10.1016/S0301-4215(01)00062-3
Pfenninger, S., Hawkes, A., and Keirstead, J. (2014). Energy systems modeling for twenty-first century energy challenges. Renew. Sustain. Energ. Rev. 33, 74–86. doi:10.1016/j.rser.2014.02.003
Pohekar, S., and Ramachandran, M. (2004). Application of multi-criteria decision making to sustainable energy planning – a review. Renew. Sustain. Energ. Rev. 8, 365–381. doi:10.1016/j.rser.2003.12.007
Rahman, M. M., Paatero, J. V., Lahdelma, R., and Wahid, M. A. (2016). Multicriteria-based decision aiding technique for assessing energy policy elements-demonstration to a case in Bangladesh. Appl. Energy 164, 237–244. doi:10.1016/j.apenergy.2015.11.091
Rai, V., and Robinson, S. A. (2015). Agent-based modeling of energy technology adoption: empirical integration of social, behavioral, economic, and environmental factors. Environ. Model. Software 70, 163–177. doi:10.1016/j.envsoft.2015.04.014
Ramakumar, R., Shetty, P. S., and Ashenayi, K. (1986). A linear programming approach to the design of integrated renewable energy systems for developing countries IEEE Transactions on Energy Conversion, EC-1, 18–24.
Ramasamy, P., Chandel, S., and Yadav, A. K. (2015). Wind speed prediction in the mountainous region of India using an artificial neural network model. Renew. Energy 80, 338–347. doi:10.1016/j.renene.2015.02.034
REN21. (2017). Renewables 2017 Global Status Report. Paris: Renewable Energy Policy Network for the 21 Century (REN21) Secretariat.
Ringler, P., Keles, D., and Fichtner, W. (2016). Agent-based modelling and simulation of smart electricity grids and markets – a literature review. Renew. Sustain. Energ. Rev. 57, 205–215. doi:10.1016/j.rser.2015.12.169
Rivera, D. G., García, J. G., Amezcua, T. H., Acevedo, C. I., Cortés, I. I., and Landa, G. (2015). “Transitioning towards a low-carbon economy in Mexico,” in Etudes, eds F. Grazi and F.-X. Bellocq (Paris: Agence Française de Développement (AFD)), 70.
Robalino-López, A., Mena-Nieto, A., and García-Ramos, J. E. (2014). System dynamics modeling for renewable energy and CO2 emissions: a case study of Ecuador. Energy Sustain. Dev. 20, 11–20. doi:10.1016/j.esd.2014.02.001
Sarica, K., and Tyner, W. E. (2013). Alternative policy impacts on US GHG emissions and energy security: a hybrid modeling approach. Energy Econ. 40, 40–50. doi:10.1016/j.eneco.2013.06.003
Sensfuß, F., Genoese, M., Ragwitz, M., and Möst, D. (2007). Agent-based simulation of electricity markets – a literature review. Energy Stud. Rev. 15, 1–29.
Shakya, S. R., Kumar, S., and Shrestha, R. M. (2012). Co-benefits of a carbon tax in Nepal. Mitigation Adapt. Strat. Global Change 17, 77–101. doi:10.1007/s11027-011-9310-1
Shukla, P., Rana, A., Garg, A., Kapshe, M., and Nair, R. (2006). Global climate change stabilization regimes and Indian emission scenarios: lessons for modeling of developing country transitions. Environ. Econ. Policy Stud. 7, 205–231. doi:10.1007/BF03354000
Shukla, P. R. (1995). Greenhouse gas models and abatement costs for developing nations: a critical assessment. Energy Policy 23, 677–687. doi:10.1016/0301-4215(95)00062-N
Shukla, P. R., and Chaturvedi, V. (2012). Low carbon and clean energy scenarios for India: analysis of targets approach. Energy Econ. 34, S487–S495. doi:10.1016/j.eneco.2012.05.002
Smajgl, A., and Bohensky, E. (2013). Behaviour and space in agent-based modelling: poverty patterns in East Kalimantan, Indonesia. Environ. Model. Software 45, 8–14. doi:10.1016/j.envsoft.2011.10.014
Sovacool, B. K. (2013). A qualitative factor analysis of renewable energy and sustainable energy for all (SE4ALL) in the Asia-Pacific. Energy Policy 59, 393–403. doi:10.1016/j.enpol.2013.03.051
Strachan, N., and Kannan, R. (2008). Hybrid modelling of long-term carbon reduction scenarios for the UK. Energy Econ. 30, 2947–2963. doi:10.1016/j.eneco.2008.04.009
Suganthi, L., and Samuel, A. A. (2012). Energy models for demand forecasting – a review. Renew. Sustain. Energ. Rev. 16, 1223–1240. doi:10.1016/j.rser.2011.08.014
Tahri, M., Hakdaoui, M., and Maanan, M. (2015). The evaluation of solar farm locations applying geographic information system and multi-criteria decision-making methods: case study in southern Morocco. Renew. Sustain. Energ. Rev. 51, 1354–1362. doi:10.1016/j.rser.2015.07.054
Tang, A. (2013). Leveraging Policy for Renewable Energy Development in Industrialized Countries and Emerging Markets. New York: Columbia University.
