- 1Department of Psychology, Zhejiang Normal University, Jinhua, China
- 2School of Psychology and Cognitive Science, East China Normal University, Shanghai, China
- 3Department of Psychology, London Metropolitan University, London, United Kingdom
- 4School of Psychology, Southwest University, Chongqing, China
- 5Peking-Tsinghua Center for Life Science, Peking University, Beijing, China
- 6Department of Physics, Shanghai Key Laboratory of Magnetic Resonance, East China Normal University, Shanghai, China
- 7Institute of Psychological and Brain Sciences, Zhejiang Normal University, Jinhua, China
Internet gaming disorder (IGD), defined as the persistent use of online games with ignorance of adverse consequences, has increasingly raised widespread public concerns. This study aimed at elucidating the precise mechanisms underlying IGD by comparing intertemporal decision-making process between 18 IGD participants and 21 matched healthy controls (HCs). Both behavioral and fMRI data were recorded from a delay discounting task. At the behavioral level, the IGD showed a higher discount rate k than HC; and in IGD group, both the reaction time (delay − immediate) and the discount rate k were significantly positively correlated with the severity of IGD. At the neural level, the IGD exhibited reduced brain activations in the dorsolateral prefrontal cortex and bilateral inferior frontal gyrus compared to HC during performing delay trials relative to immediate ones. Taken together, the results suggested that IGD showed deficits in making decisions and tended to pursuit immediate satisfaction. The underlying mechanism arises from the deficient ability in evaluating between delayed reward and immediate satisfaction, and the impaired ability in impulse inhibition, which may be associated with the dysfunction of the prefrontal activation. These might be the reason why IGD continue playing online games in spite of facing severe negative consequences.
Introduction
Internet gaming disorder (IGD) has increasingly raised widespread public concerns. It is defined as recurrent and persistent use of online games, which lead to a variety of negative consequences in terms of daily life and mental health, such as maladaptive coping, ill interpersonal relationship, and decreased academic achievements (1, 2). Experimental studies and questionnaire surveys have indicated that individuals with IGD show great behavioral and neuronal similarities to those with drug addictions, substance abuse, and gambling disorder in many aspects, involving comorbid psychiatric symptoms, behavior control, and decision-making (3–5). Nevertheless, compared with substance-related and addictive disorders (e.g., alcohol abuse disorder), a significant feature for IGD is no substance or chemical intake. In May 2013, IGD has been listed in Section “Results” of the DSM-5 as a condition warranting further studies (6–8).
Intertemporal decision-making refers to situations where people need to choose between two options: an immediate but smaller reward and a delayed but larger one (9). Delay discounting task (DDT) is a widely used paradigm in exploring intertemporal decision-making and measuring impulsive choices (10), but rarely used to detect the decision-making and planning of IGD. When the delay is shorter, people generally prefer the larger reward rather than the smaller one; but with gradually increased delay, people will shift their preference to the smaller reward rather than the larger one. Individuals who shift their preferences to smaller rewards after shorter delays would be regarded as more impulsive than individuals who shift their preferences after longer delays (11). Studies using DDT have found that delayed rewards tend to be more steeply discounted in substance addicts in relation to alcohol (12), heroin (13), cocaine (14), methamphetamine (15), and pathological gamblers (16) when compared to healthy controls (HCs). Furthermore, there is evidence that individuals with IGD are more impulsive than recreational Internet gaming users and HC (17–20). These findings raise the possibility that the IGD, in accordance with drug and gambling addicts, show myopia for the future, i.e., preference for short-term rewards (e.g., Internet games) and ignorance for long-term losses (e.g., social relationship).
Previous works with the DDT established the neural correlates of brain regions in intertemporal decision-making and then proposed a dual-valuation model, which assumed that there were two separate systems contributing to such decisions (21, 22). One system (called the “β system”) included mesolimbic dopamine projection regions and weighed the immediate rewards (i.e., nucleus accumbens and medial prefrontal cortex); the other system (called the “δ system”) included the lateral prefrontal cortical areas and weighed the delayed rewards. Human imaging studies also explored brain activations during delay discounting process in behavioral addiction and substance dependence samples. Pathological gamblers showed elevated brain activities in the dorsolateral prefrontal cortex (DLPFC) and amygdala when selecting delayed rewards compared with HC (23). Alcoholics were reported to show increased activities in the inferior frontal gyrus (IFG), insula, and supplementary motor area along with steeper discounting of delayed rewards (24). Smokers also exhibited dysfunctional brain activations in the IFG, DLPFC, and insula during the inhibition of immediate smaller rewards to gain the delayed larger ones (25). The DLPFC has been proved to be involved in behavioral inhibition, reward processing, and decision-making; the IFG is also critical for inhibition and risky decision-making; besides, the insula plays a part in cognitive function and motor control (26–28). Specifically, the altered functional connectivity in the bilateral prefrontal lobe has been detected in IGD (29).
Although previous researches have revealed decision-making deficits in IGD, the underlying mechanism of impaired ability to control their behaviors remains unclear. To explore the reasons why individuals with IGD pursue instantaneous rewarding experience regardless of long-term benefits, 21 HCs and 18 IGD were recruited to perform the DDT, which comprised a series of selections between immediate smaller monetary rewards and delayed larger monetary rewards.
Our previous study has found that the participants with IGD were prone to take risks and exhibited less activation in the IFG and superior temporal gyri when making risky choices in comparison to HC (30). A study that used Go/No-Go paradigm with gaming cue distraction found that the IGD showed impaired response inhibition and decreased brain activities in the right DLPFC (31). In individuals with IGD, viewing Internet gaming-related stimuli significantly induced increased brain activations in the prefrontal cortex, inferior parietal lobule, and striatum (19, 20, 32). These findings suggest that the brain regions associated with cognitive control, craving, decision-making, and reward induce dysfunctional effects by virtue of the frequent use of Internet games in IGD. Therefore, we hypothesized that the IGD group may show similar behavioral tendency (myopia for the future) and brain activation patterns parallel with findings in other addiction disorders. At the behavioral level, we expected to observe steeper discounting of delayed rewards in IGD compared to HC and a modulation of delayed reward representations by the severity of IGD. At the neural level, we expected IGD to show less brain activations in those brain regions (i.e., DLPFC, IFG), which are related to the evaluation of delayed rewards, and to impulse inhibition. We also expected that brain activations would be correlated with behavioral performances in IGD group.
Materials and Methods
Participants
The experiment conforms to the Code of Ethics of the World Medical Association (Declaration of Helsinki). The Human Investigations Committee of Zhejiang Normal University approved this research. All participants signed the informed consent forms before the experiment. Participants were right-handed male students (18 IGD and 21 HC) recruited through advertisements in Shanghai, PR China. Only males were included due to higher IGD prevalence in men than that in women. There were several exclusion criteria for selecting participants, including history or current neurological or mental disorders as measured by MINI international neuropsychiatric interview and the mood states scale, history or current psychiatric disease (e.g., depression, schizophrenia), and history of drug abuse (e.g., cocaine, alcohol) or any other type of behavioral addictions as measured by standard interviews and self-report instruments. All participants did not report a history of behavioral addiction, substance abuses, and mental disorders. Importantly, none of them reported brain injuries, brain surgeries, and any attention problems such as attention deficit hyperactivity disorder. In addition, all participants were told to not take any addictive substances 3 h before the experiment began, including coffee, cigarette, and alcohol.
The diagnosis of IGD was determined based on (1) a modified Young’s online Internet Addiction Test (33), which emphasized on IGD (IAT, see Supplementary Material), (2) the proposed nine-item IGD diagnostic scale based on DSM-5 (34), and (3) the criteria for time and frequency of gaming playing. Both the questionnaire and criteria were precisely translated into Chinese for the suitability of participants. To critically assess gaming behaviors and IGD symptoms, we then replaced all the statements of online activities in the original questionnaire with specific items, such as game playing or online games. The validity of the modified IAT was tested, and the Cronbach’s alpha coefficient of reliability index was an acceptable 0.90. The modified IAT consists of 20 items associated with online games including psychological dependence, compulsive use, withdrawal, related problems in school or work, sleep, family, and time management. For each item, participants were instructed to choose a number from the following scale: 1 = “Rarely” to 5 = “Always,” or “Does not Apply.” The score of the modified IAT is ranged from 20 to 100, which represents the severity of IGD. Scores over 50 indicate occasional or frequent Internet addiction problems, and scores over 80 indicate severe Internet addiction problems (35).
The demographic characteristics for both groups were shown in Table 1. The IGD and HC did not significantly differ in age and education years. In this study, the IGD group was composed of individuals who (1) scored over 50 on the modified IAT, (2) met at least five of the nine DSM-5 criteria, (3) spent at least 2 h on online games per day during the last 2 years, and (4) spent most of their online time playing online games (>80%). However, the HC group did not satisfy any above-mentioned criteria.
Task and Procedure
The whole time of the task lasted about 15 min for each participant. Participants first practiced 20 trials to be familiar with the task before completed the DDT task in the scanner. During the task, participants need to make choices between an immediate reward and a larger amount of money with a specified delayed time (e.g., now 10 Yuan versus 7 days later 12 Yuan, $1 is equal to about 6.6 Yuan). The monetary amounts varied from 12 to 15, 20, 30, 40, and 50 Yuan, and the delay time ranged from 6 h to 1, 3, 7, 30, and 90 days. Thus, there were 36 trials in 1 block, and the task consisted 2 blocks in total. The trials in this study were presented randomly in E-prime (version 2.0, Psychology Software Tool, Figure 1).
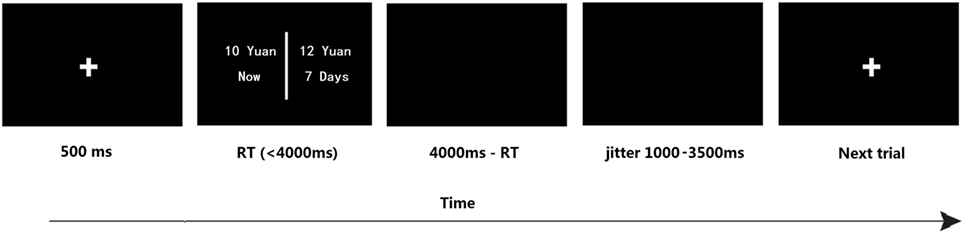
Figure 1. The timeline of one trial in the delay discounting task. The immediate but smaller option is fixed on 10 Yuan; in the delayed but larger options, monetary amounts ranged from 12 to 15, 20, 30, 40, and 50 Yuan, and the delay time ranged from 6 h to 1, 3, 7, 30, and 90 days. “Yuan” is the basic unit of money in China.
All participants were paid a guaranteed 40 Yuan (≈$6) for the participation and an extra reward (ranged from 12 to 50 Yuan) that depended on their selections in DDT task. To elicit participants’ motivation to response properly, they were informed that they would receive additional payments according to their performances during the task. For example, if they selected the fixed money on the trial, then they would gain 10 Yuan in cash; if they selected the delayed option, they would gain that amount of money in cash after the corresponding delay.
Behavioral Data Analysis
Delay discounting rate was estimated for each participant by the following hyperbolic model (36):
The V represents the subjective value of the delayed reward; A is the amount of the delayed reward; D is the length of delay to its delivery; and k is a free parameter that indicates the steepness of the discount curve. Higher k values indicate more rapid discounting and greater impulsivity (37–39). An important procedure for estimating k value was to determine the indifference points, which were the points that the fixed reward and the delayed reward were of equal subjective value for an individual. The indifference points were calculated across a series of different delay lengths and monetary amounts and were fitted into the Eq. 1. There were two steps of the behavior data analyses for DDT. In the first step, a non-linear curve-fitting program (Origin 7.0) was used to determine each participant’s best-fit values of k. The second step was to perform a log 10 transformation of the k values. The log transformation was required for these data due to their non-normal distribution (40, 41). To examine the different discount rate k of IGD and HC, an independent sample t test was performed.
Image Acquisition and Pre-Processing
fMRI data were collected using a 3T scanner (Siemens Trio) with a gradient-echo EPI T2 sensitive pulse sequence in 33 slices (interleaved sequence, 3-mm thickness, repetition time = 2,000 ms, echo time (TE) = 30 ms, flip angle 90°, field of view 220 × 220 mm2, matrix 64 × 64). Stimuli were presented by Invivo synchronous system (Invivo Company)1 through a monitor in the head coil. Structural images covering the whole brain were collected using a T1-weighted three-dimensional spoiled gradient-recalled sequence (176 slices, flip angle = 15°, TE = 3.93 ms, slice thickness = 1.0 mm, skip = 0 mm, inversion time = 1100 ms, field of view = 240 × 240 mm, and in-plane resolution = 256 × 256).