Taylor, P. G., Upham, P., McDowall, W., and Christopherson, D. (2014). Energy model, boundary object and societal lens: 35 years of the MARKAL model in the UK. Energy Res. Soc. Sci. 4, 32–41. doi:10.1016/j.erss.2014.08.007
Tomaschek, J., Kober, R., Fahl, U., and Lozynskyy, Y. (2016). Energy system modelling and GIS to build an integrated climate protection concept for Gauteng Province, South Africa. Energy Policy 88, 445–455. doi:10.1016/j.enpol.2015.10.041
Tsai, S.-B., Xue, Y., Zhang, J., Chen, Q., Liu, Y., Zhou, J., et al. (2017). Models for forecasting growth trends in renewable energy. Renew. Sustain. Energ. Rev. 77, 1169–1178. doi:10.1016/j.rser.2016.06.001
Urban, F., Benders, R., and Moll, H. (2007). Modelling energy systems for developing countries. Energy Policy 35, 3473–3482. doi:10.1016/j.enpol.2006.12.025
Vahdani, B., Mousavi, S. M., Tavakkoli-Moghaddam, R., and Hashemi, H. (2013). A new design of the elimination and choice translating reality method for multi-criteria group decision-making in an intuitionistic fuzzy environment. Appl. Math. Model. 37, 1781–1799. doi:10.1016/j.apm.2012.04.033
Van Ruijven, B., Hari, L., Van Vuuren, D. P., and De Vries, B. (2008a). The potential role of hydrogen energy in India and Western Europe. Energy Policy 36, 1649–1665. doi:10.1016/j.enpol.2008.01.020
Van Ruijven, B., Urban, F., Benders, R. M., Moll, H. C., Van Der Sluijs, J. P., De Vries, B., et al. (2008b). Modeling energy and development: an evaluation of models and concepts. World Dev. 36, 2801–2821. doi:10.1016/j.worlddev.2008.01.011
van Ruijven, B. J., van Vuuren, D. P., De Vries, B. J., Isaac, M., van der Sluijs, J. P., Lucas, P. L., et al. (2011). Model projections for household energy use in India. Energy Policy 39, 7747–7761. doi:10.1016/j.enpol.2011.09.021
Varun, G., Prakash, R., and Bhat, I. (2010). Life cycle energy and GHG analysis of hydroelectric power development in India. Int. J. Green Energy 7, 361–375. doi:10.1080/15435075.2010.493803
Vasconcelos, P. S., and Carpio, L. G. T. (2015). Estimating the economic costs of electricity deficit using input-output analysis: the case of Brazil. Appl. Econ. 47, 916–927. doi:10.1080/00036846.2014.982858
Ventosa, M., Baıllo, A., Ramos, A., and Rivier, M. (2005). Electricity market modeling trends. Energy Policy 33, 897–913. doi:10.1016/j.enpol.2003.10.013
Veselka, T., Boyd, G., Conzelmann, G., Koritarov, V., Macal, C., North, M., et al. (2002). Simulating the Behavior of Electricity Markets with an Agent-Based Methodology: The Electric Market Complex Adaptive Systems (EMCAS) Model. Vancouver, Canada: Argonne National Lab/University of Chicago. Available at: http://citeseerx.ist.psu.edu/viewdoc/download;jsessionid=ED298FC3D5D7F177D71009B4B47AE032?doi=10.1.1.500.8715&rep=rep1&type=pdf
Weidlich, A., and Veit, D. (2008). A critical survey of agent-based wholesale electricity market models. Energy Econ. 30, 1728–1759. doi:10.1016/j.eneco.2008.01.003
Weijermars, R., Taylor, P., Bahn, O., Das, S. R., and Wei, Y.-M. (2012). Review of models and actors in energy mix optimization-can leader visions and decisions align with optimum model strategies for our future energy systems? Energy Strat. Rev. 1, 5–18. doi:10.1016/j.esr.2011.10.001