The pre-processing of imaging analysis was conducted through Statistical Parametric Mapping (SPM) software package, SPM5.2 Images were slice-timed, reoriented, and realigned to the first volume. T1-co-registered volumes were then normalized to an SPM T1 template and spatially smoothed using a 6-mm full-at-half-maximum Gaussian kernel.
First-Level Regression Analysis
A general linear model (GLM) was applied to identify blood oxygen level dependence (BOLD) signal in relation to two conditions: choice of immediate smaller reward and choice of delayed larger reward. Error trials were excluded. The GLM was independently applied to each voxel to identify voxels that were significantly activated for the event types of interest. A high pass filter (cut-off period = 128 s) was applied to improve the signal-to-noise ratio by filtering out low frequency noise.
Second-Level Group Analysis
Second-level analysis was performed at the group level. First, we determined which voxels showed a main effect of delayed trials versus immediate trials within each group (IGD, HC). Second, we tested which voxels significantly differed in BOLD signal between IGD and HC [(IGDdelay − IGDimmediate) − (HCdelay − HCimmediate)]. Third, we identified clusters of contiguously significant voxels at an uncorrected threshold p < 0.05. Finally, we tested these clusters for cluster-level FWE correction p < 0.05, and the AlphaSim estimation indicated that clusters with 102 contiguous voxels would achieve an effective FWE threshold p < 0.05. The smoothing kernel was 6.0 mm, which was used during simulating false-positive (noise) maps through AlphaSim and was estimated from the residual fields of the contrast maps used in the one-sample t-test.
Correlation Analysis
Correlation analysis was calculated between brain activities and the behavioral performances to test our hypothesis. We further carried out ROI analyses with seed regions from contrast delay trials versus immediate trials. For each ROI, a representative beta value was obtained by averaging the signal of all the voxels within the ROI. The correlations among the severity of IGD, log k values, reaction time (RT), and the beta values were calculated. The RT stands for the difference between the response to delayed options and the response to immediate options (delay – immediate).
Results
Behavioral Performance
The result of independent sample t-test suggested that the k value of IGD was higher than that of HC at a marginal significant level (t = 2.01, p = 0.05, d = 0.53). The mean discounting rate k values and corresponding SDs for IGD and HC were 0.19 ± 0.16 and 0.11 ± 0.14, respectively (Figure 2A), and this indicated the IGD discounted the rewards more steeply than HC (Figure 2B). The R2 value for discounting function (0.88 for IGD and 0.71 for HC) denoted the variance accounted for by the Eq. 1. The RT (delay − immediate) of IGD was longer than HC, but it did not reach statistical significance (HC: −86 ± 213 ms, IGD: −56 ± 194 ms, t (1, 37) = 1.43, p = 0.11). In addition, the severity of IGD was significantly positively correlated with the log k values (r = 0.552, p = 0.027; Figure 3A) and RT (r = 0.530, p = 0.035; Figure 3B) in IGD group. But the correlations among these variables did not reach a significant level in HC group.
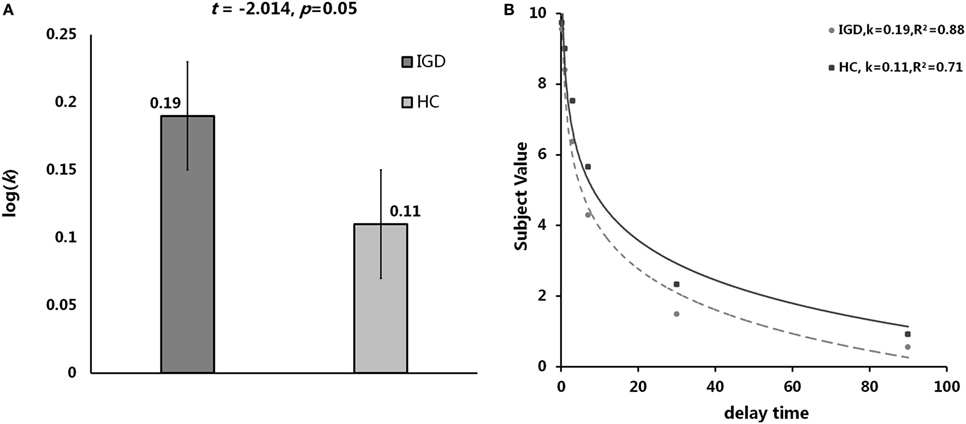
Figure 2. Delay discounting value differences between Internet gaming disorder (IGD) and healthy control (HC). (A) The IGD showed higher k value than HC. (B) Delay discount functions for HC and IGD. Points show mean indifferent points for monetary rewards as a function of delay time. R2 represents how close the fitted curve is from the actual data points. First, the variation between data points and the mean values is calculated. In least-squares fitting, the total sum of squares (TSS) includes two parts: the variation explained by regression and that not explained by regression [the residual sum of square (RSS)]. Then the R2 = 1 − RSS/TSS.
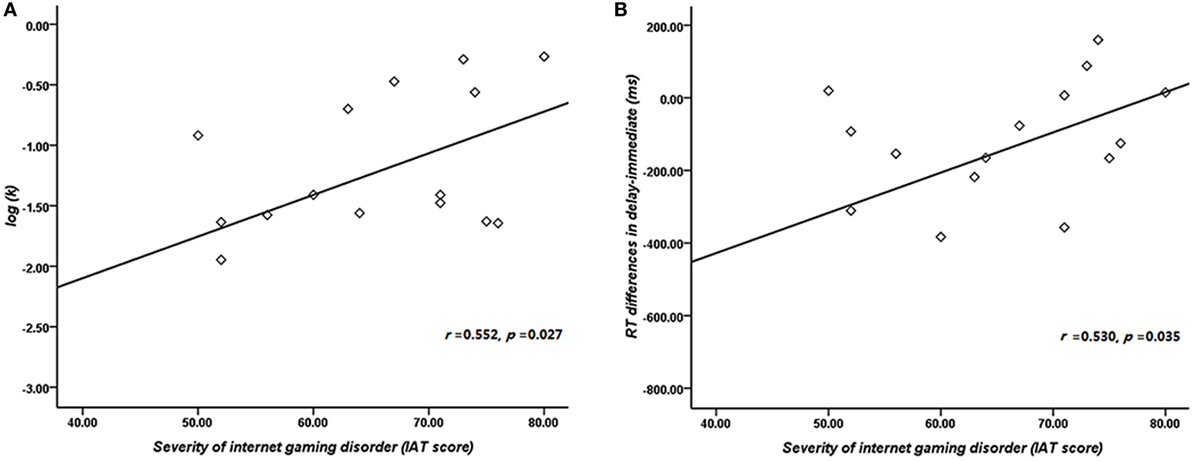
Figure 3. Correlation between the severity of Internet gaming disorder (IGD) and behavioral performance. (A) Correlation between the severity of IGD and log k. (B) Correlation between the severity of IGD and reaction time (delay − immediate). (Scores greater than 3 SDs were regarded as outliers and were excluded from further analysis.)
Imaging Results
We compared the two groups in terms of BOLD signal differences between delayed choices and immediate choices. Group comparison suggested that the IGD showed smaller BOLD signal differences, between delayed and immediate choice, over the left DLPFC and bilateral IFG than HC (Figure 4 and Table 2), which was consistent with our hypothesis. Nevertheless, the IGD did not show any greater BOLD signals in the whole brain compared to HC. In each group, the IGD showed greater brain activations in the anterior cingulate gyrus and lower brain activations in the left IFG and medial frontal gyrus for delayed choices than immediate choices; the HC showed greater brain activations in the right IFG, orbital gyrus, and middle frontal gyrus for delayed choices than immediate choices (Figure 5 and Table 3).
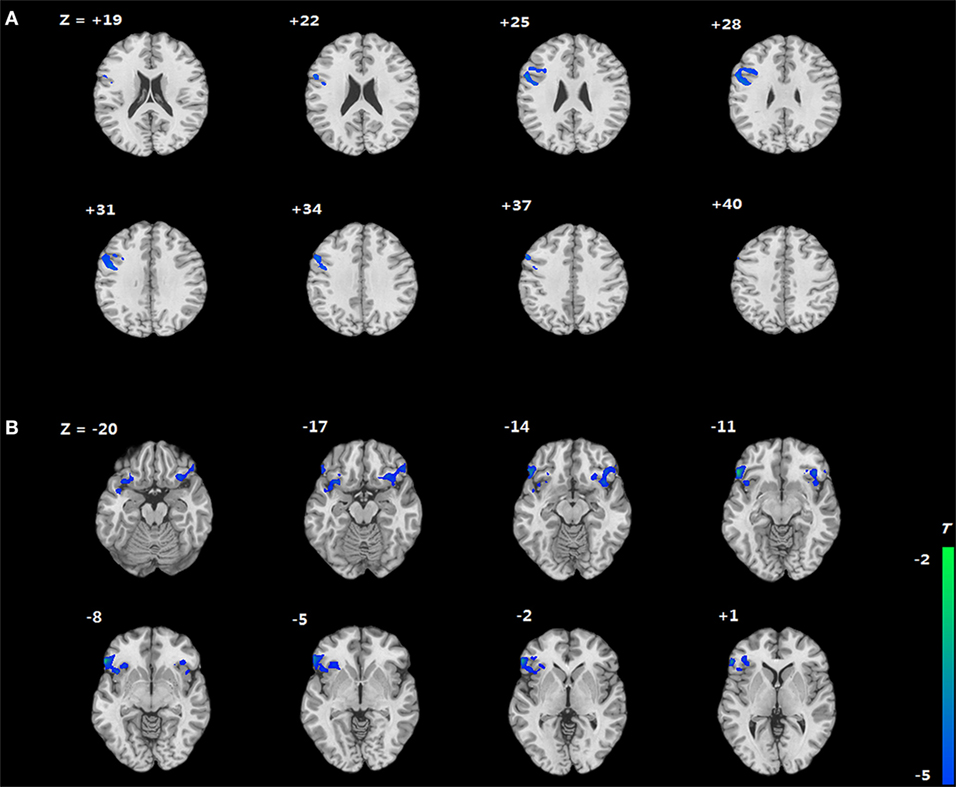
Figure 4. Brain areas showing differences in Internet gaming disorder (IGD) when comparing to healthy control (HC) [(IGDdelay − IGDimmediate) − (HCdelay − HCimmediate)]. (A) IGD show lower brain activation in left dorsolateral prefrontal cortex than HC. (B) IGD show lower brain activation in bilateral IFG than HC.
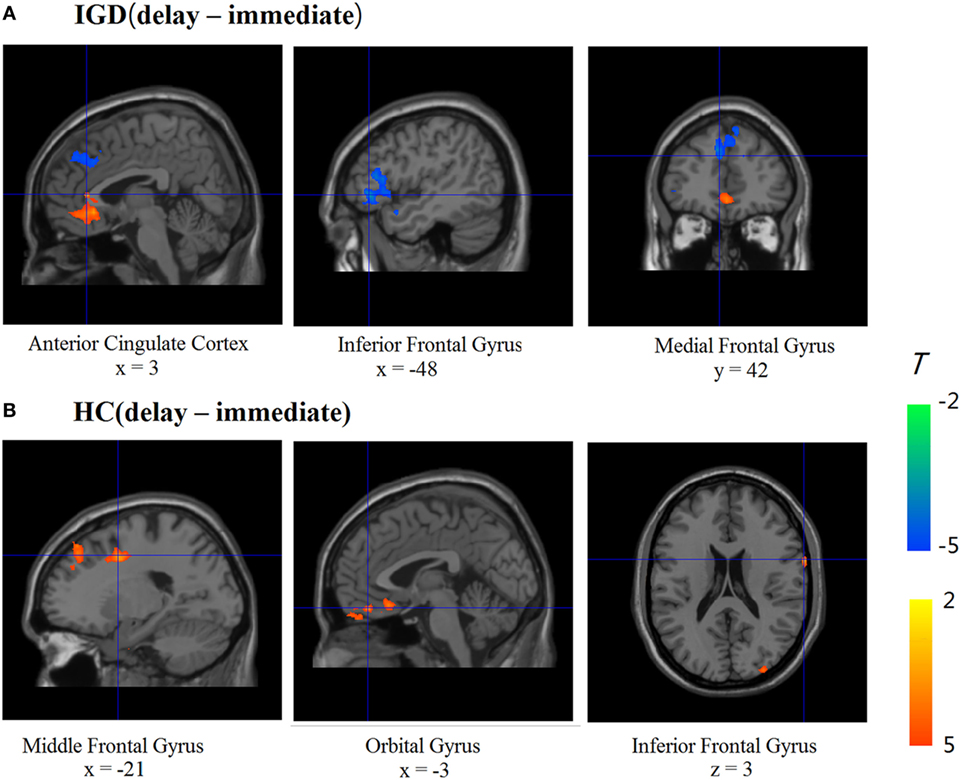
Figure 5. Brain activations change between different conditions in Internet gaming disorder (IGD) and healthy control (HC) (delay − immediate). (A) The IGD showed greater brain activation in the ACC and lower brain activations in the left inferior frontal gyrus (IFG) and medial frontal gyrus (delay > immediate). (B) The HC showed greater brain activations in the right IFG, orbital gyrus, and middle frontal gyrus (delay > immediate).