Wesseh, P. K., and Lin, B. (2016). Can African countries efficiently build their economies on renewable energy? Renew. Sustain. Energ. Rev. 54, 161–173. doi:10.1016/j.rser.2015.09.082
Wianwiwat, S., and Asafu-Adjaye, J. (2011). Modelling the promotion of biomass use: a case study of Thailand. Energy 36, 1735–1748. doi:10.1016/j.energy.2010.12.055
Wing, I. S. (2006). The synthesis of bottom-up and top-down approaches to climate policy modeling: electric power technologies and the cost of limiting US CO 2 emissions. Energy Policy 34, 3847–3869. doi:10.1016/j.enpol.2005.08.027
Wing, I. S., Joint Program on the Science and Policy of Global Change MIT. (2008). The synthesis of bottom-up and top-down approaches to climate policy modeling: electric power technology detail in a social accounting framework. Energy Econ. 30, 547–573. doi:10.1016/j.eneco.2006.06.004
Yangka, D., and Diesendorf, M. (2016). Modeling the benefits of electric cooking in Bhutan: a long term perspective. Renew. Sustain. Energ. Rev. 59, 494–503. doi:10.1016/j.rser.2015.12.265
Yu, S., Zhu, K., and Zhang, X. (2012). Energy demand projection of China using a path-coefficient analysis and PSO-GA approach. Energy Convers. Manag. 53, 142–153. doi:10.1016/j.enconman.2011.08.015
Zhao, X., and Luo, D. (2017). Driving force of rising renewable energy in China: environment, regulation and employment. Renew. Sustain. Energ. Rev. 68, 48–56. doi:10.1016/j.rser.2016.09.126
Keywords: top-down approach, bottom-up approach, system thinking, life cycle thinking, decision support analysis, low carbon transition, developing countries
Citation: al Irsyad MI, Halog AB, Nepal R and Koesrindartoto DP (2017) Selecting Tools for Renewable Energy Analysis in Developing Countries: An Expanded Review. Front. Energy Res. 5:34. doi: 10.3389/fenrg.2017.00034
Received: 02 August 2017; Accepted: 04 December 2017;
Published: 20 December 2017
Edited by:
Eunil Park, Hanyang University, South KoreaCopyright: © 2017 al Irsyad, Halog, Nepal and Koesrindartoto. This is an open-access article distributed under the terms of the Creative Commons Attribution License (CC BY). The use, distribution or reproduction in other forums is permitted, provided the original author(s) or licensor are credited and that the original publication in this journal is cited, in accordance with accepted academic practice. No use, distribution or reproduction is permitted which does not comply with these terms.
*Correspondence: Anthony Basco Halog, YS5oYWxvZ0B1cS5lZHUuYXU=
Disclaimer: All claims expressed in this article are solely those of the authors and do not necessarily represent those of their affiliated organizations, or those of the publisher, the editors and the reviewers. Any product that may be evaluated in this article or claim that may be made by its manufacturer is not guaranteed or endorsed by the publisher.
Research integrity at Frontiers
Learn more about the work of our research integrity team to safeguard the quality of each article we publish.