Correlation Results
The correlations between beta values and behavioral performance were analyzed within each group. The brain activations in the DLPFC and bilateral IFG were all significantly positively correlated with the log k values in both groups (see the results in Figure 6), and the correlation between beta value in the DLPFC and log k in the two groups was significantly different by a Fisher’s Z test (z = 2.44, p < 0.05). In IGD group, the brain activations in the bilateral IFG (delay − immediate) were positively correlated with the severity of IGD, but it did not reach the significant level (left IFG: r = 0.478, p = 0.061; right IFG: r = 0.480, p = 0.060; Figure 7); no significant correlations were found between brain activations and the severity of IGD in HC group (p > 0.1). In addition, there were no significant correlations between the brain activations and RT in each group (p > 0.1).
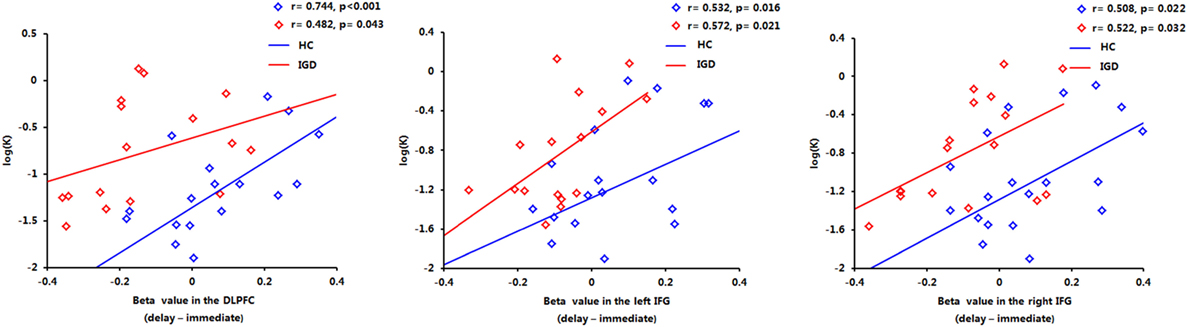
Figure 6. Positive correlations between the brain activations in the dorsolateral prefrontal cortex (DLPFC) and bilateral inferior frontal gyrus (IFG) and log k in both groups.
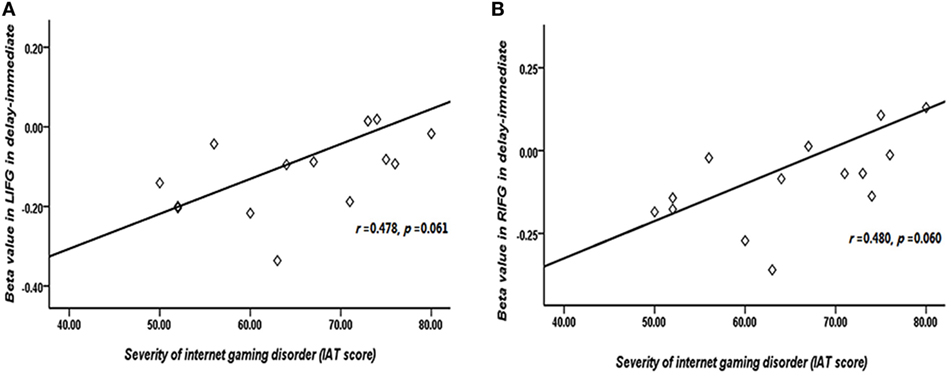
Figure 7. Correlation between the severity of Internet gaming disorder (IGD) and brain activations in the bilateral inferior frontal gyrus (IFG). (A) Correlation between peak left IFG activation (delay − immediate) and the severity of IGD. (B) Correlation between peak right IFG activation (delay − immediate) and the severity of IGD. (Scores that greater than 3 SDs were regarded as outliers and were excluded from further analysis.)
Discussion
Consistent with our hypotheses, the IGD showed higher discounting rate k and less brain activations than HC. The foregoing results indicated that the IGD group were more impulsive and might have deficient decision-making ability, which was in line with our previous study (42). In particular, we found that the left DLPFC and bilateral IFG were more deactivated in trials in which the IGD selected the delayed rewards compared to HC, which may provide evidences to further understand the mechanisms underlying IGD.
Deficient Ability in Evaluating the Delayed Reward in IGD
Compared with HC, IGD showed lower brain activations in the left DLPFC when choosing the delayed options. Consistent with this finding, Hoffman et al.’s study found that methamphetamine-dependent individuals exhibited lower activation in the DLPFC than that of HC in delayed decisions (43). According to the dual-system mode, the δ system, which included the DLPFC, was mainly used for weighting the delayed rewards (21, 22). Researchers have also found that the DLPFC primarily responds to the delays of delayed rewards, and the activation in the DLPFC is negatively related to increasing delay time (44). Specifically, there is evidence that the DLPFC plays a vital role in encoding the attributes of multiple reward predictions into an integrated value (45).
Thus, the relatively reduced brain activities in the DLPFC observed in IGD may indicate that IGD had potential deficits in evaluating the magnitudes and delays of rewards. They could not fully integrate all the information of choices, which would lead to a lower capability in decision-making, even with longer decision-making time. Furthermore, a resting-state study has identified that the individuals with IGD show reduced functional connectivity strength between the DLPFC and caudate, suggesting impaired effective modulation of the DLPFC on rewards (46), which are also observed in substance abuse populations (47). Another explanation for the results is that there may be a minimum activation threshold of the DLPFC for individuals to choose the delayed reward. The activation below the minimum threshold would connect with the decisions for the immediate reward rather than the delayed one. Because the IGD have a lower activation of the DLPFC, they reach the minimum threshold at shorter delays than HC.
In addition, the RT was positively correlated with the severity of IGD, indicating that the more serious the IGD was, the longer time they needed to make choices. The correlation findings supported the explanation that the IGD showed deficient evaluating ability of the delayed features to some extent. To sum up, we inferred that the IGD unconsciously focused on the short-term gains, which might be associated with the poor reward evaluation ability.
Impaired Impulse Inhibition in Decision-Making in IGD
Apart from for the known role in reward processing, the DLPFC, as the highest-order association area, is also responsible for executive functions such as response inhibition and multi-attribute decision-making (48, 49). Especially, studies have proved that the activity in the DLPFC will enhance when individuals exercise self-control (50). Moreover, reduced brain activation of the IFG was also observed in IGD during the inhibition processing in the present research. It has been noted that the IFG is involved in cognitive control and impulse inhibition (51, 52). Moreover, the IFG is responsible for self-control and inhibition of prepotent responses for giving up immediate gratification and seeking for long-term interests (53–55). Critically, the IFG has also been identified as a crucial structure in the process of establishing flexible association between outcomes and advantageous actions (56). In general, the DLPFC and IFG play essential roles in the deployment of self-control and impulse inhibition. In this study, the lower BOLD signal in the bilateral IFG and DLPFC may reflect that the impaired ability for the IGD to control their behaviors and inhibit their impulse.
The altered brain activities in the DLPFC and IFG have been reported in previous researches, which reveal the low capacity of impulse inhibition in response to immediate rewards in IGD. Probabilistic discounting task have detected that the IGD exhibited high level of impulsivity and diminished BOLD signal in the IFG than both HC and recreational gaming users (18, 57). During risky decision-making, the IGD showed altered modulation of the bilateral DLPFC when taking risky choices (58). Moreover, we also found that the brain activations in the DLPFC and bilateral IFG were positively correlated with the log k values, suggesting that the IGD with greater activation local to the DLPFC and IFG was more impulsive. Although attributed to extracognitive endeavor by the prefrontal activation, the IGD cannot effectively control themselves to choose the delayed reward in the selection process.
In addition, positive correlation was found between the severity of IGD and the log k values, suggesting individuals with IGD who showed more severe IGD symptoms were also more impulsive. Another positive correlation between the severity of IGD and brain activation in the bilateral IFG might indicate that the more severe the IGD was, the more endeavors they needed to engage in selecting delayed decisions. What’s more, impaired executive control and reward circuit have been detected in IGD (42), which is parallel with our findings. Taken all into consideration, the results suggested that the IGD demonstrated deficient ability in reward evaluation and impulse inhibition, which might be associated with the dysfunction of the prefrontal activation. These findings are consistent with a prior meta-analysis of fMRI studies, implicating that dysfunctional prefrontal activation plays an important role of in the neurobiological mechanism of IGD (59).
Limitations
There were several limitations ought to be noted. First, only male participants were recruited in this study, thus further studies should shed light on female participants. Second, to ease the difficulty of the tasks and let participants concentrate on the decision-making process, we did not balance the positions of the immediate options and delayed options, which might potentially bias the results.
Conclusion
In summary, this study suggested that IGD showed steeper discounting rate and altered brain activities in the DLPFC and IFG. The mechanism might lie in their impairment in both evaluating the delayed reward and impulse inhibition ability in decision-making, which was associated with the dysfunction of prefrontal function. This could be a reason why they prefer immediate satisfaction to larger delayed rewards. More broadly, our research findings also provide insights into the reason why IGD continue playing online games even when they are faced with severe negative consequences caused by excessive engagement in Internet games.
Ethics Statement
The experiment conforms to The Code of Ethics of the World Medical Association (Declaration of Helsinki). The Human Investigations Committee of Zhejiang Normal University approved this research. All subjects signed the informed consent forms before the experiment.
Author Contributions
YW contributed to experimental programming, data collection and data analyses and wrote the first draft of the manuscript. GD designed this research. YH and GD revised and improved the manuscript. JX, HZ, XL, and XD contributed to experimental programming, and data collection. All authors contributed to and have approved the final manuscript.
Conflict of Interest Statement
The authors declare that the research was conducted in the absence of any commercial or financial relationships that could be construed as a potential conflict of interest.
Acknowledgments
This research was supported by National Science Foundation of China (31371023).
Funding
The funders had no role in study design, data collection and analysis, decision to publish, or preparation of the manuscript.
Supplementary Material
The Supplementary Material for this article can be found online at http://www.frontiersin.org/article/10.3389/fpsyt.2017.00287/full#supplementary-material.
Footnotes
References
1. Király O, Nagygyörgy K, Griths MD, Demetrovics Z. Problematic online gaming. Behavioral Addictions. New York, NY: Elsevier (2014).
2. Ko CH. Internet gaming disorder. Curr Addict Rep (2014) 1(1):177–85. doi:10.1007/s40429-014-0030-y
3. Ayas T, Horzum MB. Relation between depression, loneliness, self-esteem and internet addiction. Education (2013) 133:283–90.
4. Choi SW, Kim HS, Kim GY, Jeon Y, Park SM, Lee JY, et al. Similarities and differences among Internet gaming disorder, gambling disorder and alcohol use disorder: a focus on impulsivity and compulsivity. J Behav Addict (2014) 3(4):246. doi:10.1556/JBA.3.2014.4.6
5. Dong GH, Potenza MN. A cognitive-behavioral model of Internet gaming disorder: theoretical underpinnings and clinical implications. J Psychiatr Res (2014) 58:7–11. doi:10.1016/J.Jpsychires.2014.07.005
6. American Psychiatric Association. Diagnostic and Statistical Manual of Mental Disorders. 5th ed. Washington, DC: American Psychiatric Pub (2013).
7. Griffiths MD, King DL, Demetrovics Z. DSM-5 internet gaming disorder needs a unified approach to assessment. Neuropsychiatry (2014) 4(1):1–4. doi:10.2217/npy.13.82
8. Petry NM, O’Brien CP. Internet gaming disorder and the DSM-5. Addiction (2013) 108(7):S62. doi:10.1111/add.12162
9. Green L, Fry AF, Myerson J. Discounting of delayed rewards: a life-span comparison. Psychol Sci (1994) 5(1):33–6. doi:10.1111/j.1467-9280.1994.tb00610.x
10. Rachlin H, Raineri A, Cross D. Subjective probability and delay. J Exp Anal Behav (1991) 55(2):233–44. doi:10.1901/jeab.1991.55-233
11. Ainslie G. Specious reward: a behavioral theory of impulsiveness and impulse control. Psychol Bull (1975) 82(4):463–96. doi:10.1037/h0076860
12. Petry NM, Kirby KN, Kranzler HR. Effects of gender and family history of alcohol dependence on a behavioral task of impulsivity in healthy subjects. J Stud Alcohol Drugs (2002) 63(1):83–90.
13. Kirby KN, Petry NM, Bickel WK. Heroin addicts have higher discount rates for delayed rewards than non-drug-using controls. J Exp Psychol Gen (1999) 128(1):78. doi:10.1037/0096-3445.128.1.78
14. Heil SH, Johnson MW, Higgins ST, Bickel WK. Delay discounting in currently using and currently abstinent cocaine-dependent outpatients and non-drug-using matched controls. Addict Behav (2006) 31(7):1290–4. doi:10.1016/j.addbeh.2005.09.005
15. Hoffman WF, Moore M, Templin R, McFarland B, Hitzemann RJ, Mitchell SH. Neuropsychological function and delay discounting in methamphetamine-dependent individuals. Psychopharmacology (2006) 188(2):162–70. doi:10.1007/s00213-006-0494-0
16. Miedl SF, Peters J, Büchel C. Altered neural reward representations in pathological gamblers revealed by delay and probability discounting. Arch Gen Psychiatry (2012) 69(2):177–86. doi:10.1001/archgenpsychiatry.2011.1552
17. Saville BK, Gisbert A, Kopp J, Telesco C. Internet addiction and delay discounting in college students. Psychol Rec (2010) 60(2):273–86. doi:10.1007/BF03395707
18. Wang Y, Wu L, Wang L, Zhang Y, Du X, Dong G. Impaired decision-making and impulse control in Internet gaming addicts: evidence from the comparison with recreational Internet game users. Addict Biol (2017) 22:1610–21. doi:10.1111/adb.12458
19. Dong G, Li H, Wang L, Potenza MN. Cognitive control and reward/loss processing in Internet gaming disorder: results from a comparison with recreational Internet game-users. Eur Psychiatry (2017) 44:30. doi:10.1016/j.eurpsy.2017.03.004
20. Dong G, Wang L, Du X, Potenza MN. Gaming increases craving to gaming-related stimuli in individuals with Internet gaming disorder. Biol Psychiatry Cogn Neurosci Neuroimaging (2017) 2(5):404–12. doi:10.1016/j.bpsc.2017.01.002
21. McClure SM, Ericson KM, Laibson DI, Loewenstein G, Cohen JD. Time discounting for primary rewards. J Neurosci (2007) 27(21):5796–804. doi:10.1523/JNEUROSCI.4246-06.2007
22. McClure SM, Laibson DI, Loewenstein G, Cohen JD. Separate neural systems value immediate and delayed monetary rewards. Science (2004) 306(5695):503–7. doi:10.1126/science.1100907
23. Ledgerwood DM, Knezevic B, White R, Khatib D, Petry NM, Diwadkar VA. Monetary delay discounting in a behavioral addiction sample: an FMRI pilot study. Drug Alcohol Depend (2014) 140:e117–8. doi:10.1016/j.drugalcdep.2014.02.336
24. Claus ED, Kiehl KA, Hutchison KE. Neural and behavioral mechanisms of impulsive choice in alcohol use disorder. Alcohol Clin Exp Res (2011) 35(7):1209–19. doi:10.1111/j.1530-0277.2011.01455.x
25. Luijten M, O’Connor DA, Rossiter S, Franken IHA, Hester R. Effects of reward and punishment on brain activations associated with inhibitory control in cigarette smokers. Addiction (2013) 108(11):1969–78. doi:10.1111/add.12276
26. Hampshire A, Chamberlain SR, Monti MM, Duncan J, Owen AM. The role of the right inferior frontal gyrus: inhibition and attentional control. Neuroimage (2010) 50(3):1313–9. doi:10.1016/j.neuroimage.2009.12.109
27. Menon V, Uddin LQ. Saliency, switching, attention and control: a network model of insula function. Brain Struct Funct (2010) 214(5–6):655–67. doi:10.1007/s00429-010-0262-0
28. Staudinger MR, Erk S, Walter H. Dorsolateral prefrontal cortex modulates striatal reward encoding during reappraisal of reward anticipation. Cereb Cortex (2011) 21(11):2578–88. doi:10.1093/cercor/bhr041
29. Wang Y, Yin Y, Sun YW, Zhou Y, Chen X, Ding WN, et al. Decreased prefrontal lobe interhemispheric functional connectivity in adolescents with internet gaming disorder: a primary study using resting-state FMRI. PLoS One (2015) 10(3):e0118733. doi:10.1371/journal.pone.0118733
30. Dong G, Potenza MN. Risk-taking and risky decision-making in Internet gaming disorder: implications regarding online gaming in the setting of negative consequences. J Psychiatr Res (2016) 73(1):1–8. doi:10.1016/j.jpsychires.2015.11.011
31. Liu GC, Yen JY, Chen CY, Yen CF, Chen CS, Lin WC, et al. Brain activation for response inhibition under gaming cue distraction in internet gaming disorder – The Kaohsiung journal of medical sciences. Kaohsiung J Med Sci (2014) 30(1):43–51. doi:10.1016/j.kjms.2013.08.005
32. Zhang Y, Lin X, Zhou H, Xu J, Du X, Dong G. Brain activity toward gaming-related cues in Internet gaming disorder during an addiction stroop task. Front Psychol (2016) 7(364):714. doi:10.3389/fpsyg.2016.00714
33. Young KS. Internet addiction: the emergence of a new clinical disorder. Cyberpsychol Behav (1998) 1(3):237–44. doi:10.1089/cpb.1998.1.237
34. Petry NM, Rehbein F, Gentile DA, Lemmens JS, Rumpf HJ, Mossle T, et al. An international consensus for assessing internet gaming disorder using the new DSM-5 approach. Addiction (2014) 109(9):1399–406. doi:10.1111/Add.12457
35. Young KS. Internet Addiction Test (IAT). (2009). Available from http://netaddiction.com/index.php?option=combfquiz&view=onepage&catid=46&Itemid=106
37. Evenden JL. Varieties of impulsivity. Psychopharmacology (1999) 146(4):348–61. doi:10.1007/PL00005481
38. Monterosso J, Ainslie G. Beyond discounting: possible experimental models of impulse control. Psychopharmacology (1999) 146(4):339–47. doi:10.1007/PL00005480
39. Richards JB, Zhang L, Mitchell SH, Wit H. Delay or probability discounting in a model of impulsive behavior: effect of alcohol. J Exp Anal Behav (1999) 71(2):121–43. doi:10.1901/jeab.1999.71-121
40. Mitchell SH. Measures of impulsivity in cigarette smokers and non-smokers. Psychopharmacology (1999) 146(4):455–64. doi:10.1007/PL00005491
41. Reynolds B, Richards JB, Horn K, Karraker K. Delay discounting and probability discounting as related to cigarette smoking status in adults. Behav Processes (2004) 65(1):35–42. doi:10.1016/S0376-6357(03)00109-8
42. Wang Y, Wu L, Zhou H, Lin X, Zhang Y, Du X, et al. Impaired executive control and reward circuit in Internet gaming addicts under a delay discounting task: independent component analysis. Eur Arch Psychiatry Clin Neurosci (2017) 267:245–55. doi:10.1007/s00406-016-0721-6
43. Hoffman WF, Schwartz DL, Huckans MS, McFarland BH, Meiri G, Stevens AA, et al. Cortical activation during delay discounting in abstinent methamphetamine dependent individuals. Psychopharmacology (2008) 201(2):183–93. doi:10.1007/s00213-008-1261-1
44. Ballard K, Knutson B. Dissociable neural representations of future reward magnitude and delay during temporal discounting. Neuroimage (2009) 45(1):143–50. doi:10.1016/j.neuroimage.2008.11.004
45. Kahnt T, Heinzle J, Park SQ, Haynes JD. Decoding different roles for vmPFC and dlPFC in multi-attribute decision making. Neuroimage (2011) 56(2):709–15. doi:10.1016/j.neuroimage.2010.05.058
46. Yuan K, Yu D, Cai C, Feng D, Li Y, Bi Y, et al. Frontostriatal circuits, resting state functional connectivity and cognitive control in internet gaming disorder. Addict Biol (2017) 22:813–22. doi:10.1111/adb.12348
47. Tomasi D, Volkow ND. Striatocortical pathway dysfunction in addiction and obesity: differences and similarities. Crit Rev Biochem Mol Biol (2013) 48(1):1–19. doi:10.3109/10409238.2012.735642
48. Steinbeis N, Bernhardt B, Singer T. Impulse control and underlying functions of the left DLPFC mediate age-related and age-independent individual differences in strategic social behavior. Neuron (2012) 73(5):1040–51. doi:10.1016/j.neuron.2011.12.027
49. Zysset S, Wendt CS, Volz KG, Neumann J, Huber O, von Cramon DY. The neural implementation of multi-attribute decision making: a parametric fMRI study with human subjects. Neuroimage (2006) 31(3):1380–8. doi:10.1016/j.neuroimage.2006.01.017
50. Hare TA, Camerer CF, Rangel A. Self-control in decision-making involves modulation of the vmPFC valuation system. Science (2009) 324(5927):646–8. doi:10.1126/science.1168450
51. Brown MR, Lebel RM, Dolcos F, Wilman AH, Silverstone PH, Pazderka H, et al. Effects of emotional context on impulse control. Neuroimage (2012) 63(1):434–46. doi:10.1016/j.neuroimage.2012.06.056
52. Tops M, Boksem MAS. A potential role of the inferior frontal gyrus and anterior insula in cognitive control, brain rhythms, and event-related potentials. Front Psychol (2011) 2:330. doi:10.3389/fpsyg.2011.00330
53. Aron AR, Monsell S, Sahakian BJ, Robbins TW. A componential analysis of task-switching deficits associated with lesions of left and right frontal cortex. Brain (2004) 127(7):1561–73. doi:10.1093/brain/awh169
54. Garavan H, Ross TJ, Murphy K, Roche RAP, Stein EA. Dissociable executive functions in the dynamic control of behavior: inhibition, error detection, and correction. Neuroimage (2002) 17(4):1820–9. doi:10.1006/nimg.2002.1326
55. Menon V, Adleman NE, White CD, Glover GH, Reiss AL. Error-related brain activation during a Go/NoGo response inhibition task. Hum Brain Mapp (2001) 12(3):131–43. doi:10.1002/1097-0193(200103)12:3<131::AID-HBM1010>3.0.CO;2-C
56. Ernst M, Paulus MP. Neurobiology of decision making: a selective review from a neurocognitive and clinical perspective. Biol Psychiatry (2005) 58(8):597–604. doi:10.1016/j.biopsych.2005.06.004
57. Lin X, Zhou H, Dong G, Du X. Impaired risk evaluation in people with Internet gaming disorder: fMRI evidence from a probability discounting task. Prog Neuropsychopharmacol Biol Psychiatry (2015) 56:142–8. doi:10.1016/j.pnpbp.2014.08.016
58. Liu L, Xue G, Potenza MN, Zhang JT, Yao YW, Xia CC, et al. Dissociable neural processes during risky decision-making in individuals with Internet-gaming disorder. Neuroimage Clin (2017) 14:741. doi:10.1016/j.nicl.2017.03.010
Keywords: Internet gaming disorder, decision-making, delay discounting task, dorsolateral prefrontal cortex, inferior frontal gyrus
Citation: Wang Y, Hu Y, Xu J, Zhou H, Lin X, Du X and Dong G (2017) Dysfunctional Prefrontal Function Is Associated with Impulsivity in People with Internet Gaming Disorder during a Delay Discounting Task. Front. Psychiatry 8:287. doi: 10.3389/fpsyt.2017.00287
Received: 14 August 2017; Accepted: 01 December 2017;
Published: 13 December 2017
Edited by:
Jintao Zhang, Beijing Normal University, ChinaReviewed by:
Gilly Koritzky, Argosy University, United StatesBernardo Barahona-Correa, Nova Medical School – Faculdade de Ciências Médicas, Portugal
Copyright: © 2017 Wang, Hu, Xu, Zhou, Lin, Du and Dong. This is an open-access article distributed under the terms of the Creative Commons Attribution License (CC BY). The use, distribution or reproduction in other forums is permitted, provided the original author(s) or licensor are credited and that the original publication in this journal is cited, in accordance with accepted academic practice. No use, distribution or reproduction is permitted which does not comply with these terms.
*Correspondence: Guangheng Dong, dongguangheng@zjnu.edu.cn